4.8 DIAGNOSTIC TESTS
In econometrics analysis the diagnostic tests are important to
check whether the assumptions of tradition regression are confirmed.
These tests included Normal distribution test,
Heteroscedasticity test, Autocorrelation test and
Stability test.
4.8.1 NORMALITY TEST
The hypothesis test is as follow
Ho: The residuals are normal distributed
H1 : The residuals are not normal distributed
The null hypothesis is rejected at 10% level of significant
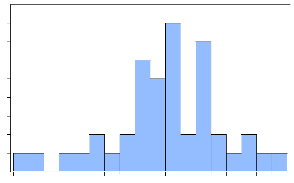
4
2
9
8
7
6
5
3
1
Series: Residuals
Sample 2007Q1 2017Q4
Observations 44
Mean
Median
Maximum
Minimum
Skewness
Kurtosis
Jarque-Bera
Probability
|
|
P a g e 40 | 48
Figure 1: Normality test results
Source: Author's computation (2019) by Eviews
8
Std. Dev.
2.88e-16 0.003337 0.071187
-0.094099 0.036401 -0.499066
3.414288
2.141154
0.342811
Since the p-value 0.3462811 is greater than 10% level of
significance, we fail to reject the null hypothesis that the error term is
normally distributed at 95 % confidence interval and conclusion made that the
error term is normally distributed.
4.8.2 SERIAL CORRELATION LM TEST
Table 12: Serial correlation LM Test
Breusch-Godfrey Serial Correlation LM Test:
F-statistic 1.188804 Prob. F(1,35) 0.2830
Obs*R-squared 1.379702 Prob. Chi-Square(1) 0.2402
Source: Eviews 7,2019
Table 4.10 reports the results of the first diagnostic test of
autocorrelation. The null hypothesis (Ho) claims that there is no
autocorrelation while the alternative hypothesis (H1) claims the opposite. The
decision rule states that the null hypothesis (H0) should be rejected if the
p-value of observed R-squared is less than the 0.05 level of significance.
Hence, there is no presence of serial correlation in the estimated model, since
the p-value of the observed R-squared is 0.2402 which is greater than the 0.05
level of significance.
4.8.3 HETEROSCEDASTICITY TEST
Table 13: Heteroscedasticity Test result
Heteroscedasticity Test: Breusch-Pagan-Godfrey
F-statistic
|
0.919697
|
Prob. F(8,33)
|
0.5129
|
Obs*R-squared
|
7.657008
|
Prob. Chi-Square(8)
|
0.4677
|
Scaled explained SS
|
19.22575
|
Prob. Chi-Square(8)
|
0.0137
|
Source: Eviews 7,2019
|
|
|
|
Table 4.13 shows the results of the second diagnostic test of
serial correlation. the null hypothesis (Ho) claims that residuals are
homoscedasticity and the alternative hypothesis claims that the residuals are
heteroscedastic and thus the variance is not constant. The rejection rule
states that the null hypothesis should be rejected if the probability value of
observation R-squared is less than the 0.05 level of significance. Since the
probability of Chi-Square of 0.2402 is greater than 0.05, the test fails to
reject the null hypothesis of constancy of variance among the residuals in the
model, and thus are deemed to be homoscedastic.
4.8.4 STABILITY OF THE MODEL
20 15 10 5
0 -5 -10 -15 -20
|
|
|
2009 2010 2011 2012 2013 2014 2015 2016 2017
|
|
|
|
|
CUSUM 5% Significance
|
|
|
|
|
|
|
Figure 4. 2 cumulative sum model stability
Source: Author's computation (2019) by Eviews
8
By analyzing the above graph, it was clear that the model is
stable because the navigating blue line of graph does not cross the borders
(the straight lines represent critical bounds at 5% significance level); this
indicates that the GDP of Rwanda have been moving in a stable way from 2007 to
2017
P a g e 41 | 48
|