III.Methodology and Data
IV.Analysis and Results
V.Limitations and further research needs
VI.Conclusion
12
VII.Recommendations
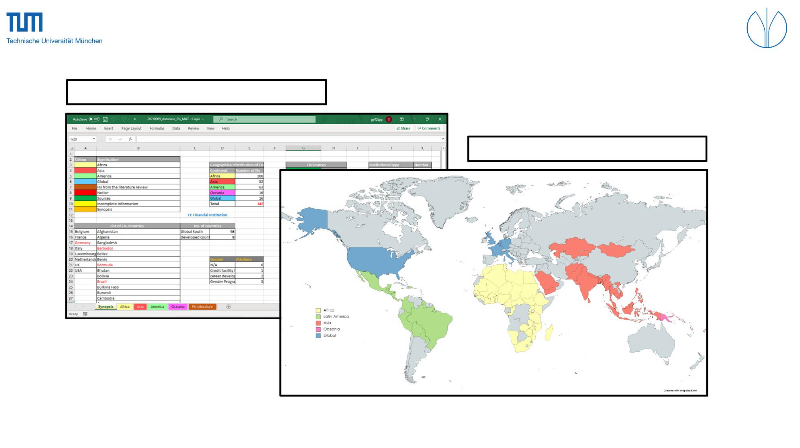
*** Description of the study ***
Database of financial institutions
Global Map of financial institutions
13
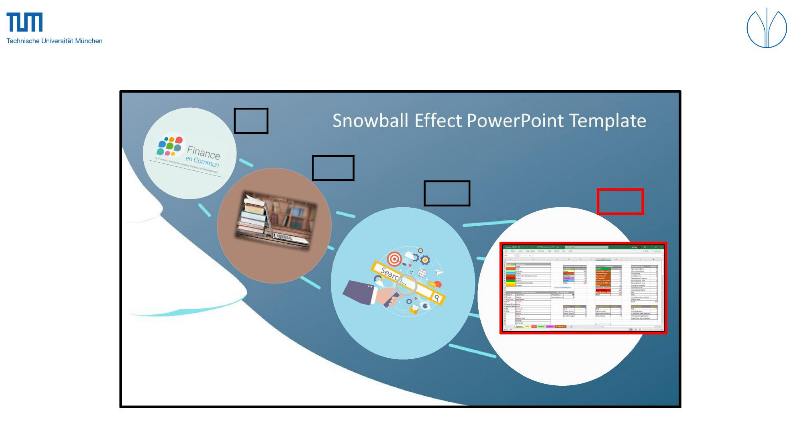
*** Building the database ***
37
201
109
347
14
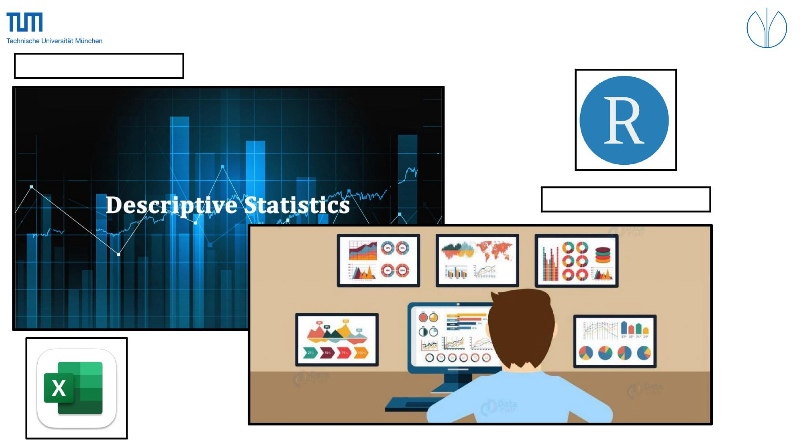
*** Statistical Analyses ***
Descriptive statistics
Cluster Analyses
15
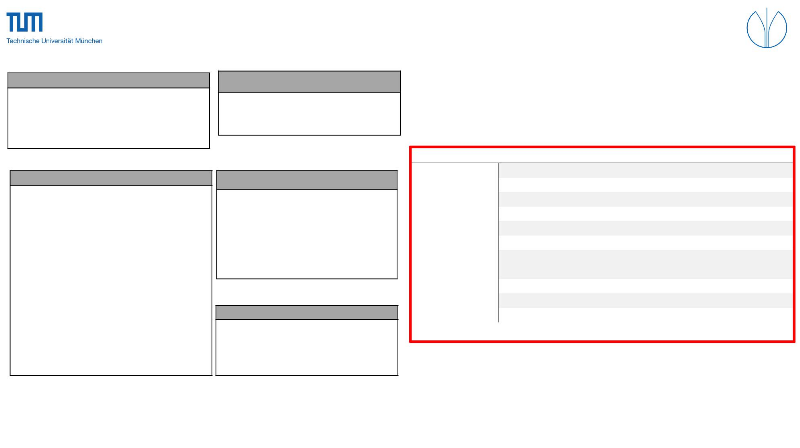
*** Data Types and Variables ***
Continent Attribute
Africa 0
Asia 1
South America 2
Global 3
Institutional type
|
Attribute
|
Agricultural Bank
|
0
|
Commercial Bank
|
1
|
cooperative
|
2
|
Credit union
|
3
|
Development Bank
|
4
|
Insurance company
|
5
|
International FI
|
6
|
International Fund
|
7
|
Islamic Bank
|
8
|
MFI
|
9
|
NGO
|
10
|
state development
bank
|
11
|
16
Agricultural loans Attribute
Credit facility for
women
Career development to female staff
Gender Programmes G3
G2
G1
Online Banking DS1
E-Products: DS2
Online Loan Application
DS3
Checking the rubric «Products and Services» AL1,
AL2 0-1
Gender Variables
Digital solution Variables
Farmer credit AL1
Agri-business credit AL2
Continent
Institutional Type
Foundation year
Number of Branches
Agricultural Loans
Gender
Digital solutions
Total assets
Total Equity
Gross Loan Portfolio
Variables Search Method Name/
Scale
checking the institute's website C 0-3
checking the rubric «about us» T 0-11
checking the rubric «our history» F Year
checking the rubric «locate us» B Number
Checking the rubric «our value» and
«events» G1, G2, G3 0-1
Verifying if «online banking» or other
e-products
DS1, DS2,
0-1
investigating the most recent «annual report»
AS $
available
DS3
investigating the most recent «annual report»
EQ $
investigating the most recent «annual report»
LP $
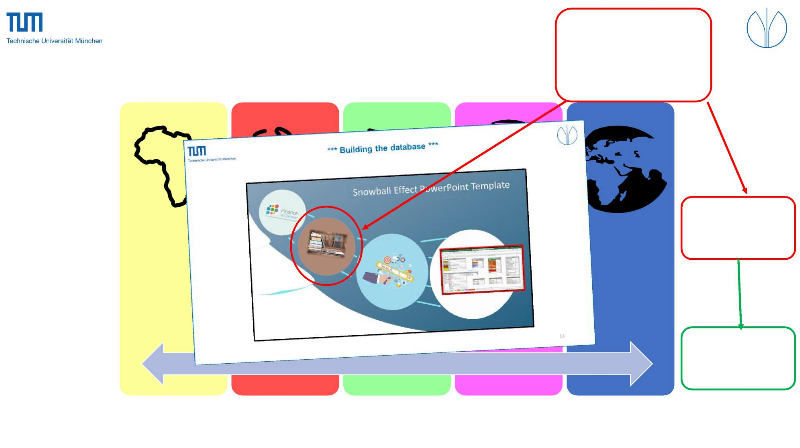
*** financial institutions sample ***
Financial institutions from the
literature review
201
Africa
Sample FIs: 200
Global
Sample FIs: 16
Total of 347 financial institutions
144
17

Plan
I.Introduction
II.Literature Review
III.Methodology and Data
IV.Analysis and Results
V.Limitations and further research needs
VI.Conclusion
18
VII.Recommendations
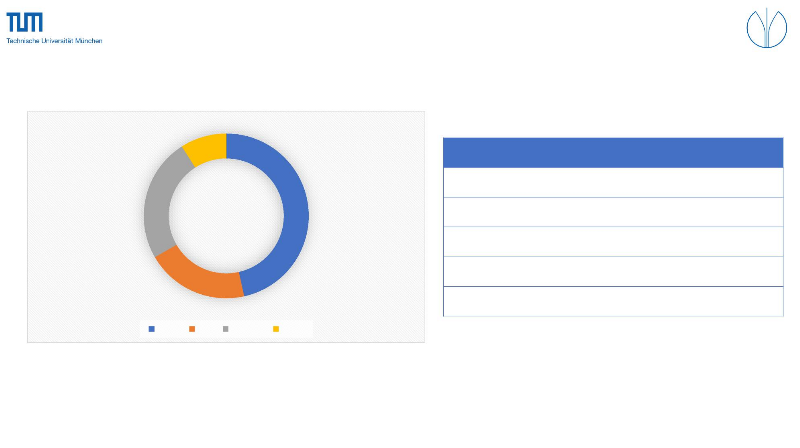
*** Geographic distribution ***
Global
9%
America
24%
Africa
47%
Asia
20%
Continent
|
|
No of FIs
|
Share
|
Africa
|
|
67
|
47%
|
South America
|
|
35
|
24%
|
Asia
|
|
29
|
20%
|
Global
|
|
13
|
9 %
|
|
Total
|
|
144
|
Africa Asia America Global
19
|
|
|
|
|
|
|
*** Distribution by institutional type ***
|
|
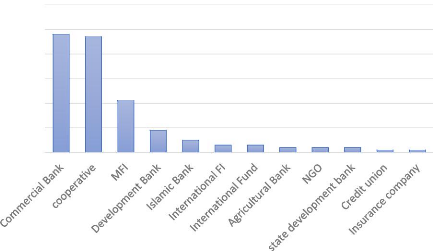
60
50
48 47
5 3 3 2 2 2 1 1
0
40
30
20
10
21
9
Institutional type Commercial Bank
|
Number
48
|
%
33%
|
Cooperative
|
47
|
33%
|
MFI
|
21
|
15%
|
Development Bank
|
9
|
6%
|
Islamic Bank
|
5
|
3%
|
International FI
|
3
|
2%
|
International Fund
|
3
|
2%
|
Agricultural Bank
|
2
|
1%
|
Credit union
|
1
|
1%
|
Insurance company
|
1
|
1%
|
NGOs
|
2
|
1%
|
State development bank
|
2
|
1%
|
Total
|
|
144
|
20
32
33
60
FREQUENCY
19
21
< 1950 1950 -1970 1970 -1990 > 1990
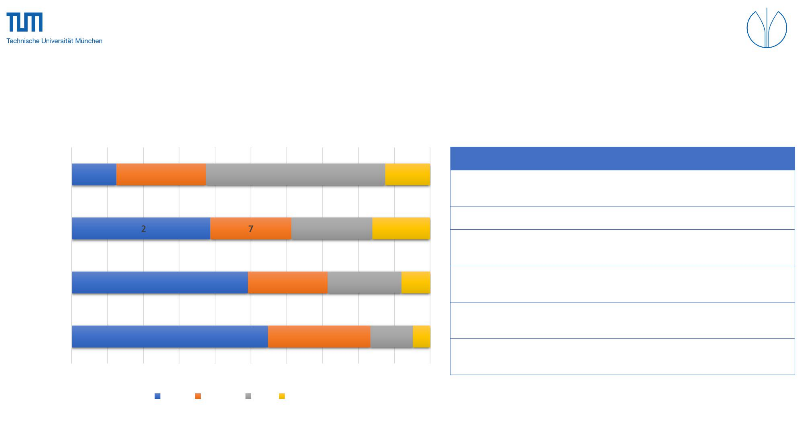
> 1000
100 - 1000
7
1
5
23
12
5
2
< 10
10 - 100
31
14
13
5
0% 10% 20% 30% 40% 50% 60% 70% 80% 90% 100%
Africa America Asia Global
1
2
4
1
*** Number of Branches per FIs ***
Number of Branches per Region
Range
|
Africa
|
Number of Branches per Region
America Asia Global Total
|
%
|
< 10
|
23
|
12
|
5
|
2
|
42
|
29%
|
10 -
100
|
31
|
14
|
13
|
5
|
63
|
44%
|
100 -
1000
|
12
|
7
|
7
|
5
|
31
|
22%
|
> 1000
|
1
|
2
|
4
|
1
|
8
|
6%
|
Grand Total
|
67
|
35
|
29
|
13
|
144
|
100%
|
22
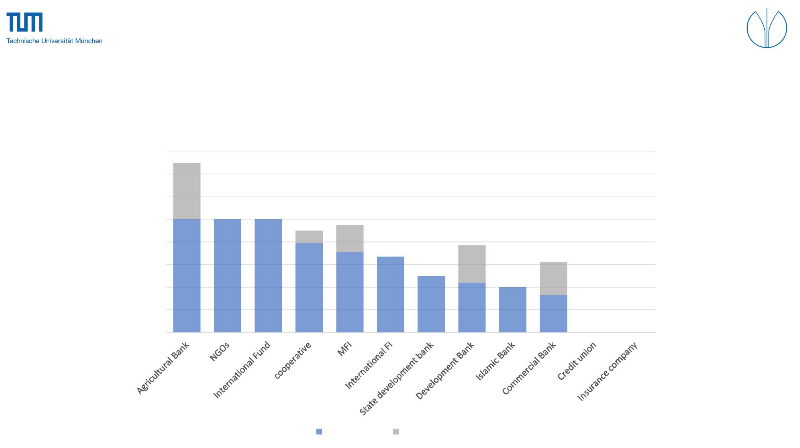
*** Agricultural loans***
160%
140%
120%
100%
80%
24%
0% 0%
50%
11%
20%
50%
0%
0% 0%
TYPE OF CREDIT OFFERED BY FINANCIAL
INSTITUTIONS
0%
44% 40%
Farmer credit Agribusiness Credit
60%
40%
79%
33%
0%
71%
67%
29%
0%
100%
100%
100%
33%
23
GENDER PROGRAMMES OFFERED BY FIS
160%
|
|
|
|
|
|
|
|
|
|
|
140%
|
|
|
|
|
|
|
|
|
|
|
120%
|
|
50%
|
|
|
|
|
|
|
|
|
100%
|
|
|
|
|
|
|
|
|
|
|
80%
|
|
|
33%
|
|
|
|
50%
|
|
|
|
|
60%
|
|
|
|
67%
|
29%
|
|
|
|
|
|
0%
|
|
33%
|
|
|
40%
|
|
|
|
|
19%
|
|
|
|
|
0%
|
|
|
50%
|
50%
|
|
|
|
20%
|
|
|
33%
|
33%
|
29%
|
0%
|
|
|
|
|
|
|
|
|
|
|
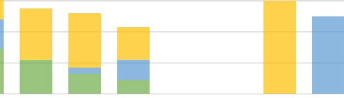
0%
60%
50%
4%
13%
22%
9%
13%
33%
35%
21%
0%
0% 0% 0% 0%
|
|
Credits facility for women Career development opportunities to
female staff Gender Programmes
|
24
|
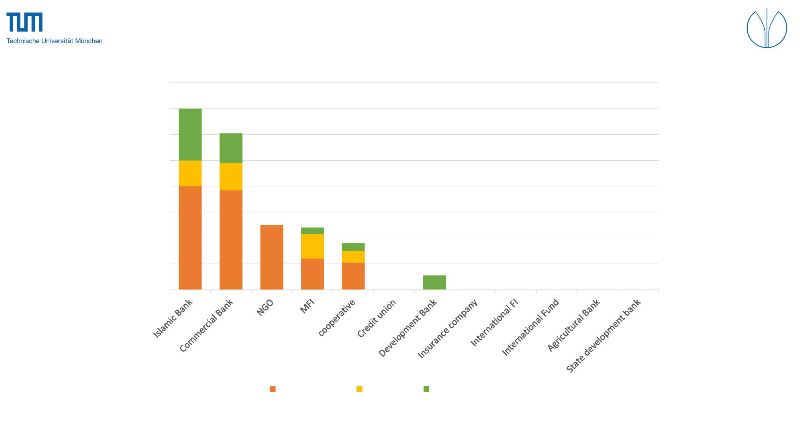
*** Digital Solutions ***
Digital solutions offered by FIs Again
40%
20%
80%
|
|
23%
21%
|
|
|
|
|
|
77%
|
|
|
|
|
5%
19%
|
|
0%
50%
|
|
|
|
6%
9%
|
24%
|
|
|
|
21%
|
0% 0% 0% 0% 0% 0% 0%
11%
160%
Online Banking E-Products Online Loan Applications
25
140%
120%
100%
80%
60%
40%
20%
0%
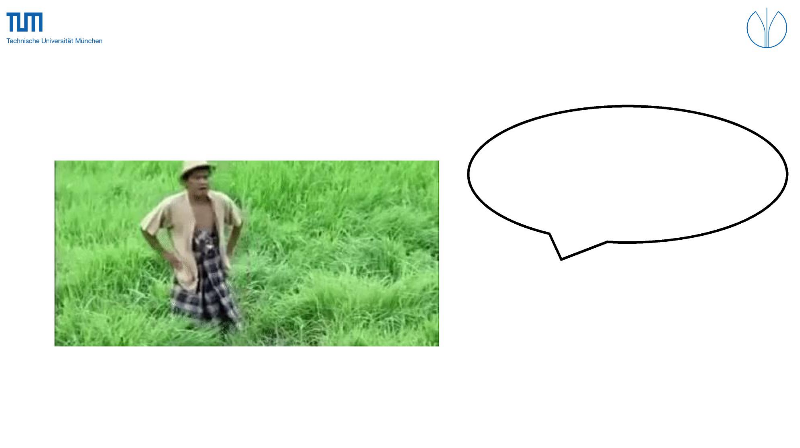
Plz sum it up !
26
Seven key characteristics of financial
institutions
1. Geographic distribution
2. institutional type
3. Foundation Year
4. Branches
5. Agricultural credit
6. Gender Equality
7. Digital solutions
1. Geographic distribution
47%
from Africa
24%
from America
4. Branches
29%
<10 branches
44%
10-100 branches
2. institutional type
33%
commercial bank
33%
cooperative
5. Agricultural credit
58%
Farmer credit
19%
Agribusiness credit
3. Foundation Year
42%
> 1990
6. Gender Equality
15% credit
facility for women
10% Career
development
7. Digital solutions
58% Online
27
banking
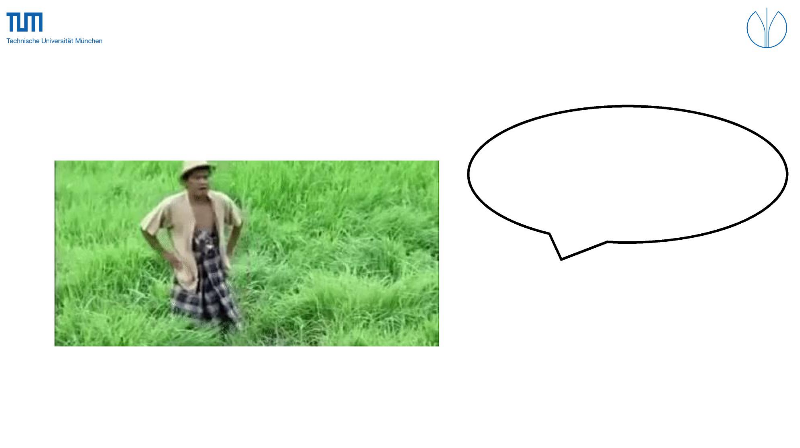
Okay descriptive Statistics ...
What about
cluster Analysis ?
28
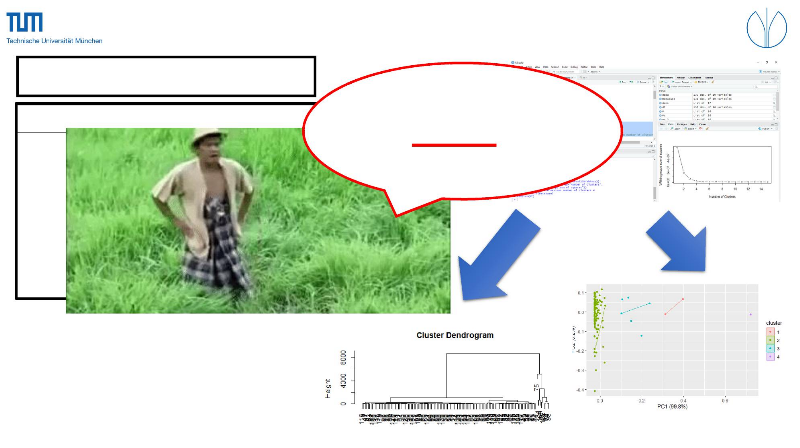
*** Cluster Analysis ***
Descriptive statistics of the dataset
Mean Median Standard
Range Mi
Sample
Variance
Deviation
C 0.96
T 3.44
F 1976
B 215.
AL1 0.58
AL2 0.19
G1 0.15
G2 0.10
G3 0.30
DS1 0.42
DS2 0.13
DS3 0.13
Wrong !
29
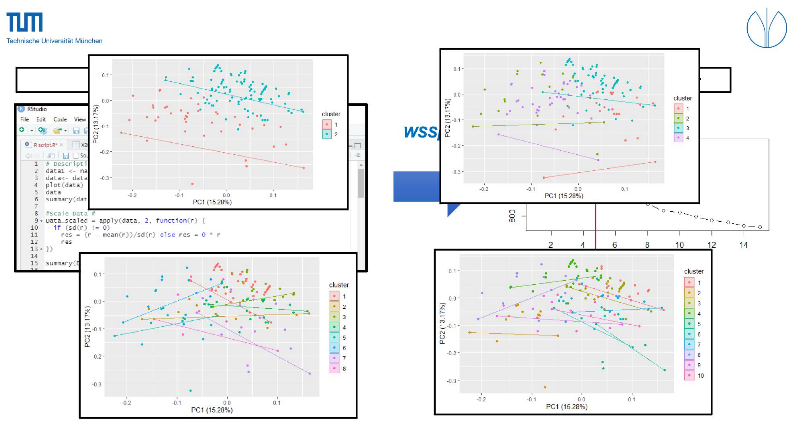
*** k-means Clustering ***
Scale Data
s
30
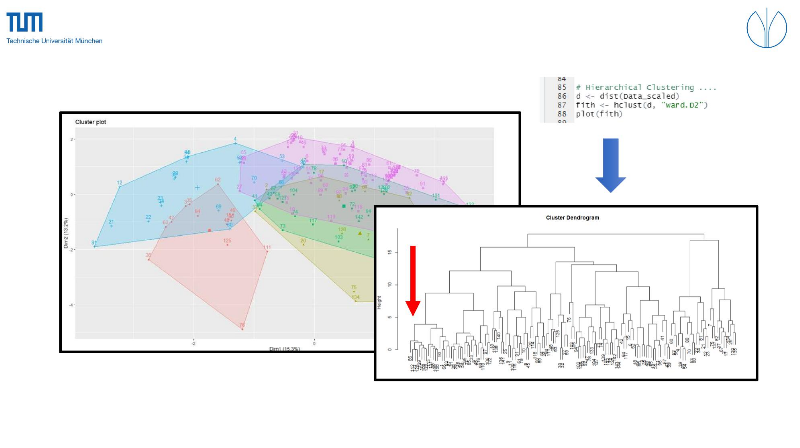
*** k-means VS hierarchical Clustering ***
31
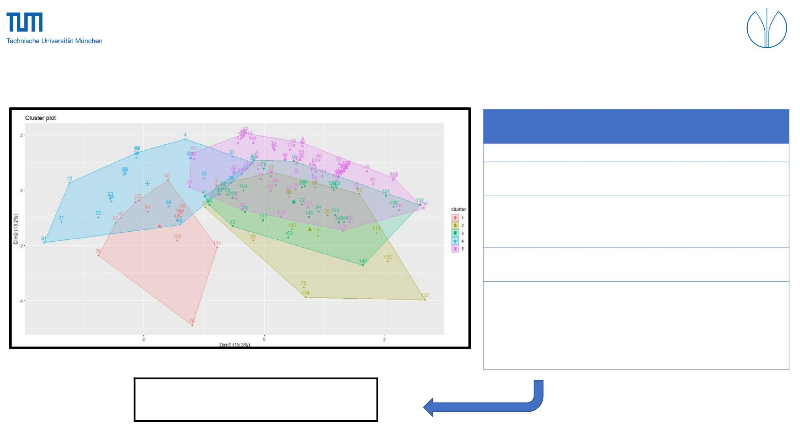
*** k-means Clustering ***
Cluster no.
1
|
Observations Number
3 18 35 36 42 48 49 50 62 63 76 84 111 125
|
Total
14
|
2
|
2 7 17 20 26 37 67 75 82 88 114 120 134 135
|
15
|
|
137
|
|
3
|
10 41 43 47 57 59 64 72 73 74 78 90 92 94 101
|
29
|
|
102 103 104 117 124 126 127 132 133 139 140
|
|
|
142 143 145
|
|
4
|
4 12 21 22 23 30 32 34 39 40 52 53 54 56 60 69
|
19
|
|
70 80 81
|
|
5
|
1 5 6 8 9 11 13 14 15 16 19 24 25 27 28 29 31
|
67
|
|
33 38 44 45 46 51 55 58 61 65 66 68 71 77 79
|
|
|
83 85 86 87 89 91 93 95 96 97 98 99 100 105
|
|
|
106 107 108 109 110 112 113 115 116 118 119
|
|
|
121 122 123 128 129 130 131 136 138 141
|
|
betweenss_totss (1? 10) Silhouette
plot
32
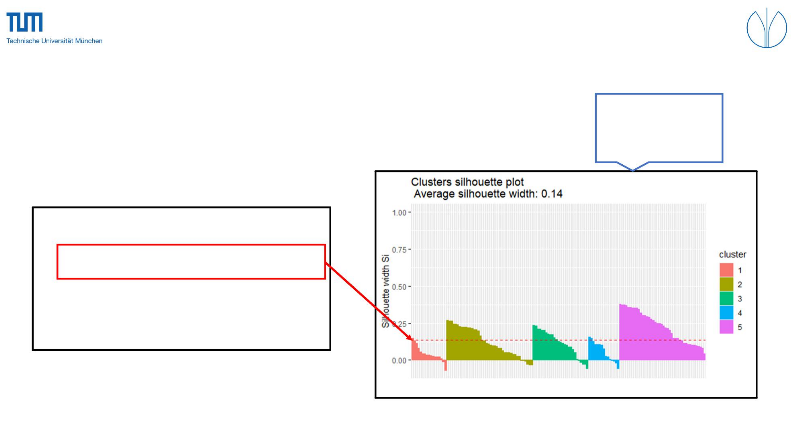
*** k-means Clustering ***
How good is 0.14?
Average silhouette width:
·
Si > 0 indicates that the observations are well
clustered.
· Si < 0 indicates that the observation was placed in
the wrong cluster.
· Si = 0 indicates that the observation is in between
two clusters
33
|
|
|
|
|
|
|
*** Extracting Results ***
|
|
34
Cluster Number
|
Average Cluster Name
Foundation
|
Average number of Branches
|
1
|
1948 Value chain oriented FIs
|
517
|
2
|
1967 Gender Staff FIs
|
433
|
3
|
1975 Farmer credit provider FIs
|
215
|
4
|
1990 Innovative digital newcomers
|
95
|
5
|
1982 Traditional Banking approaches
|
137
|
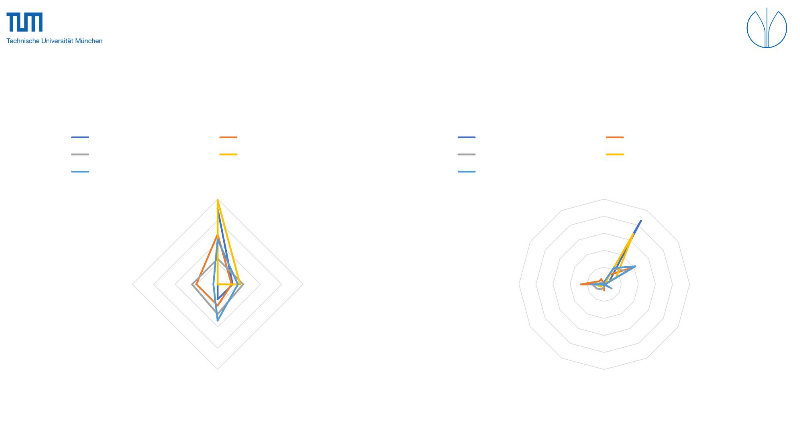
*** Extracting Results ***
Geographic distribution
Value chain oriented FIs Gender Staff FIs
Farmer credit provider FIs Innovative digital newcomers
Traditional Banking approaches
Africa
Institutional type
Value chain oriented FIs Gender Staff FIs
Farmer credit provider FIs Innovative digital newcomers
Traditional Banking approaches
Agricultural Bank
35
South America
International FI
80%
60%
40%
20%
0%
Asia
Global
state development
bank
100%
Commercial Bank
80%
NGO
60%
40%
20%
MFI
0%
Islamic Bank
cooperative
Credit union
Development Bank
International Fund
Insurance company
|
|
|
|
*** Extracting Results ***
|
|
Services
Value chain oriented FIs Gender Staff FIs
Farmer credit provider FIs Innovative digital newcomers
Traditional Banking approaches
Farmer credit
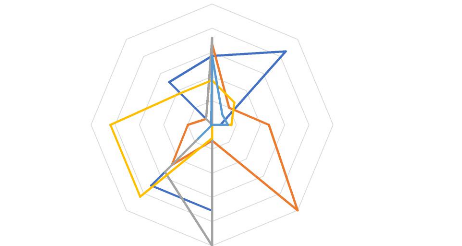
80%
Online Loan Applications
60%
40%
20%
E-Products
Online Banking
0%
agri-business credit
Credits facility for women
opportunities to female
staff
100%
36
Gender Programmes
|
*** Extracting Results***
|
|
Technische Universitet München
|
Table 30 Cluster's characteristics
Cluster Name
|
Group 1 Value chain Fls
|
Group 2 Gender Staff Fls
|
Group 3 Farmer credit provider Fls
|
Group 4
Innovative digital newcomers
|
Group 5
Traditional Banking approaches
|
Region
|
|
|
|
|
|
Africa
|
71%
|
47%
|
24%
|
79%
|
42%
|
Asia
|
14%
|
13%
|
24%
|
21%
|
19%
|
South America
|
14%
|
20%
|
28%
|
|
34%
|
Global
|
|
20%
|
24%
|
|
4%
|
Institutional Type
|
|
|
|
|
|
Agricultural Bank
|
|
|
|
|
3%
|
Commercial Bank
|
86%
|
13%
|
21%
|
68%
|
22%
|
cooperative
|
7%
|
40%
|
31%
|
16%
|
42%
|
Credit union
|
|
|
|
|
1%
|
Development Bank
|
7%
|
|
10%
|
|
7%
|
Insurance company
|
|
|
|
|
1%
|
International Fl
|
|
7%
|
3%
|
|
|
International Fund
|
|
|
7%
|
|
1%
|
Islamic Bank
|
|
|
10%
|
5%
|
1%
|
MFI
|
|
27%
|
14%
|
11%
|
16%
|
NGD
|
|
7%
|
3%
|
|
1%
|
Average Foundation
|
1940
|
1967
|
1975
|
1990
|
1982
|
Average Branches
|
517
|
433
|
215
|
95
|
137
|
Agricultural Credit
|
|
|
|
|
|
Farmer credit
|
57%
|
67%
|
72%
|
37%
|
57%
|
agri-business credit
|
86%
|
20%
|
0%
|
26%
|
12%
|
Gender Programmes
|
|
|
|
|
|
Credits facility for
women
opportunities to female staff
|
7%
0%
|
47%
100%
|
7%
0%
|
16%
0%
|
13%
0%
|
Gender Programmes
|
71%
|
13%
|
100%
|
11%
|
0%
|
Digital Solutions
|
|
|
|
|
|
Online Banking
|
71%
|
47%
|
55%
|
84%
|
16%
|
E-P rod ucts
|
0%
|
20%
|
0%
|
84%
|
0%
|
Online Loan
|
50%
|
7%
|
7%
|
37%
|
1%
|
Applications
|
|
|
|
|
|
Total Fls
|
14
|
15
|
29
|
19
|
67
|

Plan
I.Introduction
II.Literature Review
III.Methodology and Data
IV.Analysis and Results
|