Submitted at Freising, September 30, 2021
Technical University of Munich
The Role of Financial Institutions in
Value Chain Finance in the Global
South
Scientific work to obtain the degree
M.Sc. Agrarmanagement
At the Chair Group Agricultural Production and Resource Economics
of the TUM School of
Life Sciences.
Supervisors M.Sc. Roberto Villalba Camacho
Chair Group Agricultural Production and Resource Economics
M.Sc.Terese Venus
Chair Group Agricultural Production and Resource Economics
Examiner Prof. Dr. agr. Johannes Sauer
Chair Group Agricultural Production and Resource Economics
Submitted by Moahmed Ali Trabelsi
Matrikelnummer: 03703889
Giggenhausser str. 2985354, Freising +4915221671931
Declaration of authorship
I, Mohamed Ali Trabelsi, born in Tunis, Tunisia, with
matriculation number 03703889; declare that this thesis and the work presented
in it are my own. It has been generated as the result of my own original
research on the subject «The Role of Financial Institutions in Value Chain
Finance in the Global South»
I confirm that:
ü This work was done wholly in candidature for MSc
degree thesis fulfillment at the Technical University of Munich.
ü Where I have consulted published work of others, that
was always clearly credited.
ü Where I have taken some ideas from other sources, I
have mentioned the sources and except these kinds of quotes, the entire work is
mine.
Date 30.09.2021 Signature:

i
Table of Contents
Table of Contents ii
List of Figures v
List of Tables vi
List of Abbreviations vii
Acknowledgment viii
Abstract ix
1. Introduction 1
1.1. Problem statement 1
1.2. Objectives 2
1.3. Research questions and hypothesis 2
1.4. Expected results from the research 3
1.5. Organization of the thesis 3
2. Literature Review and Theoretical Background 4
2.1. Agriculture finance 4
2.1.1. Agricultural credit 4
2.1.2. Financial institutions (FIs) in the Agriculture Sector
6
2.1.3. Microfinance 7
2.2. AVCF definition 9
2.3. AVCF Challenges 11
2.4. Competitiveness of agricultural finance 14
2.5. Determinants of agricultural credit 17
2.6. Literature on AVCF 19
2.6.1. Gap of the Literature on AVCF 19
2.6.2. Available literature on AVCF 20
3. Methodology and Data 22
3.1. Description of the study 22
3.2. Building the database 23
3.3. Statistical Analyses 25
3.3.1. Qualitative Analyses: 25
3.3.2. Quantitative Analyses 25
3.3.3. Cluster Analysis 26
3.3.4. Data Types and Variables 27
3.3.5. Other components of the database 30
3.4. Structure of survey 30
ii
3.5. Sample Design 31
3.5.1. Sampling frame 31
3.5.2. Sampling techniques 32
3.5.3. FIs Listing: 33
4. Survey Design and Conceptual Framework 34
4.1. Exploring other surveys 34
4.1.1. Survey with FIs 34
4.1.2. Survey with farmers (World Bank & CGAP) 35
4.1.3. Integrated Financing for Value Chains (WOCCU) 37
4.1.4. Survey on national development bank (World Bank Group)
37
4.2. Credit Scoring for Agricultural Loans 38
4.3. Financial instruments employed by FIs 40
4.4. Survey design for FIs officials 41
4.4.1. General Information 42
4.4.2. Economic information 42
4.4.3. Credit screening, scoring, and monitoring for
Agricultural loans 42
4.4.4. Agricultural finance within value chains 43
4.4.5. Financial product & Instrument employed 43
4.5. Overview of the online questionnaire 43
5. Analysis and Results 45
5.1. Descriptive Analysis: 45
5.1.1. Geographic distribution: 45
5.1.2. Distribution by institutional type: 45
5.1.3. Foundation Year: 46
5.1.4. Number of Branches 47
5.1.5. Agricultural loans 47
5.1.6. Gender Equality 48
5.1.7. Digital Solutions 49
5.2. Cluster Analysis 50
5.2.1. Confirm Data: 50
5.2.2. Scale the data 51
5.2.3. Select segmentation variables 51
5.2.4. Define similarity measure: 51
5.2.5. Number of clusters 51
5.2.6. K-means Clustering Method 52
5.2.7. Hierarchical Clustering Method 54
5.2.8. Selected method and number of clusters 55
iii
5.2.9. Extracting Results 57
6. Discussion 62
6.1. An information provider database 62
6.2. Analysis of the clustering analysis 63
6.3. Limitations and further research needs 64
7. Conclusion and Recommendations 67
7.1. Conclusion 67
7.2. Recommendations 68
References 70
Annex A I
Annex B: Database II
Annex C: Survey for FIs Officials IX
Annex D: R Script XVI
iv

v
List of Figures
Figure 1: Sources of agriculture credit 5
Figure 2: Components of direct and indirect agriculture credit
6
Figure 3: Development Process through Micro-finance 7
Figure 4: Overview of value chain finance Triangle 10
Figure 5: Considered factors to reduce TC and risk management
in agricultural finance 15
Figure 6: Geographical location of the financial institutions
22
Figure 7: Geographical distribution of financial institutions
32
Figure 8: Listing criteria of financial institutions in the
final database 33
Figure 9: Financial institutions Questionnaire components
41
Figure 10: List of instruments enquired during the survey
43
Figure 11: Questionnaire Framework 44
Figure 12: Determination of the optimum number of clusters
52
Figure 13: Grouping Data scaled in different clusters 53
Figure 14: Clusters Visualization 53
Figure 15: Hierarchical Clustering 54
Figure 16: Validation of the number of clusters 57

vi
List of Tables
Table 1: Distribution of MFIs by institutional type 8
Table 2: The challenges of the agriculture finance according
to the literature review 12
Table 3: Determinants of credit Access 19
Table 4: Number of FIs mentioned in the literature review
21
Table 5: Composition of database 24
Table 6: Most popular Qualitative Analysis method 25
Table 7: Quantitative statistic types 26
Table 8: Data types 27
Table 9: Continent Attributes 27
Table 10: Institutional Type Attributes 27
Table 11: Agricultural loans Attributes 28
Table 12: Gender Attributes 28
Table 13: Digital Solutions Attributes 29
Table 14: financial Institutions General Information 34
Table 15: Specific loan features 35
Table 16: focal points of the World Bank Survey 36
Table 17: Lending decision variables 39
Table 18: Loan accreditation Characteristics 40
Table 19: AVCF Instrument 40
Table 20: Classification of financial institutions by
continent 45
Table 21: Classification of financial institutions by
institutional type 46
Table 22: classification of financial institutions by
foundation year 47
Table 23: Classification of financial institutions according
to the number of branches 47
Table 24: Percentage of credit offered by type of financial
institution 48
Table 25: Percentage of gender equality program offered by
type of financial institution 49
Table 26: Percentage of digital solutions offered by type of
financial institution 49
Table 27: Descriptive statistics of the dataset 50
Table 28: Cluster membership IDs using K means method 54
Table 29: Cluster membership IDs using Hierarchical method
55
Table 30: Nomination of the FIs groups 57
Table 31: Cluster's characteristics 60
List of Abbreviations

vii
ADB Asian Development Bank
AFD Agence française de développement
AfDB African development Bank
AFRACA African Rural and Agricultural Credit Association
AL1 Farmer credit
AL2 Agribusiness Credit
AOI Agriculture orientation index
AVCF Agricultural value chain finance
CB commercial banks
CGAP Consultative Group to Assist the Poor
DBs Development Banks
DS1 Online Banking
DS2 E-products Email and SMS Banking
DS3 Online loan application
FI Financial institution
G1 Credit facility for women
G2 Career development opportunities to female staff
G3 Gender Programmes
GS Global South
IDFC International Development Finance Club
IFAD International Fund for Agricultural Development
IFC International Finance Corporation
IFI International financial institutions
IIRR International Institute of Rural Reconstruction
INSE Institute of New Structural Economics at Peking
University
ISF advisory group
KIT Royal Tropical Institute
MBFI membership-based financial institutions
MFCs microfinance Companies
TC transaction costs
VC value chain
VCF value chain finance
WFDFI World Federation of Development Financing
Institutions
WBG World Bank Group
WOCCU World council of credit unions
Acknowledgment

VIII
The first thing I want to say is how grateful I am to God and
my father for the opportunity to study at the Technical University of Munich. I
would also like to extend my deepest gratitude to my sister Emna for her
support throughout the entire thesis process. Also, I want to thank my
girlfriend Myriam, as well as my friends Anas, Christian, Cyrine, Dali, Mourad,
Rached, Ramzi, Sabrina, Safa, Youssef, Wajih, Werner, and Zeineb for their
continued encouragement.
My thanks go out to Villalba Camacho Roberto and Venus Terese
for their helpful guidance and valuable comments and corrections during this
work. This work cannot be done without them. My strong gratitude to Susanne
Minges and Papaja-Hülsbergen Susanne for their continuous support during
my studies at TUM.
The internship opportunity with Agribusiness Facility for
Africa (ABF) and Green Innovation Centres for the Agriculture and Food Sector
(GIC) at the GIZ was a big milestone in my career development. It was a great
chance for learning and applying my knowledge and fresh skills in a real
working setting. I will strive to use everything I learned in the best possible
way. Through this internship, I met wonderful people and professionals who
helped me develop my experience. I would like to express my deepest thanks to
Dr. Annemarie Mathess, Carsten Schüttel, Wahid Marouani, and Melanie
Hinderer for their guidance and allowing me to participate in their projects
which helped me expand our knowledge on various topics.

ix
Abstract
Small-scale farmers and agribusinesses in the Global South
still face many barriers to access credit, despite the efforts of development
agencies, facilitator, and even financial institutions. An agricultural gap
persists that limits sector potential. The current master's thesis examines the
ways in which financial institutions make credit easier to obtain for
smallholder farmers and value chain players. The study uses a unique database
of 347 financial institutions in 106 countries from Africa, Asia, South
America, and Oceania as well as international institutions. It primarily
contains cooperatives, commercial banks, NGOs, microfinance institutions, and
agricultural banks. The database is constructed through a snowball effect
process using literature sources, online search, and open-source bank
platforms. This database contains several details about these institutions
including their institutional type, agricultural loans, gender equality, and
digital solutions.
The number of financial institutions was reduced to 144 for
the statistical analysis due to the lack of available data for several
financial institutions. Then an analysis of clusters was conducted to answer
the research question and determine patterns, similarities, and differences
among the selected financial institutions. Five clusters were identified. It
emerges from the study that financial institutions deliver customized and
enhanced rural financial services in high demand and in line with gender
issues, as proved in clusters 1, 2, and 3. Moreover, the youngest group of
institutions is cluster 4, which has the most digital solutions to offer.
Cluster five, which contains individuals primarily using traditional banking
methods, has the lowest level of financial services. This study has addressed
the research question in terms of credit provision, gender promotion, and
digital solutions, as well as identified the kind of similarities and
differences between financial institutions. Several recommendations are made in
this study, including the need to encourage women and provide digital solutions
to ease the lending process for small-scale farmers and value chain actors.
Keywords: Agricultural Value Chain Finance, financial
institutions, cluster analysis, financial products, Global South

x
Zusammenfassung
Kleinbauern und Agrarunternehmen im globalen Süden sehen
sich trotz der Bemühungen von Entwicklungsorganisationen, Vermittlern,
Maklern und sogar Finanzinstituten immer noch vielen Hindernissen beim Zugang
zu Krediten gegenüber. Es besteht weiterhin eine Lücke in der
Landwirtschaft, die das Potenzial des Sektors einschränkt. In der
vorliegenden Masterarbeit wird untersucht, wie Finanzinstitute Kleinbauern und
Akteuren der Wertschöpfungskette den Zugang zu Krediten erleichtern
können. Die Studie stützt sich auf eine einzigartige Datenbank von
Finanzinstituten in 106 Ländern Afrikas, Asiens, Südamerikas und
Ozeaniens sowie von internationalen Institutionen. Sie enthält vor allem
Verbände, Geschäftsbanken, NGOs, Mikrofinanzinstitute und
Landwirtschaftsbanken. Die Datenbank wurde in einem Schneeballeffekt-Verfahren
unter Verwendung von Literaturquellen, Internetrecherchen und
Open-Source-Bankenplattformen erstellt. Die Datenbank enthält verschiedene
Details über diese Institutionen, darunter ihre institutionelle
Einrichtung, Agrarkreditangebote, Frauenförderung und digitale
Lösungen.
Die Zahl der Finanzinstitute wurde für die statistische
Analyse auf 144 reduziert, da für mehrere Finanzinstitute keine Daten
vorlagen. Anschließend wurde eine Clusteranalyse durchgeführt, um
die Forschungsfrage zu beantworten und Muster, Ähnlichkeiten und
Unterschiede zwischen den ausgewählten Finanzinstituten zu ermitteln. Es
wurden fünf Cluster identifiziert. Aus der Studie geht hervor, dass die
Finanzinstitute maßgeschneiderte und verbesserte Finanzdienstleistungen
für den ländlichen Raum anbieten, welche auf geschlechtsspezifische
Aspekte Wert legen, wie in den Clustern 1, 2 und 3 nachgewiesen wurde.
Darüber hinaus ist die jüngste Gruppe von Instituten in Cluster 4 zu
finden, die die meisten digitalen Lösungen zu bieten hat. In Cluster 5, in
dem sich Finanzinstitute befinden, die hauptsächlich traditionelle
Bankmethoden nutzen, ist das Angebot an Dienstleistungen am geringsten. In
dieser Studie wurde die Forschungsfrage in Bezug auf die Kreditvergabe, die
Förderung der Geschlechtergleichstellung und digitale Lösungen
beantwortet und die Gemeinsamkeiten und Unterschiede zwischen den
Finanzinstituten ermittelt. In dieser Masterarbeit werden mehrere Empfehlungen
ausgesprochen, darunter die Notwendigkeit, Frauen zu fördern und digitale
Lösungen anzubieten, um die Kreditvergabe für Kleinbauern und Akteure
der Wertschöpfungskette zu erleichtern.
Schlüsselwörter: Finanzierung der
landwirtschaftlichen Wertschöpfungskette, Finanzinstitute, Clusteranalyse,
Finanzprodukte, Globaler Süden.

xi
Résumé
Les petits exploitants agricoles et les SME des pays du Sud
sont toujours confrontés à de nombreux obstacles pour
accéder au crédit, malgré les efforts des agences de
développement, les facilitateurs, les gouvernements et même des
institutions financières. Un écart financière agricole
persiste qui limite le potentiel du secteur. La présente thèse de
mémoire examine les moyens par lesquels les institutions
financières facilitent l'obtention de crédits pour les petits
exploitants agricoles et les acteurs de la chaîne de valeur.
L'étude utilise une base de données unique de 347 institutions
financières dans 106 pays d'Afrique, d'Asie, d'Amérique du Sud et
d'Océanie ainsi que des institutions internationales. Elle contient
principalement des coopératives, des banques commerciales, des ONGs, des
institutions de microfinance et des banques agricoles. La base de
données est construite par un processus d'effet boule de neige en
utilisant des sources documentaires, des recherches en ligne et des plateformes
bancaires à code source ouvert. Cette base de données contient
plusieurs détails sur ces institutions, notamment leur type
d'institution, les prêts agricoles disponible, la promotion de
l'égalité des sexes et les solutions digitales offertes par
l'institut.
Le nombre d'institutions financières a
été réduit à 144 pour l'analyse statistique en
raison du manque de données disponibles pour plusieurs institutions
financières. Ensuite, une analyse typologique a été
menée pour répondre à la question de recherche et
déterminer les modèles, les similitudes et les différences
entre les institutions financières sélectionnées. Cinq
regroupements ont été identifiés. Il ressort de
l'étude que les institutions financières fournissent des services
financiers ruraux personnalisés et améliorés, très
demandés et conformes aux questions d'genre, comme le prouvent les
regroupement 1, 2 et 3. En outre, le groupe d'institutions le plus jeune est
l'amas 4, qui a le plus de solutions numériques à offrir. La
grappe 5, qui contient des individus utilisant principalement des
méthodes bancaires traditionnelles, a le niveau le plus bas de services
financiers. Cette étude a répondu à la question de
recherche en termes d'offre de crédit, de promotion du genre et d'offre
de solutions numériques, et a identifié les similitudes et les
différences entre les institutions financières. Plusieurs
recommandations sont formulées dans cette étude, notamment la
nécessité d'encourager les femmes et de fournir des solutions
numériques pour faciliter le processus de prêt pour les petits
agriculteurs et les acteurs de la chaîne de valeur.
Mots-clés : Financement de la chaîne de valeur
agricole, institutions financières, analyse typologique, produits et
service financiers, Sud global.
ÕÎáãáÇ
ÒÒÒÒØ
ÏÒÒÒÒíÏ áÛ
æÒÒÒÒåÌÛæí
æÒÒÒÒäÌáÛ
ÇæÏ ÒÒÒÒ
ÒÒÒÒ æÉØáÛ æ
Ç ÒÒÒÒ áÛ
ÒÒÒÒÔáÛæ
íÒÒÒÒÍáÇáÛ
ÒÒÒÒ
ÇÛÕÒÒÒÒí
Çá
ÏæÒÒÒÒåÌ
ÒÒÒÒØ
ÒÒÒÒáÛ
ÒÒÒÒ åÉ
ÒÒÒÒÔä
ÒÒÒÒÍáÇáÛ
ÇÒÒÒÒíæØÉáÛ
ÒÒÒÒ
ÇæÒÒÒÒ ÍáÛ
æÏ ÇæÒÒÒÒÍÉ
ÒÒÒÒÉáÛ
ÕÌÛæÒÒÒÒÍáÛ
æÒÒÒÒÌáÛ
ÒÒÒÒå
ÏæÒÒÒÒÌæ Û
ØÉÒÒÒÒ Û
ÒÒÒÒíá ØáÛ
ÒÒÒÒ áØáÛ
ÒÒÒÒÉÍæ ì
ÒÒÒÒ æáÛæ
ÒÒÒÒØæ ÍáÛæ
ÒÒÒÒíØäÉáÛ
Çá ÒÒÒÒ æ
í ÉÒÒÒ
Ì ØáÛ
ÒÒÒÍæ Í Ñ
ÏÒÒÒÉ
ÒÒÒÍáÇáÛ
ÒÒÒ áÛ
ÒÒÒä ØÇ
ÒÒÒØ
ÏÒÒÒÍáÛ
ÒÒÒ
ÒÒÒí Ç
ÒÒÒÔ å
ÒÒÒ É
ÒÒÒí Û Õ
áÛ
ÍÒÒÒ Í
æ í
ÛÕÒÒÒØ á
ÇÒÒÒíæØÉáÛ
ÒÒÒ
ÇæÒÒÒ
ÍáÛ
ÒÒÒíá
ØáÛ ÒÒÒ
áØáÛ
ÒÒÒå
ÇåÒÒÒÒ É
ÒÒÒÉáÛ Ó
ÒÒÒ áÛ
ÒÒÒíá
ÍáÛ
ÒÒÒä í
ÏÒÒÒ Ç ÒÒÒ Û
ÏáÛ ÏÇÉÒÒÒ É
ÒÒÒÉä Û ÒÒÒ
ÒÒÒ í ÒÒÒáÛ
ÇÒÒÒ æ ÒÒÒ
æÉØáÛ æ Ç
ÒÒÒ áÛ ÒÒÒ
áØáÛ
ÒÒÒÖ
íÒÒÒ æä
íÇæÍæ ÒÒÒí
æäÌáÛ ÒÒÒ
í ØÍæ
íÒÒÒ æ
ÒÒÒí í Ç
ÒÒÒØ
ÒÒÒáæÏ 106
ÒÒÒØ
ÒÒÒíá
ØáÛ ÒÒÒ
áØ á ÏÒÒÒí
ÒÒÒí ÌÉáÛ
æÒÒÒä áÛæ
ÒÒÒíäæ ÉáÛ
ÒÒÒ ÒÒÒ Í Ç
ÒÒÒÔ ÏÒÒÒ áÛ
ÒÒÒå
æÒÒÒÉÍÉ
ÒÒÒíáæÏáÛ
ÇÒÒÒíæØÉáÛ
ÒÒÒ á Ø
ÒÒÒáÇ
ÏÒÒÒ áÛ
ÒÒÒå ì
ÒÒÒÔäÇ É
ÒÒÒíÍáÇáÛ
æÒÒÒä áÛæ
Ç ÒÒÒ áÛ
ÇÒÒÒíæØÉáÛ
ÒÒÒ áØæ
ÒÒÒíØæ
ÍáÛ ÒÒÒí
ÒÒÒØÙäØáÛæ
ÏÒÒÒÒ ØáÛ
ÒÒÒÒÍæÉØ
æÒÒÒÒä áÛ
ÒÒÒÒ äØæ
ÒÒÒÒä Éä Û
ÒÒÒÒ
æÒÒÒÒÍ áÛæ
ÒÒÒÒí Ï Û Ï
ÒÒÒÒ Ø
ÛÏÇÉÒÒÒÒ
ÒÒÒÒä í á
ÒÒÒå æä
ÒÒÒá ÒÒÒ
ÒÒÒØ ÒÒÒ
áØáÛ ÒÒÒå
ÇæÒÒÒÍ
ÇíÒÒÒ ÉáÛ
ÒÒÒØ ÏÒÒÒíÏ
áÛ ÒÒÒ ÒÒÒå
ÒÒÒä í áÛ
ÏÒÒÒ Ç
æÒÒÒÉÍÉ
ì Í á
æÉØáÛ íØÇ áÛ
Çæ ÍáÛæ í
äÌáÛ í Ûæ ØáÛ
ÌØÛ æ í Û ÕáÛ
Öæ áÛæ áØáÛ
ÏÒÒÒ ÒÒÒ
ÒÒÒ Í Û
ÇÒÒÒí ÍÉ á
ÒÒÒ áØ 144
ÒÒÒáÇ 347
ÒÒÒØ
ÒÒÒíá ØáÛ
ÒÒÒ áØáÛ
ÏÏÒÒÒ ÇíÒÒÒ
É ÒÒÒÉ ÒÒÒØ
ÒÒÒ ÒÒÒ
ÌáÅá ÏæÒÒÒ ä
áÛ ÇÒÒÒí
ÍÉáÛ ìÛ
ÒÒÒÌÇ ÒÒÒÉ
ÒÒÒ ÒÒÒíá
ØáÛ ÒÒÒ
áØáÛ ÒÒÒØ
ÏÒÒÒíÏ á ÒÒÒ
ÇáÛ ÒÒÒä í áÛ
æÒÒÒÉ
ÏÒÒÒíÏÍÉ
ÒÒÒÉ
ÒÒÒÉÇØáÛ
ÒÒÒíá
ØáÛ ÒÒÒ
áØáÛ
íÒÒÒ
ÒÒÒáÇÉÇáÇÛæ
å
ÒÒÒÔÉáÛæ
ÒÒÒØä Û
ÏÒÒÒíÏÍÉæ
æÒÒÒÍ áÛ
ÒÒÒ Í
ÒÒÒÒíí
ÒÒÒÒíá Ø
ØÏÒÒÒÒÇ
ÏÒÒÒÒ É
ÒÒÒÒÉáÛ
ÒÒÒÒíá ØáÛ
ÒÒÒÒ áØáÛ Í
ÒÒÒÒ Û ÏáÛ
ÒÒÒÒØ
ÒÒÒÒÖÉí
ÒÒÒÒ æØÌØ
ÑÒÒÒÒØÇ
2 æ 1
ÒÒÒ
æØÌØáÛ ÒÒÒ
ÒÒÒ ÒÒÒØ
íÒÒÒ äÌáÛ
íÒÒÒ Ûæ ÒÒÒ
ØáÛ ÍÏÒÒÒ Øá
ÒÒÒØ Ï ÒÒÒ Û
ÒÒÒå ÕÕÒÒÒ
Øæ ÒÒÒ ÇØ
ÒÒÒÉáÛ 4
ÒÒÒ
æØÌØáÛ ÒÒÒå
ÒÒÒ áÛ ÒÒÒ
æÉØ ÒÒÒíÍ ä
ÒÒÒØ ÒÒÒ
æØÌØ ÒÒÒ Í
äÒÒÒ ÒÒÒá
ÒÒÒ æáÇ ÒÒÒ
æ 3æ
í ÒÒÒáÛ
ÏÛ ÒÒÒ Û
ÒÒÒ
æÒÒÒÉÍÉ
ÒÒÒÉáÛ
ÒÒÒ Ø ÇáÛ
ÒÒÒ
æØÌØáÛ
åØÏÒÒÒ É
ÒÒÒÉáÛ
ÒÒÒíØÇ
áÛ ÇæÒÒÒ
ÍáÛ ÒÒÒÙ
Ø ÒÒÒÖÉ
ÒÒÒíá ØáÛ
ØÏÒÒÒÇáÛ
ÒÒÒØ
ÇæÉÒÒÒ Ø
ÒÒÒäÏÍ
åíÏÒÒÒá
ÒÒÒíÏí ÉáÛ í
ÒÒÒ ØáÛ íá
ÒÒÒ Û Çæ Û
ÒÒÒ ØáÛ ÒÒÒ
æØÏÇÉÒÒÒ í
ÕÒÒÒíÕ
Éæ
ÒÒÒÍáÇáÛ
ÇÒÒÒíæØÉáÛ
íæÒÒÒÉ
æÒÒÒíÍ
ÒÒÒØ
æÒÒÒÍ áÛ
á ÒÒÒ Ø
ÒÒÒ Û ÏáÛ
ÒÒÒå
ÒÒÒáæ äÉ
ÒÒÒíÍáÇáÛ
ÒÒÒíá
ØáÛ
íÒÒÒÒ
áÇÉÒÒÒÒÇáÇ
Ûæ ÒÒÒÒÔÉáÛ
ÒÒÒÒÌæÍ
æÒÒÒÒä
ÏÏÒÒÒÒÍ á
ÒÒÒÒ æ
ÒÒÒÒíØÇ áÛ
ÇæÒÒÒÒ ÍáÛæ
íÒÒÒÒ äÌáÛ
íÒÒÒÒ Ûæ
ÒÒÒÒ ØáÛ
ÒÒÒ Û ÏáÛ
ÒÒÒå ÒÒÒ
íÒÒÒ æÉáÛ
ÒÒÒØ ÏÒÒÒíÏ
áÛ íÏÒÒÒ É
ÒÒÒÉ
ÒÒÒÍáÇáÛ
ÇÒÒÒíæØÉáÛ
ÒÒÒ ÒÒÒ áÛ
ÒÒÒíá ØáÛ
ÒÒÒ áØáÛ
ÒÒÒ á ÖÛ
ÒÒÒÇ Û
ÒÒÒí Ø
ÇíåÒÒÒ
Éá
ÒÒÒíØÇ
ÇæÒÒÒ Í
íÏÒÒÒ Éæ
ì ÒÒÒ äáÛ
ííÌÒÒÒÔÉ
ÒÒÒáÇ
ÒÒÒÌ ÍáÛ
ÒÒÒá
ÒÒÒ
ÒÒÒØ
æäÌáÛ ÇæÏ
Éä Û íØå ØáÛ
Ç æ íÍáÇáÛ
ÇæÏ
ÒÒÒÒíá ØáÛ
ÇæÒÒÒÒ ÍáÛ
ÏæÒÒÒÒ ä áÛ
ÇÒÒÒÒí
ÍÉáÛ
ÒÒÒÒíá ØáÛ
ÒÒÒÒ áØáÛ
ÒÒÒÒÍáÇáÛ
ÇÒÒÒÒíæØÉáÛ
: Í ÒÒÒÒÉØáÛ
ÒÒÒÒØ áÛ

xii
æäÌáÛ

1
1. Introduction
1.1. Problem statement
Agriculture in developing countries is undergoing major
changes, including globalization and the transition from traditional low
production agriculture to modern high production agriculture. The result of
this process of profound changes has important consequences on poverty, risk
management and agricultural smallholders' income (Abid, Jie, Aslam, Batool,
& Lili, 2020). Smallholders face severe problems resulting from the
specificity of the production cycle. They have also to deal with climatic
factors such as extreme weather shocks and biological factors like insect
pests, crop, and livestock diseases (Fries & Akin, 2004). These production
risks are linked with price and market risks. Therefore, the variability of
production provokes high food price instability (Antonaci, Demeke, &
Vezzani, 2014). Due to this high risk, financial institutions are less
interested in financing the agricultural sector because of low profit and low
collateral (Herliana, Sutardi, Aina, Himmatul, & Lawiyah, 2018). Moreover,
Financial Institutions (FIs) consider micro-entrepreneurs as
"non-bankable», or not creditworthy because they have no previous credit
history or guarantee to offer (Yunus M. , 2007). On the other side, farmers
often face multiple challenges to access the finance they require, the outcome
is thus a financing gap that limits the potential of agriculture (UNCTAD,
2004). This financing gap which exists in the agricultural sector is estimated
at about $170 billion per year (ISF Advisors and Mastercard Foundation, 2019).
Development agencies, research institutes and donors have centered their
efforts on developing new approaches that allow different stakeholders, such as
agribusiness, and financial institutions to address this gap. The aim is to
provide innovative financial services to producers, processors and traders as
well as develop an economic and financial environment (IFAD and CPI, 2020).
Among these approaches, we can find Agricultural Value Chain
Finance (AVCF) which refers to leveraging the a value chain's connections and
social capital to improve financial flows and reduce the risks in the chain
(Miller & Jones, 2010). Whereas many of the value chain finance
transactions, instruments, and processes are not new (Robert, Chalmers, &
Grover, 2012),what is new is how AVCF is used by FIs and rural producers. What
is also innovative is the variation of the application and the different
organizations that offer finance in different innovative ways, as well as the
diversification, the intensification, and combination of mechanisms (Miller
& Jones, 2010). It also means linking financial institutions to the value
chain, providing financial services to support the flow of products, and
building on the relationships established at the chain level. This type of
financing offers alternatives to traditional requirements (KIT and IIRR, 2010).
This allows all value chain participants to benefit from it without collateral
requirements (Cuevas & Pagura, 2016). AVCF differs from other types

2
of financing in that it expands financing opportunities for
agriculture and improves repayment efficiency among chain participants. It is
not only that the nature of the funding is different, but also the motivations.
Nyoro (2007) mentions that `value chain actors are driven more by the desire to
expand markets than by the profitability of the finance' (Nyoro, 2007). The
solutions offered by AVCF can help to build a value chain, mitigate barriers,
or improve value chain operations, thereby increasing the competitiveness of
the chain (KIT and IIRR, 2010). The challenges that AVCF can face are legal
systems that enforce contracts and provide some type of ownership, lack of bank
penetration and institutions offering loans for investment in rural areas, high
transactions cost, lack of knowledge and developed infrastructure (Zander R. ,
2016).
1.2. Objectives
Recent work has focused on evaluating the access to finance at
the farmers' level (Gamage, 2013), however, there is limited evidence on the
role of Financing Institutions (Meyer R. L., 2002), in particular, in new
approaches such as Agricultural Value Chain Finance. This master's thesis aims
to build a database of financial institutions that fund agriculture in the
Global South. A number of different financial institutions are included in the
database, including a range of institutional types and banking experience, as
well as the services offered by each institution. Following that, a descriptive
analysis of the data from these institutions will describe the basic features
of the data. This analysis will provide simple summaries of data and draw
conclusions from it. A later study can use this database to conduct the online
questionnaire with these FIs. This will enable research staff to determine the
methods that financial institutions use about credit screening, scoring, and
agricultural value chain financing. A part of this thesis involves analyzing
the existing questionnaire and preparing the basis for the design of the
questionnaires [Annex C]. From this database, a cluster analysis using R
will be able to draw conclusions about lending to farmers and credit for value
chain participants. The aim of this study is to provide robust evidence
regarding the similarities and differences between financial institutions when
it comes to offering rural services to their clients.
1.3. Research questions and hypothesis
The present study will focus on the role of financial
institutions in implementing value chain finance in the Global South. This
study will address the following questions:
1) What are the key underlying characteristics of credit
provision of different types of financial institutions in the Global South?
2) What is the extent to which financial institutions promote
gender issues and offer digital solutions?

3
3) What kind similarities and differences can be observed
between financial institutions? As a result, the present work highlights the
subsequent main hypotheses H1, H2 and H3:
H1: Various trends can be seen on the basis of the variables
concerning the provision of credit by different types of financial institutions
in the Global South.
H2: Only a few financial institutions deal with gender issues
and offer digital solutions
H3: Financial institutions show several similarities and
differences in credit provisions, gender programs, and digital solutions.
1.4. Expected results from the research
The expected outcomes of this study are:
i. A database of financial institutions which fund agriculture
in the Global South.
ii. Data-driven evaluation of financial institutions' services
in the Global South
iii. An agenda for agricultural finance policy
recommendations
1.5. Organization of the thesis
Throughout this study, six sections are discussed. The
following part is a literature review which covers theoretical perspectives
about agriculture finance, AVCF definitions and challenges, and a review of
available papers, as well as agricultural credit determinants. In the third
section, we describe the study, the way the database was built, and the
statistical methods used. The fourth section focuses on the design of the
survey. In part five, we examine the results of our descriptive and cluster
analyses. Lastly, the fifth part summarizes the findings, discusses them, and
makes policy recommendations. The Annex «C» contains the
survey.

4
2. Literature Review and Theoretical Background
2.1. Agriculture finance
2.1.1. Agricultural credit
Several interventions are needed in the form of financial
institutions and instruments at the value chains (VCs) level to improve
financing in the agricultural sector. This interest has been renewed following
the economic crisis and the increase in food prices in 2008 and 2011 (Arias,
2019). In most developing countries, the level of financing and public
expenditure on agriculture remains very low. This reflects the low percentage
of the share of agricultural credit of total credit (Piñeiro, 2019).
Moreover, according to the agriculture orientation index (AOI) for credit,
agriculture financing is still also low and is just 0.4 in developing
countries, while in developed countries it is 1.95 (Arias, 2019).
According to Adams (1994), rural producers need access to
financing at the right time and this needs to be stable and reliable for more
than a few cropping seasons. This is designed to improve the production and
marketing process as well as to have access to input, new technologies and
limited resources (Zander R. , 1994). While rural credit is a powerful
instrument for poverty alleviation (Ololade & Olagunju, 2013), supporting
the agricultural sector is always complicated for FIs because access to
information is very expensive and difficult. Moreover, the soft skills in
lending to small-scale farmers are not well developed (Zander R. , 2016).
Many studies on the agricultural credit in developing
economies have shown that agricultural lending is necessary to improve
productivity in the agricultural sector (Sriram, 2007; Das, Senapati, &
John, 2009). Other studies have found that without external financing,
small-scale farmers cannot even continue their business, and this is proved in
the history and debts of people working in agriculture (Gowhar, Ganie, &
Padder, 2013). Agricultural credit is therefore a necessary element in meeting
the need for investment and bridging thegap between the farmer's income and the
expenditure in the field (Khan, Shafi, & Shah, 2011). Additionally,
Agriculture credit plays a key role in the modernization of agriculture by
removing financial constraints and accelerating the adoption of new
technologies (World Bank, 1975).
In this context, Singh et al. (2001) announced that most farm
households face a lack of funds on their side. To meet their credit
requirements, both formal and non-formal financing is available in a developing
economy. Pradhan (2013) suggests that farmers' need for credit increased
spontaneously after the Green Revolution. This was the period when
institutional sources of credit were considered major players. This was the
time when subsistence crops were replaced by cash crops. These credit sources
were classified in the following figure into
three groups by Yadav and Sharma (2015) following their
intensive literature review on agricultural credit.
Agriculture credit
Non- institutional sources
Semi- institutional Sources
Institutional Sources
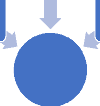

5
Figure 1: Sources of agriculture credit
Source: Yadav & Sharma (2015)
Figure 1 shows the main sources of credit that are available
to rural producers. Credit from institutional sources includes credit from the
creation of institutional framework with banks and institutions including
specific organizations established for agricultural development, commercial
banks, cooperative banks, and regional rural institutions. Non-institutional
sources cover credit from the unorganized sector such as friends, relatives,
landlords, entrepreneurs who are not part of the institutional set-up (Ijioma
& Osondu, 2015). Halfway between the institutional and non-institutional
agencies is the semi-formal configuration of microfinance and the provision of
a range of financial and non-financial services to members based on joint
responsibility (Yadav & Sharma, 2015).
Regarding the components of agricultural credits, Yadav &
Sharma (2015) highlight direct credit, which includes short-term loans,
medium-term loans, and long-term loans for agriculture and connected activities
with direct responsibility for repayment. According to Gowhar et al. (2013),
short and medium-term loans are provided by cooperatives, commercial banks, and
regional rural banks for agriculture and allied activities. Whereas, long-term
loans for agriculture are provided by rural development banks and primary
cooperatives. Short-term agriculture credit enables farmers to buy inputs such
as fertilizers, seeds, power, irrigation and the cost of the hired labor
(Osuntogun, 1980; Adebayo & G, 2008). Short-term credit is practically for
6 months. However, long-term credit is oriented toward large investments such
as irrigation pumps, tractors, and agricultural machinery (Anwarul &
Prerna, 2015). While indirect credit allows the farmer to benefit from
subsidized inputs and warehouse facilities. In this case, farmers are under
indirect repayment responsibility through fertilizers dealers and

6
input suppliers. Figure 2 summarizes the components covered
under the scope of institutional credit.
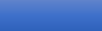
Direct Credit
Indirect Credit
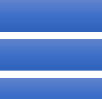
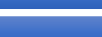
Subsidized Inputs
Warehouse facility
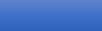
Setting up
agribusiness centres
t
Credi

Agricul
ture
Short term loans
Medium term loans
long term loans
Figure 2: Components of direct and indirect agriculture
credit Source: Yadav & Sharma (2015)
2.1.2. Financial institutions (FIs) in the Agriculture
Sector
Financial institutions (FIs) are organizations that engage in
the business of facilitating financial and monetary transactions. There are
different types of financial institutions in a developed economy. Financial
institutions cover also commercial banks, insurance companies, and brokerages
firms. Furthermore, financial institutions can differ by size, scope, and
geography. They offer a wide range of products and services such as
transactions, deposits, loans, investment, and currency exchange.
In the agricultural sector, the main source of loans for
smallholders' farmers and agribusinesses are other
agribusinesses in the VC. However, farmers and agribusinesses can benefit from
credit offered by FI. These providers of rural and agricultural finance can be
broadly Banks (commercial, agricultural banks, state development banks),
non-bank financial institutions (NBFIs, commercial MFIs, other non-bank
lenders, and leasing companies), Not-for-profit MFIs, which tend to work with
poorer clients, and Credit unions and agricultural cooperatives.
KIT and IIRR (2010) have shown that IFs have the capacity to
develop new markets for all actors in the chain and make them bank customers.
In the Global South, agriculture is the backbone of the economy, then the
capacity to benefit the sector can significantly increase the actions of
FIs.
2.1.3. Microfinance
Generally, the term MFI is given to non-profit organizations
that depend on donations and grants to enable them to fulfill their primary
social role of poverty alleviation (D'Espallier, 2012). Thus, in its limited
concept, microfinance is the provision of micro-credit for small entrepreneurs
who lack access to the formal financial system. Over time, Microfinance has
developed from providing micro-loans for low-income people to collecting
savings, micro-insurance, micro leasing, assisting with money transfer in
relatively small transactions for disadvantaged people, and finally marketing
and distributing client's products (Thai-Ha, 2020; Ferdinand & Asmah,
2012). MFIs play a key role to boost the social capital and the inclusion of
disadvantaged populations and serve hundreds of millions of low-income
borrowers (Morduch, 1999) because it allows access to credit to non-bankable
people with all the advantages from banking services (Yunus & Jolis, 1999).
The aim is to reduce poverty and develop economies, especially in Asia and
Africa. Moreover, microfinance has enabled smallholders to improve the living
standards of people, increase income and generate employment (Thai-Ha, 2020). A
web-based report on microfinance shows a practical framework for understanding
how MFIs function after a typical microfinance intervention using the
illustration in Figure1 3. The main objective of microfinance is
economic empowerment through the use of micro-credit as an entry point for
overall empowerment.

7
Donors and Banks
Micro Enterprise
Production Needs
Farm related
Micro Enterprise
Production Needs
Non-Farm related
Individual
Promotional work, Formation Implementing Org.
Credit Delivery, Recovery, Monitoring
Income generation
Economic Empowerment
Individual
Figure 3: Development Process through Micro-finance
1 Source: (Ferdinand & Asmah, 2012, p. 76), Accessed: 18 July
2021
See
https://journals.ug.edu.gh/index.php/gssj/article/download/1174/774#page=78

8
Source: Ferdinand & Asmah (2012)
Concerning the differences between these stakeholders,
microfinance is a financial service that caters to the needs of the poor and
micro enterprises, and it is typically a collateral-free short-term loan. In
contrast, commercial banks generally do business with corporate clients, SMEs
and individuals with higher incomes, and offer financing mainly based on
collateral and repayment capacity. Alternatives include central banks serving
the banking system. They facilitate cross-border transfers of money between
banks and government institutions, both domestically and abroad. This flow is
required while MFIs are complementary, not substitutes for, banks, donors, and
state banks (Miguel & Silvana, 2007). In the figure above, we can see how
effective collaboration between social welfare programs, MFIs, state Banks, and
commercial banks may lead to greater poverty alleviation.
According to Ferdinand & Asmah (2012), this reduced
process depends on microfinance's use to create a sustainable environment and
more opportunities. Success in implementing microfinance is mainly linked to
the ability of MFIs to meet the objectives of Donors and Banks in facilitating
credit approval and the increase in the percentage of borrowers' repayments.
MFIs are always adopting different innovations to expand the
delivery of microfinance in rural areas, Meyer (2007) announced several new
products, technologies, and institutional connections intending to target rural
areas and make financial services available for rural households. Some
moneylenders use standing crops as collateral for loans. Others take assets as
collateral for short-term farm and non-farm loans (World Bank, 2007).
Gonzalez & Rosenberg (2006) compiled a database that
includes 2600 MFIs with 94 million borrowers. This database encompasses several
micro-credit providers that are granted by a variety of FIs. The following
table2 1 shows the approximate share of each type of FIs in the
approval of micro-loans.
Table 1: Distribution of MFIs by institutional type
Institutional type Percentage %
State owned banks
|
30%
|
State owned Institutions
|
30%
|
NGOs
|
25%
|
Private banks and finance Companies
|
15%
|
2 Source: Gonzalez & Rosenberg (2006, p.2) Accessed 17 July
2021
See
https://www.researchgate.net/publication/228276752
The State of Microfinance -
Outreach Profitability and Poverty Findings from a Database of
2300 Microfinance Institutions

9
Source: Gonzalez & Rosenberg (2006)
In the agricultural sector, various authors have concluded
that microfinance boosts short-term agriculture investment, earnings, and
consumption (Kaboski & Townsend, 2012; Mosley & Hulme, 1998).
Unfortunately, while microfinance shows positive impact on Agriculture and
Transformation, it has not solved the challenges r thatsmall-scale farmers
face. Moreover, it has not shown a significant impact in terms of employment,
increased income flows, and physical asset accumulation (Ferdinand & Asmah,
2012; Buckley, 1997; Coleman, 1999). In this context, some Scholars have
enumerated several faults such as the high-interest rate, aggressive collection
method, and driving people into debt (Thai-Ha, 2020). Besides, Diagne und
Zeller (2001) have found following their study in Malawi that microfinance does
not show any significant effect on household's revenue (Diagne & Zeller,
2001).
Hence, microfinance has its limitations and faces several
challenges in lending to small-scale farmers. For this reason, continuous
innovations are needed to enable MFIs to be more efficient in improving
farmers' income, poverty alleviation, and economic empowerment while making
service providers more sustainable over time.
The following section focuses on the Agriculture Value Chain
Finance (AVCF) approach, which can complement and go beyond microfinance. The
important difference here is that AVCF is linked to all actors and
relationships in the chain in addition to transactions. Therefore, this concept
can join several actors in microfinance. In addition, microfinance can be part
of AVCF but must be with other financial services to address the different
needs of the chain (KIT and IIRR, 2010).
2.2. AVCF definition
There is still no unified definition of AVCF. Different
authors show an understanding with various characteristics on this subject.
However, one of the most widely accepted definitions is the one formulated by
Miller and Jones (2010), who define value chain finance as:» the flows of
funds to and among the various links within a value chain" and distinguish
between internal and external value chain finance. Likewise, authors from FAO
and AFRACA (2020) defined AVCF as two internal flows of financing between chain
actors directly within the VC and for financial service providers who use AVCF
to lend money or to invest in one or more of the chain actors. However, the
authors of KIT and IIRR (2010) have defined the VCF triangle, in which FI
engages with the actors of the chain. This triangle is among FI, the seller and
the buyer. The figure below illustrates the VCF.
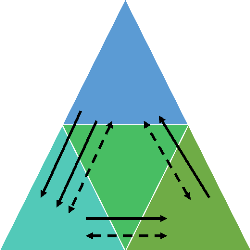
Seller
financial institution
VCF triangle
Buyer

10
Figure 4: Overview of value chain finance Triangle
Source: KIT and IIRR (2010)
This figure shows the payment, loan, and information and
services flows between the financial institutions and the seller. Additionally,
the payment and information flow between buyer and FI. Eventually, the flows of
information and services and product between the buyer and the seller.
Complementarily, a study by Carroll et al. (2012) provides a
pragmatic definition of AVCF:
...in the case of agriculture, the value chain may include
(but is not limited to) input provision, production, processing, transport,
storage marketing, and export.
Additionally, the Asian Development Bank (ADB) (2012) makes the
definition of AVCF simpler:
...organized linkages between groups of producers, traders,
processors, and service providers (including nongovernment organizations) that
join together to improve productivity and the value-added from their
activities.
Similarly, Zander (2016) presented the following
definition»:
Value chain finance (VCF) denotes all financing arrangements
within a specific value chain or from outside the chain. As the concept of
value chains and their financing is broad and multifaceted, the terms `value
chain' and `VCF' necessarily refer to a broad range of different instruments
and mechanisms.
Finally, a recent study by Villalba, Venus, & Sauer (2021)
explains Agricultural Value Chain Finance (AVCF) as:
A variety of products and approaches that allow stakeholders
from a value chain to leverage social capital and satisfy the funding needs of
the weakest actors. Cooperating within a value chain reduces risk, which can
facilitate the

11
acquisition of financing from financial institutions, and other
lenders at a lower
cost.
While no common definition has been proposed in the
literature, ADB (2012) and Carroll et al. (2012) have the constituting element
«provision», «processing», and «productivity» in
common. They show that the chain starts from the raw material stage to the
final consumer. However, other researchers explain this term as a variety of
different products, mechanisms, and instruments used by different actors in the
chain to initiate financing arrangements (Villalba, Venus, & Sauer, 2021;
Zander R. , 2016).
There is agreement between the literatures when it comes to
the flows of funds. (Miller & Jones, 2010; KIT and IIRR, 2010; AFRACA and
FAO, 2020). In addition, there are definitions of AVCF for which the authors
partially agree such as internal and external value chain (Miller & Jones,
2010; AFRACA and FAO, 2020).
The authors agreed that Internal Value chain finance takes
place within the value chain such as when an input supplier provides credit to
a farmer, or when a lead firm advances funds to a market intermediary. External
value chain finance is that which is made possible by value chain relationships
and mechanisms: for example, a bank issues a loan to farmers based on a
contract with a trusted buyer or a warehouse receipt from a recognized storage
facility.
Other authors defined AVCF as a triangle, in which, an
agreement between the actors (FI, seller and buyer) is made around the product,
the need for financing, the sharing of information, the method of
communication, and finally the way of risk management (AFRACA and FAO, 2020).
This agreement according to KIT and IIRR (2010) allows the development of the
value chain in three different ways:
a) Ensuring liquidity for the actors of the chain
b) Creation of new chains
c) Investments in existing chains
This highlights how general financing of agriculture works,
(new investments, reinvestments, and financing of current assets) and is a
useful typology for value chain development.
2.3. AVCF Challenges
About the challenges of AVCF, the authors have listed several
constraints that buyers and suppliers face in lending to farmers as shown in
the following Table 2.

12
Table 2: The challenges of the agriculture finance according
to the literature review
Challenges
|
Author(s)
|
Pearce
(2003)
|
Langen bucher
(2005)
|
Honohan and
Beck
(2007)
|
Meyer
(2011)
|
IFC
(2012)
|
AfDB
(2013)
|
Klonner and Rai
(2015)
|
Herliana
(2018)
|
ISF
(2019)
|
Financial
exclusion of farmers
|
|
X
|
X
|
X
|
X
|
X
|
X
|
X
|
X
|
information asymmetries
|
X
|
X
|
|
X
|
X
|
X
|
X
|
X
|
|
Transaction cost
|
X
|
X
|
|
|
X
|
X
|
X
|
X
|
|
High fees
|
|
|
|
|
X
|
X
|
X
|
X
|
|
insufficient amounts of credit
|
|
|
|
|
X
|
X
|
|
|
X
|
Low
infrastructure, distant location
|
|
X
|
|
|
X
|
|
|
|
|
Low Education of farmers
|
|
|
|
|
|
|
|
X
|
|
Fluctuating Production
|
|
|
|
|
|
|
|
X
|
|
Lack collateral
|
|
|
|
|
|
|
|
X
|
|
Inefficient Market
|
|
|
|
|
X
|
|
|
|
|
The challenges cited in most literature studies are the
following:
Financial exclusion of farmers: according to
Langenbucher (2005) the causes of financial exclusion for the small farmer from
the supply side are the lack of robust business models, and limited access to
equity capital. Likewise, the key features that influence value chain finance
(VCF) are the high incidence of informality (lack of documentation and
contract), the intermediation deficiency (high-interest rate and minimum
deposit), and the dominance of the banking sector (lack of information about
credit worthiness of potential clients, weaknesses of the legal system, and
high degree of corruption and inefficient bureaucracies. (Langenbucher, 2005;
Honohan & Beck, 2007; Meyer R. , 2011). Meanwhile, formal institutions are
less interested in financing the agricultural sector due to several constraints
and subsequently obtaining formal credit is a complex procedure (Herliana,
Acip, Qorri, Qonita, & Nur, 2018).
Information asymmetries: in accordance with
Pearce (2003) and Langenbucher (2005), which makes the agricultural environment
very complicated, are a lack of information about

13

14
smallholders' farmers and risk of agricultural activities. In
addition, this sector is complicated due to the unique problem of the
agricultural sector, the inadequate policy, regulatory environments, and
asymmetric information (IFC and World Bank, 2012; Herliana, Acip, Qorri,
Qonita, & Nur, 2018). In addition, several studies cited that FIs are not
aware about farmer's credit worthiness, and therefore, smallholders' farmers
are left when FIs try to mitigate risk (Klonner & Rai, 2005; IFC and World
Bank, 2012; Pearce, 2003). Thus, banks were not investing adequately to
understand the demands and nuances of value chains (VCs). This lack of
information leads to the design of financial products that are not suitable for
rural activities (AfDB, 2013).
Transaction cost: can be viewed from a
financial point of view as a difference between the price a broker pays for a
security and the price the buyer pays (Cheung, 1987). Most authors agree that
transaction costs are one of the most important constraints that FIs have to
deal with (Langenbucher, 2005; IFC and World Bank, 2012; AfDB, 2013). According
to Pearce (2003), buyer and suppliers face difficulties in lending to farmers.
Among these difficulties, he mentioned transactions cost as a major problem.
Following the bibliographical study concerning the
constraints, the authors show a partial compromise about the following
challenges:
High fees: as defined in the report of the
AfDB (2013), small farmers who already have access to loans find the terms very
rigid and the fees too high, which causes an increase in production costs. As a
result, they borrow money from family and friends, or money lenders who charge
high interest and limit their potential to expand (IFC and World Bank, 2012;
Herliana, Acip, Qorri, Qonita, & Nur, 2018).
Insufficient amounts: according to ISF
Advisors (2019), small farmers in South Asia, sub-Saharan Africa and Latin
America need about USD 240 billion for agricultural and nonagricultural
finance. Service providers are not able to meet this demand and the latest data
collection indicated that financial service providers provided only USD 70
billion distributed as follows: USD 30 billion by value chain actors, USD 21
billion by formal financial institutions, and USD 17 billion by informal and
community-based financial institutions. This shows that 70% of the global
demand of small farmers remains unsatisfied, the equivalent of USD 170 billion
and the contribution of FIs remains minimal (ISF Advisors and Mastercard
Foundation, 2019). Additionally, most loans are short-term which cannot improve
the output and income (AfDB, 2013). Moreover, lenders confront irregular
payments and slow rotation of capital (IFC and World Bank, 2012).
Low infrastructure and distant location:
While Langenbucher (2005) in this context has listed the lack of
appropriate risk mitigation and infrastructure, and no branches or limited
network in rural areas, the authors of IFC (2012) enumerated
the low population densities, low infrastructure, distant locations, and the
inefficient market, which can only worsen the situation and decrease
profitability.
Finally, other challenges were listed separately and show less
compromise between the others:
Low education of farmers one of the barriers
to agricultural finance is the low education level of small farmers (Herliana,
Acip, Qorri, Qonita, & Nur, 2018). This low literacy level of cultivators
is the main cause of the limited access to information which translates to low
efficiency in resource management and then low productivity crops. This low
productivity is due to the lack of knowledge about the right proportion of
Inputs (seeds, chemical fertilizers, and pesticides). Besides, in a present
changing environment, farmers are unable to compete which traps them in a
vicious circle of poverty.
Fluctuating Production Most banks avoid
financing agriculture on the ground of fluctuating production and uncontrolled
price risk (Herliana, Sutardi, Aina, Himmatul, & Lawiyah, 2018). The main
causes of the fluctuating production are climatic factors such as: water,
light, rainfall, and temperature which lead to unpredictable food production
(Gilbert, 2010). In addition, ecological land change and cultivated land
intensity will make the production more complicated (Xie, He, & Xie, 2017;
Xie, He, Zou, & Wu, 2016).
Lack of collateral Herliana (2018) showed
that FIs avoid financing small farmers following the low profits and lack of
collateral. Besides, rural producers do not generally have assets that IFs are
willing to accept as collateral. In addition, pledges of agricultural assets
have often been insufficient to ensure credit recovery, thus threatening the
sustainability of development Financial Institutions (FAO and ALIDE, 1996).
Inefficient Market According to IFC authors
(2012), unstable market prices aggravate the financial situation of small
farmers. Food demand is constantly rising due to growing population. however,
food supply is dropping due to increasing production cost. This imbalance in
food demand and supply is the primary cause of the price volatility (Lu, 1999).
Besides, continuous changes in input and output prices reduce the income of
small farmers (Ijioma & Osondu, 2015).
2.4. Competitiveness of agricultural finance
According to Abid et al. (2020), to achieve the objective of
reducing transaction costs (TC) and risk management, several factors should be
considered by organizing chain actors in VCF. Among these factors3,
we can mention; Agility, Innovativeness, Information sharing, Trust,
3 Source: Abid et al. (2020)

15
Information & Communication, and Contractual Governance
(Abid, Jie, Aslam, Batool, & Lili, 2020).
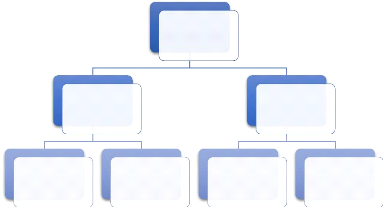
Information Sharing
Agility
Trust
Competitiveness
Information & Communication
Innovativeness
Contractual Governance
Figure 5: Considered factors to reduce TC and risk management
in agricultural finance
i. Agility: which is the ability to respond
to changing customer needs and satisfy the unique needs of each customer
with flexibility and adapt to unforeseen circumstances (Abid, Jie, Aslam,
Batool, & Lili, 2020). Then, agility in the value chain allows the FIs to
respond quickly to the needs of customers and then ensure competitiveness in
the chain. This accelerates the financial flow in the VC and reduces the
intervention of informal money lenders (Ellis, 1992). In the same scope of
work, Meyer (2007) emphasized the important role of the intervention of FIs in
identifying the unmet demand and to find where the lending cost and risks are
lower. He mentioned that this follows up requires more analysis to design
appropriate product, improve lending capacity, and make diversified loans
portfolio. Therefore, Agility in the VCF improve competitiveness with respect
to financial product and innovation (Swafford, Ghosh, & Murthy, 2006).
ii. Innovativeness: is a system in which VC
actors provide new products and services to increase customer satisfaction
(Simon & Yaya, 2012). The authors of IFC (2011) and Hult (2004) found that
FIs need to develop new credit skills and policies, credit scoring and rating
tools, as well as portfolio monitoring practices to provide high value
customers. The way of lending credit to farmers is parametric, while it is
based only on a few parameters due to the lack of collateral. This should be
improved, developed, and replicated. Generally, FIs

16
with a high degree of innovation can adapt to every change in
the environment and ensure more competitiveness within the VC. Moreover,
according to Kaufmann (2000), FIs can improve their ability by strengthening
the process of product innovation by organizing producers and buyer's
relationship. Equally, According to Röttger (2015), FIs should understand
the risks and opportunities of the sector and link loan with insurance
products.
iii. Information sharing: according to Brown
(2010) and Pagano (1993), Information sharing describes how the actors in
the chain react to each other over time. Information sharing is very important
as it improves market competitiveness, credit allocation efficiency, reduces
asymmetric information, and increases lending volume. Furthermore, the sharing
of information helps FIs to address asymmetric information using the network,
incorporate the collaboration with VC actors to design innovative product
(Miller C. , 2012; Röttger, 2015), in this regard, the authors of KIT and
IIRR (2010) and Kim (2017) highlighted that information sharing return the
relationships in the chain smooth and simplified and build better partnership.
Moreover, the cost of credit screening, monitoring and enforcement are reduced
due to chain actors, which take part in this procedure and do this work
themselves and make agile the VCF (KIT and IIRR, 2010; Kim & Chai,
2017).
iv. Trust: Trust is about how reliable and
credible the partners in the value chain consider themselves to be (Abid,
Jie, Aslam, Batool, & Lili, 2020). Trust is therefore considered as an
important element to overcome unexpected situations and to act in situations
perceived as risky (Song, 2018). According to Jones et al. (2015) trust between
stakeholders is an essential requirement for successful management of financial
flows in the value chain. In this context, Miller, and Jones (2010) mention
that trust between producers and buyers is one of the key factors to mitigate
risks. Thus, the adoption of an agile approach VCF is based on a better level
of trust (Svensson, 2001).
v. Information & Communication:
according to Ali et al. (2011) and Imtiaz et al. (2015), Information and
communication enhance the creditworthiness of smallholder's farmers, reinforce
partnerships between value chain actors, minimize the cost of stakeholder
interaction and the risk related to the VCF. For more effectiveness and risk
reduction through information and communication, the authors of IFC (2012) have
mentioned the development of insurance products, such as crop and weather
insurance products, credit life product, and emerging health and FIs should
rely on cash flow, saving or group guarantee, then within their value chain and
take advantage of all information to develop

17
adequate product that combine financial and non-financial
services. Besides, FIs should improve their capacities to assess farmer credit,
develop insurance and risk-sharing, and identify opportunities to increase
their level of comfort and reach more farmers (IFC and World Bank, 2012).
Likewise, Röttger (2015) highlighted the importance of relying on the
recommendations of group leaders and extension agents in the aim of linking
loan with insurance products, increasing loan amounts, and declining interest
rate. Zhao et al. (2019) have found that the use of information facilitates
innovation in financial services, lower costs, monitor risks, and allow for
agile financial flows.
vi. Contractual Governance: is the degree to
which a contractual partnership is legislated by a formal contract
specifying formal rules, responsibilities, and duties (Zhou & Poppo, 2010;
Cao & Lumineau, 2015). Moreover, it supports innovation-based coordination
and strict collaboration between actors. This cooperation between value chain
partners, according to Anna Grandori (2019), regulates transactions and the
pooling of resources, and thus, procedures for innovation. According to the
authors of the AFRACA (2020), AVCF offers several advantages for chain actors.
Starting with producers, AVCF makes it easier for them to get credit because of
the lack of collateral. Then, for agribusinesses, AVCF strengths the
buying-selling relationship and allows market expansion. Finally, for FIs and
investors, AVCF reduces transaction costs, improve repayments, and mitigates
risk due to more suitable financial products. Due to the absence of FIs in most
rural areas because of cost and risk of agriculture lending are too high, the
authors found that FIs can take advantage of the collaboration in the VC and
work with companies rather than directly with farmers to deal with these
risks... Eventually, the public sector has a crucial role for a suitable policy
and banking regulations that allow the application of new approaches, and
infrastructure.
2.5. Determinants of agricultural credit
Several studies have been done in the past on the
identification of the determinants of agricultural credit and the factors that
significantly influence the decision (Akpan, et al., 2013; Salami &
Arawomo, 2013; Yuan, Hu, & Gao, 2011), many variables (factors) have been
identified in the literature leading researchers to analyze their impact on the
decision of credit accreditation (Abid, Jie, Aslam, Batool, & Lili,
2020).
To start with Meyer (2007), FIs need to ask farmers before
lending, such as others engaged in economic activities for the households, cash
inflows and outflows, source of income for
repayment, and structure of the given loan. This can help FIs
to determine the amount to be lent to the specific VC, and the method to
mitigate risks. Thus, this evaluation allows determining the creditworthiness
of farmers, the types, terms, and conditions of financial products needed to
meet these demands. Other studies have also identified specific factors for the
allocation of credit such as education, marital status of the household,
contact with extension agents, years of experience in farming, land size,
gender, etc. (Aliero & Ibrahim, 2011; Dzadze, Osei, Aidoo, & Nurah,
2012; Akpan, et al., 2013). Among other socio-economic factors, being a member
of a cooperative plays a key role in access to credit (Ijioma & Osondu,
2015). A recent paper in Pakistan has shown also that health status remains one
of the determinants for credit accreditation (Saqib, Kuwornu, K.M., Panezia,
& Ubaid, 2018).
According to Gammage (2013), access to bank finance is
determined by a number of factors such as ownership type, age of the firm,
sector, and location of the business, assess tangibility, firm performance,
availability of audited financial statements, gender of the owner-manager and
perception of the owner-manager of access to bank finance, and characteristics
of the borrower at the time of evaluating loan applications. According to Abid
(2020), the most common factors are literacy, size of land, marital status, and
distance to a lending institution, age of the borrower, caste, religion, and
value of assets held by the household. Another study in Nigeria found a
significant relationship between gender, marital status, the lack of a
guarantor and access to credit (Ololade & Olagunju, 2013). In addition to
gender, agricultural experience, education level, farm size, and income,
household size and availability of collateral have a meaningful effect on loan
accreditation for farmers (Abbas, Yuansheng, Feng, & Liu, 2017).
From the review, these factors were classified into three
groups based on common characteristics, characteristic linked to the farmer
(Gamage, 2013; Ololade & Olagunju, 2013; Ijioma & Osondu, 2015), others
linked to the farm (Aliero & Ibrahim, 2011; Gamage, 2013), and finally
those linked to the economic activity (Meyer R. L., 2007; Gamage, 2013), as
shown in the Table4 3.

18
4 Source: The Author
Summary of different research on the determinants of access to
credit
Table 3: Determinants of credit Access

19
Farmer
|
Farm
|
Economic Activity
|
·
|
Education
|
·
|
Land Size
|
·
|
Cash inflows and
|
·
|
Marital status
|
·
|
Ownership type
|
|
outflows
|
·
|
Household size
|
·
|
Age of the firm
|
·
|
Source of income for
|
·
|
Years of experience
|
·
|
Sector
|
|
repayment
|
·
|
Being member of a cooperative
|
·
|
Location of the business
|
·
|
Availability of audited
|
·
|
Health status
|
·
|
Firm Performance
|
|
financial statements
|
·
|
Gender
|
·
|
Distance to a lending
|
·
|
Value of assets
|
·
|
Characteristics of the borrower
|
|
Institution
|
·
|
Availability of collateral
|
|
(caste)
|
|
|
|
|
·
|
Religion
|
|
|
|
|
·
|
Guarantor
|
|
|
|
|
2.6. Literature on AVCF
2.6.1. Gap of the Literature on AVCF
Recent studies have focused on the determinants of the sources
and amount of agricultural credit (Yadav & Sharma, 2015), and on evaluating
the access to finance at the farmers' level (Gamage, 2013). However, few
researchers have taken into consideration the contribution of financial
institutions (FIs) and the identification of the supply-side determinants of
agricultural credit (Meyer R. L., 2002; Yadav & Sharma, 2015). This could
be an area of further potential investigation, mainly, in new approaches such
as AVCF (Villalba, Venus, & Sauer, 2021).
According to Zander (2016), the research available on AVCF is
relatively scarce due to a number of major concern that restricts the knowledge
about non-bank based agriculture value chain financing. First, the hesitation
of the private sector and agri-businesses to share their information with
others due to confidentiality issues. Second, the hesitation of FIs to share
operational and performance details of their agricultural portfolio due to
confidentiality. Finally, the hesitation of analysts and authors to disclose
details on outcomes and impacts, since the initiatives are new and the results
are not sufficiently robust.

20
With respect to the adequate information on this topic, there
is a significant gap in the available literature overall. The influences of the
AVCF are little reported in the literature, even though external facilitation
needs strict monitoring of the impact of the AVCF, especially for small-scale
producers
2.6.2. Available literature on AVCF
Regarding the Types of the existing literature on AVCF, there
are three types of documents available (Zander R. , 2016):
a) Normative information and guidance
b) Descriptive documents with facts and figures
c) Anthropological and sociological studies
Concerning the studies available on the development of AVCF in
the literature, Miller and Jones (2010) discuss 5 cases of AVCF involvement; 1.
Numeric project in Kenya and Tanzania 2. Inventory credit system Niger 3.
Integrated agri-business finance model 4. Technological innovations in Kenya 5.
Integrated Agro-food in India. The analysis of actual cases and results in this
paper does not show the aspect of the implications of AVCF on agricultural or
financial sector development (Zander R. , 2016).
KIT and IIRR (2010) address the financing gaps that exist
within agricultural value chains. This book presents detailed case studies.
These include sections on results, impact, threats and challenges, and lessons
learned. Among the cases discussed we can mention; Credivida in Peru, BASIX
group in India, K-Rep Group in Kenya, Organizations supporting the soybean
value chain in Ethiopia, UCPCO and Fondo de Desarrollo Local in Nicaragua, and
Pro-rural In Bolivia.
A publication of Inter-American Development Bank (2010) has
highlighted the relationship between AVCF and local financial and agricultural
sectors. This report compared two value chains in Nicaragua (dairy and
plantains) and Honduras (plantains and horticulture) and included relative
observations on mechanisms used within the value chain financing (Coon,
Campion, & Wenner, 2010).
Another FAO publication of Da Silva and Rankin entitled
«Contract Farming for Inclusive Market Access» presented case studies
of different value chains of cacao, sugar, oil palms, and other plantations
crops which work with international markets under contract farming between lead
firms and cooperatives. This report emphasized the importance of this
instrument which is a very interesting development tool with growing
expectations for VC promoters (Da Silva & Rankin, 2013).

21
A recently published discussion paper from the Deutsches
Institut für Entwicklungspolitik, discusses the implications of the AVCF
arrangements for agricultural sector development and presents strategies that
reduce the risks for financial sector growth, with the aim of better reaching
priority segments of the rural population such as small-scale farmers and rural
micro and small enterprises (Zander R. , 2016). This paper illustrates in
detail the following 4 case studies: 1. accelerating production and
post-harvest infrastructure in Rwanda 2. Fostering AVCF in Ethiopia 3. COMPACI
project in Zambia 4. KELIKO farmer association in South Sudan.
The following table shows the different publications available
from which we have selected all the FIs that have experience in the application
of the AVCF. These FIs will be contacted for the survey later
Table 4: Number of FIs mentioned in the literature
review
Publication
|
Authors
|
Year
|
No. FIs
|
AVCF: Tools and Lessons
|
Miller and Jones
|
2010
|
45
|
Value Chain Finance
|
KIT and IIRR
|
2010
|
28+(25)
|
Financing Agriculture Value Chains in Central
America
|
Coon, Campion and
Wenner
|
2010
|
8+(27)
|
Agricultural finance for smallholder farmer
|
Daniela Röttger8
|
2015
|
8
|
Contract Farming for Inclusive Market Access
|
Da Silva and Rankin
|
2013
|
3+(17)
|
Risks and Opportunities of Non-Bank- Based
Financing
|
Zander Rauno
|
2016
|
17
|
Consultative Group to Assist the
Poor9
|
N/A8
|
2015-2016
|
44
|
World Council of Credit Unions9
|
Elissa McCarter8
|
2021
|
48
|
Total of FIs mentioned in the literature
review:
|
201
|
5 Financial institutions mentioned by Miller and Jones (2010)
8 FIs cited in bibliographic studies where the term AVCF is not
mentioned
9 Source: CGAP, 2016 Assessed: 31
July 2021 See:
https://www.cgap.org/small
holders data portal/ 9 Source: WOCCU, 2021
Assessed : 25 August 2021 See: https://www.woccu.org/

22
3. Methodology and Data
Our research aims to examine how FIs can offer finance to
support the actors along the value chain utilizing an AVCF approach. To our
knowledge, no previous research has investigated how FIs use AVCF to support
their clients.
3.1. Description of the study
The study presented in this master's thesis is based on data
collected by the author between July and September 2021 from financial
institutions' websites in several developing countries in Africa, Asia,
Oceania, and South America. It aims to highlight the extent to which the
financial institutions can attract smallholders' farmers and agribusiness to
benefit from the study presented in this master's thesis heir services.
In total, 106 countries are included in this database
[Annex B]. Among them are 98 developing countries and 8 developed
countries, respectively Belgium, France, Germany, Italy, Luxembourg, the
Netherlands, the UK, the USA.
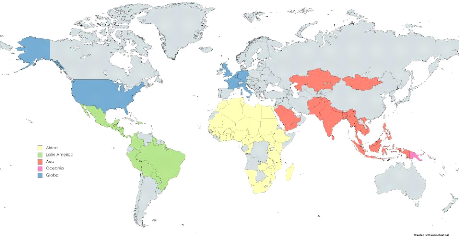
Figure 6: Geographical location of the financial
institutions
This database contains not only general information about the
FIs, but also specific information detailed in the following section. This
database will be used in the future to conduct a survey to enquire about the
application of AVCF by FIs in several languages for the convenience of the
participants.

23
3.2. Building the database
Increasing numbers of FIs in the global south are involved in
the agricultural sector and report their progress in recent years (Gonzalez
& Rosenberg, 2006). The following database encompasses initially
agricultural Banks. Afterward, it has been extended to reach Commercial Banks,
state development banks, non-bank financial institutions (NBFIs), leasing
companies, microfinance institutions (MFIs), credit unions, insurance
companies, NGOs, international financial institutions, credit unions, and
agricultural cooperatives. The purpose of investigating different FIs with
different branches is to enquire if they apply agriculture value chain
finance.
The research purpose consists in reaching the maximum number
of FIs in the Global South and collecting the maximum amount of information
about these FIs to facilitate sampling later. Qualitative methods were employed
to identify FIs, their type, foundation year, number of branches, and if
available agricultural credit, gender programs, and digital solutions. I used
several strategies to create the database starting with the common databases
available, websites, and especially the FIs cited in the bibliographic studies.
The database sources are:
I. Finance in Commons6: a website that provides
limited information on many Commercial, Housing, agricultural, Export, and
Local Banks. This database contains 39 agricultural banks worldwide, with $
1,221,281 MIs USD total assets and $ 49,306 MIs USD total equity. The database
is collected as part of the research program «Realizing the Potential of
Public Development Banks and Development Finance Institutions for Achieving
Sustainable Development Goals» launched by the AFD, INSE, and IDFC. The
database is published annually and can be found online at PDBs database
The rest of the information includes net Interest Income, net Profit, and level
of income (AFD, INSE & IDFC, 2021).
II. Case studies from the Literature Review: All FIs
mentioned in the literature offering finance with a VC approach were
included (Table 4; section 2.8.2). These FIs show at least one application of
the AVCF concept in one or more countries (case of an international
institution). Moreover, other institutions cited in surveys with MFIs, studies
on the agricultural credit, research on access to bank finance, and paper on
credit policy were taken into consideration for the database.
6 Source: AFD, 2021 Accessed: 20 July 2021 See
https://financeincommon.org/pdb-database
III. FIs' websites: For more information on the FIs, their
official website is consulted to determine the contact (Email), the
responsible person or the CEO, and the availability of agricultural loans or
agribusinesses loans.
IV. Internet search engines: to identify all FIs that fund
agricultural projects in the French and Arabic speaking countries we have
searched for each country with these terms «agricultural»,
«banks», «MFIs», «financing»,
«agriculture», «agricultural», «sector», and
«country name».
The following table shows the sources of FIs for the composition
of the database:
Table 5: Composition of database
FIs Sources
|
No.
|
Finance in common
|
37
|
Case studies from the Literature Review
|
201
|
FIs' websites & Internet search engines
|
109
|
Total FIs
|
347
|
I
II
III & IV

24
After eliminating double counting, these sources have been
consolidated into a single database that includes about 347 FIs. The database
covers the country of origin of every FI, acronym, foundation year, the number
of branches, name of CEO if available, E-mail, and website. These contacts were
determined with the aim to survey the role of financial institutions in Value
Chain Finance in the Global South.
In addition to the FIs, the database covers development funds,
NGOs, and international financial institutions such as IFC and IFAD which
finances agriculture and supports the value chain approach in many countries.
There are thousands of other financial institutions that have been financing
the agricultural sector for hundreds of years without being considered
participatory in the AVCF (Zander R. , 2016). Furthermore, the database
captures only a small fraction of funding bodies and relatively little
information about the participating institutions.

25
3.3. Statistical Analyses
3.3.1. Qualitative Analyses:
The term qualitative data refers to any data which is not
numerical. Our case involves geographical variables, the types of institutions,
the availability of agriculture loans, gender, and digital solutions. Depending
on our objectives and research questions, we will focus only on thematic
analysis from the six most popular qualitative methods listed below.
Table 6: Most popular Qualitative Analysis method
Qualitative Analysis Method
1 Content Analysis
2 Narrative Analysis
3 Discourse Analysis
4 Thematic Analysis
5 Grounded Theory
6 IPA: Interpretative Phenomenological
Analysis
Thematic Analysis: This method focuses on
themes and patterns and examines meanings in a dataset. Essentially, this kind
of analysis draws similarities from a large body of data that is often quite
large and arranges them into themes. By focusing on specific themes, the study
makes sense and has more meaning. It also allows us to compare several sets of
variables. In this case, it would be feasible to review a few hundred FIs'
websites to identify which one deals in agricultural credit. This would be an
opportunity to find out also about the offers, services, and orientation of
FIs.
3.3.2. Quantitative Analyses
In quantitative analyses, we analyze data that is numerically
based, or data that can be translated literally into numbers without losing the
context, such as the gender in our case. This method is employed in the current
thesis for three purposes; namely, to measure differences between groups, to
analyze relationships between variables, and to test hypothesis. These tasks
will require a combination of descriptive statistics and inferential
statistics. The differences between the two are that descriptive statistics
focus on describing the contents of the sample, while inferential statistics
seek to make predictions about the population.
The population in this context refers to the full set of
financial institutions that we are interested in researching. However, we can
only have access to a subset of these institutions. The reduced sample size is
explained in detail in Section 3.5.2 of the report. In order to answer our

26
research question, we will use both types of quantitative
analysis. The following table explores both types of statistics.
Table 7: Quantitative statistic types
descriptive statistics inferential statistics
1) Mean (average)
2) Median (midpoint)
3) Mode (most common)
4) Standard deviation
5) Variance
6) Skewness
|
1) Correlation analysis
|
There are many advantages to using descriptive statistics.
Among them are that they provide a macro and micro picture of data, facilitate
spotting errors and anomalies, and suggest which inferential statistics to use.
In the other hand, inferential statistics determine predictions between two or
more groups and most important relationships between two or more variables.
3.3.3. Cluster Analysis
Using Excel functions will enable us to do descriptive and
inferential statistics, but we will need to use the software RStudio to run
cluster analysis and process complex functions.
A cluster analysis or clustering is a data mining process in
which a set of observations is grouped so that elements belonging to the same
group are more similar than those in other groups. These groups are known as
clusters (Vandeginste, et al., 1998). These are the two methods used to analyze
clusters: K-means Clustering and Hierarchical Clustering. Here's how both
methods work:
a) K-means Clustering Method: This algorithm is usually known
as K-means, which is based on centroid values. The idea is to define k
centroids, one for each cluster. After this, each point is associated with the
nearest centroid within a data set. In the absence of pending points, a
premature grouping is done in the first step. Here, the algorithm relates the
new centroids to the new cluster centers determined from the previous step. The
algorithm stops when no further changes are observed between one iteration and
the next (Vandeginste, et al., 1998).
b) Hierarchical Clustering Method: It is expressed as a tree,
also known as a dendrogram, and it involves the development of a hierarchical
classification. It involves the division of large groups into smaller ones.
They are then split again until there is only a single meteorite in each group
(Vandeginste, et al., 1998).
3.3.4. Data Types and Variables
Along with the bank's name, the continent, and the acronym,
there are ten additional variables highlighted in the search. Of them, seven
are completely accessible on the official websites of the financial
institutions, and three are partially accessible. The table below shows the
variables and the method for finding them.
Table 8: Data types
Variables Search Method Name/ Scale
Continent Institutional Type Foundation year Number of
Branches Agricultural Loans Gender
Digital solutions
Total assets
Total Equity
Gross Loan Portfolio
checking the institute's website C 0-3
checking the rubric «about us» T 0-11
checking the rubric «our history» F Year
checking the rubric «locate us» B Number
Checking the rubric «Products and Services» AL1, AL2
0-1
Checking the rubric «our value» and «events»
G1, G2, G3 0-1
Verifying if «online banking» or other e-products DS1,
DS2, 0-1
available DS3
investigating the most recent «annual report» AS $
investigating the most recent «annual report» EQ $
investigating the most recent «annual report» LP $
To capture the variety of information, several attributes (dummy
variables) and sub-variables have been assigned to the seven previous variables
according to the following tables.
Table 9: Continent Attributes
Continent Attribute
Africa
|
0
|
Asia
|
1
|
South America
|
2
|
Global
|
3
|
Each continent (C) has been assigned a unique attribute between 0
and 3 as show in the previous table.
Table 10: Institutional Type Attributes
Institutional type Attribute
0
1
2
3
4
5
6

27
Agricultural Bank Commercial Bank cooperative
Credit union
Development Bank Insurance company
International FI
International Fund Islamic Bank
MFI NGO
7
8
9
10
state development bank 11
Each Institutional type (T) has been assigned a unique attribute
between 0 and 11 as show in the previous table.
Regarding Foundation year (F) Number of Branches (B), As the data
are numerical, they will be analyzed directly.
Table 11: Agricultural loans Attributes
Agricultural loans Attribute
Farmer credit
|
AL1
|
Agri-business credit
|
AL2
|
Farmer credit, AL1: The financial
institutions included in this database provide a range of financial products,
such as consumer credit, advances, salary advances, mortgages, car loans, or
asset acquisitions as well as cashback loans. Apart from these loans, some FIs
offer specific services and products to farmers, including Farm input loans to
meet the need for financing inputs (such as seeds and chemicals), machinery,
labor, and harvesting. Further, agricultural credit tailored to meet any
economic need of the farmer, such as the purchase of merchandise or other
income, with flexible amounts and long-term terms and fast processing is
offered. During data processing, we will assign "1" to AL1 where institutions
offer agricultural credit to small-scale farmers, and "0" to AL1 when no such
credit is offered, or the offer is restricted to the above-mentioned
non-agricultural credit.
Agribusiness Credit, AL2: This is a loan
designed to help support the value chain players in the agricultural inputs and
equipment manufacturing, stocking, importing, exporting, trading, and storing
sectors. The credit facility is intended to facilitate agri-business
enterprises and finance working capital and operational needs across the value
chain. Beneficiaries may include agri-dealers, agro-processors, input
manufacturers, and agro-importers and exporters. If there is an agribusiness
credit, a "1" will be assigned and if there is none, a "0"
Table 12: Gender Attributes
Gender Attribute

28
Credit facility for women
|
G1
|
Career development to female staff
|
G2
|
Gender Programmes
|
G3
|
Credit facility for women, G1: Many financial
institutions have developed direct lending programs targeting women in the
agricultural value chain. The objective is to finance women-led small and
medium enterprises, strengthen their inclusion in agriculture, and encourage
them to engage in commercial activities. In this case, a «1» will be
assigned to G1, and «0» otherwise.
Career development, G2: Some financial
institutions promote gender equality at work, which means that women can enjoy
the same rewards, opportunities, and resources as men at a company, which
include equal pay and benefits for comparable jobs with comparable
responsibilities, equal opportunities for promotions and career progression,
and equal consideration of needs. G2 will be assigned "1" in this instance and
«0» otherwise.
Gender Programmes G3: Other banks offer
gender programs and celebrate events such as International Women's Day, sign
agreements with associations protecting women's rights by offering specific
services. In addition, they allow women to participate in a draw to win a
savings account or a scholarship dedicated to the education program abroad.
Other initiatives are available for women who excel in their
studies to participate in workshops with senior executives and receive answers
to their bugging questions. This aim is to empower youth females in taking a
strong stance on their future. G3 will be assigned «1» in this case
and «0» otherwise.
Table 13: Digital Solutions Attributes
Digital solution Attribute
Online Banking E-Products:
DS1
DS2
Online Loan Application
|
DS3
|
Online Banking DS1: Some financial
institutions offer a secure online banking platform that could be used to
access banking services efficiently. The portal offers clients the ability to
review account balances, obtain statements, send money, pay bills, and review
transaction history. DS1 would receive a "1" if this were available; otherwise,
it would receive a "0".

29
E-Products: A variety of products are
available to clients to keep them informed and simplify banking. Email banking,
in which the account history over a specific period is sent. Mobile banking
features include SMS alerts for withdrawals and deposits, as well as debit card
transactions, funds transfers, and other transactions. Users can also access
and manage their savings accounts and transfer money from their account to a
beneficiary or anyone else using mobile banking. A "1" will be assigned to DS2
if one of these facilities is available, and an "0" if not.

30

31
Online Loan application: In several financial
institutions, clients can apply for a personal loan online, securely, and
easily. The process is fast and secure. Once the loan is submitted, the details
of the facility fees and due date will be displayed, and SMS confirmation will
be sent. DS3 will be assigned "1» in this case
Concerning international financial institutions, the number of
agencies is being replaced by the number of countries in which it operates or
the number of foreign offices. For Gender and Digital Solutions, it was checked
whether the project focuses on women or presents a digital footprint of its
approach. Lastly, the total assets and capital are substituted by the total
amount granted by the institution for the project.
Concerning the last three variables (Total assets, Total
Equity, Gross Loan Portfolio) and due to the lack of these information in
several financial institutions, we will not consider them during the
analysis.
3.3.5. Other components of the database
Additional data in the database include the institute's
website, its general email address, the name of a CEO/manager who works in the
institute, and if available, his personal contact information. The last cases
are attributed to mentions of the institute in the literature and contact
information of the author.
3.4. Structure of survey
Quantitative methods were used in a survey in order to measure
the role of FIs in facilitating the AVCF in the Global South (GS). The purpose
is to determine the financial products, credit screening and scoring for
agriculture loans, mechanisms, and Instrument used by FIs in the aim of
reducing TC, managing risks, and assisting small-scale farmers to access
financing.
Online Survey: conducted in the Global South
(GS) with FIs which apply and do not apply the AVCF. To define the survey
questions, an extensive and careful review of the scientific literature will be
carried out to check standard questions that have been used and employed by
major survey, then develop the most appropriate questions. The period of the
survey was in July-September 2021.
The survey contains 5 sections:
1) General information about FIs
2) Economic information
3) Agricultural credit: screening, scoring and monitoring
4) Agricultural finance within value chains
5) Financial product & Instrument employed
A standardized survey was designed using the platform
Qualtrics used to create this survey, number questions, generating test
responses and reviewing survey accessibility. Therefore, to facilitate data
processing and for a quick tabulation of results, only multiple-choice
questions will be used, and before starting the survey, a pretest of the survey
and the pre-coding of these closed ended questions will be carried out.
Finally, the data collected was analyzed using Microsoft Excel and R for result
generation. This resultant analysis of the data will make it possible to draw
conclusions about the effectiveness of the use of the Agricultural Value Chain
Finance (AVCF) by financial institutions (FIs). This assessment provides
evidence to decision-makers, development agencies, and all types of rural FIs
and shows priority areas that require intervention regarding the accreditation
of credit for small-scale farmers.
3.5. Sample Design
3.5.1. Sampling frame
This database includes several independent financial
institutions from different provenances in the Global South with a total sample
size of 347 institutions. It was designed to produce reliable estimation at the
international level. Accordingly, the universe for the database includes FIs
mentioned as per criteria identified in table 5; FIs from the platform Finance
in Common, FIs from literature reviews on AVCF, FIs in other surveys and
studies that do not include the AVCF methodology, and FIs from the internet
search engines.
According to Dados & Connell (2012), The term "Global
South" commonly refers to regions in Latin America, Asia, Africa, and Oceania.
It is part of a family of terms, including "Third World" and "Peripheral," that
refer to regions outside of Europe and North America that are considered mostly
low-income and often politically or culturally marginalized. The use of the
term «Global South» reflects a shift from a central focus on
development or cultural difference to a focus on geopolitical power relations.
Our sampling frame for the database included countries around the globe with at
least one FI that funds the agricultural sector in the Global South even if the
FI is not a member of this zone. The next figure shows the classification of
the chosen countries by continents.
Database: 347 financial institutions across the
5 continents:
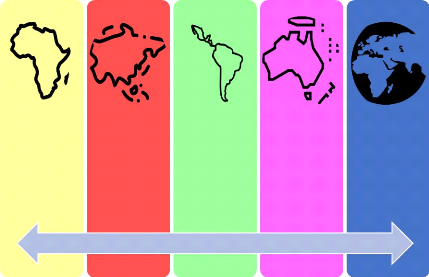
Africa
Sample FIs: 200
Asia
Sample FIs:
52
Latin America
Sample FIs:
63
Oceani
a
Sample FIs:
16
Global
Sample FIs:
16

32
Figure 7: Geographical distribution of financial
institutions
3.5.2. Sampling techniques
The total sample size of 347 FIs was divided into two
categories based on their source. The categories are FIs encountered while
conducting the bibliographic study and FIs obtained from other sources (Google
& Platform: Finance in Common). It was decided to eliminate the second
group (FIs not cited in the literature) as a means of reducing the sample size
and facilitating processing. As a result, the sample (202 FIs) will be
considered for time considerations. The exemption will apply only to financial
institutions that lack an official or functional website. Due to the different
orientations and types of agricultural interventions of these FIs, the
differences in information available, the difficulty of gathering the same
information under the same categories chosen, and above all to give meaning to
the descriptive analysis, the final data sample will be reduced by 57 FIs.
In this case, the snowball effect will be used to construct
this database. The database is then finalized by separating each FIs through
the source, official website & Information available, and type of
intervention in the agricultural sector. To account for the unavailability of
the official website, missing information about the FIs, the target sample size
was decreased to 144 FIs assuming a participation rate of 42%.
FIs website were checked and validated before it was added to
the database. Figure 8 shows the selected criteria and summarizes the
stratification method.
1st Stratification (FIs Sources)
FIs from the Bibliography
Google & Finance in Common

2nd Stratification (FIs officials website)
Global South regions
Funding Agriculture

3rd Stratification (FIs intervention type)
Local FIs
International FIs

33
Figure 8: Listing criteria of financial institutions in the
final database
The full information about the database can be found in Annex
B. 3.5.3. FIs Listing:
A spreadsheet with FI's data was listed in an excel file as
described int the previous section. There are six sheets in this Excel file.
First, we provide a summary of the general classification of each FI, and we
explain the different colors and abbreviations used. A further four Excel
sheets bearing the names respectively Africa, Asia, America, and Oceania were
then created, holding FIs from the search engines and from the finance in
common platform. Finally, the sixth Excel file contains the final sample with
all the information required for data processing.

34
4. Survey Design and Conceptual Framework
4.1. Exploring other surveys
4.1.1. Survey with FIs
In the bibliographic reference, only an interview made by
Röttger (2015) was conducted with FIs providing agricultural finance to
smallholders' farmers in Uganda, Kenya, Benin, and Cameroon. The FIs involved
are two commercial banks (CB), three microfinance Companies (MFCs) and three
membership-based financial institutions (MBFI).
This survey7 contained primarily general
information about FIs such as the country of origin, year of establishment,
total assets, Gross loan portfolio, deposits, number of clients and branches.
Then, the author has determined the percentage of the agriculture, loan
portfolio of the total loan portfolio for the interviewed FIs and classified
their loan product as a short-term loan (=12 months) and medium-term loans (=12
months).
Table 14: financial Institutions General
Information
Financial Institutions General Information
|
· Country of Origin
· Year Of establishment
· Total assets
· Gross loan Portfolio
· Deposits
· Number of clients
· Number of branches
|
Source: Röttger (2015)
|
|
The author has identified the specific loan features for both
short-term and medium-term loans such as Loan amount, Loan term, repayment
schedule, collateral requirements and joint liability and other features. She
has found that these FIs imply low entry barriers, more specifically for
short-term loans, flexible repayment schedule, collateral requirements like
group lending, and cash collateral and personal guarantors
Meanwhile, the authors of KIT and IIRR (2010) have listed the
key characteristics used to depict financial product. These are amount and
period of the loan, the disbursement, the way of repayment, the interest rate,
and transactions cost.
7 Source: UMM Thematic Paper Assessed: 21 July 2021
See
https://www.e-
mfp.eu/sites/default/files/resources/2015/03/UMM%20Thematic%20paper%202014
frankfurt final.pdf

35
Following the review of the two references, the specific loan
features are summarized in the following Table.
Table 15: Specific loan features
Loan Features
|
· Loan amount
· Loan term
· Repayment schedule
· Collateral requirements
· Liability
· Other features
|
Source: Röttger (2015) & KIT and IIRR (2010)
|
Through these previous studies, we can conclude that there is
consistent evidence that FIs play not just an important role in financing the
agricultural VCs but engage with chain actors to develop new financing models,
mitigate risks more effectively, and lower transactions costs. Nonetheless,
there is a lack of robust research on the paramount role of FIs in implementing
approaches such as AVCF, and the mechanisms used by FIs concerning credit
screening, scoring, rating tools, and portfolio monitoring practices, which
should be improved, developed, and replicated.
4.1.2. Survey with farmers (World Bank &
CGAP)
The World bank8 in cooperation with the
Consultative Group to Assist the Poorest (CGAP) conducted a nationally
representative survey of smallholder farmers in Bangladesh, Ivory Coast,
Mozambique, Nigeria, Tanzania, and Uganda. The survey investigated the farming
and non-farming activities, financial practices, and interests, as well as
challenges and aspirations of smallholder families at the national level in six
countries. Besides, this survey in each country has a nationally representative
sample of about 3,000 families who indicated that agriculture makes a
significant contribution to their household's livelihoods, income, or
consumption. In total, more than 300,000 data points on the financial lives of
smallholder families were collected from household surveys (World Bank and
CGAP, 2017).
This Smallholder Families Data Hub provide data about the
Poverty Status, Income Sources, Regular Expenses, Household Segmentation, Crops
and Livestock, Saving for Agriculture, Agricultural Markets, Financial
Inclusion, Financial Tools, and Trust in Financial Services Providers of
small-scale farmers. This table summarizes the focal points of the above
findings. This research provides more detail on these focal points and profiles
of the four main segments
8 Source: CGAP Assessed: 31 July 2021
See
https://www.cgap.org/small
holders data portal/

36
of smallholder households in the six developing countries.
These findings are designed to identify opportunities for FIs, government, and
NGOs to better adapt the financial tools and meet the needs of smallholder
families
Table 16: focal points of the World Bank Survey
Poverty Status
|
Income Sources
|
Regular Expenses
|
Household Segmentation
|
Crops and
Livestock
|
ü Less than $ 1.25/day
ü Between $
1.25 and $2.50/day
ü Above poverty
|
ü Crops sale and Livestock
ü Business in
Retail and Manufacturing
ü Salary from regular jobs
ü Rent Land
ü Remittances
ü Occasional job
|
ü Pay utility bills
ü Pay a school fee
ü Deposit money
ü Send/receive money
|
ü Farming for sustenance
ü Battling the elements
ü Options for growth
ü Strategic agricultural entrepreneurship
|
ü Growing crops
ü Raising animals
|
Saving for
Agriculture
|
Agricultural Markets
|
Financial Inclusion
|
Financial Tools
|
Trust in FIs
|
ü Very important
ü Somewhat important
ü Not important
|
ü Wholesaler
ü Retailer
ü Direct to public
ü Processor
ü Other
ü Government Agency
ü Cooperative
|
ü Included
ü Not included
|
ü Bank
ü Mobile Money
ü MFI
ü Cooperative
ü Semi-formal savings
ü Post office bank
|
ü Banks
ü Bank agents
ü MFI
ü Mobile money providers
ü Savings groups
|
|
The goals of this Smallholder Survey are first, generation of
a clear picture of the smallholder sector (household demographics, agricultural
profile, poverty status, market access). Second, a segmentation of smallholder
households in the six countries according to the most compelling variables (at
least these focal points) that emerge. Third, a characterization of the demand
for financial services in each segment, considering the needs, attitudes and
perceptions of clients related to both agricultural and financial services.
Fourth, an explanation of the financial needs of each segment and how they can
be met, with both informal and formal service s, and where there may be
promising opportunities to add value (Anderson, 2017).

37
4.1.3. Integrated Financing for Value Chains
(WOCCU)
The publication9 of the World Council of Credit
Unions illustrates how credit unions can fill the agricultural lending hole and
create market links. It explores how these unions can provide value chain
finance to improve productivity, promote economic growth, and ensure food
security among small farmers (WOCCU, 2009). Moreover, a large part of their
success in value chain finance is due to their strong community ties, presence
in rural areas, and experience lending to low-income individuals and small
businesses. Additionally, they developed new risk management strategies for
lending to these producers.
At each stage of agricultural lending, these credit unions
have developed 10 ways to manage risk. The steps involved are:
1. Ensure demand for crops on the market
2. Establish proper policies and procedures
3. Evaluate real financing needs.
4. Assuring individual loans with appropriate guarantees
5. Diversify the portfolio of loans
6. Adapt loan terms based on crop seasons.
7. Using vouchers to distribute loans
8. Encourage farmers to diversify their crops
9. Monitoring crop performance
10. Using the Credit Union to receive payments
This publication argued that value chain finance methodology
can be relatively inexpensive to implement and can be adapted to different
contexts, products, and environments. However, the value chain must be managed
by someone who can bring together, facilitating, and ensuring that business
relations are maintained to result in an efficient value chain that meets all
participants' needs.
4.1.4. Survey on national development bank (World Bank
Group)
The paper summarizes the main findings from the 2017 survey of
the national Development Banks conducted worldwide. Sixty-four DBs were
surveyed from around the world, mainly from middle-income countries. Among the
conclusions in this report is that although DBs tend to be small in terms of
assets, governments use them to provide financial services to sectors or
regions that are not well served by private intermediaries. Additionally,
development banks operate in multiple economic sectors, contribute to global
development agendas, and support
9 Source : WOCCU (2009) assessed : 08/10/2021
https://www.woccu.org/documents/value
chain techguide

38
the private sector within their jurisdictions. Several
institutions that participated in this survey noted that they face various
challenges, including enhancing their risk management capacity and adapting
better monitoring and evaluation frameworks (WBG & WFDFI, 2018).
There are 16 sections in this questionnaire with 138
questions. We see the use of a variety of question types including yes/no,
multiple choice, and open-ended questions. These 16 divisions in this
survey10 are as follows:
1) General information 9) Pricing and subsidies
2) Mandate 10) Profitability, asset quality, and efficiency
3) Size 11) Corporate governance
4) Loan portfolio and guarantees 12) Transparency and
disclosure
5) Countercyclical Role 13) Prudential regulation and
supervision
6) Funding 14) Restructuring
7) Business model 15) Monitoring and evaluation practices
8) Products and services 16) Challenges
Source: World bank Group, 2018
4.2. Credit Scoring for Agricultural Loans
For the stability and the profitability of FIs, loan contracts
must perform well, and the screening of loan applications from agribusinesses
should minimize credit risks and reduce TC. In this regard, a credit analysis
must be done by FIs before making a decision on credit accreditation as part of
the examination process. This credit analysis takes into consideration the
assessment of the financial backgrounds and history of the applicant.
Therefore, a good credit management assists FIs in loan pricing, determining
the amount of credit, reducing the default risk, increasing debt repayment, and
predicting the credit worthiness of borrowers (Limsombunchai, Gan, & Lee,
2005). Even more so, credit scoring not only assist FIs in loan approval, but
also on loan monitoring and assessment of loan portfolio risks (Turvey &
Brown, 1999).
According to Plata and Nartea (1998), credit analysis is the
first step in a loan request. This covers the determination of the candidate's
strength, estimating the probability of failure and reducing to an acceptable
level the risk of nonpayement. In this context, several authors found that this
method based on the evaluation of a loan officer sound to be not efficient
(Crook, 1996; Glassman & Wilkins, 1997).
10 WBG & WFDFI (2018) Assessed 09/10/2021
https://documents1.worldbank.org/curated/en/977821525438071799/pdf/2017-Survey-of-National-development-banks.pdf

39
A study made by Limsombunchai et al. (2005) on the estimation
of lending descision for agricultural loans has enumerated several factors used
by FIs on credit scoring. These factors include borrowers' liquidity,
profitability, solvency, and repayment capacity. These variables can be
inferred directly from the applicant's financial status. In addition to that,
moneylenders consider the farmer's personal attributes, entreprise type,
region, and many other factors mentioned in Section 2.5 (Table 3). On top of
that, the relationship between the bank (lender) and the borrower influence the
availabilty and cost of the loan (Petersen & Rajan, 1994; Harhoff &
Korting, 1998).
Limsombunchai et al. (2005) have developped a logistic model
for the credit scoring which is a function11 of the borrower
characteristics, credit risk, proxies, relationship indicators and dummy
variables. This variables excluded are the estimation of borrower's liquidity
and repayment capacity. The credit scoring models is as follows:
Lending decision = f (Borrower characteristics, Credit risk
proxies, Relationship indicators, Dummy variables)
The variables of the function are summarized in the following
table as well as those excluded from the function.
Table 17: Lending decision variables
Borrower characteristics
|
Credit risk
|
Relationship indicators
|
Dummy variables
|
Excluded variables
|
I Assets I Age
I Education
|
I Collateral
I Return on assets
I Leverage ratio
I Capital turnover
ratio
|
I Borrowing from
others
I Duration
|
I Province
I Farm type
I Loan Type
I Loan size
I Lending year
|
I Borrower's
liquidity
I Repayment capacity
|
Source: (Limsombunchai, Gan, & Lee, 2005, p.1199)
|
According to the authors, these variables are going to be
rated by (+) or (-) in order to determine the lending decision. For example
assets, age, collateral, capital turnover ratio, and duration are positevely
related to the chance of a good credit. However leverage ratio and borrwing
from other are negatively related to the chance of a good credit
(Limsombunchai, Gan, & Lee, 2005).
On the basis of the case studies showing the practice of the
AVCF approach in the following value chain; potato in Peru, milk and organic
quinoa in Bolivia, cotton in Tanzania, sal leaf in India, rice in Rwanda, honey
and tea in Kenya, soybean in Ethiopia, coffe in Nicaragua. The
11 Source: Limsombunchai, Gan, & Lee, 2005, p. 1199 Assessed
: 22 July 2021 See
https://thescipub.com/pdf/ajassp.2005.1198.1205.pdf

40

41
authors of KIT and IIRR (2010) have established the key factors
to depict financial products summed up in the following table12.
Table 18: Loan accreditation Characteristics
Product and financial flows
|
Risk management
|
Information flows
|
ü Purpose
ü Amount
ü Period
ü Disbursement
ü Repayment
ü Interest Rate
ü Transactions costs
|
|
Securitization Liability
|
|
Information required to
apply
Information required during season
Time lag between application and payment
|
Source: (KIT and IIRR, 2010)
|
|
|
On this background of the last reference, we can determine the
factors and variables for Credit screening, scoring and monitoring for
Agricultural loans (Limsombunchai, Gan, & Lee, 2005; KIT and IIRR, 2010).
This will be used in the following section to determine the questions for part
D of the survey
4.3. Financial instruments employed by FIs
There are several ways to categorize the terms and describe
the different financial products and instruments, e.g., Wenner (2006)
categorizes these terms by product financing, receivables financing, physical
asset collateralization, risk mitigation and structured enhancements. However,
Miller and Jones (2010) in their books: «Agricultural Value Chain
Finance Tools and Lessons» have categorized these terms and
conditions differently. They classified the 16 instruments by 5 financial
products as shown in the following Table13.
Table 19: AVCF Instrument
Product financing
|
Receivable's financing
|
Physical asset
collateralization
|
Risk mitigation
products
|
Financial
enhancements
|
|
Trader credit
|
|
Trade
|
|
Warehouse
|
|
Insurance
|
|
Securitization
|
|
Input supplier
|
|
receivables
|
|
receipts
|
|
Forward
|
|
instruments
|
|
credit
|
|
finance
|
|
Repurchase
|
|
contracts
|
|
Loan
|
|
Marketing
|
|
Factoring
|
|
agreements
|
|
Futures
|
|
guarantees
|
|
credit company
|
|
Forfaiting
|
|
Financial leasing
|
|
|
|
Joint venture finance
|
|
Lead firm financing
|
|
|
|
|
|
|
|
|
Source: (Miller & Jones, 2010, p. 56-57)
|
|
|
|
12 Source: KIT and IIRR, 2010
Assessed: 25 July 2021
See
https://www.kit.nl/wp-content/uploads/2018/08/1610
chainfinance-d8.1.pdf
13 Source: Miller & Jones, 2010
Assessed: 22 July 2021
See
http://www.fao.org/3/i0846e/i0846e.pdf
The authors have mentioned that the use of these terms'
changes according to the countries, moreover it can have other appellation and
application of the instruments beside other legal terms. This table includes
the traditional forms of credit and other more sophisticated and complex forms,
and therefore some instruments may not be applicable to small-scale farmers,
but on the other hand applicable for agro-industries and wholesalers. These
sophisticated high-level instruments can in any case stabilize prices and
reduce financing risk (Miller & Jones, 2010).
4.4. Survey design for FIs officials
After reviewing the literature and exploring several
references, considering the content of other surveys, and the revision of
determinants of agriculture credit, credit scoring mechanisms and the different
instruments presented early, we designed the online questionnaire for bank
officials Annex C. This was based on the consideration of other surveys
oriented for both farmers and FIs. To capture the complexity of the FIs survey,
the online questionnaire consisted of five sections already announced in part
3.4 [structure of the survey]. For each FIs, an online questionnaire was
administered to the CEO or head of office or director, to collect the
information.
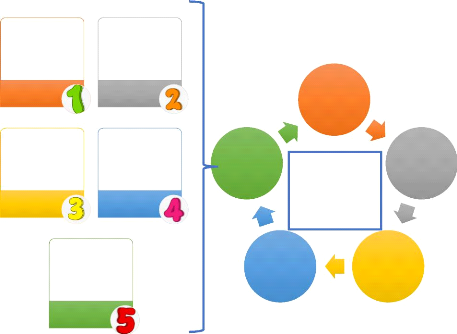
· General Information
· Agricultural finance
Section
Section
· Financial product
Section
· Economic Information
· Credit scoring
Section
Section
most financial instrume nt employed
Loans approval
Data from the five
sections generates relevant information about FIs
agenda
Major FIs informati on: Country & Type
way of using the concept AVCF
business of FIs; branches
& Clients
Figure 9: Financial institutions Questionnaire
components

42
The FIs questionnaire collect first General & Economic
Information, on whether each FIs contributes to VCF or participate to the AVCF.
The information was later used to identify how FIs apply the approach AVCF in
the Global South. Information on assets and Branches were also collected to
derive the socioeconomic status of the FIs and the priority of financing the
agricultural sector. In addition, credit accreditation criteria, financial
products, and instrument employed were also enquired to find out how the FIs is
funding rural producers. The below figure summarizes the sections of the
survey.
All 5 sections were translated in Arabic, French, and Spanish,
then pretested in August 2021. After the pretest, debriefing Sessions were held
with university staff and Experts to modify the online questionnaire based in
the observations and the Feedback from the pretest. Next the questionnaires
were finalized and a script in the platform Qualtrics were programmed to
facilitate data collection. The script was tested and validated by the
Supervisors.
The following is in an explanation of theses 5 sections
building on other studies. This methodology was designed to answer a few
questions about the activities of FIs in the agricultural sector in the Global
south.
4.4.1. General Information
This part will be devoted to general information about the
institute and the position of the respondents. This first section permits the
understanding and the segmentation of FIs according to several key
characteristics (e.g., demographics, institutional type, experience in the
agricultural field) and what type of FIs emerge? What is the position of the
person who responded to the survey? How many years has he or she worked in
agricultural finance?
This first part will allow the classification of FIs and then
draw conclusions based on the latter segments.
4.4.2. Economic information
This second section will collect economic information from FIs
such as (Gross loan Portfolio, No of Branches, and No. of Clients) and will
determine the importance of the agricultural sector among the other branches in
terms of budget from capital granted for it and in terms of customers.
Additionally, this part highlights the attitudes and perceptions of FIs. How
they perceive their agricultural activities? What is the priority sector? What
is the penetration rate?
4.4.3. Credit screening, scoring, and monitoring for
Agricultural loans
This part will determine the characteristics of credit
accreditation and evaluate credit screening, scoring, and monitoring mechanisms
for agriculture loans. This will allow us to have statistics on the different
key characteristics on lending decision and to determine the differences in

43
consideration of this criteria between the different countries
and continents. Which criteria does each segment of FIs demand, from the
perspectives of both rural producers (age, education, marital status,
agricultural experience, etc.) and financial products (e.g., amount, term,
repayment schedule, collateral)?
4.4.4. Agricultural finance within value
chains
This section will investigate the FIs-official of the
financial institute on the knowledge and application of the AVCF. This will
help us determine the percentage of FIs that finance agriculture with this
approach and understand the opportunities to improve the financial inclusion
for small-scale farmers and agribusinesses in the one hand, and in the other
hand mitigate risk and reduce transaction cost for the FIs. Where are the
opportunities to add value with the approach AVCF?
4.4.5. Financial product & Instrument
employed
Based on the instruments determined by Miller and Jones
(2010), this last section will investigate the respondents on the instruments
used. What formal suite of financial mechanisms does each segment of FIs
currently use? These 16 instruments were classified into 5 groups: Product
financing, Receivable's financing, Physical asset collateralization, Risk
mitigation products, and financial enhancement as shown in the figure below.
Trader credit
Input supplier
Marketing credit company
Lead firm financing
Trade receivables finance
Factoring
Forfaiting
Warehouse receipts
Repurchase agreements
Financial leasing
Insurance
Forward contracts
Futures
Securitizatio
n
instruments
Loan
guarantees
Joint Venture finance




Product financing
Receivables financing
Physical asset collateralizatio
n
Risk mitigation products
Financial enhancements
Figure 10: List of instruments enquired during the
survey
4.5. Overview of the online questionnaire
During the bibliographic study, few research, discussion, and
paper have identified a gap in the contribution of FIs in the agricultural
credit (Meyer R. L., 2007; Yadav & Sharma, 2015),

44
evoked the needs, desires, and perceptions of FIs. This master's
thesis involved defining the end objective by doing the following:
§ Drawing conclusions from existing survey
§ Considering the objectives and needs of FIs
§ Accounting for FIs-officials Feedback
§ Learning from the ongoing financial agendas in each
continent.
Using these building blocks, a framework for the online survey
was developed to be shared with FIs and to capture all relevant elements of the
AVCF. The framework thus comprised five main sections as previously announced
is summarized by the following figure:
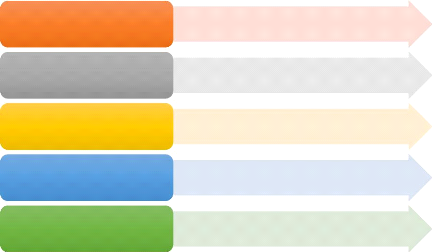
Economic Information
General Information
Agricultural finance
within value chains
Credit scoring
meschanisms · Assess Borrower Characteristics
Instrument
· Identify and profile FIs
· Determine the agricultural experience
· Measure penetration rate
· Find out the priority sector
· Discover Loan accreditation criteria
· Examine the practices of the application of the
AVCF
· Determine the potential products and services
Figure 11: Questionnaire Framework
5. Analysis and Results
5.1. Descriptive Analysis:
5.1.1. Geographic distribution:
When examining the number of financial institutions, Africa
dominates the database, accounting for 47% of all institutions. The Asian and
South American FIs have a relatively low proportion of their cases studied in
this database, at 24% and 20%, respectively. The rest of the database is
composed of international financial institutions (IFI). The global scope of the
IFIs enables them to operate in different developing countries and help promote
agriculture development, sustainable resource management, and resilience. An
overview of the database can be found in the following Table 20.
Table 20: Classification of financial institutions by
continent
Continent
Africa
South America
Asia
Global
Total
No of FIs
|
Share
|
67
|
47%
|
35
|
24%
|
29
|
20%
|
13
|
9 %
|
|
144
|

45
5.1.2. Distribution by institutional type:
Based on the literature review on agricultural finance, our
database suggests that they account for less than ten percent of total FIs.
Most agricultural credit is provided by cooperatives, microfinance
institutions, and partly by commercial banks (Table 20). Financial institutions
in this database were classified according to the descriptions found on their
own websites, predominantly in the rubric "about us" or "history".
To gain an understanding of the most important FIs, commercial bank as
described in the literature are financial institutions that accept deposits,
provide services of checking accounts, offer various loans, and offer products
like personal loans and business loans for both individuals and small
businesses. However, cooperative are organizations where many small farms work
together to produce and sell crops together. The cooperatives also provide the
farmers with short-term agricultural loans which allow them to carry out many
different agricultural and farming activities in the collective. In addition, a
significant share of this database consists of MFI which is an alternative
banking service that is available to unemployed or low-income individuals who
would otherwise have no access to financial services, and development bank
which provides capital to create productive investment opportunities, often in
conjunction with technical assistance. Finally, Islamic bank is a financial or
banking entity operating under shariah (Islamic law) and making

46
profits through equity participation, which entails the
borrower giving the bank a share of their profits instead of paying
interest.
In light of the observations and case studies in this
database, the cooperatives and MFIs do not deliver enough information to
provide a meaningful sample or claim that agriculture financing is primarily
provided by cooperatives. In some cases, cooperative groups are organized and
monitored by non-governmental organizations, in others by international
financial institutions and international funds, and in yet others, commercial
banks lend to the cooperatives that then distribute the funds among the farms
according to various financial institutions' websites which provide information
on this. Additionally, there are cooperatives that collect farm savings and
distribute them as needed in an organized and priority manner to their
registered members.
Cooperation has an important role to play in increasing
agriculture credit availability, connecting commercial financial institutions,
and ensuring small-scale farmers' financial inclusion.
Table 21: Classification of financial institutions by
institutional type
Institutional type Number %
Commercial Bank 48 33%
Cooperative 47 33%
MFI 21 15%
Development Bank 9 6%
Islamic Bank 5 3%
International FI 3 2%
International Fund 3 2%
Agricultural Bank 2 1%
Credit union 1 1%
Insurance company 1 1%
NGOs 2 1%
State development bank 2 1%
Total 144
5.1.3. Foundation Year:
As shown in Table 22, according to this database, 42% of the
financial institutions have been established since 1990. The rest of the FIs
are over 50 years old. Furthermore, when examining the data, there are no
conclusive evidence that financial institutions invest in agriculture after
several years in the banking industry.

47
Table 22: classification of financial institutions by
foundation year
Foundation Year
|
Frequency Percentage
|
|
Before 1950
|
19
|
13%
|
1950 - 1970
|
32
|
22%
|
1970 - 1990
|
33
|
23%
|
After 1990
|
60
|
42%
|
Total
|
|
144
|
5.1.4. Number of Branches
Based on analysis of the number of branches per FIs, we find that
29% have less than 10 branches, 44% are in the interval [10-100], and 22% have
between 100 and 1000 branches. Eventually, only eight FIs in this database have
more than 1000 branches in the origin country.
Table 23: Classification of financial institutions
according to the number of branches
Range
|
Africa
|
Number of Branches of FIs per Region
America Asia Global Grand Total
|
Percentage
|
< 10
|
23
|
12
|
5
|
2
|
42
|
29%
|
10 - 100
|
31
|
14
|
13
|
5
|
63
|
44%
|
100 - 1000
|
12
|
7
|
7
|
5
|
31
|
22%
|
> 1000
|
1
|
2
|
4
|
1
|
8
|
6%
|
Grand Total
|
67
|
35
|
29
|
13
|
144
|
100%
|
According to Table 23, more than half of the individuals in
this simple with more than 1000 branches are from Asia. Several factors can
explain this, including the high concentration of population in India.
5.1.5. Agricultural loans
A total of 84 Farmer Loans (AL1) and 24 Agribusiness Credit
(AL2) were found among the 144 FIs in this database. There are several
important institutions contributing to this financial offer - mostly
cooperatives. They hold the top position with 37 AL1 and 5 AL2 (for value chain
players), followed by commercial banks with 16 loans for farmers and 14 for
value chain players. Next come the microfinance institutions, which offer 15
agricultural loans and 5 chain loans. Both types of loans are provided by all
agricultural banks.
As for the other institutional types, the agricultural loan
offer is either minimal or absent since development banks or international
funds provide these loans through other organizations or financial institutions
including cooperatives and micro enterprises.

48
Table 24: Percentage of credit offered by type of financial
institution
Type
|
Sum of AL1
|
% AL1
|
Sum of AL2
|
% AL2
|
Agricultural Bank
|
2
|
100%
|
1
|
50%
|
NGOs
|
2
|
100%
|
0
|
0%
|
International Fund
|
3
|
100%
|
0
|
0%
|
cooperative
|
37
|
79%
|
5
|
11%
|
MFI
|
15
|
71%
|
5
|
24%
|
International FI
|
2
|
67%
|
0
|
0%
|
State development bank
|
1
|
50%
|
0
|
0%
|
Development Bank
|
4
|
44%
|
3
|
33%
|
Islamic Bank
|
2
|
40%
|
0
|
0%
|
Commercial Bank
|
16
|
33%
|
14
|
29%
|
Credit union
|
0
|
0%
|
0
|
0%
|
Insurance company
|
0
|
0%
|
0
|
0%
|
Total
|
84
|
|
28
|
|
Table 24 shows that all agricultural banks, international
funds, and NGOs offer credit to small-scale farmers in this database, although
cooperatives and MFIs are more likely to use this form of credit. International
funds are slightly less prominent in this database. In terms of agribusiness
loans (AL2), only 50% of agricultural banks offer these services, 29% of
commercial banks, 33% of development banks, and 24% of MFIs.
5.1.6. Gender Equality
For gender equality, the 144 FIs sample covered 22 Credits
facility for women (G1), 15 Career development opportunities to female staff
(G2), and 43 Gender Programmes (G3).
Generally, other institutional types have little direct
interaction with VCs and are more concerned with regional development. Because
of this, these institutions almost have no gender-related offerings.
As a result of reviewing table 25, we can learn that 50% of
agricultural banks and NGOs offer women-credit, 50% of nongovernmental
organizations and 50% of state developmental banks provide gender equal
employment opportunities and more than 50% of international funds, Islamic
banks, and nongovernmental organizations provide assistance and programs to
women.

49
Table 25: Percentage of gender equality program offered by
type of financial institution
Type
|
Sum of G1
|
%G1
|
Sum of G2
|
%G2
|
Sum of G3
|
%G3
|
Agricultural Bank
|
1
|
50%
|
0
|
0%
|
0
|
0%
|
NGO
|
1
|
50%
|
1
|
50%
|
1
|
50%
|
International FI
|
1
|
33%
|
1
|
33%
|
1
|
33%
|
International Fund
|
1
|
33%
|
0
|
0%
|
2
|
67%
|
MFI
|
6
|
29%
|
4
|
19%
|
6
|
29%
|
Development Bank
|
2
|
22%
|
0
|
0%
|
3
|
33%
|
Commercial Bank
|
6
|
13%
|
2
|
4%
|
17
|
35%
|
cooperative
|
4
|
9%
|
6
|
13%
|
10
|
21%
|
Credit union
|
0
|
0%
|
0
|
0%
|
0
|
0%
|
Insurance company
|
0
|
0%
|
0
|
0%
|
0
|
0%
|
Islamic Bank
|
0
|
0%
|
0
|
0%
|
3
|
60%
|
State development bank
|
0
|
0%
|
1
|
50%
|
0
|
0%
|
Total
|
22
|
15%
|
15
|
10%
|
43
|
30%
|
5.1.7. Digital Solutions
In terms of digital solutions, we found that 60 FIs out of 144
offer Online Banking (DS1), 19 FIs offer E-Products (DS2), and 18 FIs offer
Online Loan Applications (DS3). First place goes to the commercial banks with
37 DS1, 10 DS2, and 11 DS3. Cooperatives rank second, followed by MFIs and
Islamic banks. The other typical institutions do not have a strong focus on
digital solutions, whose offers are very limited.
Table 26: Percentage of digital solutions offered by type
of financial institution
Type
|
Sum of DS1
|
% DS1
|
Sum of DS2
|
%DS2
|
Sum of DS3
|
%DS3
|
Islamic Bank
|
4
|
80%
|
1
|
20%
|
2
|
40%
|
Commercial Bank
|
37
|
77%
|
10
|
21%
|
11
|
23%
|
NGO
|
1
|
50%
|
0
|
0%
|
0
|
0%
|
MFI
|
5
|
24%
|
4
|
19%
|
1
|
5%
|
cooperative
|
10
|
21%
|
4
|
9%
|
3
|
6%
|
Credit union
|
0
|
0%
|
0
|
0%
|
0
|
0%
|
Development Bank
|
0
|
0%
|
0
|
0%
|
1
|
11%
|
Insurance company
|
0
|
0%
|
0
|
0%
|
0
|
0%
|
International FI
|
0
|
0%
|
0
|
0%
|
0
|
0%
|
International Fund
|
0
|
0%
|
0
|
0%
|
0
|
0%
|
Agricultural Bank
|
0
|
0%
|
0
|
0%
|
0
|
0%
|
State development bank
|
0
|
0%
|
0
|
0%
|
0
|
0%
|
Total
|
60
|
42%
|
19
|
13%
|
18
|
13%
|
The table shows that more than two thirds of commercial banks
and Islamic banks offer online banking, that about 20% of MFIs, Islamic banks
and commercial banks offer e-products, and that 40% of Islamic banks and 23% of
commercial banks offer online loan applications.

50
5.2. Cluster Analysis
Our Data Analytics work often requires a large dataset (144
observations of 12 variables), which are nevertheless similar to one another.
Therefore, together with similar observations within a cluster, we may need to
organize them in a few clusters. These Clustering techniques are used in such
situations to identify segments within the data. The similarity and the
difference between data observations (e.g., geographical variables and
institutional types) can also be understood mathematically using distance
metrics, and different segmentation solutions can then be proposed. This
section will consider 2 types of clustering methods. We will first consider
k-means clustering, then Hierarchical Clustering.
5.2.1. Confirm Data:
Clustering can be done even when data are not metric; however,
many statistical methods currently used for clustering require that the data
themselves are metric: not only must the data be numbers themselves, but the
numbers also have a numerical significance, as explained in section 3.3.4. This
is required to compute distances between observations, and distances are
usually computed only using metric data. However, it is possible to define
distances also for non-metric data (e.g., continent, institutional type,
agricultural credit, etc.). In our case, the data are metric, so the next step
is to evaluate the descriptive statistics. Before moving forward, we check the
descriptive statistics of the data to understand it better.
Table 27: Descriptive statistics of the dataset
|
Mean
|
Median
|
Standard Deviation
|
Sample Variance
|
Range
|
Minimum
|
Maximum
|
C
|
0.96
|
1
|
1.04
|
1.08
|
3
|
0
|
3
|
T
|
3.44
|
2
|
3.16
|
9.95
|
11
|
0
|
11
|
F
|
1976.50
|
1984
|
30.36
|
921.78
|
180
|
1838
|
2018
|
B
|
215.08
|
30.5
|
661.57
|
437670.13
|
5653
|
0
|
5653
|
AL1
|
0.58
|
1
|
0.49
|
0.24
|
1
|
0
|
1
|
AL2
|
0.19
|
0
|
0.40
|
0.16
|
1
|
0
|
1
|
G1
|
0.15
|
0
|
0.36
|
0.13
|
1
|
0
|
1
|
G2
|
0.10
|
0
|
0.31
|
0.09
|
1
|
0
|
1
|
G3
|
0.30
|
0
|
0.46
|
0.21
|
1
|
0
|
1
|
DS1
|
0.42
|
0
|
0.49
|
0.24
|
1
|
0
|
1
|
DS2
|
0.13
|
0
|
0.34
|
0.12
|
1
|
0
|
1
|
DS3
|
0.13
|
0
|
0.33
|
0.11
|
1
|
0
|
1
|

51
5.2.2. Scale the data
The fact that some variables have very different ranges and
scales can often lead to problems: the results can be largely shaped by a few
large values. It is recommended to standardize the data, for instance by making
some raw attributes have, for example, a mean of zero and a standard deviation
of one, or to scale them between 0 and 1 to avoid such issues. After examining
the statistical description of the data, and before proceeding with the
clustering analysis, this step14 was carried out with the
function(r). This step of scaling is achieved by dividing the values in every
column by the corresponding 'scale' value from the argument if the value is
numeric. If the value is not numeric, then the values are divided by the
standard deviation or root-mean-square.
In our case, each variable has a mean of zero and a standard
deviation of one, as expected. A copy of the R script is attached in [Annex
D].
5.2.3. Select segmentation variables
The choices of which variables to use for clustering are
crucial decisions that will greatly influence the clustering solution. Every
variable chosen should have a significant impact on clustering. This
exploratory research provides an idea of which factors may differentiate
regions, type, etc. In our case, we can use the 7 categories of variables
(Continent, Institutional Type, Foundation, Number of branches, Agricultural
credit, Gender equality, and Digital Solutions) for segmentation, and the
remaining 3 (Total assets, Total equity, and Gross loan Portfolio) cannot be
considered as the data are incomplete.
5.2.4. Define similarity measure:
In clustering and segmentation, objects are related in some
way. Most statistical methods for clustering include a measure of distance. For
example, the Euclidean distance and the Manhattan distance are common measures.
We will use K-means here, which is based implicitly on pairwise Euclidean
distances between data points, and where the sum of squared deviations from
centroid is equal to the sum of pairwise Euclidean distances divided by the
number of points.
5.2.5. Number of clusters
Clustering and segmentation can be done statistically in a
number of ways, so it might make sense to try several approaches before finally
selecting one that is statistically robust, interpretable, and actionable -
among other aspects.
14 In the R script, this step is under the heading # scale
data

52
Throughout this study, we will utilize two widely used
methods: the K-means Clustering Method, and the Hierarchical Clustering Method.
Each method requires that we decide on how to measure distance/similarity
between our observations. However, one difference to highlight is that K-means
requires the user to specify the number of segments to create, whereas
Hierarchical Clustering does not.
First, let's look at the K-means method. WSSplot will be used
to determine the optimum number of clusters. Below is the result of this
function for the data scaled points.
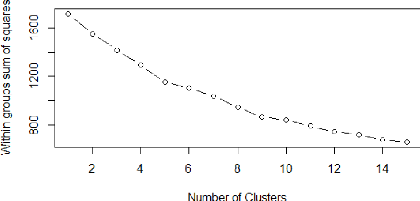
Figure 12: Determination of the optimum number of
clusters
5.2.6. K-means Clustering Method
According to one of the most common typical cluster methods,
the Euclidean distance of the observation can be used as a criterion for
determining the optimum number of clusters. This option specifies how the
distance between each observation point and the cluster center is calculated.
This case will utilize the default Euclidean distance for measuring the
distance between two points by using the length of the straight line between
them.
The 144 provided observation points will be clustered in 2, 4,
5 and 8 clusters respectively. The other algorithm parameters will remain the
same except for the number of clusters. By segmenting the data, it will be
possible to develop (potentially more refined) segment-specific insights.
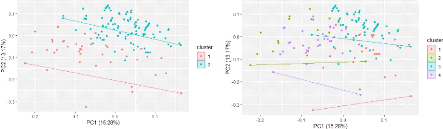

53
Data scaled Grouping in 2 Clusters Data scaled Grouping in 4
Clusters
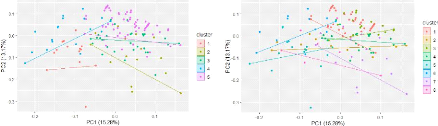
Data scaled Grouping in 5 Clusters Data scaled Grouping in 8
Clusters
Figure 13: Grouping Data scaled in different
clusters
To visualize the relationship between the category's variables
(C, T, F, B, AL1, AL2, G1, G2, G3, DS1, DS2, DS3), we demonstrate this with the
function fviz_cluster as shown in the Figure 14 below.
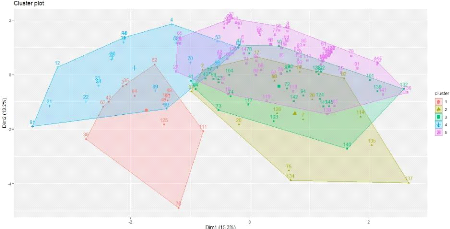
Figure 14: Clusters Visualization

54
Using the function $cluster we can determine to which
cluster belong each of these observations. Then, all data observations were
clustered in four membership groups
Table 28: Cluster membership IDs using K means method
Cluster no.
|
Observations Number
|
|
|
|
|
|
Total
|
1
|
3 18 35 36 42 48 49 50 62 63 76 84 111 125
|
|
|
|
|
14
|
2
|
2 7 17 20 26
|
37 67 75
|
82 88 114 120 134 135 137
|
|
|
|
|
15
|
3
|
10 41 43 47
|
57 59 64
|
72 73 74 78 90 92 94 101 102 103
|
104
|
117
|
124
|
126
|
29
|
|
127 132 133
|
139 140
|
142 143 145
|
|
|
|
|
|
4
|
4 12 21 22 23 30 32 34 39 40 52 53 54 56 60 69 70 80 81
|
|
|
|
|
19
|
5
|
1 5 6 8 9 11
|
13 14 15
|
16 19 24 25 27 28 29 31 33 38 44 45 46
|
51
|
55 58 61
|
67
|
|
65 66 68 71
|
77 79 83
|
85 86 87 89 91 93 95 96 97 98 99
|
100
|
105
|
106
|
107
|
|
|
108 109 110
|
112 113
|
115 116 118 119 121 122 123 128
|
129
|
130
|
131
|
136
|
|
|
138 141
|
|
|
|
|
|
|
|
A second way to identify if there is a relationship is to use
Hierarchical Clustering (next Section).
5.2.7. Hierarchical Clustering Method
Hierarchical clustering figure out how many segments our data
has. Additionally, this method gives a visual representation of how the data
might be clustered. It produces a plot called the Dendrogram often helpful for
visualization. For instance, in this case the dendrogram was based on the
Euclidean distance metric and R's ward.D hierarchical clustering
option is as follows:
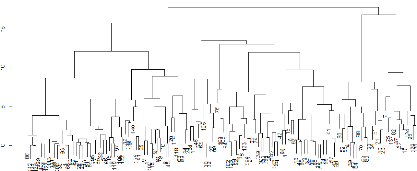
Figure 15: Hierarchical Clustering
As shown in the Dendrogram, clustering is accomplished by
aggregating observations by narrowing them down to pairwise observations
closest to each other and merging smaller

55
groups into larger groups based on which groups are closest in
proximity. Our data are merged eventually into one segment. According to the
heights of the tree branches, the clusters that have merged at that level of
the tree are very different one from the other. The longer lines indicate the
differences between the clusters below. This method combines the closest data
points/groups first, then the farthest, resulting in increased branches heights
when you traverse the tree from the ends to the roots.
Even with many observations, dendrograms can be useful for
segmentation because, in general, branches grow logarithmically with data
numbers. By analyzing the dendrograms, we can understand our data and the
segments that exist in it better in practice. This Hierarchical Clustering
found 4-segment solution (using Euclidean distance and hclust with
option ward.D). All data observations were clustered in four
membership groups.
Table 29: Cluster membership IDs using Hierarchical
method
|
Cluster
Membership 1
|
Cluster
Membership 2
|
|
Cluster
Membership 3
|
Cluster
Membership 4
|
Observation
|
7 75
|
137 82 37 67
|
60
|
52
|
56
|
4
|
39
|
40
|
84 111 48 36 63
|
86 112 121 128
|
Number
|
2 17
|
134 26 120
|
54
|
88
|
70
|
53
|
80
|
22
|
125 30 32 27 69
|
129 107 109 123
|
|
133
|
|
34
|
81
|
21
|
12
|
23
|
|
76 103 105 94
|
113 131 98 108
|
|
|
|
|
|
|
|
|
|
102 126 90 92 47
|
130 93 1 66 83 87
|
|
|
|
|
|
|
|
|
|
78 133 104 127
|
31 28 29 16 51 58
|
|
|
|
|
|
|
|
|
|
73 74 139 101
|
95 77 85 33 5 46
|
|
|
|
|
|
|
|
|
|
132 72 124 42
|
116 99 110 97
|
|
|
|
|
|
|
|
|
|
143 144 42 64
|
106 122 19 119
|
|
|
|
|
|
|
|
|
|
117 15 6 62 18 49
|
138 140 136 141
|
|
|
|
|
|
|
|
|
|
50 3 35 44 65 13
|
25 9 11 100 115
|
|
|
|
|
|
|
|
|
|
55 71 96 57 43 59
|
91 61 79 10 8 45
|
|
|
|
|
|
|
|
|
|
|
20 114 118 68 89
|
|
|
|
|
|
|
|
|
|
|
14 24
|
Total
|
12
|
|
17
|
|
|
|
|
|
53
|
59
|
5.2.8. Selected method and number of clusters
The numbers above represent the clusters that our observations
belong to when we use K-means method for 5 clusters and hierarchical method for
4 clusters, for the same total number of observations. Because we use different
methods, we do not need the observations to be in the same clusters, nor do the
segment profiles that we will find next (A comparison of IDs between both
methods was done using four clusters). However, one feature of statistically
robust Clustering is that the observations are segmented according to similar
characteristics regardless of the methodology used. In other words, the
segments' profiles should not vary too

56
much when we use different approaches or variations of the
data. Therefore, the K-means clustering method will be our main focus. Using
the WSSplot method (section 5.2.5), it is possible to determine the
point where the kink in the curve may occur so that an optimum number of
clusters can be determined. Another method15 is to run K-means
Clustering from 1 to 10 and use the function betweenss_totss to
compare and determine the best number of numbers.
By crossing the hierarchy downwards, we can see that in the
hierarchical method we will get different clusters, starting with 4 clusters at
the height of 15, 8 clusters at the height of 10, 33 clusters at the height of
5, and even more clusters as the height becomes lower (figure 15). We will
focus on K-means Clustering to avoid this unnecessarily large number of
clusters. The difference between these two methods is that with K-means you can
test, select, and verify the optimum number of clusters. However, if you choose
a minimum height, when using the hierarchical method, the number of clusters
may appear to be excessive.
Moreover, it is found that the 5 segments found are relatively
resistant to changes in data subsets and clustering methods. In general, the
observations are grouped in the same clusters with no major changes.
Segmentation is judged by the robustness of both the statistical
characteristics as well as many qualitative criteria: institutional type,
agricultural credit, gender, and digital solutions.
This segmentation was validated using silhouette coefficient
with the function silhouette to determine the robustness of our
clustering. In this case, the silhouette width is understood as follows:
· Si > 0 indicates that the observations are well
clustered.
· Si < 0 indicates that the observation was placed in
the wrong cluster.
· Si = 0 indicates that the observation is in between two
clusters
In this silhouette plot, there is no negative silhouette
width, and the maximum value is greater than 0.14, indicating that our
clustering using five groups is good. The method of mounting the clusters is
always more reliable if the ratio is so close to 0.5. If, however, this method
shows a significant result, the size of the clusters and the individuals differ
from those determined by the previous method.
15 In the R script, this step is under the heading # Choosing
K
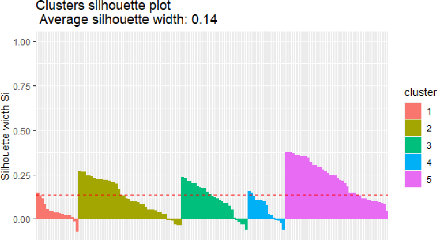
Figure 16: Validation of the number of clusters

57
5.2.9. Extracting Results
Having chosen five clusters, we want to learn more about who
the financial institutions in these clusters are and how the segments can be
interpreted. The data within each of the selected segments must be visualized
and understood for this purpose. As a result, we can extract the clusters from
the data and add them to our initial data to perform some descriptive analysis.
To analyze each cluster, we will name it according to the most significant
pattern, as shown below.
Cluster Number Average Foundation Cluster
Name
1 1948 Value chain oriented FIs
2 1967 Gender Staff FIs
3 1975 Farmer credit provider FIs
4 1990 Innovative digital newcomers
5 1982 Traditional Banking approaches
Table 30: Nomination of the FIs groups

58
# Value chain oriented FI:
This cluster has the highest average age in the sample and
most financial institutions are African, with 14% coming from each Asia and
South America. In addition, this sample consists of mainly commercial banks
with a 68% share, 7% are cooperatives, and the same share is held by
development banks. Located in the cluster are the oldest average foundation
years 1948 and the most average number of agencies, 517.
There is a high rate of agri-business and SME lending among
these financial institutions, which funds actors in the value chain with an 86%
percentage., therefore the value chain finance specialty in this segment.
Moreover, 57% of the institutions in the sample provide agricultural credit to
farmers, ranked 3rd among the clusters. In addition, 71% of these firms
emphasize gender programs, but only 7% of them provide credit facilities for
women, and like 3 other groups have do not invest in women's Equality staff.
Among these FIs, 71% offer online banking, and half offer online loan
applications.
# Gender Staff FI:
In terms of geographic location, this segment is very diverse,
with half of the FIs coming from Africa, 13% from Asia, 20 % IFI, and 20% from
South America. Concerning institutional type, cooperatives account for 40% of
the sample, followed by MFIs at 27%, commercial banks at 13%, and state
development banks, NGOs, and international financial institutions at 7% each.
In this cluster, the average foundation year is 1967, younger than the cluster
before, and the average number of branches is 433.
As indicated above, the Gender Staff FI group is made up of
FIs that 100% guarantee gender equality in the recruitment and selection of
employees. Moreover, 47% of FIs offer credit facilities to women, and 13% offer
gender-specific programmes such as workshops, events, and scholarships.
In relation to rural lending, 67% of FIs offer agricultural
loans to small farmers, while 20% lend to businesses. This segment is less
digitally oriented, as only 47% offer online banking and 20% offer E-products,
while 7% offer online loan applications.
# Farmer credit provider FIs:
The segment consists of 29 institutions, 28% from South
America, 24% are IFI, 24% from Asia, and 24% from Africa. Regarding
institutional types, cooperatives make up 31%, commercial banks 21%, MFIs 14%,
and Islamic banks and development banks each make up 10%. A few other typical
institutions include NGOs, international FIs, and international funds. There
are 215 branches in this group on average and the average foundation year is
1975.

59
As far as rural lending goes, this cluster is the most
extreme, where 72% FIs offer credits to small-scale farmers while 0% offer loan
to agribusinesses.
All financial institutions advocate gender equality and
empower women through a range of programs. However, fewer than 7% of FIs offer
credit to women, and there is no interest in offering employment opportunities
to female staff.
In terms of digital solutions, half of the FIs provide online
banking, however, only 7% offer online loan applications.
# Innovative digital newcomers
This cluster has 19 financial institutions, 79% of which are
from Africa and 21% from Asia. 68 percent are commercial banks, 16 percent are
cooperatives, 11 percent are microfinance institutions, and 5 percent are
Islamic banks. Compared to other groups, this group has a very small number of
branches, 95 on average. In this cluster, the average FI foundation year is
1990, making it the youngest.
There are the lowest bids for small farmers in this cluster,
with 37% offering credit to farmers, 26% lending to value chain players, and
gender equality isn't strongly addressed; 16% of small business loans go to
women, and 11% promote gender programmes.
Additionally, this group is characterized by digitalization as
84% of FIs offer online banking services, as well as other electronic products
like SMS Banking, Email Banking, and Mobile Loans. Moreover, 37% of the FIs in
this segment offer Online Loan Applications.
# Traditional Banking approaches
This last group, with the largest number of members, was made
up of 48% Africans, 34% South Americans, 19% Asians, and 4% global. The most
dominant institution type is cooperative, representing 42% of total
institutions, followed by commercial banks, 16% of microfinance institutions,
7% of development banks, 3% of agricultural banks, and 1% for credit unions,
insurance companies, international funds, Islamic banks, and nonprofits. FIs in
this traditional cluster have an average age of 39 and 137 branches on
average.
This segment is the lower regarding bank services, where we
found that half of this group offers agricultural credit for farmers, and 12%
offer agribusiness credit. Gender-specific projects are not well supported by
this group, only 13 provide women facility credit. Finally, the lower digital
solutions offer with only 16% FIs offer online banking, 0% E-products, and 1%
online loan application.
Below is a summary of each cluster's most important
characteristics.
Table 31: Cluster's characteristics

60
Cluster Name
|
Group 1 Value chain FIs
|
Group 2 Gender Staff FIs
|
Group 3 Farmer credit provider FIs
|
Group 4
Innovative digital newcomers
|
Group 5
Traditional Banking
approaches
|
Region
|
|
|
|
|
|
Africa
|
71%
|
47%
|
24%
|
79%
|
42%
|
Asia
|
14%
|
13%
|
24%
|
21%
|
19%
|
South America
|
14%
|
20%
|
28%
|
|
34%
|
Global
|
|
20%
|
24%
|
|
4%
|
Institutional Type
|
|
|
|
|
|
Agricultural Bank
|
|
|
|
|
3%
|
Commercial Bank
|
86%
|
13%
|
21%
|
68%
|
22%
|
cooperative
|
7%
|
40%
|
31%
|
16%
|
42%
|
Credit union
|
|
|
|
|
1%
|
Development Bank
|
7%
|
|
10%
|
|
7%
|
Insurance company
|
|
|
|
|
1%
|
International FI
|
|
7%
|
3%
|
|
|
International Fund
|
|
|
7%
|
|
1%
|
Islamic Bank
|
|
|
10%
|
5%
|
1%
|
MFI
|
|
27%
|
14%
|
11%
|
16%
|
NGO
|
|
7%
|
3%
|
|
1%
|
state development
bank
|
|
7%
|
|
|
1%
|

61
Average Foundation
|
1948
|
1967
|
1975
|
1990
|
1982
|
Average Branches
|
517
|
433
|
215
|
95
|
137
|
Agricultural Credit
|
|
|
|
|
|
Farmer credit
|
57%
|
67%
|
72%
|
37%
|
57%
|
agri-business credit
|
86%
|
20%
|
0%
|
26%
|
12%
|
Gender Programmes
|
|
|
|
|
|
Credits facility for
women
opportunities to female staff
|
7%
0%
|
47%
100%
|
7%
0%
|
16%
0%
|
13%
0%
|
Gender Programmes
|
71%
|
13%
|
100%
|
11%
|
0%
|
Digital Solutions
|
|
|
|
|
|
Online Banking
|
71%
|
47%
|
55%
|
84%
|
16%
|
E-Products
|
0%
|
20%
|
0%
|
84%
|
0%
|
Online Loan
|
50%
|
7%
|
7%
|
37%
|
1%
|
Applications
|
|
|
|
|
|
Total FIs
|
14
|
15
|
29
|
19
|
67
|
6. Discussion
6.1. An information provider database
The database provides a snapshot of financial institutions
engaged in agricultural sectors in the Global South, including African, Latin
American, Asian, and international financial institutions. The different kinds
of institutions such as commercial banks, cooperatives, development banks,
Islamic banks, cooperatives, and credit unions, as well as international funds
and institutions, each finance the agricultural sector differently. As a
result, the database can be used to determine the similarities and differences
among these institutions and understand how financial institutions, make
available offers in the agricultural sector to meet the needs of rural
clients.
There is a large amount of data that is available in the
database from websites of financial institutions, starting with the
geographical location and type of institution, which, in this case, do not
appear to be correlated with the offer of agricultural loans in this case.
Furthermore, the database listed the year the financial institute was founded
to highlight the years of experience in banking on the one hand, and to
determine whether this has any direct bearing on agriculture financing, but
this is not the case in the present sample. As well, the number of branches of
financial institutions and the relative importance of their dissemination in
providing small-scale farmers with information and access to agricultural
credit are not strongly linked in this database to agricultural credit offer.
However, based on the literature review, it was indicated that rural areas were
lacking an appropriate infrastructure, have no branches, or have a limited
network which makes the rural fundraising situation more difficult
(Langenbucher, 2005). Similarly, in other publications, low population
densities, fewer bank branches and distant locations were cited as factors that
reduce profitability of rural finance (IFC and World Bank, 2012).
Among the other important categories of information contained
in this database are the availability of agricultural credit for farmers as
input financing, labor payment, and harvest and storage, as well as credit for
agribusiness and agricultural SMEs. As cited in the literature review of (ISF
Advisors and Mastercard Foundation, 2019; UNCTAD, 2004), this category of
variables shows a financing gap for the agricultural sector, despite the use of
the approach VCF by many FIs. This sample contains only a little over half of
the institutions that provide agricultural credit, and even fewer when it comes
to financial institutions that provide financing to value chain participants,
not even one fifth.
Regarding gender equality, observations show that not many
gender programs are offered such as women's empowerment events, women's savings
accounts, scholarships, workshops,
62
but when it comes to staff equality and credit for women, the
available options are far from expectations.
In terms of digital solutions, the database indicates that
increasingly financial institutions rely on online banking; on the other hand,
e-banking products such as SMS, Email, and Mobile Loans are less and less
common, and are only used in countries where smart phones are not yet widely
available. As for online loan applications, FIs are experimenting with this new
method, but the products available are very limited. According to the
literature, FIs can enhance their ability to offer competitive products by
strengthening the relationship between producers and buyers, which will lead to
more innovative products (Kaufman, Wood, & Theyel, 2000).
Due to the inaccessibility of information on the part of
farmers, and the low awareness of farmers' products by institutions, it is
possible to argue that agricultural credit for small-scale farmers, as well as
for value chain actors, exists in a weak and even random manner. Further, some
financial institutions are reluctant to lend to the agricultural sector because
of the lending risk as mentioned in the literature review (Herliana, Acip,
Qorri, Qonita, & Nur, 2018).
6.2. Analysis of the clustering analysis
In fact, when assessing the factors that could facilitate the
development of agricultural loans, the cluster analysis has revealed that
regardless of geographic location, age of the institution, and number of
branches, financial institutions with value chain lending programs offer more
agricultural credit to farmers and support women with a range of programs. The
majority of these FIs offer Online Banking, in addition to online loan
application. In fact, this analysis has also demonstrated that when financial
institutions promote gender equity strongly or programs that support women
either directly or indirectly, they immediately offer moderate credit for
women, agricultural credit for small farmers, and several digital solutions. A
key finding of the study is that financial institutions offer customized and
enhanced rural financial services that are in high demand and in line with
gender issues and digital solutions. This was shown by the results of cluster
analyses for the first, second, and third segments with the names respectively
of Value Chain FIs, Gender Staff FIs, and Farmer Credit Provider FIs.
In contrast, when FIs provide low-level financial services and
tend to use traditional banking methods, they do not promote gender equality or
offer digital solutions, as shown in the cluster 5 (Traditional Banking
approaches). Nevertheless, technology-oriented solutions are at the center of
the most youthful cluster, with an average foundation year of 1990, hence the
name Innovative digital newcomers.
63
Previously, the analysis looked at the variables of
agricultural loans, women's equity, and digital solutions together. Keeping in
mind that years of banking experience and number of branches may not be
statistically significant. Findings indicate that commercial banks provide most
of the credit to value chain approaches, while cooperatives are the most
popular for agriculture loans, both with MFIs being gender equity-oriented
organizations. As explained in the previous section, the dominance of
commercial banks in the first segment Value chain oriented FIs and the fourth
group Innovative digital newcomers in providing financial services and digital
solutions may be explained by the fact that these types of institutions have
the resources necessary to provide these services. While there are more
individuals from these types of organizations may explain.
Based on table 19, commercial banks, cooperatives, and
microfinance institutions represent 81 percent of our simple. However, this
database shows all agricultural banks, international funds, and NGOs offering
loans to small farmers, although cooperatives and MFIs are most likely to use
it. International funds appear less frequently than agricultural banks.
Agribusiness loans are the exclusive domain of 50% of agricultural banks, 29%
of commercial banks, and 33% of development banks as well as 24% of
microfinance institutions according to the 144 FIs sample
Additionally, table 21 shows that several FIs such as
development bank are the least likely to provide agricultural loans. This could
be because these institutions may be included as facilitators or brokers for
several agricultural development projects. Furthermore, these institutions
often provide financial aid through other financial institutions, and that is
the major case for this sample of database according to the websites of FIs.
6.3. Limitations and further research needs
The evaluation of the database provides valuable information
about the potential for diversifying financial offerings and introducing
innovative products that cater to rural clients' needs.
First, this database was created based on an English
literature review, so many financial institutions from North Africa, South
America, and Asia were not considered.
Second, the structure of the database causes some information
to be lost due to specific categories of data being classified into.
Consequently, some of the information cannot be considered. This information
relates to farmer credit for instance, where several FIs divide this product
into several categories such as input loans, fertilizer loans, harvest credit,
etc....Nevertheless, other FIs include this type of credit under one category
of farmer loan. Furthermore, 4 FIs from the 144 in the sample work with service
providers or collect information
64
about farmers for credit risk screening purposes. These
factors are collected and used to determine whether a farmer is credit worthy
or not. However, this information is not considered since these FIs represent
only 3% of the sample. Therefore, a deeper analysis must be conducted of an
expanded and more diverse set of financial institutions taking into account
this important information.
Third, the data base also included several categories of
variables, such as Total assets, Total equity, Gross loan portfolio, and number
of clients. Since many websites did not have information on these variables, we
did not take them into account. Consequently, these cannot be considered and
high-level information, such as penetration rate, cannot be calculated at this
point.
Fourth, the lack of credible or official websites of many
financial institutions, which reduced the sample by 50 FIs. This is rather than
their significant role in funding VC initiatives as described in the
bibliographic study. In order to address these issues, an online survey should
be conducted with FIs representatives to gather the maximum amount of
information necessary for analysis. In this regard, an online questionnaire is
crucial to analyze the way in which FIs currently fund the agriculture sector,
including the approach AVCF. Having a clear understanding of how FIs apply the
AVCF is crucial on the one hand! On the other hand, it is essential to provide
an accurate depiction of the AVCF's features and ways it can improve access to
finance for all value chain players.
Also, the foundation believes more research needs to be done
on gender equality programs and their relationship to agricultural finance. A
study is needed to determine whether promoting women's empowerment and gender
equality can promote lending risk reduction and increase credit facilities in
the agricultural sector. Though cluster analysis did not show a significant
relationship between digital solutions and agricultural credit, evaluating how
digital tools can be used to increase farmers' awareness of credit offers by
financial institutions is a high priority.
A weakness of the analysis is the neglect of some variables
including geographical location (G), institutional type (T), and foundation
year (F), and the number of branches (B) due to the use of the binary method of
accreditation for the qualitative variables. Thus, after duplicating the
agricultural loan variables into two variables (AL1 and AL2), gender equality
into three variables (G1, G2, G3), and digital solutions into three variables
(DS1, DS2, DS3), we arrived at 12 variables in total. As a result, cluster
analyses were influenced by inequalities in importance, of which eight
variables were essentially only three (although they were duplicated) while the
remaining four, which are more numerous, were given less significance.
65
To rectify this, it is necessary to give coefficients for each
category of variables, according to their significance. The purpose of this is
to distinguish the order of relevance of the variables and help make the
analysis more logical.
66
7. Conclusion and Recommendations
7.1. Conclusion
Although FIs have introduced innovative services to help
improve rural access to services, it is yet to be determined if these can
contribute to increased access in the rural economy. Since the FIs' website
does not contain other important information, such as number of clients,
penetration rates, etc..., much attention has been given to agricultural loans,
gender equality, and digital solutions in this study.
First, this investigation was based on a database of 144 FIs
from the literature review, including 67 FIs from Africa, 29 from Asia, 35 from
South America, and 13 IFIs. An analysis of the financial institutions reveals
that, internationally, 58 percent offer credit to farmers, and 19 percent offer
credit to value chain actors. Moreover, there are no restrictions on the
availability of credit for small-scale farmers among the agricultural banks,
international funds, and NGOs in this database, although local cooperatives and
microfinance institutions are more likely to offer it. This confirm the
Hypothesis H1 that Various trends can be seen based on the variables
concerning the provision of credit by different types of FIs in the Global
South.
Second, to enhance rural finance, there is a crucial need to
ensure farmers have access to credit, promote the empowerment of women and
promote the use of digital technology. It has been found that while 58 percent
of FIs currently provide some type of agricultural credit, only 31 percent of
FIs have programs geared toward empowering women, and 42 put digital solutions
on the table. This statement answers the second research question and confirm
the hypothesis H2, where not most FI deal with gender issues and offer digital
solutions.
In these circumstances, the FIs are limited in their ability
to request rural financial services. In addition, the results of the study are
only able to provide some indication on the number, availability, and link
between agricultural loans, gender, and digital solutions, but not on their
impact, and therefore cannot accurately assess the potential of those services
in improving access to agriculture.
Third, when examining the relationship between agricultural
credit offers and the other variables, the differences and similarities between
financial institutions could be determined through the method cluster analysis,
which identified five clusters according to their main patterns. This cluster
analysis classified all the observations into five groups, starting first with
those who are value chain oriented. A second segment was corresponding with
personnel with higher gender equality. The third segment mostly serves small
farmers. Among the characteristics of the fourth cluster is its youthfulness
and digital orientation. The fifth group
67
provides the minimum services due to its Traditional Banking
approach. Analyzing the similarities and differences between these FIs provided
an answer to the third research question as well as confirmed the hypothesis
H3.
In the study, the most available information in a financial
institution's website is geographic location, institutional type, year of
foundation, number of branches, agricultural loans offered, gender equity and
digital solutions. However, cluster analysis reveals that this relationship
with digital solutions was not statistically significant, even when financial
institutions were high in digital solution rates. In addition, it was revealed
that there were no differences in agricultural finance offers depending on the
number of branches or years of banking experience.
A critical feature of this database is that it offers a new
outlook on rural finance services in the Global South. This is evidenced by
rural financial institutions offering enhanced services designed in line with
the needs of women. In addition to the finance gap in the agriculture sector,
the limited adoption of digital products by FIs highlights a digital gap.
Agricultural finance cannot be fully effective without more credit facilities
for women, and innovative digital solutions.
7.2. Recommendations
The analysis of the database contributes to broadening the
understanding of the potential of credit provision, gender, and digital
solutions in rural finance. In this sense, the assessment allows offering some
recommendations for further analysis:
As spoken by the professor Muhammed Yunus at the Australian
Business Chambers Forum in Melbourne: ««While we celebrated, we
noticed something. The money we gave to women was more effective. It did better
to the family.»»
In this regard, as part of the rural finance, farmer credit
should not be considered as the single solution to the challenges that
small-scale farmers face. In fact, gender could play a role in improving the
farmers' access to credit but need to be supported by other factors that are
determinant for the operation of these models such as digital tools. There is a
need for digital solutions to cater to the needs of small farmers. Some
e-products (Email Banking, SMS Banking) will enable farmers to manage and
consult their accounts, transfer money easily. In addition, online credit
applications can reduce transaction costs greatly. A database of information
about farmers should be collected by FIs to ease the lending process. Another
effective tool for improving rural finance is the AVCF, which reduces
transaction costs, manages risks, and offers better opportunities to value
chain actors.
68
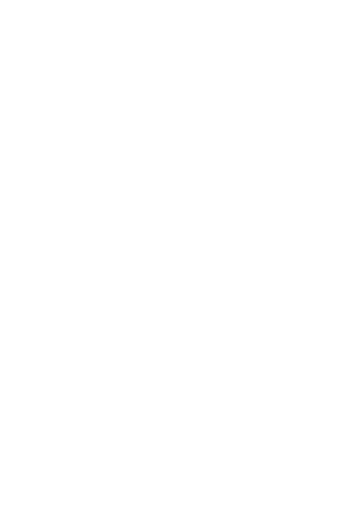
References
Abbas, A. C., Yuansheng, J., Feng, W. A., & Liu, D. (2017).
Famers' access to credit: Does collateral. (A. Goodness, Ed.) Cogent
Economics & Finance. doi:
https://doi.org/10.1080/23322039.2017.1369383
Abid, A., Jie, S., Aslam, W., Batool, S., & Lili, Y. (2020).
Application of structural equation modelling to develop a conceptual model for
smallholder's credit access. The mediation of agility and innovativeness in
organic food value chain finance. PLOS ONE, 15(8), e0235921. doi:
https://doi.org/10.1371/journal.pone.0235921
Adams, D. W. (1994). Using contracts to analyse informal finance.
(F. J. Bouman, & O. Hospes, Eds.) Financial landscapes reconstructed:
The fine art of mapping development, 179-192.
Adebayo, O. O., & G, A. R. (2008). Sources and Uses of
Agricultural Credit by Small Scale Farmers inSurulere Local Government Area of
Oyo State. Anthropologist, 10(4), 3134.
AFD, INSE & IDFC. (2021, 07 20). Key Indicators by
bank. Retrieved from Finance in Common:
https://afdshiny.shinyapps.io/developmentbanksdatabase/
AfDB. (2013). Agricultural Value Chain Financing (AVCF) and
Development for Enhanced
Export Competitiveness (Vols. (Vol. 136) [E-book]).
African Development Bank.
AFRACA and FAO. (2020). Agricultural value chain finance
innovations and lessons: Case studies in Africa. doi:10.4060/ca6345en
Akpan, S. B., Patrick, I. V., Udoka, S. J., Offiong, E. A., Okon,
U. E., & Comment, G. (2013). Determinants of Credit Access and Demand among
Poultry Farmers in Akwa Ibom State, Nigeria. American Journal of
Experimental Agriculture,, 3(2), 293 307. doi:10.9734/AJEA/2013/2810
Ali, J., & Kumar, S. (2011). Information and communication
technologies (ICTs) and farmers' decision-making across the agricultural supply
chain. 31(2), 149-59.
Aliero, H. M., & Ibrahim, S. (2011). An analysis of farmers
access to formal credit in the rural areas of Nigeria. African Journal of
Agricultur al Research, 6(17).
Anderson, J. (2017). National Survey and Segmentation of
Smallholder Households in Bangladesh: Household Level Data. Ref:
BGD_2016_SHS_v01_M. Washington, D.C: World Bank. Retrieved 8 1, 2021, from
https://microdata.worldbank.org/index.php/catalog/2839/study-description#metadata-identification
Antonaci, L., Demeke, M., & Vezzani, A. (2014). The
challenges of managing agricultural
price and production risks in sub-Saharan Africa. ESA Working
paper, 14-09. Retrieved from
http://www.fao.org/3/i3907ee/i3907ee.pdf
Anwarul, H., & Prerna, T. (2015). Credit Policy for
Agriculture in India - An Evaluation. (ICRIER, Ed.) Supporting Indian Farms
the Smart Way: Rationalising Subsidies and Investments for Faster, Inclusive
and Sustainable Growth.
Arias, J. (2019). Financing and financial inclusion for
agricultural and rural transformation. IICA.

70

Bibliography
Asian Development Bank. (2012). Support for agricultural value
chain development. (Manilla, Ed.)
Brown, M. Z. (2010). The emergence of information sharing in
credit markets. 19(2), 255-78.
Buckley, G. (1997). Microfinance in Africa: Is it either a
Problem or the Solution. World Development, 25(7), 1081-1093.
Cao, Z., & Lumineau, F. (2015). Revisiting the interplay
between contractual and relational governance: A qualitative and meta-analytic
investigation. 33, 15-42.
Carroll, T., Stern, A., Zook, D., Funes, R., Rastegar, A., &
Lien, Y. (2012). Catalyzing
smallholder agricultural finance. Washington, DC: Dalberg
Global Development Advisors.
CGAP. (2016). CGAP Smallholder Families Data Hub.
Retrieved from Consultative Group to Assist the Poor:
https://www.cgap.org/small_holders_data_portal/
Cheung, S. (1987). Economic organization and transaction
costs.
Coleman, B. E. (1999). The Impact of Group Lending in Northeast
Thailand. Journal of Development Economics, 60, 105-141.
Coon, J., Campion, A., & Wenner, M. (2010). Financing
agriculture value chains in Central America (IDB). Washington, DC:
Inter-American Development Bank.
Crook, J. N. (1996). Credit scoring: An overview. The
University of Edinburgh: Department of Business Studies.
Cuevas, C., & Pagura. (2016). AGRICULTURAL VALUE CHAIN
FINANCE A GUIDE FOR BANKERS. World Bank Group. Retrieved from
https://www.mfw4a.org/sites/default/files/resources/Bankers_Guide_to_AVCF.pdf
D'Espallier, B. (2012). Unsubsidized Microfinanoe Institutions.
Economics Letters, 120(2).
Da Silva, C. A., & Rankin, M. (2013). Contract farming
for inclusive market access. Rome: Food and Agriculture Organization of
the United Nations.
Dados, N., & Connell, R. (2012). The Global South.
American sociological Association, 11(1),
12-13. doi:10.1177/1536504212436479
Das, A., Senapati, M., & John, J. (2009). Impact of
agricultural credit on agriculture production: An empirical analysis in India.
Reserve Bank of India Occasional Papers, 2(30), 75-107.
Diagne, A., & Zeller, M. (2001). Access to Credit and
its Impacts in Malawi. Washington D.C: International Food Policy Research
Institute.
Dzadze, P., Osei, M. J., Aidoo, R., & Nurah, G. K. (2012).
Factors determining access to formal credit in Ghana: A case study of
smallholder farmers in the Abura Asebu Kwamankese district of central region of
Ghana. Journal of Development and Agricultural Economics, 14, 416
423.
Ellis, F. (1992). Ellis F. Agricultural policies in developing
countries. FAO and ALIDE. (1996). Collateral in rural loans. Rome.
Ferdinand, A., & Asmah, E. (2012, June). A Brief survey on
the literature on microfinance and Agriculture. Ghana Social Science
Journal, 9(1).

71

Bibliography
Fries, B., & Akin, B. (2004). Value chains and their
significance for addressing the rural
finance challenge. MicroREPORT, Accelerated Microenterprise
Advancement Project.
Gamage, P. (2013). Determinants of access to bank finance for
small and medium-sized enterprises: The case of Shri Lanka. Corporate
Ownership and Control, 10(3), 402- 409. doi:
https://doi.org/10.22495/cocv10i3c3art6
Gilbert, C. L. (2010). How to understand high food prices. J.
Agric. Econ, 61, 398-425.
Glassman, C. A., & Wilkins, M. H. (1997). Credit scoring:
probabilities and pitfalls. J. Retail Banking Service,.
Gonzalez, A., & Rosenberg, R. (2006). The State of
Microfinance - Outreach, Profitability, and Poverty. Paper Presented at the
Meeting Access to Finance: Building Inclusive Financial System.
Washington, D.C: The World Bank.
Gowhar, B. A., Ganie, A. H., & Padder, M. J. (2013). A study
on institutional credit to agriculture sector in India. (B. U. Department of
Economics, Ed.) International Journal of current research and academic
review, 1(4), 72-80. Retrieved from
www.ijcrar.com
Grandori, A. F. (2019). Contracting for the unknown and the logic
of innovation. 16(2), 413- 26.
Harhoff, D., & Korting, T. (1998). Lending relationships in
Germany- empirical evidence from survey data. J. Banking and Finance,
22, 1317-1353.
Herliana, S., Acip, S., Qorri, A., Qonita, H. A., & Nur, L.
(2018). The Constraints of Agricultural Credit and Government Policy Strategy.
MATEC Web of Conferences, 215. doi:10.1051/matecconf/201821502008
Herliana, S., Sutardi, A., Aina, Q., Himmatul, A. Q., &
Lawiyah, N. (2018). The Constraints of Agricultural Credit and Government
Policy Strategy. MATEC Web of Conferences, 215, 02008.
doi:10.1051/matecconf/201821502008
Honohan, & Beck. (2007). Making Finance Work for Africa,.
Washington, D.C, 2007.
Hult, G. H. (2004). Innovativeness: Its antecedents and
impact on business performance. (Vol. 5). Ind Mark Manag.
IFAD and CPI. (2020). IFAD, CPI, Chiriac, D., Naran, B., &
Falconer, A. (2020, November). Examining the Climate Finance Gap for
Small-Scale Agriculture. Climate Policy Initiative.
https://www.ifad.org/documents/38714170/42157470/climate-finance-gap_smallscale_agr.pdf/34b2e25b-.
Climate Policy Initiative. Retrieved from IFAD, CPI, Chiriac, D.,
Naran, B., & Falconer, A. (2020, November). Examining the Climate Finance
Gap for
https://www.ifad.org/documents/38714170/42157470/climate-finance-gap_smallscale_agr.pdf/34b2e25b-
IFC and World Bank. (2011). Scaling Up Access to Finance for
Agricultural SMEs Policy Review and Recommendations. 84(2011th ed).
Retrieved from
https://www.gpfi.org/sites/gpfi/files/documents/G20_Agrifinance_Report%20%28FINA
L%20ONLINE%29.pdf
IFC and World Bank. (2012). Innovative Agricultural SME Finance
Models. Global
Partnership for Financial Inclusion. (2012th ed., Vol. 116).
International Finance Corporation & World Bank.

72

Bibliography
Ijioma, J. C., & Osondu, C. K. (2015). Agricultural Credit
Sources and Determinants of Credit, Acquisition by Farmers in Idemili Local
Government. Journal of Agricultural Science and Technology. doi:doi:
10.17265/2161-6264/2015.01.004
Imtiaz SY, K. M. (2015). Telecom sector of Pakistan: Potential,
challenges and business opportunities. 32(2), 254-8.
ISF Advisors and Mastercard Foundation. (2019). Pathways to
Prosperity. Rural and Agricultural Finance State of the Sector Report,
61. Retrieved from
https://docplayer.net/209577056-Pathways-prosperity-rural-and-agricultural-finance-state-of-the-sector-report-november-2019.html
Jones, G. E. (2015). Reconciling information gaps in organic farm
borrowers' dealings with farm lenders. Agric Financ Rev, 75(4),
469-83.
Kaboski, J. P., & Townsend, R. M. (2012). The Impact of
Credit on Village Economies. American Economic Journal: Applied Economics,
4(2).
Kaufman, A., Wood, C., & Theyel, G. (2000). Collaboration and
technology linkages: a strategic supplier typology. 21(6), 649-63.
Khan, N., Shafi, M. M., & Shah, M. (2011). Review of past
literature on agriculture credit in rural area of Pakistan. Sarhad J.
Agriculture, 27(1), 103-110.
Kim, M., & Chai, S. (2017). The impact of supplier
innovativeness, information sharing and strategic sourcing on improving supply
chain agility. Global supply chain perspective, 187, 42-52.
KIT and IIRR. (2010). Value chain finance: Beyond microfinance
tor rural entrepreneurs. Royal Tropical Institute, Amsterdam; and International
Institute of Rural Reconstruction, Nairobi. Royal Tropical Institute,
Amsterdam; and International Institute of Rural Reconstruction,
Nairobi.
Klonner, S., & Rai, A. S. (2005). Adverse selection in credit
markets. Evidence from South Indian bidding. Retrieved from
http://eml.berkeley.edu/~webfac/emiguel/e271_s06/klonner.pdf
Langenbucher, A. (2005). Warehouse receipt financing and related
collateralized lending
mechanisms. Background briefing material prepared for the
Commodity Risk Management Group.
Limsombunchai, V., Gan, C., & Lee, M. (2005). An Analysis of
Credit Scoring for Agricultural Loans in Thailand. American Journal of
Applied Sciences, 2(8), 1198-1205.
Lu, F. (1999). Three Grain Surpluses-Evolution of China's Grain
Price and Marketing Policies (1978-1999). Symposium on China's Agricultural
Trade and Policy, 251-268.
MapChart. (2021, 08 08). The Advanced World map adds
political and geographical features to the basic World map. Retrieved from
World Map: Advanced:
https://mapchart.net/world-advanced.html
Meyer, R. (2011). Subsidies as an instrument in agriculture
finance: A review. The International Bank for Reconstruction and
Development. The World Bank.
Meyer, R. L. (2002). Track Record of Financial Institutions in
Assisting the Poor in Asia. Retrieved from
http://hdl.handle.net/11540/4154.
License: CC BY 3.0 IGO

73

Bibliography
Meyer, R. L. (2007). Analysing and financing value chains:
Cutting edge developments in value chain analysis. Paper presented at the
3rd African Microfinance Conference: New Options for Rural and Urban Africa.
Uganda.
Miguel, D., & Silvana, P. (2007, 08 30). Commercial Banks
and Microfinance. Munich Personal RePEc Archive. Retrieved from
https://mpra.ub.uni-muenchen.de/10229/
Miller, C. (2012). Agricultural value chain finance strategy and
design. Technical note. Agric value Chain Financ Strateg Des Tech
note.
Miller, C., & Jones, L. (2010). Agricultural value chain
finance Tools and Lessons (Vols. 195 [E-book]). (P. A. Publishing, Ed.)
United Kingdom: Food and Agriculture Organization
of the United Nations. Retrieved from
http://www.fao.org/3/i0846e/i0846e.pdf
Morduch, J. (1999). The Role of Subsidies in Microfinance:
Evidence from the Grameen Bank. Journal of Development Economics,
60(1).
Mosley, P., & Hulme, D. (1998). Micro-enterprise Finance: Is
there a Trade-off. Recent Research on Microfinance: Implications for
Policy.
Muiruri, E. (2007). Strategic partnership for fi nance.
presentation at the AFRACA Agribanks Forum.
Nyoro, J. (2007). Financing agriculture: Historical perspective.
presentation at the AFRACA Agribanks Forum.
Ololade, R. A., & Olagunju, F. I. (2013). Determinants of
Access to Credit among Rural Farmers in Oyo. Global Journal of Science
Frontier Research, 13(2), 8. Retrieved 07 21, 2021
Osuntogun, A. (1980). Some aspects of farm level credit use
in Nigeria. World Agricultural Economics and Rural Abstracts.
Pagano, M., & Jappelli, T. (1993). Information sharing in
credit markets. 48(5), 1693-718.
Pearce, D. (2003). Rural finance innovation case study. Buyer and
supplier credit to farmers: Do donors have a role to play? (BASIS-CRSP)
Conference on Paving the Way Forward for Rural Finance, (p. Paper
presented at Broadening Access and Strengthening Input market Systems).
Washington DC, USA.
Petersen, M. A., & Rajan, G. R. (1994). The benefit of
lending relationships: Evidence from small business data. J. Finance,
49, 3-38.
Piñeiro, V. (2019). Financing and financial inclusion for
agricultural and rural transformation. (MTID, Ed.) Research Gate, 3.
Retrieved from
https://www.researchgate.net/publication/338804997
Plata, V., & Nartea, G. N. (1998). Credit analysis procedures
of rural lenders in Canterbury. Paper. New Zealand: Agril. and
Resource Econ. Soc.
Pradhan, N. C. (2013). Persistence of Informal Credit in Rural
India: Evidence from All India Debt and Investment Survey and Beyond.
Working paper No. 05.
Robert, F., Chalmers, G., & Grover, D. (2012). Value Chain
Finance Guide: . Tools for Designing Project Interventions that Facilitate
Investment in Key Value Chain UpgradesACDI/VOCA,. Retrieved from
http://www.fao.org/sustainable-food-value-chains/library/details/en/c/267130/

74

Bibliography
Röttger, D. (2015). Agricultural finance for smallholder
farmers: Rethinking traditional microfinance risk and cost management
approaches.
Salami, A., & Arawomo, D. F. (2013). Empirical Analysis of
Agricultural Credit in Africa: Any Role for institutional Factors? Working
Paper no.192,.
Saqib, S. E., Kuwornu, K.M., J., Panezia, S., & Ubaid, A.
(2018). Factors determining subsistence farmers' access to agricultural credit
in flood-prone areas of Pakistan. Kasetsart Journal of Social Sciences,
39, 262-268.
Simon, A., & Yaya, L. (2012). Improving innovation and
customer satisfaction through systems integration.
Singh, R. P. (2001). Rural credit and participation of credit
agencies An analysis. Indian Cooperative Review, 38(4), 211-220.
Song, H. Y. (2018). Financial service providers and banks' role
in helping SMEs to access finance.
Sriram, M. S. (2007). Productivity of Rural Credit.
International Journal of Rural Management, 3(2), 245-268.
doi:10.1177/097300520800300204
Svensson, G. (2001). Extending trust and mutual trust in
business relationships towards a synchronised trust chain in marketing
channels. Manag Decis.
Swafford, P., Ghosh, S., & Murthy, N. (2006). A framework for
assessing value chain agility. Int J Oper Prod. Int J Oper Prod
Manag.
Thai-Ha, L. (2020). Microfinance and Social Development: A
Selective Literature Review. Fulbright University Vietnam, Fulbright
School of Public Policy and Management. Asian Development Bank (ADB).
Turvey, C., & Brown, R. (1999). Credit scores for a federal
lending institution: The case of Canada's farm credit corporation. Agril.
Finance(50), 47-57.
UNCTAD. (2004, Novermber 16-17). Financing commodity based trade
and development. Innovative agriculture financing mechanisms. Retrieved from
tinyurl.com/y8azepg
Vandeginste, B., Massart, D., Buydens, L., De Jong, S., Lewi, P.,
& Smeyers-Verbeke, J.
(1998). Cluster analysis. Data Handling in Science and
Technology, 57-68. doi:10.1016/s0922-3487(98)80040-3
Villalba, R., Venus, T., & Sauer, J. (2021). Agricultural
Value Chain Financing: building a framework for an Ecosystem Approach.
AARES Annual Conference. Australian Agricultural and Resource
economics society.
WBG & WFDFI. (2018). 2017 Survey of national development
banks. Malaysia: world bank group.
Wenner, M. (2006). Lecciones aprendidas en el fi nanciamiento de
las cadenas agrícolas de valor: El caso del BID. presentation at the
Latin American.
WOCCU. (2009). Technical Guide Integrated Financing for Value
Chains. Washington, DC: World Council of Credit Unions.
WOCCU. (2021). Direct Members. Retrieved from World
Council of Credit Unions:
https://www.woccu.org/our_network/membership

75

Bibliography
World Bank. (1975). Agriculture Credit Sector Policy Review. (W.
Bank, Ed.)
World Bank. (2007). Trade, Production, and Protection Database
1976-2004. The World Bank Economic Review.
World Bank and CGAP. (2017, December 13). Smallholder
Families Data Hub. (J. Anderson, Editor, & Consultative Group to
Assist the Poor) Retrieved July 31, 2021, from CGAP:
https://www.cgap.org/small_holders_data_portal/
Xie, H. L., He, Y. F., & Xie, X. (2017). Exploring the
factors influencing ecological land change for China's. J. Clean. Prod.,
142, 677-687.
Xie, H. L., He, Y. F., Zou, J. L., & Wu, Q. (2016).
Spatio-temporal difference analysis of cultivated land use intensity based on
emergy in the Poyang Lake Eco-economic Zone of China. J. Geogr. Sci.,
26, 1412-1430.
Yadav, P., & Sharma, A. (2015). Literature, Agriculture
Credit in Developing Economies: A Review of Relevant. International Journal
of Economics and Finance, 7(12), from 219. doi:10.5539/ijef.v7n12p219
Yuan, Y., Hu, Y., & Gao, P. (2011, July 24-26). Farmers'
choice and informal credit markets in China. Paper presented at the
Agricultural & Ap plied Economics Association s
2011 AAEA & NAREA Joint Annual Meeting,
Pittsburgh,Pennsylvania, July 24.
Yunus, M. (2007). Creating a world without poverty. Social
business and the future of capitalism. New York: Public Affairs.
Yunus, M., & Jolis, A. (1999). Banker to the Poor:
Micro-Lending and the Battle against World. Public Affairs.
Zander, R. (1994). Barriers of credit access in rural Sri Lanka.
(F. J. Bouman, & O. Hospes, Eds.) Financial landscapes reconstructed:
The Fine Art of Mapping Development, 139-208.
Zander, R. (2016). Risks and Opportunities of Non-Bank-Based
Financing for Agriculture: the Case of Agricultural Value Chain Financing.
Deutsches Institut für Entwicklungspolitik GmbH. Bonn: Deutsches
Institut für Entwicklungspolitik and German Development Institute.
Retrieved 07 18, 2021, from
https://www.die-gdi.de/uploads/media/DP_7.2016.pdf
Zhao, Q., Tsai, P.-H., & Wang, J.-L. (2019). Improving
financial service innovation strategies for enhancing china's banking industry
competitive advantage during the fintech revolution:. A Hybrid MCDM model,
11(5), 14-19.
Zhou, K., & Poppo, L. (2010). Exchange hazards, relational
reliability, and contracts in China: The contingent role of legal
enforceability. 41(5), 861-81.

76

Bibliography
Annex A
CEO E-mail-form
English
|
Dear Sir/Madam CEO
I am Mohamed Ali Trabelsi, a master student at the Technical
University of Munich (TUM School of Life Sciences). I am doing my master thesis
under
|
the supervision of Prof. Dr. Johannes Sauer, Roberto Villalba,
and Terese
|
Venus.
|
This thesis consists of an online survey with financial
institution on
agricultural finance and the implication of the "agriculture
value chain finance" approach.
I am looking forward for your support for the realization of the
survey, and I would be grateful if you can answer this online survey. You will
find the link here and note that all information remains confidential.
|
Thank you very much in advance. Sincerely,
Mohamed Ali Trabelsi
|
French
|
Cher Monsieur le directeur général, chère
Madame la directrice générale,
Je suis Mohamed Ali Trabelsi, étudiant en master a
l'université technique de Munich (TUM School of Life Sciences). Je suis
entrain de faire ma
|
mémoire de master sous la supervision de Monsieur Prof.
Dr. Johannes
|
Sauer, Monsieur Roberto Villalba, et madame Terese Venus.
|
Cette mémoire consiste en une enquête en ligne avec
les instituts financière sur le financement agricole et l'implication de
l'approche "agriculture value chain finance".
Je vous sollicite votre support pour la réalisation de
l'enquête et je vous demande votre accord pour répondre a cette
enquête en ligne.
Le lien vous trouvez ici, et notez que toutes les informations
restent confidentielles.
Je vous remercie infiniment d'avance. Cordialement,
Mohamed Ali Trabelsi
|

I

E-mail Form
Annex B: Database

II

Continent
|
Financial Institution
|
Country
|
Type
|
Foundation
|
Branches
|
Africa
|
Cameroon
Cooperative Credit Union League
|
Cameroon
|
cooperative
|
1968
|
12
|
Africa
|
Société Générale Côte
d'Ivoire
|
Côte d'ivoire
|
Commercial Bank
|
1962
|
28
|
Africa
|
Banque Nationale D'Investissement
|
Côte d'ivoire
|
Commercial Bank
|
1959
|
22
|
Africa
|
Ecobank
|
Côte d'ivoire
|
Commercial Bank
|
1989
|
47
|
Africa
|
Société Ivoirienne de Banque
|
Côte d'ivoire
|
Commercial Bank
|
1962
|
66
|
Africa
|
Nouvelle Société Interafricaine d'Assurance
|
Côte d'ivoire
|
Insurance company
|
1906
|
0
|
Africa
|
Advans Côte d'Ivoire
|
Côte d'ivoire
|
MFI
|
2005
|
9
|
Africa
|
MicroCred Côte d'Ivoire
|
Côte d'ivoire
|
MFI
|
2010
|
23
|
Africa
|
MUCREFAB - Microfinance
|
Côte d'ivoire
|
MFI
|
1994
|
9
|
Africa
|
Fonds International pour le Développement de la Retraite
Active
|
Côte d'ivoire
|
MFI
|
2011
|
8
|
Africa
|
Union Nationale des Coopératives d'Epargne et de
Crédit de Côte d'Ivoire
|
Côte d'ivoire
|
MFI
|
1976
|
4
|
Africa
|
Eswatini Association of Savings and Credit Co-operatives
|
Eswatini
|
cooperative
|
1964
|
40
|
Africa
|
Nib International Bank
|
Ethiopia
|
Commercial Bank
|
1999
|
189
|
Africa
|
Harbu Microfinance Institution Share Company
|
Ethiopia
|
MFI
|
2005
|
13
|
Africa
|
Commercial Bank of Ethiopia
|
Ethiopia
|
Islamic Bank
|
1942
|
1714
|
Africa
|
National Association of Cooperative Credit Unions of The
Gambia
|
Gambia
|
cooperative
|
1992
|
6
|
Africa
|
Ghana Co- operative Credit Union Association Ltd.
|
Ghana
|
cooperative
|
1955
|
11
|
Africa
|
Equity Bank
|
Kenya
|
Commercial Bank
|
1984
|
10
|
Africa
|
Agricultural Finance Corporation
|
Kenya
|
Agricultural Bank
|
1963
|
8
|
Africa
|
Faulu Kenya
|
Kenya
|
MFI
|
1991
|
67
|
Database
Africa
|
Family Bank
|
Kenya
|
Commercial Bank
|
1984
|
92
|
Africa
|
Cooperative Bank
|
Kenya
|
Commercial Bank
|
1931
|
100
|
Africa
|
Unity Finance
|
Kenya
|
cooperative
|
1974
|
15
|
Africa
|
Eclof Kneya
|
Kenya
|
MFI
|
1994
|
43
|
Africa
|
Juhudi Kilimo
|
Kenya
|
MFI
|
2004
|
39
|
Africa
|
Malawi Union of Savings and Credit Co-operatives, Ltd.
|
Malawi
|
cooperative
|
1962
|
47
|
Africa
|
Millennium bim - Particulares
|
Mozambique
|
Commercial Bank
|
1995
|
138
|
Africa
|
socremo banco de microfinanças
|
Mozambique
|
Commercial Bank
|
1998
|
13
|
Africa
|
African Banking Corporation
|
Mozambique
|
Commercial Bank
|
1999
|
10
|
Africa
|
Banco Mais
|
Mozambique
|
Commercial Bank
|
1999
|
7
|
Africa
|
Banco Único
|
Mozambique
|
Commercial Bank
|
2010
|
2
|
Africa
|
Barclays Bank
|
Mozambique
|
Commercial Bank
|
1991
|
3
|
Africa
|
Central Bank of West African States
|
Niger
|
International FI
|
1962
|
3
|
Africa
|
SunTrust Bank
|
Nigeria
|
Commercial Bank
|
2009
|
8
|
Africa
|
Keystonebank
|
Nigeria
|
Commercial Bank
|
1981
|
154
|
Africa
|
Union Bank of Nigeria
|
Nigeria
|
Commercial Bank
|
1917
|
320
|
Africa
|
UBA Group Corporate
|
Nigeria
|
Commercial Bank
|
1948
|
67
|
Africa
|
Zenith Bank
|
Nigeria
|
Commercial Bank
|
1990
|
390
|
Africa
|
Guaranty Trust Bank
|
Nigeria
|
Commercial Bank
|
1990
|
231
|
Africa
|
Standard Chartered Nigeria
|
Nigeria
|
Commercial Bank
|
1999
|
42
|
Africa
|
Polaris Bank Limited
|
Nigeria
|
Commercial Bank
|
2018
|
260
|
Africa
|
Heritage Bank Plc
|
Nigeria
|
Commercial Bank
|
1875
|
50
|
Africa
|
Bank of Kigali
|
Rwanda
|
Commercial Bank
|
1966
|
79
|
Africa
|
Banque Populaire Du Rwanda
|
Rwanda
|
Commercial Bank
|
1975
|
193
|
Africa
|
Association of Microfinance Institutions in Rwanda
|
Rwanda
|
MFI
|
2007
|
185
|
Africa
|
Seychelles Credit Union
Asia
|
Seychelles
|
Credit union
|
1970
|
3
|
Africa
|
Development Bank of Southern Africa
|
South Africa
|
Development Bank
|
1983
|
0
|
Africa
|
Land and Agricultural
|
South Africa
|
Development Bank
|
1912
|
9
|
|
|
|
|
|
Database
|
|
III
|
|
|
|
|
|
|
|
|
|
|
|
|
|
|
|
|
|
|
|
|
Development Bank of South Africa
|
|
|
|
|
Africa
|
Equity Bank South Sudan Limited
|
South Sudan
|
Commercial Bank
|
2009
|
5
|
Africa
|
National
Microfinance Bank
|
Tanzania
|
Commercial Bank
|
1997
|
226
|
Africa
|
FBME LIMITED
|
Tanzania
|
Commercial Bank
|
1990
|
4
|
Africa
|
Amana Bank
|
Tanzania
|
Islamic Bank
|
2011
|
10
|
Africa
|
Savings and Credit Cooperative Union League of Tanzania Ltd.
|
Tanzania
|
cooperative
|
1992
|
0
|
Africa
|
Azania Bank Ltd
|
Tanzania
|
Commercial Bank
|
1995
|
27
|
Africa
|
CRDB Bank
|
Tanzania
|
Commercial Bank
|
1996
|
260
|
Africa
|
Centenary Bank
|
Uganda
|
MFI
|
1985
|
63
|
Africa
|
Stanbic Bank
|
Uganda
|
Commercial Bank
|
1991
|
15
|
Africa
|
Mercantile Credit Bank
|
Uganda
|
Commercial Bank
|
1981
|
0
|
Africa
|
Uganda Cooperative Savings & Credit Union, Ltd.
|
Uganda
|
cooperative
|
1972
|
100
|
Africa
|
FINCA
|
Uganda
|
MFI
|
1992
|
29
|
Africa
|
UGAFODE Microfinance Limited (MDI)
|
Uganda
|
MFI
|
1994
|
19
|
Africa
|
First National Bank
|
Zambia
|
Commercial Bank
|
1838
|
22
|
Africa
|
ZANACO Bank
|
Zambia
|
Commercial Bank
|
1969
|
60
|
Africa
|
Stanbic Bank
|
Zambia
|
Commercial Bank
|
1906
|
63
|
Africa
|
Barclays Bank
|
Zambia
|
Commercial Bank
|
1991
|
47
|
Africa
|
National Association of Savings and Credit Unions
|
Zambia
|
cooperative
|
1969
|
0
|
Africa
|
National Association of Cooperative Savings & Credit Unions
of Zimbabwe
|
Zimbabwe
|
cooperative
|
1980
|
118
|
Asia
|
Islamic Investment and Finance Cooperatives Group
|
Afghanistan
|
cooperative
|
2009
|
18
|
Asia
|
BRAC Bank
|
Bangladesh
|
Commercial Bank
|
2001
|
9
|
Asia
|
Bangladesh Commerce Bank Ltd
|
Bangladesh
|
Commercial Bank
|
1998
|
48
|
|
|
|
|
|
Database
|
|
IV
|
|
|
|
|
|
|
|
|
|
|
|
|
|
|
|
|
|
|
|
Asia
|
Prime Bank Limited
|
Bangladesh
|
Commercial Bank
|
1995
|
146
|
Asia
|
ONE Bank Ltd.
|
Bangladesh
|
Islamic Bank
|
1999
|
103
|
Asia
|
Standard Bank Ltd
|
Bangladesh
|
Islamic Bank
|
1999
|
34
|
Asia
|
sonali bank
|
Bangladesh
|
Islamic Bank
|
2007
|
1228
|
Asia
|
Grameen Bank
|
Bangladesh
|
MFI
|
1983
|
2568
|
Asia
|
HDFC Bank
|
India
|
Commercial Bank
|
1994
|
5653
|
Asia
|
ational Bank for Agriculture and Rural Development
|
India
|
Development Bank
|
1982
|
32
|
Asia
|
National Dairy Development Board of India
|
India
|
cooperative
|
1965
|
2
|
Asia
|
Basix India
|
India
|
MFI
|
1996
|
50
|
Asia
|
KBS Bank
|
India
|
Commercial Bank
|
1996
|
29
|
Asia
|
YES Bank
|
India
|
Commercial Bank
|
2003
|
1000
|
Asia
|
Reserve Bank of India
|
India
|
State
development bank
|
1935
|
4
|
Asia
|
South Indian Federation of Fishermen Societies
|
India
|
cooperative
|
1980
|
0
|
Asia
|
Small Industries Development Bank of India
|
India
|
Commercial Bank
|
1990
|
33
|
Asia
|
National Agricultural Cooperative Federation
|
Korea
|
cooperative
|
1974
|
86
|
Asia
|
Mongolian
Confederation of Credit Unions
|
Mongolia
|
cooperative
|
2008
|
22
|
Asia
|
Nepal Federation of Savings & Credit Cooperative Unions.
Ltd.
|
Nepal
|
cooperative
|
1963
|
34
|
Asia
|
National
Cooperative Bank Ltd. (NCBL)
|
Nepal
|
cooperative
|
2003
|
373
|
Asia
|
Quedan and Rural Credit Guarantee Corporation
|
Philippines
|
cooperative
|
1978
|
14
|
Asia
|
National
Confederation of Cooperatives
|
Philippines
|
cooperative
|
1977
|
119
|
Asia
|
Development Bank of the Philippine
|
Philippines
|
state
development bank
|
1947
|
127
|
Asia
|
Philippine
Federation of Credit Cooperative
|
Philippines
|
cooperative
|
1960
|
0
|
Asia
|
Federation of Thrift & Credit
|
Sri Lanka
|
cooperative
|
1978
|
985
|
|
|
|
|
|
Database
|
|
V
|
|
|
|
|
|
|
|
|
|
|
|
|
|
|
|
|
|
|
|
|
Cooperative Societies Ltd.
|
|
|
|
|
Asia
|
bank for agriculture and agricultural cooperatives
|
Thailand
|
Development Bank
|
1966
|
1327
|
Asia
|
Small and Medium Enterprise
Development Bank
|
Thailand
|
Development Bank
|
2005
|
27
|
Asia
|
Cooperative Bank of Vietnam
|
Vietnam
|
cooperative
|
2013
|
100
|
America
|
Barbados Co- operative & Credit Union League Ltd.
|
Barbados
|
cooperative
|
1957
|
29
|
America
|
Belize Credit Union League Ltd.
|
Belize
|
cooperative
|
1956
|
7
|
America
|
Bermuda Credit Union Co-op Society
|
Bermuda
|
cooperative
|
1972
|
0
|
America
|
Sartawi Foundation
|
Bolivia
|
MFI
|
1989
|
40
|
America
|
PROFIN Foundation
|
Bolivia
|
MFI
|
2001
|
0
|
America
|
Pro-rural
|
Bolivia
|
cooperative
|
2001
|
0
|
America
|
Sicredi
Participações
|
Brazil
|
cooperative
|
2000
|
2000
|
America
|
Cayman Islands Civil Service Association Cooperative Credit Union
Ltd.
|
Cayman Islands
|
cooperative
|
1975
|
46
|
America
|
Federación Nacional de Cooperativas de Ahorro y
Crédito Financieras
|
Colombia
|
cooperative
|
2012
|
3205
|
America
|
Banco BAC San José S.A.
|
Costa Rica
|
Commercial Bank
|
1952
|
11
|
America
|
Federación de Cooperativas de Ahorro y Credito de Costa
Rica R.L.
|
Costa Rica
|
cooperative
|
1999
|
14
|
America
|
The Curacao Federation of Cooperatives
|
Curacao
|
cooperative
|
1961
|
6
|
America
|
Asociación de Instituciones Rurales de Ahorro y
Crédito, Inc.
|
Dominican Republic
|
cooperative
|
1991
|
24
|
America
|
Federación de Asociaciones Cooperativas
|
El Salvador
|
cooperative
|
1966
|
130
|
America
|
Cooperativas Micoope
|
Guatemala
|
cooperative
|
1963
|
300
|
America
|
Guyana Co- operative Credit Union League
|
Guyana
|
cooperative
|
2009
|
29
|
America
|
National Bank for Agricultural Development
|
Honduras
|
Agricultural Bank
|
1950
|
10
|
|
|
|
|
|
Database
|
|
VI
|
|
|
|
|
|
|
|
|
|
|
|
|
|
|
|
|
|
|
|
America
|
fundacioncovelo
|
Honduras
|
MFI
|
1985
|
0
|
America
|
VisionFund
|
Honduras
|
MFI
|
1995
|
17
|
America
|
Jamaica Co- operative Credit Union League Ltd
|
Jamaica
|
cooperative
|
1942
|
102
|
America
|
Banco Mercantil del Norte
|
Mexico
|
Commercial Bank
|
1899
|
20
|
America
|
Trust Funds for Rural Development
|
Mexico
|
Development Bank
|
1954
|
131
|
America
|
Nacional Financiera
|
Mexico
|
Development Bank
|
1934
|
3
|
America
|
Caja Popular Mexicana
|
Mexico
|
cooperative
|
1951
|
20
|
America
|
St. Patrick's Co- operative Credit Union
|
Montserrat
|
cooperative
|
2009
|
0
|
America
|
Banco Lafise
|
Nicaragua
|
Commercial Bank
|
1985
|
47
|
America
|
Credinka
|
Peru
|
cooperative
|
1994
|
90
|
America
|
Financiera Confianza
|
Peru
|
MFI
|
1991
|
115
|
America
|
Banco de Credito del Peru
|
Peru
|
Commercial Bank
|
1889
|
370
|
America
|
Fovida
|
Peru
|
cooperative
|
1987
|
3
|
America
|
Banco Bilbao Vizcaya
Argentaria/Peru
|
Peru
|
Commercial Bank
|
1951
|
300
|
America
|
St. Kitts & Nevis National Co- operative League Ltd.
|
Saint Kitts & Nevis
|
cooperative
|
2009
|
4
|
America
|
St. Lucia Co- operative League Ltd.
|
Saint Lucia
|
cooperative
|
1977
|
15
|
America
|
St. Vincent and the Grenadines Co- operative League, Ltd.
|
Saint Vincent & the
Grenadines
|
cooperative
|
1962
|
4
|
America
|
Co-operative Credit Union League of Trinidad & Tobago
|
Trinidad & Tobago
|
cooperative
|
1947
|
80
|
Global
|
Root Capital
|
International
|
NGO
|
1999
|
4
|
Global
|
Inter-American Development Bank
|
International
|
Development Bank
|
1959
|
28
|
Global
|
Rabobank
|
International
|
cooperative
|
1895
|
428
|
Global
|
International Finance Corporation
|
International
|
International FI
|
1956
|
184
|
Global
|
Incofin Investment Management
|
International
|
International Fund
|
2001
|
5
|
Global
|
Grameen Foundation and Fair Trade international
|
International
|
NGO
|
1997
|
2568
|
Global
|
Shared Interest
|
International
|
cooperative
|
1990
|
51
|
|
|
|
|
|
Database
|
VII
|
|
|
|
|
|
|
|
|
|
|
|
|
|
|
|
|
|
|
|
Global
|
Financial services and enterprise development
|
International
|
International Fund
|
1968
|
13
|
Global
|
European Microfinance Platform
|
International
|
MFI
|
1900
|
130
|
Global
|
Triodos Bank
|
International
|
Development Bank
|
1980
|
109
|
Global
|
International Raiffeisen Union
|
International
|
cooperative
|
1968
|
52
|
Global
|
International Fund for Agricultural Development
|
International
|
International FI
|
1977
|
177
|
Global
|
Inter-American Foundation
|
International
|
International Fund
|
1969
|
22
|
|
|
|
|
|
Database
|
VIII
|
|
|
|
|
|
|
|
|
|
|
|
|
|
|
|
|
|
|
|
Annex C: Survey for FIs Officials
Dear colleague,
We at the Technical University of Munich (TUM), Germany
are conducting a survey about financial institutions. We would like to
understand how your institution finances the agriculture sector, specifically
financial products, the credit screening and scoring mechanisms employed, and
the instrument used to mitigate risk and reduce transaction costs.
ü Why have I been chosen? Following a bibliographic
search, we have identified your institute as meeting certain criteria eligible
for this study
ü What will I be asked? general questions about your
institute, the economic situation, and the method of financing the agricultural
sector.
ü How my data will be stored? All the information we
obtain will remain strictly confidential and anonymous.
Researcher's details: Mohamed Ali Trabelsi,
Email: dali.trabelsi@tum.de
More Information: Supervisors: Roberto Villalba and
Terese Venus.
If you would like further details, please check the university
[TUM School of Life Sciences] website or this YouTube Link.
By consenting to take part, you agree to the following.
I have read the information sheet.
I have had the opportunity to ask question I understand my data
will be anonymous
Q0. The survey will take about 20-30 minutes. Do you consent
to take part? Choose an item. FORCE RESPONSE
ASK ONLY IF YES IS SELECTED IN Q0
1.0 General Information
Q1.1 How would you best describe your institution? Choose an
item.
Q1.2 Please select the Continent: Choose an item.
Q1.3 Please select the Country Choose an item.
Q1.4 When was your institutions established Choose an item.
Q1.5 Could you select your position please? Choose an item.
Q1.6 How many years have you been working in agricultural
finance? Choose an item.
Q1.7 How many years has your institution been working in
agricultural finance? Choose an item.
Survey with Financial Institutions in the Global South -
Multiple-respondent questionnaire
|
IX
|
|
|
2.0 Economic information
Q2.1 What where the total assets of your institutions? Click or
tap here to enter text. Q2.2 What was your institution's total equity? Click or
tap here to enter text.
Q2.3. What was the total gross loan portfolio of your
institution? Click or tap here to enter
text.
Q2.4 How many branches did your institution have? Click or tap
here to enter text. Q2.5 How many employees did your institution have? Click or
tap here to enter text. Q2.6 Which one of the following is the priority sector
Choose an item.
3.0 Agricultural credit: screening, scoring and
monitoring
Q3.1 Do you offer credit to all your customer? Choose an
item. FORCE RESPONSE ASK ONLY IF YES IS SELECTED IN Q3.1
*Product and Financial flows*
Q3.2 What lending products does your institution offer?Check all
that apply.
ALLOW MULTIPLE ANSWERS
3.2.1. Agricultural loan
|
?
|
3.2.2. Animal Husbandry loan
|
?
|
3.2.3. Consumption loan
|
?
|
3.2.4. Production loan (input)
|
?
|
3.2.5. Income generating activities
|
?
|
3.2.6. Working capital loans
|
?
|
3.2.7. Asset building
|
?
|
3.2.8. Lease financing
|
?
|
3.2.9 Micro-leasing (equipment)
|
?
|
3.2.10. Emergencies
|
?
|
3.2.11. Commercialization credit
|
?
|
3.2.12. Factoring (delay payment)
|
?
|
3.2.13. Others
|
?
|
Q3.3 What is the average loan per Borrower or range does your
institution offer to client? Choose an item.
Q3.4 What time frame for loans does your institution provide?
ALLOW MULTIPLE ANSWERS
3.4.1. Short-term Loan
|
?
|
3.4.2. Medium-term Loan
|
?
|
3.4.3. Long-term Loan
|
?
|
X
Survey with Financial Institutions in the Global South -
Multiple-respondent questionnaire
Q3.5 How is the loan disbursed? Choose an item.
Q3.6 How must the loan be repaid? Choose an item.
Q3.7 What is the interest rate per month?Choose an item.
Q3.8 What is the interest rate per year?Choose an item.
Q3.9 What is the percentage of other additional if incurred
Choose an item.
*Risk management*
Q3.10 How is the loan securitized? ALLOW MULTIPLE
ANSWERS
|
3.10.1 Securitization based on sales contract
|
?
|
3.10.2 Loan is percentage of value of produce
|
?
|
3.10.3 Collateral provided
|
?
|
3.10.4 Transaction carried out through bank account
|
?
|
3.10.5 Warehouse inventory
|
?
|
3.10.6 Deposit
|
?
|
3.10.7 Producer organizations members (group account)
|
?
|
3.10.8 Assessment and investigation
|
?
|
3.10.9 Legal document
|
?
|
3.10.10 Household Item/ Leased Item
|
?
|
3.10.11 Peer pressure from group, pledged to group
|
?
|
3.10.12 Borrower must own the land
|
?
|
Q3.11 Who has to repay the loan after lending?
3.11.1. Individual Farmer/Borrower
|
?
|
3.11.2. Cooperative
|
?
|
3.11.3. Farmer group
|
?
|
3.11.4. neighborhood groups
|
?
|
3.11.5. Association
|
?
|
3.11.6. Marketing organization
|
?
|
*Information flows*
Q3.12 Which of the following information does the institution
require before the loan is disbursed? ALLOW MULTIPLE ANSWERS
|
3.12.1 recent audited financial statement
|
?
|
3.12.2. recent interim financial statement
|
?
|
3.12.3. Cash flow prognosis for new season
|
?
|
3.12.4. Projected profit
|
?
|
3.12.5. Review of previous season (yield, contract, etc.)
|
?
|
3.12.6. development of market prices
|
?
|
3.12.7. realized investment
|
?
|
3.12.8. identity card
|
?
|
3.12.9. status in registry financial systems
|
?
|
3.12.10. Registration of membership
|
?
|
Survey with Financial Institutions in the Global South -
Multiple-respondent questionnaire
|
XI
|
|
|
3.12.11. Basic plan on how the loan will be used
|
?
|
3.12.12. Credit history
|
?
|
3.12.13. Potential buyers
|
?
|
3.12.14. Group registration certificate
|
?
|
3.12.15. Application letter
|
?
|
3.12.16. current year management account
|
?
|
3.12.17. Account number
|
?
|
3.12.18. Reputation
|
?
|
3.12.19. Characteristics of the family
|
?
|
3.12.20. Number of Ha owned
|
?
|
3.12.21. Area planted
|
?
|
Q3.13 What additional information do you require during the loan
period? ALLOW MULTIPLE ANSWERS
3.13.1. Monthly savings of group
|
?
|
3.13.2. Report of monthly meetings
|
?
|
3.13.3. A field credit officer
|
?
|
3.13.4. Check crop progress
|
?
|
3.13.5. Price of agricultural inputs
|
?
|
3.13.6. Farm inspection by specialist
|
?
|
3.13.7. Monthly project monitoring report
|
?
|
Q3.14 How long take on average between the application and the
disbursement of the loan? Choose an item.
*Borrower characteristics*
Q3.15 Which of the following affect the lending decision?
ALLOW MULTIPLE ANSWERS
|
3.15.1. Assets
|
?
|
3.15.2. Age
|
?
|
3.15.3. Education
|
?
|
3.15.4. Marital status
|
?
|
3.15.5. Number of children
|
?
|
3.15.6. Employment
|
?
|
3.15.7. Farm type
|
?
|
3.15.8. Landholding size
|
?
|
3.15.9. Borrower's Liquidity
|
?
|
3.15.10. Repayment capacity
|
?
|
3.15.11. Relationship indicators
|
?
|
3.15.12. Collateral requirements
|
?
|
3.15.13. Province
|
?
|
3.15.14. Other features
|
?
|
Q3.16 What is the most suitable age for getting the credit?
ASK ONLY IF AGE IS SELECTED IN 3.15
3.16.1. < 25-year-old ?
3.16.2. 26 - 35-year-old ?
Survey with Financial Institutions in the Global South -
Multiple-respondent questionnaire
|
XII
|
|
|
3.16.3. 36 - 45-year-old ?
3.16.4. >46-years-old ?
03.17 Which of the following education level is most reliable for
credit allocation? ASK ONLY IF EDUCATION IS SELECTED IN 3.15
3.17.1. Illiterate
|
?
|
3.17.2. Literate
|
?
|
3.17.3. Primary
|
?
|
3.17.4. Middle Class
|
?
|
3.17.5. High School
|
?
|
3.17.6. Intermediate
|
?
|
3.17.7. Graduation
|
?
|
3.17.8. Post-Graduation and Above
|
?
|
3.17.9. Any professional course & Technical
|
?
|
03.18 What farmer's Marital Status do the financial Institution
consider as an advantage before lending? ASK ONLY IF MARITAL STATUS IS
SELECTED IN 3.15
03.18.1. Married
|
?
|
03.18.2. Unmarried
|
?
|
03.18.3. Widow
|
?
|
03.18.4. Divorced
|
?
|
03.18.5. Separated
|
?
|
03.19. What number of children is an advantage for an
agricultural credit? ASK ONLY IF NUMBER OF CHILDREN IS SELECTED IN
3.15
3.19.1. None
|
?
|
3.19.2. 1-2 Children
|
?
|
3.19.3. 3-5 Children
|
?
|
3.19.4. > 6 children
|
?
|
03.20 Does your institute consider farmer Employment, if yes,
which of the following is a key factor? ASK ONLY IF EMPLOYMENT IS SELECTED
IN 3.15
3.20.1. Employed
|
?
|
3.20.2. Unemployed
|
?
|
3.20.3. self Employed
|
?
|
3.20.4. Professional
|
?
|
03.21 What types of agriculture activity (Farm type) eligible for
a credit? ASK ONLY IF FARM TYPE IS SELECTED IN 3.15
3.21.1. Arable Farming
|
?
|
3.21.2. Pastoral Farming
|
?
|
3.21.3. Mixed Farming
|
?
|
3.21.4. Subsistence Farming
|
?
|
3.21.5. Commercial Farming
|
?
|
XIII
Survey with Financial Institutions in the Global South -
Multiple-respondent questionnaire
3.21.6. Extensive and Intensive Farming ?
3.21.7. Nomadic Farming ?
3.21.8. Sedentary Farming ?
3.21.9. Poultry Farming ?
3.21.10. Fish Farming ?
Q3.22 How many Ha of agricultural land (Landholding Size) do
farmers at least operate to be qualified for a loan? ASK ONLY IF
LANDHOLDING SIZE IS SELECTED IN 3.15
3.22.1. Landless ?
3.22.2. < 1Ha ?
3.22.3. 1-5 Ha ?
3.22.4. 6-10 Ha ?
3.22.5. 11 Ha ?
Q3.23 What types of guarantees are accepted by your institute?
ASK ONLY IF COLLATERAL IS SELECTED IN 3.15
3.23.1. Livestock ?
3.23.2. equipment ?
3.23.3. guarantor ?
3.23.4. Land ?
3.23.5. Others ?
Q3.24 Which of the relationship indicators does your institute
take into consideration? ASK ONLY IF NUMBER OF RELATIOINSHIP INDICATORS IS
SELECTED IN 3.15
3.24.1. Borrowing from others ?
3.24.2. Duration for repayment ?
3.24.3. Others ?
4.0 Agricultural finance within value chains
Q4.1 Do your institution use any of these practices?Choose an
item.
Q4.2 Are you familiar with this approach of AVCF or have you ever
heard about it? Choose an item. FORCE RESPONSE
ASK ONLY IF YES IS SELECTED IN Q4.3
5.0 Financial product & Instrument
employed
Q5.1 Out of the following five financial products, which type of
financial product are you familiar with/do your institution apply in
agriculture lending? ALLOW MULTIPLE ANSWERS
5.1.1 Product financing ?
5.1.2 Receivables financing ?
5.1.3 Physical asset collateralization ?
5.1.4 Risk mitigation products ?
Survey with Financial Institutions in the Global South -
Multiple-respondent questionnaire
|
XIV
|
|
|
5.1.5 Financial enhancements ?
ASK ONLY IF PRODUCT FINANCING IS SELECTED IN Q5.1
05.2 Which of the following instruments has <INSERT 01.1
INSTITUTION> ever used?
5.2.1 Trader credit
|
?
|
5.2.2 Input supplier credit
|
?
|
5.2.3 Marketing Credit Company
|
?
|
5.2.4 Lead firm financing
|
?
|
ASK ONLY IF RECCEIVABLES FINANCING IS SELECTED IN
Q5.1
05.3 Which of the following instruments has <INSERT 01.1
INSTITUTION> ever used?
5.3.1 Trade receivables finance
|
?
|
5.3.2 Factoring
|
?
|
5.3.3 Forfaiting
|
?
|
ASK ONLY IF PHYSICAL ASSET COLLATERALIZATION IS SELECTED IN
Q5.1
05.4 Which of the following instruments has <INSERT 01.1
INSTITUTION> ever used?
5.4.1 Warehouse receipts
|
?
|
5.4.2 Repurchase agreements
|
?
|
5.4.3 financial leasing
|
?
|
ASK ONLY IF RISK MITIGATION PRODUCTS IS SELECTED IN
Q5.1
05.5 Which of the following instruments has <INSERT 01.1
INSTITUTION> ever used?
5.5.1 Insurance
|
?
|
5.5.2 Forward contracts
|
?
|
5.5.3 Futures
|
?
|
ASK ONLY IF FINANCIAL ENHANCEMENTS IS SELECTED IN
Q5.1
05.6 Which of the following instruments has <INSERT 01.1
INSTITUTION> ever used?
5.6.1 Securitization instruments
|
?
|
5.6.2 Loan guarantees
|
?
|
5.6.3 Joint venture finance
|
?
|
047. Ask question/Feedback Click or tap here to enter text.
We thank you Sir/Madam <INSERT 01.6 Name> for your time
spent taking the survey Your response has been recorded
*****
XV
Survey with Financial Institutions in the Global South -
Multiple-respondent questionnaire
Annex D: R Script
# Descriptive Analysis #
data1 <- na.omit(X20210922_database_FIs_MAT)
data<- data1[-1]
plot(data)
data
summary(data)
#Scale Data #
Data_scaled = apply(data, 2, function(r) {
if (sd(r) != 0)
res = (r - mean(r))/sd(r) else res = 0 * r
res
})
summary(Data_scaled)
install packages#
install.packages("dplyr")
install.packages("ggplot2")
install.packages("ggfortify")
install.packages("factoextra")
install.packages("clusterSim")
# load required libraries #
library(stats)
library(dplyr)
library(ggplot2)
library(ggfortify)
library(factoextra)
# WSS plot function #
wssplot <- function(Data_scaled, nc=15, seed=1234){
wss <- (nrow(Data_scaled)-1)*sum(apply(Data_scaled,2,var))
for (i in 2:nc){
set.seed(seed)
wss[i] <- sum(kmeans(Data_scaled, centers=i)$withinss)}
plot(1:nc, wss, type="b", xlab="Number of Clusters",
ylab="Within groups sum of squares")}
# Hierarchical Clustering ....
d <- dist(Data_scaled)
fith <- hclust(d, "ward.D2")
plot(fith)
# WSS Plot to choose maximum number of clusters #
wssplot(Data_scaled)
# Choosing K
k<- list()
for (i in 1:10){
k[[i]]<- kmeans(na.omit(data), i)
}
k[[9]]
k
betweenss_totss <- list()
for (i in 1:10) {
betweenss_totss[[i]]<-k[[i]]$betweenss/k[[i]]$totss
}
plot (1:10, betweenss_totss, type = "b" ,
ylab = "between SS / Total SS", xlab = "clusters(k)")
for (i in 1:4){
plot(data, k[[i]]$cluster)
# K-Means cluster #
KM= kmeans(Data_scaled,5)#
# Evaluating Cluster Analysis#
# Cluster plot 5#
autoplot(KM,Data_scaled,frame=TRUE)
# Segmentation & Visualization #
KM.clusters <- KM$cluster
rownames(Data_scaled) <- data1$id
fviz_cluster(list(data=Data_scaled,cluster=KM.clusters))
# cluster Membership #
KM$cluster
table(KM.clusters, data1$id)
table(data1$id, KM.clusters)
# Clusters Center #
KM$centers
# descriptive statistics at the cluster level
library(clusterSim)
desc <- cluster.Description(Data_scaled,KM$cluster)
print(desc)
summary(desc)
library(factoextra)
sil <- silhouette(KM$cluster, dist(Data_scaled))
fviz_silhouette(sil)
|