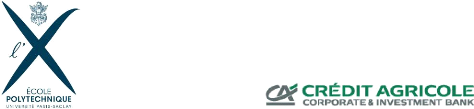
RAPPORT DE STAGE
Diplôme escompté : Master
Probabilités et Finance
Entreprise d'accueil: Credit
Agricole CIB
Equipe GMD - Structures Rates
présenté et soutenu
par
Kaiza Amuh
le 19 Septembre 2014
Sujet du stage:
Etude du modèle ZABR et du Normal SABR
Maître de stage: Vincent Porte
Jury
M. Emmanuel Gobet, Examinateur
11
iii
Résumé
Dans ce document, nous exposons et résolvons deux
problèmes rencontrés par les praticiens avec l'utilisation du
modèle SABR : un problème de densité négative et un
problème de contrôle des ailes. Pour le premier problème,
nous proposons un modèle initialement développé par
Philippe Balland, le SABR Normal. Ce dernier permet d'effectuer du pricing sans
arbitrage mais présente deux inconvénients majeurs: un temps de
calcul excessif et le fait même de changer de modèle de base. Le
second problème quant-à lui nous amène à introduite
un nouveau modèle, extension du SABR, appelé modèle ZABR.
Celui-ci permet de contrôler les ailes du smile grâce à un
paramètre particulier mais présente aussi des arbitrages lorsque
l'on tente de faire du pricing directement avec les volatilités
implicites obtenues en les injectant dans les formules de pricing usuelles de
vanilles provenant des modèles de Black-Scholes et de Bachelier.
Néanmoins, nous exposons une technique de migration vers un
modèle à volatilité locale équivalent au ZABR,
essentiellement basée sur de la projection markovienne, qui permet de
faire un pricing plutôt "à la Dupire". Ceci permet effectivement
d'éviter tout arbitrage, de part la construction même des
modèles à volatilité locale. Nous exposons aussi deux
façons de calibrer le modèle ZABR, une première assez
simple mais peu précise, et une seconde plus fastidieuse
découlant du modèle à volatilité locale
équivalent au ZABR. Nous faisons ensuite une comparaison des
différents temps de calcul et montrons enfin comment le contrôle
des ailes de la volatilité implicite peut permettre une couverture plus
efficace contre les risques liés à chacun des paramètres
du modèle.
iv
Abstract
In this document, we highlight and solve two problems that are
encountered by practitioners with the use of the SABR model : a negative
density problem and a wings control problem. For the first one, we propose a
model initially developed by Philippe Balland, the Normal SABR. The latter
allows arbitrage-free pricing but presents two main drawbacks: an excessive
computation time and the change of the basis model. The second problem leads to
the introduction of a new model, an extension of SABR called ZABR. This one
offers a parameter that controls the wings of the smile but also yields
arbitrage while attempting a direct pricing with obtained implied volatility,
using usual vanilla pricing formulae from Black-Scholes and Bachelier model.
However, we expose a trick in order to migrate towards a local volatility model
equivalent to ZABR, mainly based on markovian projection, that allows a
"Dupire-like" pricing. Doing this completely eliminates arbitrage, because of
the very construction of local volatility models. We also present two different
ways to calibrate the ZABR model, a first one simple but inaccurate, and a
second one which is a direct consequence of the equivalent local volatility
modelling and a little bit harder to implement.
v
Acknowledgement
Foremost, I would like to express my sincere gratitude to my
advisor Vincent Porte and his colleague Harry Bensusan for their continuous
support for my internship, their motivation, enthusiasm, and immense knowledge.
Their guidance helped me each time of my research and during the drafting of my
report.
Beside my advisor, I would like to thank the whole Structured
Rates team of Crédit Agricole, specially Eric N., Thomas T. and Nicolas
B. for their availability and their insightful comments.
My sincere thanks also go to all my teachers, specially
Emmanuel Gobet, Nicole El Karoui and Vincent Lemaire for their art of
dispensing courses very clearly.
ACKNOWLEDGEMENT
? ACKNOWLEDGEMENT
vii
Contents
Résumé ..................................... iii
Abstract ..................................... iv
Acknowledgement ............................... v
Contents
..................................... vii
Introduction 1
1 Problems encountered with SABR model 3
1 Negative density problem ......................... 3
2 Wings Control ............................... 7
2 Normal SABR 11
1 Equivalent SABR local volatility ..................... 12
2 Asymptotic expansion with different base models ...........
13
3 Approximation for normal SABR .................... 15
4 Pricing formula with normal SABR as base ............... 16
3 The ZABR model 21
1 Short maturity expansion ........................ 22
2 Application to benchmark models .................... 24
2.1 Local Volatility model : case ~(ót) = 0 .............
24
2.2 Degeneracy into a SABR model : case c(ó) =
áó ....... 25
3 Expansion for the ZABR model ..................... 26
3.1 Implied volatility computation ..................
26
3.2 Graphical results ......................... 27
3.3 Fast calibration of the model's parameters
........... 29
4 Finite difference volatility ........................ 30
5 Calibrating the Volatility function .................... 32
Conclusion 35
Appendices 35
A Numerical pricing under Normal SABR model 37
1 Density for Normal SABR ........................ 37
2 Computation of functions Ö and ê ...................
38
viii CONTENTS
CONTENTS
B Equivalence between Normal and Log-normal Implied
Volatility 41
1 Another pricing formula for call options in the Bachelier model
. . . . 42
2 Asymptotics of the implied normal volatility ..............
45
2.1 First and Second order expansion ................ 45
2.2 Accuracy of asymptotic expansions ............... 47
3 Comparing greeks and delta-hedged portfolios .............
49
Bibliography 53
1
Introduction
European options are often priced and hedged using Black's
model, or equivalently, the Black-Scholes model. In Black's model there is a
one-to-one relation between the price of a European option and the volatility
parameter óLN. Consequently, option prices are
often quoted by stating the implied volatility
óLN, the unique value of the volatility which
yields the option's dollar price when used in Black's model. In theory, the
volatility óLN in Black's model is a constant.
In practice, options with different strikes K require different
volatilities óLN to match their market prices.
Handling these market skews and smiles correctly is critical
to fixed income and foreign exchange desks, since these desks usually have
large exposure across a wide range of strikes. Yet the inherent contradiction
of using different volatilities for different options makes it difficult to
successfully manage these risks using Black's model.
The development of local volatility models by Dupire [11] and
Derman-Kani [10] was a major advance in handling smiles and skews. Local
volatility models are self-consistent, arbitrage-free, and can be calibrated to
precisely match observed market smiles ans skews. Currently these models are
the most popular way of managing smile and skew risk. However, the dynamic
behaviour of smiles ans skews predicted by local volatility models is exactly
the opposite of the behaviour observed in the marketplace: when the price of
the underlying asset decreases, local volatility models predict that the smile
shifts to higher prices. In reality, asset prices and market smile move in the
same direction. This contradiction between the model and the marketplace tends
to de-stabilize the delta and vega hedges derived from local volatility models,
and often these hedges perform worse than the naive Black-Scholes' hedges.
To resolve this problem, Hagan, Kumar, Lesniewski and Woodward
derived the SABR model, a stochastic volatility model in which the asset price
and the volatility are correlated. Singular perturbation techniques are used by
the former authors in order to obtain the prices of European options under the
SABR model, and from these prices they obtained a closed-form algebraic formula
for the implied volatility as a function of today's forward price and the
strike. This closed-form formula for the implied volatility allows the market
price and the market risks, including vanna and volga risks, to be obtained
immediately from Black's formula. It also provides good, and sometimes
spectacular, fits to the implied volatility curves observed in the marketplace.
More importantly, the formula shows that the SABR model captures the correct
dynamics of the smile, and thus yields stable hedges.
2 INTRODUCTION
INTRODUCTION
Why models ? Objectively, it is no good pricing a liquid
asset; getting its price directly from the market is largely sufficient. The
purpose of models is the pricing of illiquid or scarce assets, such as vanilla
options with extreme strikes. Thus, a usable model is one which doesn't break
down under extreme conditions. However, SABR model is rather used a reading
tool: market data is usually transformed into model parameters through
calibration to vanilla assets. Then, the obtained market data (stored as a
matrix of SABR parameters) is used for the calibration of more complicated
models designed for the pricing of exotic options.
However, since the financial crisis that began in 2007, the
american Federal Reserve conducts monetary policy to achieve maximum
employment, stable prices, and moderate long-term interest rates. In addition,
the Fed purchased large quantities of longer-term Treasury securities and
longer-term securities issued or guaranteed by government-sponsored agencies
such as Fannie, Mae or Freddie Mac. With such low rates, the SABR model,
endowed with Hagan approximation for implied volatility, yields arbitrage. This
arbitrage is observable through the negative density of the underlying
process.
Furthermore, Interest rate option desks typically need to
maintain very large amounts of interlinked volatility data. For each currency,
there might be 20 expiries and 20 tenors, that is, 400 volatility smiles.
Furthermore, the smiles might be linked across different currencies.
Interpolation of observed discrete quotes to a continuous curve is needed for
the pricing of general caps and swaptions. At the same time, extrapolation of
options quotes are needed for constant maturity swap (CMS) pricing. The SABR
model only has four parameters to handle the mentioned tasks, which is not
enough flexibility to exactly fit all option quotes.
In this document, we shall outline some problems encountered
with SABR model nowadays. We will first solve each problem, then highlight a
new model that solves both of our problems.
3
Chapter 1
Problems encountered with SABR
model
Introduction
1 Negative density problem
The industry's standard SABR model (SABR stands for
Stochastic-Alpha-Beta-Rho) is a stochastic volatility model defined as
follows:
{ dFt =
ótFtâdWt1
dót =
áótdWt2
|
with d(W1, W2)t = ñdt
(1.1)
|
Where
· ñ E] - 1,1[ represents the link between the
forward and its volatility.
· â E [0, 1] is the elasticity of the forward's
backbone and is usually assumed constant, requiring then no calibration.
· á > 0 is the parameter that represents the
volatility of the volatility process.
Such a model presents a huge advantage because it takes into
account the randomness of the volatility parameter of a CEV process. It is then
crucial to be able to make a fair pricing under this model. A key is to rather
compute a lognormal implied volatility and then plug it into the Black-Scholes
formula in order to retrieve the price of the option.
In [26], Hagan et al. used singular perturbation
theory and found the following closed form formula for the implied volatility
in the SABR model
C z ~
óBS(K, F) = -

(FK)(1-â)/2 (1 +
(124)2 log2(F/K) +
(1-92ô4 log4(F/K) +
...) x(z)
ó0
- â)2á2
ñâíá2 - 3ñ2 2
C1 + [ (1
24(F/K)1-â +
4(FK)(1-â)/2 + 24 í ]
tex + ...
(1.2)
CHAPTER 1. PROBLEMS ENCOUNTERED WITH SABR
MODEL
v1-2ñz+z2+z-ñ
where x(z) = log(
1-ñ ) and z =
í á(F
K)(1-â)/2
log(F/K)
This formula has the advantage to be fast to compute and,
more, is a closed formula. Generally, closed formulas are preferred in the
financial industry because of their rapidness and few need of resources.
The same formula could also be obtained applying infinite
dimensional analysis and Malliavin calculus. In [34], the author considered a
slightly more general model which converges towards the original SABR model and
used a large deviation approach based on the non degeneracy of Malliavin
covariance. The Dynamic SABR model is rather used for FX Option markets.
Malliavin calculus can be used in a more general scope : the
decomposition of a process into consecutive Wiener chaos yields an exact
solution to all stochastic differential equations, provided they really have a
unique one [...].
However, even though this formula apparently suits to our
needs, it produces arbitrage for sufficiently low rates and long maturities.
That arbitrage is also observable when â is set to low values.
In order to highlight the arbitrage, let's compute the
probability density function of the underlying:
Let pF denote the underlying probability density function
(which is then supposed to exist) and PF the corresponding repartition
function.
If we compute the price of a call option under the suitable
forward probability QT, we'd have:
Ct = EQT
((FT - K)+
) t
?Ct
|
|
?
|
(FT1{FT
>K} - K1{FT >K})
|
?K =
|
?K
|
4
1. NEGATIVE DENSITY PROBLEM
?2Ct
?K2
|
= -EQT
(1{FT >K}) t
= -QT
(FT > K) Z +8
= - pF
(y)dy
K
[ ~
?
= PF
(K) -
lim
y?+8 PF
(y)
?K
|
=
pF(K)
Where Ct denotes the price of a European call option of strike
K, written on the underlying
(Ft)t.
Thus, for a set of strikes, we first compute implied
volatilities with Hagan formula, then price a set of calls for each strike, and
finally compute a numerical derivative of the call price twice according to the
strike.
The result is the so-called probability density that we have
plotted in the following picture for several maturities.
1. NEGATIVE DENSITY PROBLEM
5
CHAPTER 1. PROBLEMS ENCOUNTERED WITH SABR MODEL
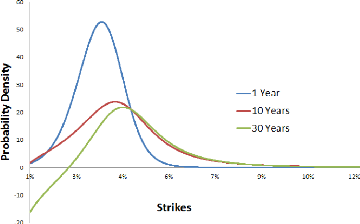
Figure 1.1: F0 = 0.0325, ó0 = 0.087,
á = 0.47, â = 0.7, ñ = -0.48
Indeed, as we can see it on the above picture, we may have
some negative densities while increasing the option's maturity.
In order to improve the accuracy of the implied volatility
approximation, Henry Labordere used a heat kernel expansion on a Riemann
manifold endowed with an Abelian connection in [27] and found an approximation
for a more general scope of stochastic volatility models. Applying this
asymptotic development to SABR model yields:
óN(K,T) = S0(K)(1 + TS1(K)) (1.3)
where
1 (x l aauñsinh(d(x))
- 1 S0(x)2xF
S0(x) = S(x) log \Fl , 8 (x) _ 4
(/Eâ-1) d(x) 28(x)2 log
a(x)a(F)xâFâ
q
á(x1-â - F
1-â)
q(x) = 1 - â , a(x) = óô +
q(x)2 + 2ó0ñq(x)
S(x) = 1 log q(x)
u+ o(1 o-0+p +pp) a(x)
d(x) = argch -q(x)ñ -
ó0ñ2 + a(x)
á ó0(1 -
ñ2)
N/
~ = KF
The above implied volatility is a normal one, i.e. retrieved
from an inversion of the pricing formula in the Bachelier Model. For
comparison, we can approximately find back a Black-Scholes implied volatility
through the following equivalence:
óN =
|
2 ~S-K ~
3
V'
S - K log KS(log S-log K)
2 2
log S - log K óLN 1 -(log S
- log K)2 óLNT + O (T log(T)
(1.4)
|
CHAPTER 1. PROBLEMS ENCOUNTERED WITH SABR
MODEL
In particular, uN ~ S-K
log S-log K óLN when T
? 0. We gave a detailed proof of this
equivalence in Appendix B.
The following picture shows how Labordere's approximation
improves the accuracy of implied volatility asymptotics.
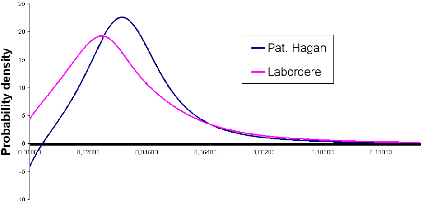
Figure 1.2: F0 = 0.0325, u0 =
0.087, á = 0.47, 9 = 0.7, p = -0.48, T = 15Y
For a 15 years expiry, the negative densities observed with
the Hagan expansion simply vanish. Despite that accuracy, for a sake of rigor,
we make some model parameters "worse" and track the behaviour of the
probability density. We know that a SABR model with parameter 9 = 1 and
constant volatility is identically a Black-Scholes model. We can therefore
reasonably expect the model to spread from the basic Black-Scholes when 9 ? 0.
In the following picture, we choosed 9 = 0.4: let's see what happens.
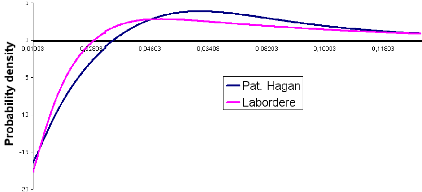
6 1. NEGATIVE DENSITY PROBLEM
Figure 1.3: F0 = 0.0325, u0 =
0.087, á = 0.47, 9 = 0.4, p = -0.48, T = 15Y
CHAPTER 1. PROBLEMS ENCOUNTERED WITH SABR
MODEL
As we can remark it, both Hagan and Labordere approximations
fail under extreme conditions. This is not really surprising since those
formulas are simply short-maturity expansion results. Hence, we address a more
qualitative question: which one practitioners prefer between fast to compute
approximations and heavy accurate calculus ?
The SABR model can be used to accurately fit the implied
volatility curves observed in the marketplace for any single exercise date.
More importantly, it predicts the correct dynamics of the implied volatility
curves. This makes the SABR model an effective means to manage smile risk in
markets where assets only have a single exercise date; these markets include
swaption ans caplet/floorlet markets.
2 Wings Control
We now address the issue of wings control. The price of
illiquid assets extremely depends on the shape of the implied volatility wings.
This is for example the case of the price of a CMS, specially due to the
computation of convexity adjustments. A high out-of-money implied volatility
yields high prices.
Here is for example how SABR parameters control the smile: The
lower we set á, the more we spread the smile...
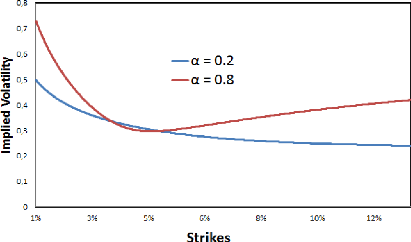
Figure 1.4: F0 = 0.0325, u0 = 0.087, â = 0.7, p = -0.48,
T = 15Y
2. WINGS CONTROL 7
The higher we set â, the flatter the smile gets...
8 2. WINGS CONTROL
CHAPTER 1. PROBLEMS ENCOUNTERED WITH SABR
MODEL
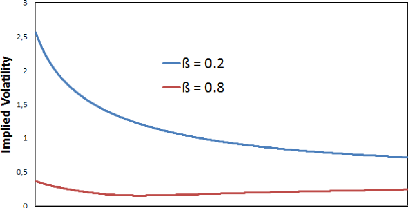

Figure 1.5: F0 = 0.0325, ó0 = 0.087,
á = 0.47, p = -0.48, T = 15Y
This is not surprising since for 9 = 1 and a constant volatility,
we face a Black-Scholes model and the latter produces nothing but a flat smile
!
Increasing p rotates the smile in a counter-clockwise
direction.
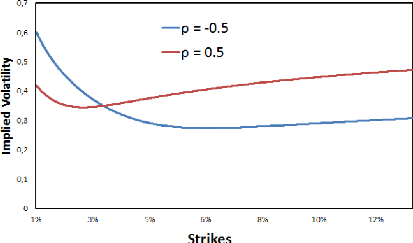
Figure 1.6: F0 = 0.0325, ó0 = 0.087,
á = 0.47, 9 = 0.7, T = 15Y
We therefore focus on finding other model parameters, or at
least, adapted transformations of SABR model that may provide additional
control features.
2. WINGS CONTROL 9
CHAPTER 1. PROBLEMS ENCOUNTERED WITH SABR
MODEL
Practitioners usually focus on changing the backbone shape of
SABR model, that is, the curve of ATM implied volatilities for different
strikes. In order to add more control parameters to the model, we can replace
the ?(F) = F'3 in the underlying SDE
by:
· ?(F) = F'3(F) with
â(F) = â0 + (â8 -
â0) (1 - e-F/Fmax)
where Fmax is typically much larger than the forward
rate F0. This gives a control on the upper-wing of
the smile.
· ?(F) = F'3 x
(F/F1)$1+1
(F/F2)$2+1. This
parametrisation allows us to control both lower
and upper wings.
· ?(F ) = F $1
1+F$1-$2 .
The later was suggested to me by the Fixed Income Derivatives
Quants of Crédit Agricole, and has the particularity to converge to
different SABR models. Indeed,
(1.5)
F '31
lim ?(F) = lim = F'32
F?+8
F?+8 1 +
F'31-'32
F '31
lim ?(F ) = lim = F '31
F ?0
?0
F 1 +
F'31-'32
This model tends then to a
SABR(â1) for low values of the forward and a
SABR(â2) for high forwards.
Despite those improving attempts, practitioners still face a
major problem: the above listed backbone transformations lead to a full control
of the smile, both liquid and illiquid regions. As highlighted in the
introduction, models are needed for illiquid assets; however, models should
first behave well for liquid assets for a sake of calibration. If one modifies
SABR's behaviour for the whole smile, one although fits illiquid region's
behaviour but also loses the liquid region's behaviour. This is therefore a
destruction of the cornerstone of our model.
What we need is another model that provides a real parameter
for wings control without changing the model's behaviour for liquid assets. We
will therefore propose a new model which is able to change wings without
(sensibly) touching the liquidity region.
Conclusion
In addition to the negative density problem for very
in-the-money vanilla options, the SABR model lacks an additional control
parameter for very out-of-money options.
In the next chapter we will expose solutions for both of
these problems. We shall develop a Normal SABR model which solves the negative
density problem, and then study the ZABR model that controls the wings.
CHAPTER 1. PROBLEMS ENCOUNTERED WITH SABR MODEL
10 2. WINGS CONTROL
11
Chapter 2
Normal SABR
Introduction
As mentioned in the previous chapter, problems with the SABR
implementation through the Hagan expansion, such as the breakdown of the
expansion for high volatility and the possibility of negative probabilities for
very low strikes, did not matter at the time but now constitute a pressing
problem for the swap and rates options markets. In this chapter, we present a
solution to these problems based on Philippe Balland and Quan Tran
expansion (see [7])
The SABR backbone function ?(.) satisfies the usual
linear growth and Hölder continuity conditions to ensure that the SABR
stochastic differential equation admits a unique solution when appropriate
boundary conditions are specified.
In the original dynamics, ?(F) = Fâ
and the forward rate is assumed to be absorbed at zero. The
constant elasticity of variance (CEV) â is typically greater
than zero and smaller than one in interest rate applications. Negative rates
can be accommodated by assuming that (Ft +
Ä)t follows SABR dynamics. The model is very popular
among practitioners because it provides an intuitive parametrisation of
volatility smiles.
Unfortunately, the asymptotic formula derived by Hagan et
al. (2002) loses accuracy for long-dated expiries, especially when the CEV
exponent is close to zero or when the volatility-of-volatility is large. This
loss of accuracy is problematic from a practical point of view because the
density can become negative near the forward. New techniques have recently been
proposed to improve the accuracy in the original expansion of the implied
volatility. When the correlation is zero, Antonov & Spector [35] derived an
exact expression for the price of a vanilla option based on a double integral.
When the correlation is non-zero, the authors proposed using an approximately
equivalent SABR model with zero correlation.
Small CEV exponents are typically used to represent swaption
and caplet smiles at the long end of the curve, where the asymptotic formula
also breaks down. Based on this observation, we perform an asymptotic expansion
of the implied volatility corresponding to Normal SABR with absorption at zero,
instead of Black-Scholes. We find that the resulting approximation is more
accurate than the original SABR
and the measure d Q
dQ
Q except for the drift of ót:
ñt. We note that Jt has the same dynamics under Q and
12 1. EQUIVALENT SABR. LOCAL VOLATILITY
CHAPTER. 2. NOR.MAL SABR.
expansion and results in significant calculation time saving
when compared with solving the one-factor equivalent local volatility PDE.
1 Equivalent SABR local volatility
As explained in [15] and [25], we can obtain an accurate
approximation of the local volatility equivalent to SABR. The local volatility
g(t, K) for SABR is given by the following expression:
g(t, K)2 = ?(K)2E [ó2
t ä (Ft - K)] (2.1)
E [ä (Ft
- K)]
We denote the numerator of this expression (the so-called
local time) by Lt, and the denominator (the process's probability density) by
Dt. In this section, we derive an approximation for g(t, K) by simple
applications of Itô's lemma and Girsanov's theorem. We have included this
derivation as it will serve as the basis for our normal SABR expansion.
The SABR local time Lt is approximated by introducing the
process:
1 du
J(Ft,ót) =ót jFt
(u) (2.2)
and observing that:
Lt = ó0?(K)E [eáWt2-2tä(Jt)]
(2.3)
By applying Itô's lemma and performing the change of measure
dbQ
dQ =
eáWt2-21á2t,
we derive:
Lt = ó0?(K)
bE [ä(Jt)]
1 (2.4)
dJt = \/q(Jt)dcWt - 2
ÿ?(Ft)ótdt
where q(J) = 1 - 2ñáJ +
á2J2 and (Wt)t is a brownian motion
under bQ.
The SABR density Dt is similarly approximated by performing the
change of
-áWt2-21á2t :
E [ä(Jt)/ót]
D=
?(K)
=
measure dQ
dQ = e
E [ä(Jt)]eá2t
ó0?(K) (2.5)
p
dJt = q(Jt)d Wt + ÿq(Jt)dt - 21
ÿ?(Ft)ótdt We define the martingale:
dñt ÿq(Jt)
= d W (2.6)
ñt Nq(Jt)
CHAPTER 2. NORMAL SABR
1 !
Z t ÿd(Ju)
Xt = ñt exp ÿ?(Fu)óuq(Ju)du (2.7)
2 0
It follows that the SABR density satisfies:
i
E hq(J0)
eá2t
q(Jt)ä(Jt)
q(J0)ó0?(K)
Dt =
=
E hexp C2 R0
ÿ?(Fu)óuÿqqt du) /Jt =
0i
×
q(J0)ó0?(K)
(2.8)
E [ä(Jt)]
Since the volatility ót only appears in the drift
expression of Jt, we conclude that
bE[ä(Jt)]
E[ä(Jt)]
= 1 + O(t2). We consequently have:
g(t, K)2 = q(J0)ó20?(K)2e 2
(ñáÿ?(K)-21ÿ?(F0)Z0))t
+ O(t2) (2.9)
We finally derive the following first-order approximation in
time of the SABR local volatility:
p
g(K) = ó0?(K) 1 + 2ñáf(K) +
á2f(K)2,
Z K
1 du (2.10)
f(K) = ó0 ?(u)
F0
Using this equivalent local volatility, we obtain Hagan's first
order approximation for the implied volatility under SABR using standard
results for local volatility:
ln (K/F0) =(2.11)
R f(K;?) dí
0 v1+2ñáí+á2í2
ln (K/F0)
IV (K; ?, á, ñ) =
RK du
0 g(u)
The SABR local volatility behaves like a CEV dynamic near
zero. The absorption at zero is ignored in the above approximation because we
are using Black-Scholes as the base model for our implied volatility
calculation. Hence, we can expect to improve accuracy by choosing a base model
with a dynamic absorbed at zero.
2 Asymptotic expansion with different base models
Suppose that we can accurately integrate the following
instance of the SABR dynamics:
?
????
????
|
dFt = ót?base(Ft)dWt1
dót = áótdWt2
ót=0 = b0
|
(2.12)
|
|
where á and ñ are as in SABR. By matching the
first-order implied volatility approximations, that is, IV (K; ?base, á,
ñ) = IV (K; ?, á, ñ), we derive the base
2. ASYMPTOTIC EXPANSION WITH DIFFERENT BASE MODELS 13
CHAPTER 2. NORMAL SABR
implied volatility b0 so that the base model and
SABR share the same implied volatility to first order in time:
u
ó0(1 - r
JFK0
(Pbasde(u)
b0 = (2.13)
K1-â - F1-â
0
We consider two base candidates. Our first one is SABR with
shifted lognormal backbone:
?
base 1 (F) = pF + (1 - p)F0 (2.14)
This base
dynamics can be integrated by inverting a Laplace transform. Al-
though tractable, this requires a double integration.
Our second candidate is normal SABR with absorption at zero:
?base 2 (F) = lim â?0
|
Fâ = 1{F>0} (2.15)
|
|
We will see in the next section that SABR with a normal
backbone can be accurately approximated with limited calculation cost. We
observe that the initial normal volatility to use when approximating SABR with
this base model is as follows:
ó0(1 - â)(K
- F0)
b0 = (2.16)
K1-â - F
1-â
0
In the case where we attempt to approximate SABR with an
extended backbone ?(.) instead of a CEV backbone, then our formula for
b0 is generalised as follows:
ó0(K - F0)
(2.17)
b0 = rK du
JF0 ?(u)
As observed in [4], the SABR dynamics calibrated to swaption
smiles do not imply Constant Maturity Swap (CMS) levels consistent with the
market. Various methods have been proposed to address this issue. These
attempts to steepen the upper-strike wing while not affecting the liquid region
and the lower wing too much. They are based on modifying either the density,
conditionally to being in the upper-wing or directly the SABR dynamics.
We can gain control on the upper-wing steepness by assuming
the following backbone:
?(F) = Fâ(F)
F~F~, a~ (2.18)
â(F) = â0 +
(â8- â0)(1 - e- )
where Fmax is typically much larger than the forward
rate F0 in order to localise the effect of double beta to the
high-strike wing.
An alternative is to use the following double-beta backbone
to control both lower and upper wings:
?(F) = Fâ x
(F/F1)â1 + 1 (2.19)
(F/F2)â2 + 1
14 2. ASYMPTOTIC EXPANSION WITH DIFFERENT BASE MODELS
CHAPTER 2. NORMAL SABR
This parametrisation allows us to account for the extra risk
premium for high-strike volatilities and for the fact that traders typically
increase â when interest rates become very low.
3 Approximation for normal SABR
We obtain the following formula for a call option under the
normal SABR model by applying the Tanaka-Meyer formula to a call payout (see
[8]):
T
E[(FT - K)+] = (F0 -
K)+ + 2 J b20E
[u2tä(Ft - K)]
dt (2.20)
0
where ut = ót/b0 with
ó0 = b0. We observe that:
E [u2t
ä(Ft - K) = E
[utä(Xt)]
Xt = Ft - K (2.21)
ut
Finally, we denote by Pu the probability measure associated
with the Radon derivative ut = ót/b0 and
obtain the following formula for a call option under normal SABR:
T
E[(FT - K)+] = (F0 -
K)+ + 2 Z bEu
[ä(Xt)] dt (2.22)
The process (Xt)t satisfies:
p
dXt = b0 q(Xt)dW u
(2.23)
t?ô
where q(X) = 1 -
2ñáX +
á2X2,
á = á/b0 and r is the first
time F hits zero.
The stopping of the diffusion is a consequence of using SABR
with vanishing CEV coefficient. As explained in [25], accounting for this
stopping is important because the support of SABR is the positive half line and
our base model must share with SABR the same behaviour at zero otherwise our
lower-strike wing will be too steep. The importance of using interest rate
models with absorbing and reflecting boundaries is discussed in [12].
Ignoring the volatility-of-volatility, we approximate r
as the first time X hits its expected barrier level under Pu, at
which point Xô = Eu [-K/ut] = -K.
This approximation does not compromise the accuracy of our call price because
it only affects option prices with very low strikes. We can gain additional
control on the lower-wing steepness by assuming that X is absorbed at
the level Fmin - K where Fmin = (p -
1)/pF0 is negative, that is, 0 < p < 1.
We define the following process:
!
xt
It = I(Xt) = / du = 1
ln q(Xt) - ñ + áXt
(2.24)
o q(u) á 1 -
ñ
We can derive an approximation for the density of
(Xt)t at zero using the reflection principle for Brownian
motion (see Appendix A):
3. APPROXIMATION FOR NORMAL SABR 15
CHAPTER 2. NORMAL SABR
Eu [ä(Xt)] = q(X0)14
b0 v2ðt
~ ~
e- B2
b2 0t - e-
b2
× Ë(t) × 0t
16 4. PRICING FORMULA WITH NORMAL SABR AS BASE
I(F0 - K) 2I(Fmin - K) - I(F0 - K)
B = v2 , C = v2
~ ~
Ë(t) e- 8 á2t × Ö
1 t, I0
b0
~ 3 Z t ~ ~
du
Ö(t, z) = E exp 8á2(1 -
ñ2) /Wt = z
f(Wu)
0
1 ~(1 + ñ)2e-2áW + (1 -
ñ)2e2áW + 2(1 - ñ2)~ f(W)
= 4
|
(2.25)
|
|
Hence, we obtain the following approximation for call prices
under SABR:
2 C2
E[(FT - K)+] = (F0 - K)+ + q(F0 -
K)1/4b0 T1ef0 ,L e° (e b20t - e b20t) dt
2v2ð o t
ó0(K - F0) b0 = R K du
F0 ?(u)
ê(t, z) = -8á2 +
?tlnÖ(t, z) 1
(2.26)
The function ê(t, z) is independent of K and only
depends on the SABR parameters á and ñ.
~ 1 ~
1 3 1
2 2 2
(2.27)
We have the following first-order approximation:
Ö(t, z) = exp
[-18á2t
+ 16á2(1 - ñ2) C f(0) +
f(z)/
t]
+ O(t2)
We can estimate ê(t, z), Ö(t, z) more accurately
without any major increase in calculation time. First, we pre-compute by
forward induction Ö (Ti,îjvTi) on a fixed-time grid {Ti}i<N and
an N(0, 1)-mesh {îj}j<M as explained in Appendix A.
~ ~
Finally, we approximate ê s, I0 by a constant
êi over each interval (Ti-1, Ti): b0
êi 1 2
= -8á +
|
h ~ i h ~ i
ln Ö Ti, I0 - ln Ö Ti-1, I0
b0 b0
|
(2.28)
|
|
|
where Ö(Tk, z) is obtained by cubic spline interpolation of
{Ö (Tk, îjvTk) : j = 0,..., M - 1}.
4 Pricing formula with normal SABR as base
From our previous calculations, we derive the following
approximation for the price of an option on a SABR underlying (Ft)t
using normal SABR as a base for our asymptotic expansion:
CHAPTER 2. NORMAL SABR
N
E[(FT-K + = (F0 - K)#177; + q(F0 - K)1/4b
é (âa2+~z)Ti 1~ (T Io × J
) ) 2v2ð o ( Z-1 bo / i
i=1 \
Ji =
|
1 Z Ti 1 vt
2 Ti-1
|
~ ~
e- B2
eêit b2 0t - e- C2
b2 0t dt
|
|
(2.29)
The above integrals Ji are calculated using formula (7.4.33) in
[20]:
I
~ ~v ~ ~~
+ e-2|ë|v-ê -êT - |ë|
erf v + 1
T
T r2
~teêu-û2 du
=Le2|ë|v-ê (erf (-êT + - T(2.30)
where
Z x ~ v ~
2
erf(x) = ?ð e-t2dt = 2N x 2 - 1
(2.31)
0
and v-ê is either imaginary or real. The error function
with complex argument can be estimated using the infinite series approximation
of Abramowitz & Stegun (see formula 7.1.29 in [20]) as suggested in [8]:
+8
e
x2 2
-
n2
-
n2 + 4x2 (fn(x, y) + ign(x, y))
e
4
X
e-x2
erf(x + iy) = erf(x) + 2ðx (1 - cos 2xy +
isin2xy) + ð
n=1
fn(x, y) = 2x - 2x cosh(ny) cos(2xy) + n sinh(ny)
sin(2xy) gn(x, y) = 2x cosh(ny) sin(2xy) + n sinh(ny) cos(2xy)
(2.32)
In practical application, it is sufficient to include the first
10 terms to ensure a very good accuracy. From the above expression, we can
calculate analytical expressions for the cumulative and density functions.
In the following picture, we have plotted the implied density
obtained when pricing under Normal SABR. For a sake of comparison, we have also
plotted densities obtained from Hagan and Labordere approximations.
4. PRICING FORMULA WITH NORMAL SABR AS BASE 17
18 4. PRICING FORMULA WITH NORMAL SABR AS
BASE
CHAPTER 2. NORMAL SABR
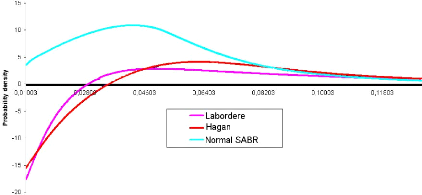
Figure 2.1: F0 = 0.0325, u0 = 0.087, á
= 0.47, 9 = 0.4, p = -0.48, 'y = 1, T = 15Y
Indeed, the negative density problem is solved, even for
extreme model parameters.
The main drawback is a high computation time, mainly due to
the computation of transition probabilities. The latter is however performed
once for all strikes.
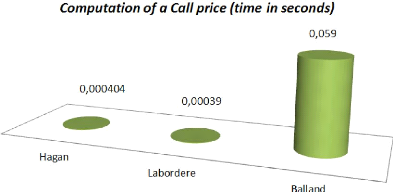
Figure 2.2: F0 = 0.0325, u0 = 0.087, á
= 0.47, 9 = 0.4, p = -0.48, 'y = 1, T = 15Y
The above picture shows how much time it actually takes to
price under Normal SABR. This pricing takes 200 times more time than the Hagan
and Labordere approximations but that is the price we pay in order to eliminate
arbitrage.
4. PRICING FORMULA WITH NORMAL SABR AS BASE 19
CHAPTER 2. NORMAL SABR
Conclusion
The Normal SABR model solves the negative density problem
observed with the Hagan approximation. However, it introduces another issue,
the excessive computation time for pricing. Indeed, practitioners prefer closed
form formulas for pricing (such as Black-Scholes) and changing the whole
pricing kernel can quickly become a trip to Pandemonium. Moreover, solving only
the negative density problem leaves untouched the wings control one. In the
next chapter, we will introduce a wings controlling model and show how to
compute arbitrage-free prices.
CHAPTER 2. NORMAL SABR
20 4. PRICING FORMULA WITH NORMAL SABR AS BASE
Chapter 3
The ZABR model
Introduction
Interest rate option desks typically need to maintain very
large amounts of inter-linked volatility data. For each currency, there might
be 20 expiries and 20 tenors, that is, 400 volatility smiles. Furthermore, the
smiles might be linked across different currencies. Interpolation of observed
discrete quotes to a continuous curve is needed for the pricing of general caps
and swaptions. At the same time, extrapolation of options quotes is needed for
constant maturity swap (CMS) pricing. For these purposes, the industry uses to
approximate SABR model using expansions as in [26]. The implied volatility
expansions have the advantages that they are fast and simple to code but as
mentioned in the previous chapter, these expansions are not very accurate,
particularly not for long maturities nor low strikes.
With the low rates we have today, this problem is more acute
than ever. Furthermore, the SABR model only has four parameters to handle the
above-mentioned tasks, which is not enough flexibility to exactly fit all
option quotes. In this chapter, we extend the stochastic volatility process to
include a constant elasticity of variance (CEV) skew on the volatility of
volatility. The CEV volatility process allows us to have more explicit control
of the extrapolated high-strike volatilities, which in turn allows better
control of CMS prices. Further, we will use a non-parametric volatility
function for the spot process, which enables us to have an exact fit to all the
observed quotes and gives us the ability to model negative option strikes.
In this chapter, instead of buying into heat kernel
expansions, we use a short-maturity expansion for the implied volatility of the
option. The short maturity expansion also yields results for the short-maturity
limit of the Dupire forward volatility ( [11]), that is, the short-maturity
limit of the conditional expected local variance
V(F)2 = uim
t?0
|
|
]
dhF it
dt /Ft = F (3.1)
|
|
21
We provide two procedures to directly calibrate the model to
observed CMS prices: an implicit method that works by iteration of the
connection from parameters to price in a non-linear solver (see Section 3.3),
and a direct method that infers the
22 1. SHORT MATURITY EXPANSION
CHAPTER 3. THE ZABR MODEL
parameters of the model from an arbitrage-free continuous
curve of option prices (see Section 5).
1 Short maturity expansion
We consider the slightly more general model:
( dFt =
ót?(Ft)dWt1
with d(W1,
W2)t = ñdt (3.2)
dót =
c(ót)dWt2
The non-parametric form of the volatility function ?(.)
allows us to have a perfect fit to any discrete or continuous set of observed
arbitrage-free options quotes. We can write the price of an European call
option on a fixing FT as:
Ct = E [(FT -
K)+ /Ft] = g (t, Ft, v(t))
where v(t) is the implied normal volatility and g is the
normal (Bachelier) option pricing formula:
g(ô, x, v) = (x - K)JV x - K +
vôfv.Vô)z
Cv~K) , ô = T
- t (3.3)
Applying Itô's lemma to 3.3 yields:
dCt = -gôdt +
gxdFt +1
gxxd(F)t + gvdvt +
2gvvd(v)t +
gxvd(F, v)t (3.4)
where subscripts
denote partial derivatives. In the following, we assume vt > 0
Define Xt = Ft-K. Using Itô's
lemma yields:
vt
1
dXt =
vt
|
dFt -Ft 2Kdvt -
2d(F,v)t + Ft
-3Kd(v)t
vt vt
vt
|
|
(3.5)
(3.6)
1
= (dFt - Xtdvt) +
O(dt)
vt
d(X)t = v2
(d(F)t + Xt d(v)t -
2Xtd(F, v)t)
t
2
gxx
gxx
The normal option pricing function, g, has the following
properties:
gv = vôgxx
Cx - K
gvv = v
x - K
v
gxv =
1
0 = -gô +
2v2gxx
Using the above properties, we can transform equation 3.4
into:
1. SHORT MATURITY EXPANSION
23
CHAPTER 3. THE ZABR MODEL
1 ]
dCt - gxdFt = 2gxx [v2
t (d(X)t - dt) + 2ôvdvt (3.7)
The left
hand side of 3.7 is the change in value of a hedged portfolio. Taking
conditional expectations yields:
1
0 = 2gxxv2
1 t E (d(X)t - dt/Ft) +
gxxôvtE (dvt/Ft) (3.8)
For small
maturities, ô -+ 0, and we have
2gxxv2t E
(d(X)t - dt/Ft) 0 (3.9)
As gxx > 0 for v > 0, and for any diffusion, E
(d(X)t - dt/Ft) = 0 is equivalent to
d(X)t = dt, we obtain the arbitrage condition:
Note that this is a diffusion condition rather than the drift
condition that we normally see in financial mathematics. As the function X
H X(f, ó) must be a function of the state variables
(Ft, ót), the diffusion condition 3.10 leads to the
differential equation:
1 = (XfdFt +
Xódót)2
dt (3.11)
=
ó2t
?(Ft)2X2f
+
E(ót)2X2ó
+
2ñót?(Ft)c(ót)XfXó
Given the function ?(.), we need to solve this non-linear
first order differential equation subject to the boundary condition X(f = K,
ó) = 0. Once we have the solution X(f, ó), we can find the
implied volatility as:
F - K
=
v(3.12) X(F,ó0)
We note that the error of the implied volatility is
O(ô). The result implies that for any choice of ?(F), any function X =
X(f, ó) that satisfies d(X)t = dt leads to an implied
volatility given by v = (F - K)/X.
We could have chosen to derive the short-maturity expansion
in implied Black-Scholes (lognormal) volatility v instead of implied normal
volatility. Instead of X,
we should then have chosen the transformation
. The diffusion condi-
X = ln(F/K)
v
tion would be the same so X = X. This relates
short-maturity implied lognormal and normal volatilities, as in Appendix B
(first order equivalence), by the simple relationship:
v ln(F/K)
= (3.13)
v F - K
The expansion results that we present in the following can
easily be switched between use in implied normal and implied lognormal
volatility form by use of equivalence formulae.
CHAPTER 3. THE ZABR MODEL
2 Application to benchmark models
Before we address the very ZABR model results, we first of all
apply the short-maturity expansions from the previous section to well known
models. Those models can be retrieved while varying the function c(.).
2.1 Local Volatility model : case ~(ót)
= 0
In this case, ót = 1, and the differential equation 3.11
reduces to ordinary differential equation (ODE):
X2f?(F)2 = 1 (3.14)
Using the boundary condition X(F = K) = 0, we find the
solution:
Z F 1
X = ?(u)
K
|
du (3.15)
|
|
v = v =
with corresponding implied normal and Black volatilities given
by:
F - K
f F K ?(u)du
1
(3.16)
ln(F/K)
f F K ?(u)du
1
These results appear in many places, for example in [19]. We
note that 3.15 implies the following relationship between X and the forward
volatility:
Suppose we have X from a stochastic volatility model like
3.2, that is, given as the solution to 3.11 for some volatility functions ?(F),
c(ó) and correlation ñ. Let's define the function V by:
~?X ~-1
V(K) = - (3.18)
?K
and consider the deterministic local volatility model:
dFt = V(Ft)dWt (3.19)
It now follows that:
XLV =
|
Z S K
|
V(u)-1du = X (3.20)
|
|
24 2. APPLICATION TO BENCHMARK MODELS
So the stochastic volatility model 3.2 and the local
volatility model 3.19 will produce the same short-maturity expansion option
prices.
The above is a short-maturity limit version of the general
result by Gyongy and Dupire (see [13] and [6]), that the model:
CHAPTER 3. THE ZABR MODEL
dFt = a(t, Ft)dWt , F0 = F0 (3.21)
produces the same option prices as the model 3.2 if a(., .) is
chosen to be:
~dhF it ~
a(t, k)2 = E dt /Ft = k (3.22)
We conclude that in the short-maturity limit, the conditional
expected variance of the underlying is related to the transformed variable X
by:
-2
V(F)2 t~o E
[dhdtit/Ft =
F~ = (?K\ (3.23)
This constitutes a way of relating the two dimensional
pricing problem 3.2 to the simpler one-dimensional pricing problem 3.19. We
will make use of this relationship to generate arbitrage-free prices later.
2.2 Degeneracy into a SABR model : case €(ó) =
áó
Here, we will solve the diffusion condition for the lognormal
volatility process case. First, we use the transformation:
Y :=
LF
?(u)
1
du (3.24)
and we get:
dY = dWt1 -
áYdWt2 + O(dt)
= [1 + á2Y 2 -
2ñáY ]1/2 dBt + O(dt) (3.25)
= J(Y )dBt +
O(dt)
where (Bt)t is a new Brownian motion. As Y (F = K)
= 0, we can now get X by normalising the volatility of Y , hence:
fY X=J J(u)-1du
= 1ln(J(Y ) - ñ + áY\
o 1--p
v =
|
F - K
|
|
(3.26)
|
|
|
|
ln(F/K) X
|
|
|
For the CEV case ?(F) = ?0Fâ, we have:
1
Y = ó0?0
F1-â - K1-â
(3.27)
1 -â
These formulas are basically the result of Hagan et al
[26]. This is extended to include maturity and various refinements for the
CEV case. The Hagan result does,
2. APPLICATION TO BENCHMARK MODELS 25
CHAPTER. 3. THE ZABR. MODEL
however, produce implied volatility smiles that are prima
facie identical to those produced with formula 3.26.
We can also use 3.26 to retrieve the forward volatility
function of SABR from:
?X ?K =
|
?X
?Y
|
?Y ?K =
|
J(Y ) Ç0?(K))
(3.28)
|
|
26 3. EXPANSION FOR. THE ZABR. MODEL
Hence:
V(K) = J(Y
)ó0?(K) (3.29)
This result could also be deduced from results in [25].
3 Expansion for the ZABR model
We now consider the extended SABR model where the volatility
process is of the CEV type: c(ó) =
áó,y
3.1 Implied volatility computation
Once again, we introduce the intermediate variable:
F
Y = ó,y-2 1
x ?(u)
|
du (3.30)
|
|
For which Itô expansion yields:
dY = ó,y-1 (dW t 1 +
(ã - 2)áYdW 2 +
O(dt) (3.31)
0 t
Let's define X = ó1-,y
0 f(Y ), for some function
f(.), and we get:
dX =ó1-,y
0 f'(Y)dY + (1 -
ã)áf(Y)dWt2
+ O(dt)
[ ] t + O(dt) (3.32)
= f'(Y)dW t 1 +
(ã - 2)áY f'(Y) +
(1 - ã)áf(Y) dW 2
We conclude that the diffusion condition 3.9 is satisfied if f
solves the ODE:
1 = A(Y )f'(Y
)2 + B(Y )f(Y
)f'(Y ) + Cf(Y
)2
A(Y ) = 1 + (ã -
2)2á2Y 2 + 2ñ(ã -
2)áY
B(Y ) = 2ñ(1 -
ã)á + 2(1 - ã)(ã -
2)á2Y
C = (1 -
ã)2á2
f(0) = 0
The above ODE can be rearranged as:
|
(3.33)
|
|
f'(Y ) =
Y -B(Y )f + B(Y
)2
f2 - 4A(Y )(Cf2 - 1))
F(Y, f) (3.34)
2
CHAPTER 3. THE ZABR MODEL
which can be solved by standard techniques for integration of
ODEs. We can evaluate the solution for all strikes one sweep by:
= -ó0
?(K)-1
ã-2
?Y ?K
?K =
ó1-ã
0
=
-ó-1
0 F (Y,
óã-1
0 X)
?(K)-1
(3.35)
?X
?f ?K
X(K =
F) = Y (K
= F) = 0
Again, we can find the forward volatility function as:
/?X\-1=
ó0?(K)f'(Y
)-1 =
ó0?(K)F
(Y, óã-1
0 X)-1 (3.36)
V(K) = -
?K
Equations 3.35 and 3.36 will typically be evaluated at
ó0 = 1. Rather than numerically solving the
two ODEs in 3.35 separately, we favour solving 3.33 as a joint system.
It should here be noted that the ODE representation 3.33 has
previously been obtained by Balland (see [24]) for the lognormal case. Further,
it should be noted that Henry-Labordere has a treatment of the general non-CEV
case (see [27]).
3.2 Graphical results
In order to solve the ODE 3.33, we have the choice between a
classical Euler scheme and an 4th order
Runge Kutta relaxation. The former is faster but deliver unstable solutions,
whereas the latter, even though slower, yields excellent solutions in terms of
stability. We therefore chose a RK4 method to solve the ODE. After solving it,
we find a value for X which leads to the implied volatility. The following
picture plots obtained lognormal implied volatilities for different values of
ã.
3. EXPANSION FOR THE ZABR MODEL 27
28 3. EXPANSION FOR THE ZABR MODEL
CHAPTER 3. THE ZABR MODEL
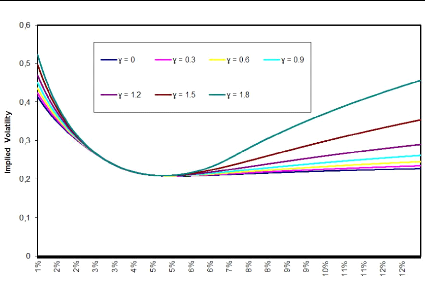
Figure 3.1: F0 = 0.0325, u0 = 0.087, á
= 0.47, 9 = 0.7, p = -0.48, T = 15Y
Increasing 'y lifts the wings of the implied volatility smile
whereas the smile for strikes close to at-the-money are visibly unaffected.
This can in turn be used to give us better control over the CMS prices.
Here is an illustration of how to control CMS prices through
the wings. When we increase á, we lift the wings and therefore raise the
CMS prices. We can then decrease 'y and therefore lower back the wings and the
CMS prices, as we can see it through the following illustration.
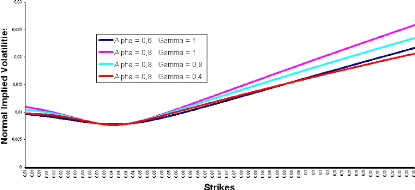
Figure 3.2: F0 = 0.0325, u0 = 0.087, 9 =
0.7, p = -0.48, T = 15Y
In terms of computation time, we have plotted the time it takes
to compute an implied volatility in a ZABR('y = 1) and compared it with the
time taken by Hagan
CHAPTER. 3. THE ZABR. MODEL
and Labordere approximations.
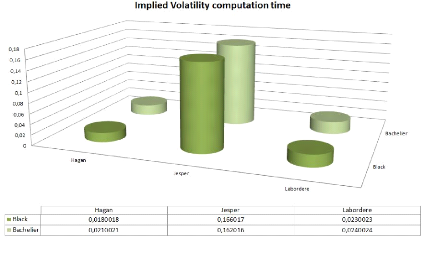
Figure 3.3: F0 = 0.0325, ó0 = 0.087, á = 0.47,
â = 0.7, ñ = -0.48, ã = 1, T = 15Y
It takes 10 times more time for computing implied volatility
under the ZABR model, in comparison with Hagan and Labordere approximations.
However, this is just the price to pay for gaining control of the wings !
3.3 Fast calibration of the model's parameters
For quick identification of the model parameters, the
following second-order Taylor expansion is convenient:
v(K) = v(F) + v,(F)(K - F) +
12v,,(F)(K - F)2 + O ((K -
F)3) v(F) = ó0?(F)
1 v,(F) =2
hó0-1 ñá + ó0? (F)i
v,,(F) 6ó0?(F)hó02(7-1) ((-5 +
2ã)ñ2 + 2) + óô
(2?(F)?,,(F) - ?,(F)2)i
(3.37)
Let's consider a CEV case where we set ?(K) = ù (K-F
)â
have:
(F -F )â and ó0 = 1. Then we
v(F) = ù
v,(F) = 21 [~
ñá + Fùâ - F
~~(-5 + 2ã)ñ2 + 2 á2 +
ù2â(â - 2) ~
v,,(F) = 1
6ù (F - F)2
3. EXPANSION FOR. THE ZABR. MODEL
|
(3.38)
29
|
|
CHAPTER 3. THE ZABR MODEL
|
\ v(K1), ...,
|
|
For a given set of discrete quotes
|
|
|
be used for regressing the triple v(F), v'(F),
v"(F). One can in turn solve 3.38 to get parameters estimates for â,
ñ, á.
4 Finite difference volatility
Using the implied volatility coming from the short-maturity
expansions 3.16, 3.26 and 3.35, directly for pricing using 3.3 will not give
arbitrage-free options prices. Our short-maturity expansions suffer from the
same problem of potential negative implied densities for low strikes as the
original Hagan expansion. The ZABR model contains an enhanced feature that can
help us avoid negative density problems. Let's plot the implied probability
density function for extreme model parameters and see how it reacts to the
changes in ã values.
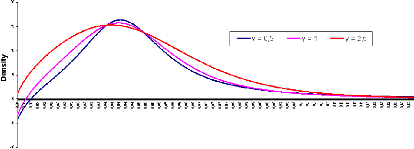
Figure 3.4: F0 = 0.0325, ó0 = 0.087,
á = 0.47, â = 0.7, ñ = -0.48, T = 15Y
If we keep increasing ã, the density tends to be more and
more positive... Anyway, this way of skipping negative densities doesn't give
us enough flexibility in the use of the ZABR model.
In order to definitely avoid this problem, we will instead
use the forward volatilities derived in 3.29 and 3.36 as the basis for our
pricing.
The forward volatility V(K) can be used to generate
option prices as the solution of the Dupire forward PDE (see [5]).
?C(T,K)
?T =
?
?
?
2V(K)2 ?2
?(T2,K)
C(0, K) = (F - K)+
(3.39)
30 4. FINITE DIFFERENCE VOLATILITY
The usual way of solving this numerically is to set up a time
discretisation with multiple time steps and then use a finite difference
solver. However, to gain speed, we will instead use the single time step
implicit finite difference approach introduced in [15]. Here we need to solve
the ODE:
C(T, K) - 2T
è(K)2?2C(T, K)
1 ?K2 = (F - K)+ (3.40)
4. FINITE DIFFERENCE VOLATILITY 31
CHAPTER 3. THE ZABR MODEL
Z0
It is shown in [15] that this approach generates a set of
arbitrage-free call prices for any choice of è. It is also shown that
the one-step finite difference price is the Laplace transform of the solution
to 3.39. The Laplace transform of the Gaussian distribution is the Laplace
distribution:
8 t/T 1 F - Kl T
IF K|
vt
vt
dt = e 2v2 (3.41)
v
v
2v2
which is peaked at K = F. Therefore if we choose è =
V, we will also get a peak in the densities.
Instead, we will find an adjustment for the forward
volatility function based on our expansion results. As option prices generated
by 3.39 and 3.40 should be the
same, we can substitute ?2C(T,K) ?C(T,K)
?K2 = 2 ?T from 3.39 into 3.40 and rearrange to
V2
find:
è(K)2 = V(K)2 C(T, K) - (F -
K)+
T ?C(T,K)
?T
V(K)2g(T, F,v) - (Fv)
- K)+
T ?g(T
?T
|
(3.42)
|
|
~ ~
1 - î Ö(-î)
= 2V(K)2 , with î= v X
ö(î) T
= V(K)2P(X)2
where the second (approximated) equality involves the
approximation of the option prices by our expansion result.
The function P(X)2 can conveniently be
approximated with a third or fifth order polynomial. Specially:
Ö(X) X
ö(X) anun, u = 1 (3.43) 1 + pX
n
where the constants p, a1, a2, ... can be found in (26.2.16)
and (26.2.17) of [20]. The finite difference discretisation of 3.40 is:
~ ~
1- 1 2Tè(K)2 ?2 C(T, K) = (F - K)+
(3.44)
?K2
This equation can be represented as a tridiagonal matrix
equation on the grid K0, K1, ..., Kn, which in turn can be solved
for C(T, Ki) in linear CPU time using the tridiag() algorithm in [31].
As an alternative to the finite difference solution 3.44, one
could use the exact solution methodology for ODEs of the type 3.40 described in
[3]. However, for this methodology to be computationally effective, the forward
volatility function è(K) needs to be well approximated by a piecewise
linear function with few knot points over the full domain of the solution. This
is generally not the case here. We have therefore chosen to base our solution
on 3.44.
We can see that the finite difference generated option prices
have corresponding implied densities that are positive, that is, arbitrage is
precluded. We can also
CHAPTER 3. THE ZABR MODEL
see that using our forward volatility result,
V(K), directly in the
single time step finite difference solver produces a density that is peaked
around at-the-money. This, however, is eliminated when using the adjusted
forward volatility
è(K).
5 Calibrating the Volatility function
We first consider the case where we have a continuous curve
of arbitrage-free option prices. This could for example be produced by
Andreasen & Huge interpolation scheme ( [15]) or come from another ZABR
model. We can calculate the forward volatility function by the discrete Dupire
equation:
è(K)2
= 2C(T,
K) - (F -
K)+ (3.45)
T ?2C(T,K)
?K2
Using 3.36, we can calibrate the volatility function:
F (Y, óã-1
0 X)
è(K)
?(K) =
(3.46)
ó0P(X)
?Y
where X and Y are found from 3.35 as the solution to the ODE
system:
ó0 P
(X)
ã-1
?X P(X)
(3.47)
?K =
è(K)
X(K =
F) = Y (K
= F) = 0
The above ODE system can be solved for all strikes in one
sweep. However, typically, we prefer to calibrate directly to the observed
discrete quotes. This is done by solving the ODEs in 3.35 and 3.36 and
including the one-step finite difference adjustment 3.42:
?X ?K =
F (Y, óã-1
0 X)
(3.48)
ó0?(K)
P(X)ó0?(K)
è(K) =
F (Y,
óã-1
0 X)
X(K =
F) = Y (K
= F) = 0
32
5. CALIBRATING THE VOLATILITY FUNCTION
After solving numerically the above system, we can find the
option prices using the one-step finite difference algorithm in 3.44. On top of
this, we can use a nonlinear solver to calibrate the volatility function
ó(K) to observed
discrete option quotes. As we get all option prices in one sweep, we can
include CMS forwards and option quotes in the calibration without additional
computational costs.
CHAPTER 3. THE ZABR MODEL
Even though non-linear iteration is involved, this procedure
is very fast. Typically, we can calibrate a non-parametric volatility function
with 10 knot points to a given smile in roughly 50 iterations, which takes
approximately one millisecond of CPU time.
When it comes to outright pricing speed, the ZABR model is
capable of generating 100'000 smiles, each consisting of 256 strikes in
approximately seven seconds. It should be stressed that this includes both
numerical ODE and finite difference solutions. This is actually faster than
direct use of Hagan's SABR expansion, which takes 10 seconds for the same task.
The reason for this difference is mainly that one time-step finite difference
is faster at producing prices than the Black formula. An alternative to the
ZABR model for producing arbitrage-free options prices is the Fourier-based
models, found in [2] for example. For a displaced Heston model (see [1]),
numerical solution for 100'000 smiles consisting of 256 strikes via the fast
Fourier transform with the Black-Scholes formula used as a control variate
takes around 18 seconds (see [14]). It should be noted that this type of model
is considerably less flexible with respect to fitting discrete quotes and more
difficult to implement.
Though we generally use 3.48 in conjunction with a non-linear
solver for the calibration, the direct calibration methodology 3.47 is relevant
as it admits direct calibration of one ZABR model to another.
The stochastic process (Xt)t
has unit diffusion and thus, in the sense of the short-maturity
limit, is normally distributed. So it is natural to use a uniform spacing in X
and a non-uniform spacing in K. For this, the ODE system 3.48 can conveniently
be transformed to:
?Y
|
óã-1
0
|
?K =
|
F (Y, óã-1
0 X)
|
?K
|
ó0?(K)
|
|
|
|
?X =
(3.49)
F (Y, óã-1
0 X)
P (X)ó0?(K)
è(K) = F (Y,
óã-1
0 X)
Y (X = 0) = 0, K(X = 0) = F
In our implementation, we solve 3.49 on a uniform X grid to
generate and fix a non-uniform strike grid k0, k1, ... , kn
that is used in the numerical solution of 3.48 during
calibration and pricing. As a final remark, we note that ODEs in this section
typically will be solved at ó0 = 1.
Conclusion for the ZABR model
We have used a simple method to derive short-maturity
expansion for forward volatilities from stochastic volatility models. The
solution is an ODE that can be solved numerically for all strikes in one sweep.
Finally, we used a one-step finite difference scheme to generate option prices.
That approach is very fast and it generates arbitrage-free option prices. We
have added flexibility to the original SABR model to get an exact fit of all
quoted option prices and better control of the
5. CALIBRATING THE VOLATILITY FUNCTION 33
34 5. CALIBRATING THE VOLATILITY FUNCTION
CHAPTER 3. THE ZABR MODEL
wings of the smile for improved CMS pricing. Also, we can add
CMS prices to the calibration without additional computational costs.
35
Conclusion
This research internship focused on solving two main problems
encountered with the SABR model in the financial industry:
· an arbitrage problem observed through the negative
density of the underlying,
· a lack of flexibility in wings control
We first developed the Normal SABR model and solved the
negative density problem (Chapter 2). We then solved the wings control problem
by another model, the ZABR model, which is just an extension of the SABR model
where one replaces the (usually) lognormal volatility process by a CEV
volatility process, gaining then a control on the smile's wings through the CEV
exponent 'y (Chapter 3). We remarked that a direct use of computed implied
volatilities for pricing doesn't yield arbitrage-free prices and we finally
used a Markovian Projection and found an equivalent local volatility model that
is rather used for pricing.
For each solved problem, we gain accuracy but we pay back a
computation time, specially for the Normal SABR model. Anyway, the time lost
with the ZABR model is worth the wings control and the arbitrage-free prices
obtained. The ZABR model is therefore usable in pricing libraries without
additional excessive costs.
Beyond the subjects studied in my internship, as suggested
through picture 3.2, one can efficiently hedge against model parameters' risk
by using the 'y parameter to thwart the movements of the other model
parameters. Here, we did it manually but it can be very interesting to look for
particular relationships between 'y and (á, 9, p) in terms of a
parametric function that will be calibrated while readjusting the level of 'y
according to how the other parameters move. We should therefore look for a
stability in the smile shape despite the changes in other parameters of the
model. This can be done by matching the slope and the convexity of the obtained
smiles around the strike. Numerical resolution yields optimization
algorithms.
We can therefore look forward to finding closed form formulas
in the ZABR model scope. My idea is to rely on the Labordere's heat kernel
expansion on a Riemann manifold; and the research continues...
CONCLUSION
36 CONCLUSION
Appendix A
Numerical pricing under Normal
SABR model
1 Density for Normal SABR
We approximate the density of (Xt)t
at zero, that is, EQu
[ä(Xt)]. As previously explained, the process
(Xt)t satisfies:
V
dWt = b0 q(Xt)dW u t?ô
= X0 = F0 - K
where q(X) =
1-2ñáX
+á2X2,
á-á/b0,
(Wtu)t is a zero-drift Brownian motion
under Qu, and ô is the first time X hits -K.
This is achieved by defining the following process:
Xt
(I(X)) du = 1ln
(V(Xt) - ñ + áX\
t -- 10Vq(u)
á 1 - ñ
1 (1 +
ñ)2e2áI + (1 -
ñ)2e2áI + 2(1 -
ñ2)) q(X) = g(I)
- 4
The process It - I(Xt) admits the
following dynamic:
V b2 01{t<ô}dt
1 + á2X2 t -
2ñáXt
1
dIt = b0dWt?ô - 2
áXt -
ñá
37
We define the process At =
q(Xt)1/4q(X0)1/4
and observe that:
~ ~
2
dlnAt = dlnñt + -1 + 31 -
ñ á2bo1{t<ô}dt
8 8 q(Xt)
dñt 1
=
ñt 2
áXt -
ñá
V1 +
á2X2t -
2ñáXt
b0dWt?ô
The martingale (ñt)t defines
a new measure Qñ and we have:
dIt = b0dW Qñ
t?ô
APPENDIX A. NUMERICAL PRICING UNDER NORMAL SABR MODEL
where (W Qñ
t )t is a Brownian motion under Qñ.
We observe that:
EQu [ä(Xt)] = q(X0)1/4EQu
[Atä(Xt)] = q(X0)1/4Ë(t)EQñ
[ä(Xt)]
[exp (-8 á2b20(t t?ô 11= EQñ ?
ô) + 3á2b20(1 -82)
o du g(Iu)du)) /It = 0J
Ignoring the stopping time in above expression for Ë(t)
and using áb0 = á, we derive:
Ë(t) e- a á2t × Ö(t, I0
b0
(:á2(1
1z) = EQñ [exp - ñ2) t
f(W?)) /WQñ = zJ
1 f(W) = 4 ((1 + ñ)2e-2áW +
(1 - ñ)2e2áX + 2(1 -
ñ2))
where (W Qñ
t )t is a Qñ-Brownian motion with initial value zero.
Since Ö(t, z) depends exclusively on ñ and
á, this function can be pre-calculated or alternatively approximated as
follows:
Ö(t, z) = exp [á2(1 - ñ2) (f(0) +
f(z) / t] + O(t2)
We define ê(t, z) = -18á2
+ 136á2(1 - ñ2)
(f(0) + f(z)) + O(t)
Since EQñ [ä(It)] = EQñ
[ä(Xt)], we finally derive using the reflection principle for
Brownian motions:
EQu [ä(Xt)] = q(X0)1/4
b0 v2ðt
I(F0 - K)
B=
2I(Fmin - K) - I(F0 - K)
v2 , C = v2
B2 - C2
× ef0 ê(s,b00)ds × [eTht - e bit
38 2. COMPUTATION OF FUNCTIONS Ö AND ê
2 Computation of functions Ö and ê
We propose a simple algorithm to calculate the functions Ö
and ê. Let's choose a time grid {Ti : i = 0, ... , N} such that T0 = 0,
TN = T and we simplify the computation of Ö(Ti, B) with the following
approximation:
IE [exp
3 Tz dul l(z)
(8á2(1 - ñ2) Jo ?(Wu)/ I WT% =
zJ
Then, we calculate Øi : î 7? Öi (îvTi) on
a set of symmetric Hermite nodes that appear in the Gauss-Hermite integration.
One chooses M = 2m for a symmetric set and {îk : k = 1, ..., M} such
that:
APPENDIX A. NUMERICAL PRICING UNDER NORMAL SABR MODEL
E [f(î)] = XM pkf(îk).
k=1
We can calculate Øi by forward induction:
Øi(îk) E [Øi-1(æTi-1) exp
(ëÄTi 1 J /æTi = îkJ exp (1ÄTi 1
40(si-1æTi-1)/ \ ~(siîk)
si =pTi ë =
16á2(1 - ñ2), æt
=NAWt.
The conditional expectation can be analytically computed since
æTi-1 and æTi are unit normal variables with correlation
ñi =q Ti .
Let's consider the following function:
Fi-1(z) = Øi-1(z) exp ~
ëÄTi 1

?(si-1z)
Then we have:
~ Øi(îk) = E Fi-1(ñiîk + q1 - 401
exp(ëÄTi(1))
Using the decomposition of Fi-1(z) in its basis cubic spline
functions, we can write:
Fi-1(z) = XM Fi-1(îj)èj(z). j=1
By integrating the above, we can simplify the former equation as
follows:
Øi(îk) =
|
XM j=1
|
~ tt ëÄT ëÄTi
pkj x Øi-1(S9) x exp itt +
?(si-1sj) ?(siîk))
|
ci(z) = A1i (z - îi)3 + B1i (z -îi) - A0i
(z - îi+1)3 - B0i (z - îi+1) Lil0 = îi, L0 = -50,
Ui6=M = îi+1, UM = 50.
2. COMPUTATION OF FUNCTIONS Ö AND ê 39
~ ~ q ~~
pkj = pkj(ñi) = E
(i) èj ñiîk + 1 - ñ2 i
æ
where p(i)
kj satisfies Pj p(i)
kj = 1 but can be negative.
A crucial observation for time saving is the fact that the
pseudo-transition proba-
bilities pkj only depends on the mesh îk and the grid Ti.
Consequently, the respective
expectations only need to be computed once.
We can analytically calculate the pseudo-transition
probabilities. The function
èj is a cubic spline with value zero at every node except
at z = îj where it takes
value 1.
èj(z) = XM 1{Li<z<Ui}ci(z),
i=1
APPENDIX A. NUMERICAL PRICING UNDER NORMAL SABR MODEL
where A0i, B0i, A1i, B1i are calculated using the standard cubic
spline algorithm. We finally compute the pseudo-transition probabilities:
pkj(ñ) = XM
ñ3? [A1iI3(li, ui, vi) -
A0iI3(li, ui, vi+1)] + ñ?
[B1iI1(li, ui, vi) - B0iI1(li,
ui, vi+1)] , i=0
Li - ñîk
li = (1 - ñ2)1/2, ui =
|
Ui - ñîk
(1 - ñ2)1/2, vi =
|
ñîk - îi
p
(1 - ñ2)1/2, ñ? = 1 -
ñ2
|
Where In(a, b, c) = E
[1{a<î<b}(î + c)n]. By
integration, we obtain:
I1(a, b, c) = c [N (b) -
N(a)] + fz(a) -
fz(b)
I3(a, b, c) = (c2 +
3c)[N(b) - N(a)] + [3c(c
+ a) + a2 + 2]
fz(a) - [3c(c + b)
+ b2 + 2] fz(b)
where fZ(.) is the Gaussian density.
40 2. COMPUTATION OF FUNCTIONS Ö AND ê
41
Appendix B
Equivalence between Normal and
Log-normal Implied Volatility
Asymptotics of implied volatility are important for different
reasons. On the one hand, they give information on the behaviour of the
underlying through the moment formula [29] or the tail-wing formula [30]. On
the other hand, they allow a full correspondence between vanilla prices and
implied volatilities. With such a correspondence, asymptotics in call prices
can be easily transformed into asymptotics in implied volatilities. When
applied to a specific model, asymptotics are widely used as smile generators
[26]. In practice, other models are then used for pricing options using tools
like Monte-Carlo simulations.
So far, all the asymptotics studied by authors concern
asymptotics for implied lognormal volatility. In this chapter, we consider
implied normal volatility which refers to the Bachelier model. Why is it
interesting to consider normal implied volatility? One the one hand, for short
maturities, the Bachelier process makes more sense than the Black-Scholes
model. Indeed, the behaviour of the underlying from one day to another is
generally well approximated by a Gaussian random variable [32]. That's the
reason why the Bachelier model is very popular in high frequency trading [21].
On the second hand, the "breakeven move" of a delta-hedged option is easily
interpreted as normal volatility. Generally, the P&L of a book of
delta-hedged option is positive if the (historical) volatility of the
underlying is greater than a breakeven volatility which has to be expressed in
normal volatility. Moreover, it makes more sense to compare implied normal
volatilities with historical moves of the underlying as can be done by a market
risk department. Likewise, some markets such as fixed-income markets with
products like spread-options are quoted in terms of implied normal volatility
[16]. Finally, the skewness of swaption prices is much reduced if priced in
terms of normal volatility instead of lognormal volatility. Therefore, it is
important to have a robust and quick way to compute implied normal volatilities
from market prices and also to be able to switch between lognormal volatilities
and normal volatilities.
What kind of asymptotics should we consider? Most of the
approximations in option pricing theory are made under the assumption that the
maturity is either small (see the Hagan et al. formula [26]) or large
[17]; it is actually assumed that
42 1. ANOTHER PRICING FORMULA FOR CALL OPTIONS IN THE
BACHELIER MODEL
APPENDIX B. EQUIVALENCE BETWEEN NORMAL AND LOG-NORMAL
IMPLIED VOLATILITY
a certain time-variance U2T is either
small or large. A possible way to derive such approximations is to replace the
factor of volatility U by EU and then set E = 1.
This can be done at the partial differential equation level (see all the
techniques coming from physics [26]) as well as directly at the stochastic
differential equation level with the help of the Wiener chaos theory for
instance [33]. Other types of asymptotics are obtained by considering large
strikes. In our approach, we unify all those types of asymptotics (see [18] and
[9] for the lognormal case). Indeed, we obtain an approximation of the implied
normal volatility as an asymptotic expansion in a parameter À
for À « 1 and it turns out that
À -+ 0 when T -+ 0
or K -+ +00.
This study is organized as follows. We first give another
expression for the pricing of a European call option which involves an
incomplete Gamma function (Proposition 1.1). Then, we inverse this function
asymptotically and obtain an expansion of normal implied volatility. This is
particularly important if we want to quickly obtain the implied normal
volatilities from call prices as is the case in high frequency trading [21].
The formula is also potentially useful theoretically if, given an approximation
for the price of a European call option or a spread option (for instance in the
framework of the Heston or the SABR model), we want to obtain an approximation
of the normal implied volatility. Finally, we restrict our formula to the order
0 and we compare it to a similar formula for the lognormal case. Then, we
obtain an equivalence between normal volatility and lognormal volatility. We
use it also to compare the Black-Scholes greeks to the Bachelier greeks.
Finally, we consider a delta-hedged portfolio and we compute the breakeven move
in the normal case as well as in the lognormal case.
1 Another pricing formula for call options in the
Bachelier model
In the Bachelier model, the dynamic of a stock
(St)t?R+ is given by:
( dSt = UNdWt,
(B.1) S0 = S
The so-called normal volatility UN is related to the
price of a call C(T, K) struck at K with maturity
T by the following formula:
(S - K ) (S - K
)
\/
C(T, K) = (S - K)
\/ + UN T fz
\/ ,
UN T UN T
(B.2)
( ) Z x
1 -x2
with fz(x) =
\/2ð exp
and (x) = fZ(u)du.
2 -8
Following Ropper-Rutkowski [23], we can isolate the volatility
UN in the pricing formula.
APPENDIX B. EQUIVALENCE BETWEEN NORMAL AND LOG-NORMAL IMPLIED
VOLATILITY
Definition 1.1. Let us denote by TV (K,T) (or simply TV ) the
time-value of a European call option struck at strike K with maturity T. Then
TV (T, K) := C(T, K) - (S - K)+ .
Proposition 1.1. In the Bachelier model, we
TV (T, K) =
|
{
|
1 (S-K)2
if K =6S,
4vð 2,
2ó2NT
v
óN v2ð otherwise
T
|
(B.3)
|
where (a, z) is the incomplete Gamma function:

(a, z) =
+8
ua-1 exp(-u)du
f
v
Proof. We have C(T,K)
S = f(î, è) with î := K S and è :=
óN S and
T
f(î,u) := (1 - î)N \1 - î /u + ufz \1
u
By differentiation, we have:
?u(î,u)=-(1u2î)2fz \1u/+- îfzu-
îu 1 --
2
u21
/ \ u/fz\1u/
fz
\1 -î/
u
where we have used fz(î) =
-îfz(î). Since f(î, 0) = C(0,K)
S = (1 -î)+ , we
deduce that:
f(î,è) = (1 -î)+ + fB /1
u
fz ( I du
o \ If we set
F(î, è) :=fB fz ( \1 u du
o
then we have:
C(T, K)
S
|
Kv !
= (1 - î)+ + F S , ó T
S
|
Let's assume that both è =6 0 and î =6 1. With the
change of variable v := 1-î
u ,
we get:
+8
F(î,è) = |1 -î| f1 î|
è
|
fz(v) dv v2
|
So, with a new change of variable u :=
12v2, we have:
1. ANOTHER PRICING FORMULA FOR CALL OPTIONS IN THE BACHELIER
MODEL 43
APPENDIX B. EQUIVALENCE BETWEEN NORMAL AND LOG-NORMAL IMPLIED
VOLATILITY
Z +8
1
F(î, è) = 4vð |1 - î|
~2
2è
|
u-
|
3
2 exp(-u)du
|
4vð1 |1 -
î|(-12,|12è2|2)
v2ð
where (a, z) is the incomplete Gamma function. At the money, we
simply have C(T, K) = óNvT
It's clear from Proposition 1.1 that the real-valued function
T 7? C(T, K) is non-decreasing, positive, C(0, K) = (S - K)+ and
limT?+8 C(T, K) = +8. So, given the price of a European
call option C, there is a unique real number óN(T, K) such that C(T, K)
= C with a normal volatility óN = óN(T, K). We say that
óN(T, K) is the normal implied volatility
Remark 1.1. We can easily see that |Ks|
depends only on arKT (only one variable).
One of the interests of Proposition 1.1 is that there are
efficient algorithms to compute the inverse of the incomplete Gamma function.
In particular, it is implemented in Matlab. Therefore, it is always easy to get
the implied normal volatility from call prices [22]. Such a task is not always
easy in the lognormal case [28], especially when we are far from the money.
Corollary 1.1. Let p be an integer. Then,
(ó
TV T K) = NT) 2 ex _(S - K)2 p-1 1 k
(2k + 1)! óNT k + R
TV( v2ð(S - K)2 p (
2ó2NT ) ( ) k! ((S -
K)2) p
k=0
with |Rp| = (2p + 1)!
01110111P
p! ( (S - K)2 )
(B.4)
The above equation comes naturally from a well known
asymptotic expansion of (a, z) for large z (see Formula 6.5.32 in [20]).
Remark 1.2. From either pricing formula B.2 or B.3, we can
notice that we can use the same trick to price large strike and short maturity
European options (as expansions in both cases are similar)... This comes from
the fact that:
C(ë2T, ëS + (1 - ë)K) =
ëC(T, K) for any non-negative real ë. This is particular to the
Bachelier model.
For a comparison with the lognormal case, it can be
advantageous to introduce the following notations.
44 1. ANOTHER PRICING FORMULA FOR CALL OPTIONS IN THE BACHELIER
MODEL
APPENDIX B. EQUIVALENCE BETWEEN NORMAL AND LOG-NORMAL IMPLIED
VOLATILITY
Definition 1.2. For K 6=, we set èN := óN s
T xN := -1, ãN := log (4vð),
uN :=
|xN |
2è2 - 1
log(TV (T,K)
N
S ).
x
Then by Corollary 1.1, for K =6 S and p ? N*,
4vð
|
|
TV (T, K)
|
= u
|
3/2 e N
|
1 uLN
|
X p-1 k=0
|
(1)k k k 2k aLNuLN + RpLN
|
|xN|
|
|
S
|
(B.5)
(B.6)
~
2 /
with uLN := ~LN, èLN := óLNV T,
xLN := log C S , RpLN ? Ù (èx) ,
xLN
1 x2 i
LN and(2k + 1)!! := 11(2j + 1).
j!(2j + 1)!! 8
aLkN := (2k + 1)!! Xn
k=0
j=0
Here, óLN denotes the lognormal implied volatility.
2 Asymptotics of the implied normal volatility 2.1 First and
Second order expansion
-
3/2
1 uN
u
X p-1 k=0
N e
Let us assume that K =6 S. Using B.5, we get:
ukuN + O (uN))
= eãNe-1/ë
Therefore, by Lemma 1 of [9], we get the following
proposition.
Proposition 2.1. Let us denote by TV the time-value of a
European call option, óN its implied normal volatility and T the
maturity of the option. Set ë := - 1
log(T SV ),
ãN := log
(4|x,z)
and xN = KS - 1. Let's assume that K =6S. Then, in the case
when T ? 0, we have the following expansion for the time-variance of the call
option: ó2NT = (S-K)2
2 uN with
3_2_29_3_2/9
_ \ 3 log(A)
+ (ã2N
-32ãN + 2) A3 + o(ë3)
(B.7)
In the lognormal case [9], for short expiries, the asymptotic
expansion of ó2LNT is
2. ASYMPTOTICS OF THE IMPLIED NORMAL VOLATILITY 45
APPENDIX B. EQUIVALENCE BETWEEN NORMAL AND LOG-NORMAL IMPLIED
VOLATILITY
given by ó2LNT = log2(K/S)
2 uLN with
99
uLN =ë - 2ë2log(ë)
+ãLNë2 +
4ë3log2(ë) + 14 - 3ãLN I
ë3log(ë)
(N - ë3 + o(ë3)
+ 2ãLN - u1 (B.8)
LN := log
XLN 2
-
ã
4vðex 2 and ui:= - LN| 16 2
First, we note that ë = uLN + o(uLN). Then comparing the two
results B.7 and B.8 for K =6 S, we obtain:
uN = uLN + (ãN - ãLN)u2LN + O (u3LN
log(uLN))
So,
~SxN 2
ó2 LNT + O ~T2 log(T)~
(B.9)
N = ó2LN + 2(ãN -
ãLN)S2x2 Nó4
xLN x4 LN
Since xN
xLN
|
S-K
= log(S)-log(K) > 0, we deduce that
|
~ 1/2
SxN
óN =
xLN
SxN
=
xLN
1 + 2(ãN - ãLN)ó2 LN + O
~T2 log(T)~
óLN T
x2 LN (B.10)
2 1/2
ó
ã
-ã
ó
x

LN (1 + (
N
LN)
2NT) + O (T2 log(T))
LN
46 2. ASYMPTOTICS OF THE IMPLIED NORMAL VOLATILITY
Moreover, we have:
Hence, we get:
K
|
ãN - ãLN =
|
~xLN ~
xLN
2 + log xN
|
S
? ~S-K ~ ?
-
log ,/KS(log S-log K) ?+ O ~T 2
log(T)~
óN = log(S) - log(K)óLN ?1 - (log S -
log K)2 ó2 LNT
Note also that at the money, the situation is quite easy. On the
one hand, we have (cf. Proposition 1.1)
r
2ð
óN = T C
On the other hand, we have ( [9], Proposition 2.1):
v !
óLN T
C = erf 2v2
Therefore, we state the following result.
APPENDIX B. EQUIVALENCE BETWEEN NORMAL AND LOG-NORMAL IMPLIED
VOLATILITY
Corollary 2.1.
· if K =6 S, we
have:
UN =
|
2 S-K
S - KULN 1 - log KS(log
S-log K) U2 T + O (T2
log(T)
log S - log K (log S - log
K)2 LN
|
(B.11)
In particular, UN ~
S-K
log S-log KULN when T ? 0.
· At the money, we have UN =
/2ðerf óLNT and UN
SULN 1 aLNT
2v2=- +
24
o(T).
In particular, UN ~ SULN when T ?
0.
Remark 2.1. When K ? S, we can check that
In other terms, for K =6 S,
|
S-K
log vKS(log S-log K) 1
(log S-log K)2 24
|
|
ULN =
S(m m1) UN, where
m = S = xN + 1 is the Moneyness. (B.12) This
formula was known (even if it was not stated explicitly) in the SABR model (see
Hagan et al. formula [26] ). By differentiatinf Formula B.12 with
respect to m, it turns out that the Black-Scholes skew ?óLN
?m at the money (m = 1) generated by
the Bachelier model is ?óLN
?m = -1 óN S (ULN is by
definition the implied lognormal 2
volatility). Therefore, the Bachelier model is highly skewed
ATM (a slope of -50%× óNS ). Another way to explain this
feature is that given call prices, when we use the BS model, the function
ULN is a decreasing and convex function of m, i.e., it generates a
skew, while the function UN is a rather flat function of m. Thus,
normal volatility is most suited for products such as swaptions for
instance.
2.2 Accuracy of asymptotic expansions
Here we address the question of the accuracy of our
expansions. Therefore, we proceed by backward induction of the lognormal
implied volatility as described bellow:
· Let's choose several random lognormal implied
volatility and compute a Call price through Black & Scholes formula without
interest rate
· From each Call price, we calibrate the volatility
parameter from a Bachelier model by numerical inversion of the pricing formula.
We therefore obtain the likely "true" normal implied volatilities equivalent to
our initial lognormal volatilities
· For comparison, we respectively apply the first and
second order approximations with our initial lognormal implied volatilities and
we obtain other normal volatilities that we can compare with the former ones
Applying the above process leads to the following curve where
we have plotted comparison curves for different maturities.
The first order approximation supposes an affine relationship
between normal and lognormal implied volatilities whereas the second order
supposes a parabolic one. We can observe that the raise of the maturity
deteriorates our asymptotic expansions. The more we increase the option's
expiry, the more our approximation is credible.
2. ASYMPTOTICS OF THE IMPLIED NORMAL VOLATILITY 47
48 2. ASYMPTOTICS OF THE IMPLIED NORMAL VOLATILITY
APPENDIX B. EQUIVALENCE BETWEEN NORMAL AND LOG-NORMAL IMPLIED
VOLATILITY
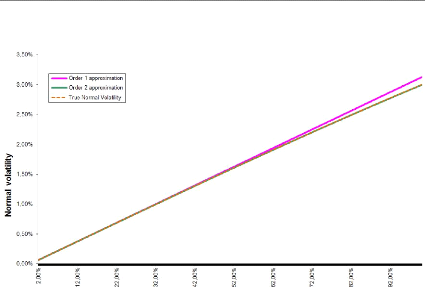
- Order 1 approximation
- Order 2 approximation --- True Normal Volatility
2,50% -
2,90% -
ô ô
V
3,50% -
3,00% -
Lognormal volatility
Figure B.1: T = 1, F0 = 0.0325, K =
0.03
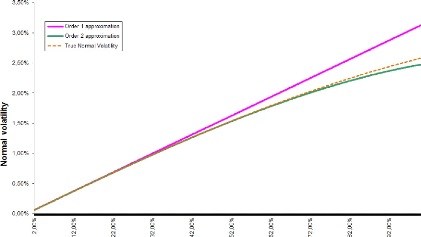
- Order 1 approximation
- Order 2 approximation --- True Normal Volatility
3,09%
3,50%
2,50%
2,99%
0,59%
0,0C%
V V V
ô ô ô ô ô
N N N N
V
Lognormal volatility
Figure B.2: T = 1, F0 = 0.0325, K =
0.03
APPENDIX B. EQUIVALENCE BETWEEN NORMAL AND LOG-NORMAL IMPLIED
VOLATILITY
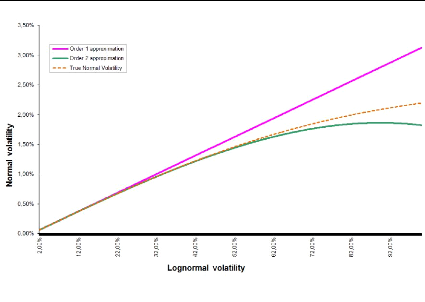
Figure B.3: T = 1, F0 = 0.0325, K
= 0.03
3 Comparing greeks and delta-hedged portfolios
Let's denote by ON, FN, íN,
ÈN (resp. OLN, FLN, íLN,
ÈLN), the delta, gamma,
vega and theta in the Bachelier (resp. Black-Scholes) model. For
instance, íN = ?C
?óN .
By differentiating B.2, we get:
CS - K)
ON = N (B.13)
óN vT
On the other hand, it is known that:
OLN =
NClnS - lnK 1 1
+ (B.14)
óLNv T 2ULN T)
So, by Corollary 2.1, we get: OLN ~ ON for a
maturity T « 1. By differentiating B.13, we obtain:
ü fz CS )
(B.15)
FN =
óN T óN T
In the Black-Scholes model, we have:
= 1 ClnS - lnK 1 )
FLN SóLNvT fz óLNvT +
2óLNvT (B.16)
Hence, with the help of Corollary 2.1,
lnS - lnK
FN ~ S - K FLN (B.17)
Now we consider the Vega. It is shown above that:
3. COMPARING GREEKS AND DELTA-HEDGED PORTFOLIOS 49
APPENDIX B. EQUIVALENCE BETWEEN NORMAL AND LOG-NORMAL IMPLIED
VOLATILITY
C(T, K) = (S - K)+ + S
0
|
v óN T S
|
f(1 - K/S ) z u du
|
So, differentiating w.r.t. óN, we get:
/ (S - K)2
íN T = S 2ð 2T 2~2 T
) (B.18)
N
In contrast, the vega in the Black-Scholes model id
/T ((lnS - lnK
+2óTNT)2)
íLN = S 2ð exp (B.19)
2ó2 LNT
Likewise, we can compare the two thetas. We then have the
following proposition
Proposition 3.1. When T ? 0, and under the hypothesis of
bounded volatilities, we have:
ÄN ~ ÄLN íN ~
íLN
N S lnS - lnK
LN
~ S - K
S - K
ÈN ~ ÈLN S(lnS -
lnK)ÈLN
|
(B.20)
|
The first equivalence ÄN ~ ÄLN
shows that hedging in the Bachelier framework is more or less like hedging
in a Black-Scholes framework. However, the "breakeven move" of a delta-hedged
portfolio is not the same. By definition, the "breakeven move" of a
delta-hedged portfolio is the number u such that over a short horizon ät,
P&L > 0 if the change in S is > u. In general, we have:
(ÄS)2
1
=
2
1
P &L = -Èät + 2
[(ÄS)2 - u2]
(B.21)
So, with ät = 1,
m - 1uN (B.23)
lnm
uLN =
with m = K/S. So, at the money, uLN ~ uN.
However, if K < S (resp. K > S)
then uLN < uN (rep. uN >
uLN.
50
3. COMPARING GREEKS AND DELTA-HEDGED PORTFOLIOS
r
2È
u = .(B.22)
Using Proposition 3.1, we find that the "breakeven move" uLN
in the Black-Scholes model is related with the "breakeven move" uN
in the Bachelier model by:
3. COMPARING GREEKS AND DELTA-HEDGED PORTFOLIOS
51
APPENDIX B. EQUIVALENCE BETWEEN NORMAL AND LOG-NORMAL
IMPLIED VOLATILITY
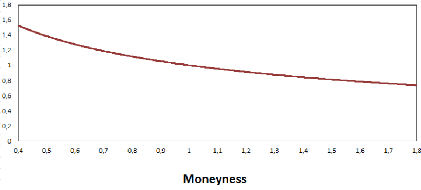
Figure B.4: The volatility smile of the Bachelier model
(normalized by the factor óN
S where óN
denotes the normal volatility
and the ratio of "breakeven moves"
uLN/uN.
The following graph represents the function in
7?
ln(m)
m-1 which gives the smile
of the Bachelier model (cf Corollary 2.1) as well as the ratio of
"breakeven moves" uLN .
uN
So, depending on the view of the trader on the short term dynamic
of the underlying (normal or lognormal diffusion), he will adjust or not the
"breakeven move"
of his delta-hedged portfolio by the factor lnm
m-1.
APPENDIX B. EQUIVALENCE BETWEEN NORMAL AND LOG-NORMAL IMPLIED
VOLATILITY
52 3. COMPARING GREEKS AND DELTA-HEDGED PORTFOLIOS
53
Bibliography
[1] Lipton A. A closed-form solution for options with
stochastic volatility with applications to bond and currency options.
Review of Financial Studies, 6, 1993.
[2] Lipton A. The vol smile problem. Risk February,
2002.
[3] Lipton A. and A. Sepp. The vol smile problem. Risk
February, 2011.
[4] Jesper Andreasen and Brian Huge. Expanded forward
volatility. Risk Magazine, 2013.
[5] Dupire B. Pricing with a smile. Risk January,
1994.
[6] Dupire B. A unified theory of volatility. Working
paper, BNP Paribas, 1996.
[7] Philippe Balland and Quan Tran. Sabr goes normal.
Risk Magazine, 2013.
[8] Eric Benhamou and Olivier Croissant. Local time for the
sabr model: Connection with complex black scholes and application to cms and
spread options. Working Paper, 2007.
[9] Grunspan C. Asymptotic expansions of the lognormal
implied volatility: A model free approach. Preprint, 2011.
[10] E. Derman and I. Kani. Stochastic implied trees:
Arbitrage pricing with stochastic term and strike structure of volatility.
Int J. Theoritical and Applied Finance, 1998.
[11] B. Dupire, M.A. H. Dempster, and S. R. Pliska. Pricing
and hedging with smiles, in mathematics of derivative securities. Cambridge
University Press, 1997.
[12] Robert Goldstein and William Keirstead. On the term
structure of interest rates in the presence of reflecting and absorbing
boundaries. Working Paper, 1997.
[13] Gyongy I. Mimicking the one-dimensional marginal
distributions of processes having an itô differential. Probability
Theory and Related Fields, 71, 1986.
[14] Andreasen J. and L. Andersen. Volatile volatilities.
Risk December, 2002.
[15] Andreasen J. and B. Huge. Volatility interpolation.
Risk March, 2011.
54 BIBLIOGRAPHY
BIBLIOGRAPHY
[16] Kienitz J. and Wittke M. Option valuation in
multivariate sabr models - with an application to the cms spread. Preprint,
2010.
[17] Fouque JP., Papanicolaou G., Sircar R., and Solma K.
Singular perturbations in option pricing. SIAM Journal on Applied
Mathematics, 63(5), 2003.
[18] Gao K. and Lee R. Asymptotics of implied volatility to
arbitrary order. Preprint, 2011.
[19] Andersen L. and R. Ratcliffe. Extended libor market
models with stochastic volatility. Working paper, General Re Financial
Products, 2002.
[20] Abramowitz M. and Stegun IA. Handbook of mathematical
functions. Dover, 1966.
[21] Avellaneda M. and Stoikov S. High-frequency trading in a
limiot order book. Quantitative Finance, 8(3), 2008.
[22] Li M. and Lee K. An adaptative successive
over-relaxation method for computing the black-scholes implied volatility.
Quantitative Finance, 2009.
[23] Roper M. and Rutkowski M. A note on the behaviour of the
black-scholes implied volatility close to expiry. International Journal of
Theoretical and Applied Finance, 12(4), 2009.
[24] Balland P. Forward smile. Presentation, ICBI Global
Derivatives, 2006.
[25] Doust P. No arbitrage sabr. Working paper, Royal
Bank of Scotland, 2010.
[26] Hagan P., Kumar D., Lesniewski A., and Woodward D.
Managing smile risk. Wilmott Journal, pages 84 108, Jul 2002.
[27] Henry-Labordere P. Analysis, geometry and modeling in
finance. Chapman and Hall, 2008.
[28] Jaeckel P. By implication. Wilmott Journal, nov
2006.
[29] Lee R. The moment formula for implied volatility at
extreme strikes. Mathematical Finance, 14(3), Jun 2004.
[30] Benaim S. and Friz P. Smile asymptotics ii: models with
known moment generating functions. Journal of Applied Probability,
45(1):16 32, 2008.
[31] Press W., B. Flannery, S. Teukolsky, and W. Vetterling.
Numerical recipes in c. Cambridge University Press, 1992.
[32] Schachermayer W. and Teichmann J. How close are the
option pricing formulas of bachelier and black-merton-scholes? Mathematical
Finance, 18(1):155 170, 2008.
[33] Osajima Y. General asymptotics of wiener functionals and
application to mathematical finance. Preprint, 2007.
BIBLIOGRAPHY 55
BIBLIOGRAPHY
[34] Osajima Yasufumi. The asymptotic expansion formula of
implied volatility for dynamic sabr model and fx hybrid model. Mitshubishi
UFJ Securities Co., 2007.
[35] Osajima Yasufumi. General short-rate analytics. Risk
Magazine, 2011.