|
INTERNATIONAL COURSE PROGRAMME MASTER IN
Physical Land Resources
|
|
|
|
Ghent University
Free University of Brussels
Belgium
Forest degradation, a methodological approach using
remote sensing techniques: Literature Review
Jean-fiston MIKWA
Promotor: Prof. Dr. Rudi Goossens
Academic Year 2010 - 2011
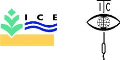
ii
Table of contents
0. Introduction 1
1. Remote Sensing, an Overview 2
1.1. Definitions 2
1.1.1. Analog remote sensing 2
1.1.2. Digital Remote Sensing 2
1.2. Digital image analysis 5
1.2.1. Image Acquisition/Selection 5
1.2.2. Pre-processing 5
1.2.3. Classification 5
1.2.3.2. Combined Approaches. 6
1.2.3.3. Advanced Approaches. 6
1.2.3.4. Object-Based Approaches ( polygon approach) 6
1.2.4. Post-processing 7
1.2.5. Accuracy Assessment 7
1.3. Digital Image Types 7
1.3.1. Multispectral Imagery 7
1.3.2. Hyperspectral Imagery 8
1.3.3. Digital Camera Imagery 8
1.3.4. Other Imagery 8
2. Forest Degradation 8
2.1. Key concepts to forest degradation 8
2.2. Main causes of forest degradation 9
3. Mapping forest degradation 10
3.1. Remote sensing in forest degradation 10
3.2. Forest change detection analysis 11
3.4. Indirect methods of forest degradation mapping 13
3.5. Relevancy of different forest degradation approach 14
3.6. The use of vegetation indices as NDVI concept to assess
forest degradation 15
3.7. Forest canopy change and remote sensing 16
3.8. Comparing Forest Inventory and Remote Sensing Measurement
for forest degradation
mapping 17
3.9. Estimating Forest Volume Using Remote Sensing 17
3.10. Estimating forest biomass using remote sensing 18
3.11. Estimating Forest Carbon Stocks from Remotely Sensed Data
18
4. Conclusion 19
5. References 20
1
0. Introduction
Forest degradation is a serious problem, environmentally,
socially and economically particularly in developing countries. It is estimated
that as much as 850 million hectares (ITTO, 2002) of forests and forest lands
are degraded. Yet it is difficult to quantify the scale of the problem since at
national and sub-national levels forest degradation is perceived differently by
the various stakeholders who have different objectives.
Forest degradation has adverse impacts on forest ecosystems
and on the goods and services they provide. Many of these goods and services
are linked to human well-being and some to the global carbon cycle and thus to
life on Earth.
Policy makers and forest managers need information on forest
degradation. They need to be able to monitor changes happening in forests. They
need to know where forest degradation is taking place, what causes it and how
serious the impacts are in order to prioritize the allocation of scarce human
and financial resources to the prevention of degradation and to the restoration
and rehabilitation of degraded forests. (Simula , 2009).
In addition, reviewing on forest degradation is required to
demonstrate efforts to tackle the problem and meet global objectives and
targets. The proposed new Biodiversity Target includes a target on reduction of
forest degradation. The agreement to establish a mechanism under the UNFCCC
aimed at reducing emissions from deforestation and forest degradation (REDD) in
developing countries has added a political dimension and the potential
availability of substantial funds to reward developing countries that manage to
reduce the level of forest degradation.
Accurate and up-to-date land use/cover assessments are
important to define natural resource management strategies and policies for
conservation especially in forest areas. Understanding the causes and
consequences of land cover change and their cascading effects on many
components of functional ecosystems, are the case for identifying negative
effects on biological resources and human development ( Bicheron et al,2008;
Bunker et al, 2005).
Satellite remote sensing provides a meaningful method for
detecting vegetation or land cover changes (Smith et al, 2004). Changes in the
composition and spatial distribution of forest cover are a major environmental
concern, affecting many biological, biochemical and ecological processes.
Remotely sensed data are widely used to understand and manage environmental
resources by determining land cover/use changes such as quantification of
forest degradation. By comparing the images taken in different times, the
changes in landscape level can be easily detected. Monitoring land cover and
land cover change at regional and global scales often requires sensors data to
identify and map landscape features and patterns with sufficient detail (
2
Defries et al., 2001).Detailed and updated resource
inventories are needed to support land use planning and sustainable management
.
This literature review addresses how remote sensing techniques
can be used to assess forest degradation directly or indirectly by mean of
different type of degradation process occurring in the forest area.
1. Remote Sensing, an Overview
1.1. Definitions
Remote sensing can be defined as learning something about an
object without touching it. As human beings, we remotely sense objects with a
number of our senses including our eyes, noses, and ears. ( Cogalton,2010); for
Thomas et al.(2004) ,remote sensing is the science and art of obtaining
information about an object, area, or phenomenon through the analysis of data
acquired by a device that is not in contact with the object, area or phenomenon
under investigation.
The field of remote sensing can be divided into two general
categories: analog remote sensing and digital remote sensing. Analog remote
sensing uses film to record the electromagnetic energy. Digital remote sensing
uses some type of sensor to convert the electromagnetic energy into numbers
that can be recorded as bits and bytes on a computer and then displayed on a
monitor.
1.1.1. Analog remote sensing
The field of analog remote sensing can be divided into two
general categories: photointerpretation and photogrammetry. Photo
interpretation is the qualitative or artistic component of analog remote
sensing. Photogrammetry is the science, measurements, and the more quantitative
component of analog remote sensing. Both components are important in the
understanding of analog remote sensing
1.1.2. Digital Remote Sensing
While analog remote sensing has a long history and tradition,
the use of digital remote sensing is relatively new and was built on many of
the concepts and skills used in analog remote sensing. Digital remote sensing
effectively began with the launch of the first Landsat satellite in 1972. Since
the launch of Landsat 1, there have been tremendous strides in the development
of not only other multispectral scanner systems, but also hyperspectral and
digital camera systems. However, regardless of the digital sensor there are a
number of key factors to consider that are common to all. For Campbell ,(2007)
these factors include:
3
- Spectral Resolution
Spectral resolution is typically defined as the number of
portions of the electromagnetic spectrum that are sensed by the remote sensing
device. These portions are referred to as «bands.»A second factor
that is important in spectral resolution is the width of the bands.
Traditionally, the band widths have been quite wide in multispectral imagery,
often covering an entire color (e.g., the red or the blue portions) of the
spectrum. If the remote sensing device captures only one band of imagery, it is
called a panchromatic sensor and the resulting images will be black and white,
regardless of the portion of the spectrum sensed. More recent hyperspectral
imagery tends to have much narrower band widths with several to many bands
within a single color of the spectrum.
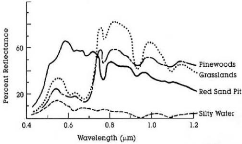
Figure 1 comparison of spectrums of vegetation,bare soil,snow and
water, in Asner et al,2004 - Spatial Resolution
Spatial resolution is defined by the pixel size of the
imagery. A pixel or picture element is the smallest two-dimensional area sensed
by the remote sensing device. An image is made up of a matrix of pixels. The
digital remote sensing device records a spectral response for each wavelength
of electromagnetic energy or «band» for each pixel. This response is
called the brightness value (BV) or the digital number (DN). In Cogalton, 2009;
the range of brightness values depends on the radiometric resolution. If a
pixel is recorded for a homogeneous area then the spectral response for that
pixel will be purely that type. However, if the pixel is recorded for an area
that has a mixture of types, then the spectral response will be an average of
all that the pixel encompasses. Depending on the size of the pixels, many
pixels may be mixtures.
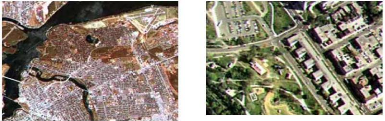
4
Figure 2 : Spatial resolution of different types of sensors,
respectively for spot and Ikonos in Canada center for remote sensing
CCRS,2003
- Radiometric Resolution
The numeric range of the brightness values that records the
spectral response for a pixel is determined by the radiometric resolution of
the digital remote sensing device. These data are recorded as numbers in a
computer as bits and bytes (Jensen, 2007). A bit is simply a binary value of
either 0 or 1 and represents the most elemental method of how a computer works.
If an image is recorded in a single bit then each pixel is either black or
white. No gray levels are possible. Adding bits adds range. If the radiometric
resolution is 2 bits, then 4 values are possible (2 raised to the second power
= 4). The possible values would be 0, 1, 2, and 3. Early Landsat imagery had
6-bit resolution (2 raised to the sixth power = 64) with a range of values from
0 to 63. Most imagery today has a radiometric resolution of 8 bits or 1 byte
(range from 0 to 255). Some of the more recent digital remote sensing devices
have 11 or even 12 bits.
- Temporal Resolution
Temporal resolution is defined by how often a particular
remote sensing device can image a particular area of interest. Sensors in
airplanes and helicopters can acquire imagery of an area whenever it is needed.
Sensors on satellites are in a given orbit and can only image a selected area
on a set schedule. Landsat is a nadir sensor; it only images perpendicular to
the Earth's surface, and therefore can only sense the same place every 16 days.
Other sensors are pointable and can acquire off-nadir imagery.
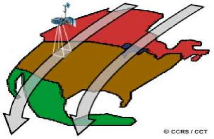
Figure 3 : temporal resolution movement of a sensor in CCRS, 2003
Table 1 Digital characteristics of some satellite are given below, personal
compilation
satellite
|
sensor
|
Ground resolution
|
Radiometric resolution
|
Temporal resolution
|
landsat
|
MSS
|
80m
|
-
|
18 days
|
landsat
|
Thematic Mapper
|
30 m
|
6 bit
|
16 days
|
Spot
|
XS(multispectral)
|
20 m
|
6 bit
|
6 days
|
spot
|
panchromatic
|
10 m
|
6 bit
|
5 days
|
Ikonos
|
Multispectral
|
4 m
|
11 bit
|
2,9 days
|
5
ikonos
|
panchromatic
|
1 m
|
11 bit
|
2,9 days
|
Quickbird
|
|
0,5 m
|
11bit
|
1-3,5 days
|
1.2. Digital image analysis
Digital image analysis in digital remote sensing is analogous
to photo interpretation in analog remote sensing. It is the process by which
the selected imagery is converted/processed into information in the form of a
thematic map. Digital image analysis is performed through a series of steps.
These steps include: (1) image acquisition/selection, (2) pre-processing
including image enhancement, (3) classification, (4) post-processing, and (5)
accuracy assessment.
1.2.1. Image Acquisition/Selection
Selection or acquisition of the appropriate remotely sensed
imagery is foremost determined by the application or objective of the analysis
and the budget. Once these factors are known, the analyst should answer the
questions presented previously. These questions include: what spectral,
spatial, radiometric, temporal resolution and extent are required to accomplish
the objectives of the study within the given budget? Once the answers to these
questions are known, then the analyst can obtain the necessary imagery either
from an archive of existing imagery or request acquisition of a new image from
the appropriate image source.
1.2.2. Pre-processing
Pre-processing is defined as any technique performed on the
image prior to the classification. There are many possible pre-processing
techniques. However, three of the most important techniques include: geometric
registration, radiometric/ atmospheric correction, and numerous forms of image
enhancement.
1.2.3. Classification
Classification of digital data has historically been limited
to spectral information (tone/color). While these methods attempted to build on
the interpretation methods developed in analog remote sensing, the use of the
other elements of photo interpretation beyond just color/tone has been
problematic. In addition, digital image classification has traditionally been
pixel based. A pixel is an arbitrary sample of the ground and
represents the average spectral response for all objects occurring within the
pixel. The earliest classification techniques tended to mimic photo
interpretation and were called supervised classification techniques..
6
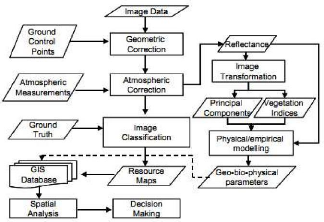
Figure 4 : A schematic diagram of general image processing
procedures, Campbell, 2007
1.2.3.2. Combined Approaches.
Many remote sensing scientists have attempted to combine the
supervised and unsupervised techniques together to take the maximum advantage
of these two techniques while minimizing the disadvantages. Many of these
examples can be found in the literature. A technique by Jensen (2005)
1.2.3.3. Advanced Approaches.
Using supervised or unsupervised classification approaches
only work moderately well. Even the combined approaches only improve our
ability to create accurate thematic maps a little more than using each
technique separately. Therefore, a large amount of effort has been devoted to
developing advanced classification approaches to improve our ability to create
accurate thematic maps from digital remotely sensed imagery. While there are
many advanced approaches, this paper will only mention three: (1)
classification and regression tree (CART) analysis; (2) artificial neural
networks (ANN); and (3) support vector machines (SVM).
1.2.3.4. Object-Based Approaches ( polygon
approach)
By far the greatest advance in classifying digital remotely
sensed data in this century has been the widespread development and adoption of
object-based image analysis (OBIA). Traditionally, all classifications were
performed on a pixel basis. Given that a pixel is an arbitrary delineation of
an area of the ground, any selected pixel may or may not be representative of
the vegetation/land cover of that area. (Gamanya et al.,2008) In the OBIA
approach, unlabeled
7
pixels are grouped into meaningful polygons that are then
classified as polygons rather than individual pixels. This method increases the
number of attributes such as polygon shape, texture, perimeter to area ratio,
and many others that can be used to more accurately classify that grouping of
pixels (Blaschke et al., 2008).
1.2.4. Post-processing
Post-processing can be defined as those techniques applied to
the imagery after it has been through the classification process--in other
words, any techniques applied to the thematic map. It has been said that one
analyst's pre-processing is another analyst's post-processing. It is true that
many techniques that could be applied to the digital imagery as a
pre-processing step may also be applied to the thematic map as a
post-processing step. This statement is especially true of geometric
registration. While currently most geometric correction is performed on the
original imagery, such was not always the case. Historically, to avoid
resampling the imagery and potentially removing important variation
(information), the thematic map was geometrically registered to the ground
instead of the original imagery (Jensen, 2005).
1.2.5. Accuracy Assessment
Accuracy assessment is a vital step in any digital remote
sensing project. The methods summarized here can be found in detail in Green et
al., (2009). Historically, thematic maps generated from analog remotely sensed
data through the use of photo interpretation were not assessed for accuracy.
However, with the advent of digital remote sensing, quantitatively assessing
the accuracy of thematic maps became a standard part of the mapping project.
Once the error matrix is generated, some basic descriptive
statistics including overall, producer's, (Cogalton, 2010) and user's
accuracies can be computed. In addition, there are a number of analysis
techniques that can be performed from the error matrix. Most notable of these
techniques is the Kappa analysis, which allows the analyst to statistically
test if one error matrix is significantly different than another.
1.3. Digital Image Types 1.3.1. Multispectral
Imagery
The dominant digital image type for the last 40 years has been
multispectral imagery, from the launch of the first Landsat in 1972 through the
launch of the latest GeoEye and DigitalGlobe sensors.(Tucker,1985)
Multispectral imagery contains multiple bands (more than 2 and less than 20)
across a range of the electromagnetic spectrum. While there has been a marked
increase in spatial resolution, especially of commercial imagery, during these
40 years it should be noted that there continues to be a great demand for
mid-resolution imagery.
8
1.3.2. Hyperspectral Imagery
Hyperspectral imagery is acquired using a sensor that collects
many tens to even hundreds of bands of electromagnetic energy. This imagery is
distinguished from multispectral imagery not only by the number of bands, but
also by the width of each band. Multispectral imagery senses a limited number
of rather broad wavelength ranges that are often not continuous along the
electromagnetic spectrum. Hyperspectral imagery, on the other hand, senses many
very narrow wavelength ranges (e.g., 10 microns in width) continuously along
the electromagnetic spectrum (Palace et al, 2008).
1.3.3. Digital Camera Imagery
Most digital camera imagery is collected as a natural color
image (blue, green, and red) or as a color infrared image (green, red, and near
infrared). Recently, more projects are acquiring all four wavelengths of
imagery (blue, green, red, and near infrared). The spatial resolution of
digital camera imagery is very high with 1-2 meter pixels being very common and
some imagery having pixels as small as 15 cm.
1.3.4. Other Imagery
There are other sources of digital remotely sensed imagery
that have not been pre-sented in this paper. These sources include RADAR and
LiDAR. Both these sources of imagery are important, but beyond the scope of
this paper. RADAR imagery has been available for many years. However, only
recently has the multifrequency component of RADAR imagery become available
(collecting frequencies of imagery simultaneously and not just multiple
polarizations) that significantly improves the ability to create thematic maps
from this imagery. LiDAR has revolutionized the collection of elevation data
(Maidment et al., 2007) and is a valuable source of information that can be
used in creating thematic maps (Im et al., 2008). In the last few years, these
data have become commercially available and are being used as a vital part of
many mapping projects.
2. Forest Degradation
2.1. Key concepts to forest degradation
Martin et al.( 2009) developed a way for understanding forest
degradation as followed, common indicators for monitoring and assessing forest
degradation can be developed for the following key elements to be used in
assessing forest degradation :
· Biodiversity (e.g. species composition and richness,
habitat fragmentation);
9
· Biomass (e.g. growing stock, forest structure);
· Forest goods obtained (compared against sustainably
managed forests);
· Forest health (e.g. fire, pest and diseases, invasive and
alien species);
· Soil quality (as indicated by cover, depth and
fertility).
For Simula (2009) terms degradation is a change process within
the forest, which negatively affects the characteristics of the forest. The
combination of various forest characteristics (forest quality) can be expressed
as the structure or function, which determines the capacity to supply forest
products and services (IPCC, 2003). Forests may be degraded in terms of loss of
any of the goods and services that they provide wood, food, habitat, water,
carbon storage and other protective socio-economic and cultural values
(Guariguata, 2009).
According to FAO (2002) degradation is typically caused by
disturbances, which vary in terms of the extent, severity, quality, origin and
frequency. The change process can be natural (caused by fire, storm, drought,
pest, disease) or it can be human induced (unsustainable logging, excessive
fuelwood collection, shifting cultivation, unsustainable hunting,
overgrazing).
Perceptions regarding forest degradation are many and varied,
depending on the driver of degradation and the main point of interest (Souza,
2005). In relation to REDD it is likely to entail a reduction in the capacity
to sequester carbon, but a forest may also be degraded in terms of loss of
biological diversity, forest health, productive or protective potential or
aesthetic value.
Forest degradation is generically defined as the reduced
capacity of a forest to provide goods and services (FAO, 2002). However, in the
context of climate change, the International panel on climate change IPCC
(2003) developed a definition of forest degradation that focuses on
human-induced changes in the carbon cycle in the long run:
A direct human-induced long-term loss (persisting for X years
or more) of at least Y% of forest carbon stocks [and forest values] since time
T and not qualifying as deforestation or an elected activity under Article 3.4
of the Kyoto Protocol, (ITTO, 2005).
2.2. Main causes of forest degradation
Many natural factors and human activities can affect forest
health and vitality leading to a gradual or sudden decrease in forest growth,
tree mortality and to a decline in the provision of forest goods and services.
For Fargan et al. (2009), Wild or human-induced fires, pollution, floods,
nutrients and extreme weather conditions such as storms, hurricanes, droughts,
snow, frost, wind and sun are among abiotic agents that may be responsible for
a loss of health and vigor of forest ecosystems. Biotic influences of forest
conditions include insect pests, diseases
10
and invasive species and can either consist of fungi, plants,
animal or bacteria. Humans are also a major factor of forest health
deterioration as overexploitation, competing land uses, poor harvesting
techniques or management can negatively impact forest ecosystems.
In the study of Herold et al. (2009), Forest degradation can
have any number of causes, dependent on resource condition, environmental
factors, socio-economic and demographic pressure and incidents for example
pests, disease, fire, and natural disasters. The understanding and separation
of different degradation processes is important for the definition of suitable
methods for measuring and monitoring. Various types of degradation will have
different effects on the forest storage carbon and result in different types of
indicators that can be used for monitoring degradation using in situ
and remote methods (i.e. trees being removed, canopy damaged etc.).
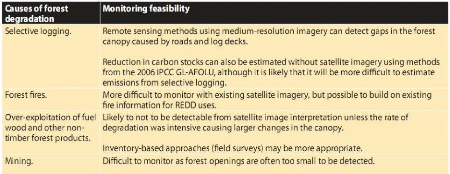
Table 2: causes of degradation and impacts on monitoring (adapted
from GOFC-GOLD, 2008)
3. Mapping forest degradation
3.1. Remote sensing in forest degradation
In the idea of the study of Kauppie et al. (2006) the way to
quantify change in the forest is to select four forests attributes area,
volume, density of growing stock, biomass, and sequester carbon) that provide a
useful starting point for global forest monitoring. According to Cogalton
(2007), these dates are particularly essential when attempting to estimate
forest volume, biomass and carbon using remote sensing technology.
11
Lambin (1999) explained the role of remote sensing in forest
degradation, in this study, the author is trying to explain how spectral,
spatial and temporal information could be used to access the concept of forest
degradation.
In the study of Herold et al. (2009) they explained that
mapping forest degradation with remote sensing data is more challenging than
mapping deforestation because the degraded forest is a complex mix of
different land cover types (vegetation, dead trees, soil, shade) and the
spectral signature of the degradation changes quickly (i.e., < 2 years)
(Souza et al. 2009 in herold et al). High spatial resolution sensors
such as Landsat, ASTER and SPOT have been mostly used so far to address forest
degradation. However, very high resolution satellite imagery, such as Ikonos or
Quickbird, and aerial digital imagery acquired with videography has been used
as well. Methods for mapping forest degradation range from simple image
interpretation to highly sophisticated automated algorithms
(GOFC-GOLD,2008).
Higher spatial resolution imagery is more suitable for
detection of specific forest degradation impacts. For example, Ikonos imagery
can easily detect forest canopy structural damage (Read et al., 2003; Souza, Jr
et al., 2005), but, given the cost for image acquisition and computational
challenges to extract information from these very high spatial resolution
images, their use in operational applications such as monitoring logging is
limited.
3.2. Forest change detection analysis
Various classifications of change in forest ecosystems have
been proposed. Aldrich (1985) approached the variability in forest cover from a
thematic angle, enumerating nine general forest disturbance classes: no
disturbance, harvesting (areas subjected to timber removal operations),
silvicultural treatments (e.g., thinning), land clearing (vegetation removal
and site preparation), insect and disease damage (epidemic conditions), fire
(prescribed burning and wildfire), flooding (man-caused and natural),
regeneration (artificial or natural), Other (not fitting any of the above
categories).
Coppin (2001) tried to explain different types of change
detection in forest ecosystems with remote sensing using digital imagery, he
used many techniques change detection, image acquisition, data reprocessing for
change detection methods, multidimensional temporal feature space analysis,
image differencing, etc.
Since early days of earth observation systems, various
techniques of change detection have been developed for forest monitoring using
high resolution optical remote sensing. These approaches are focused on the
identification of forest cover change, described by Geist (2006),
12
Highly Detectable
|
Detection limited & increasing data/effort
|
Detection very limited
|
·
|
Deforestation
|
·
|
Selective logging
|
·
|
Harvesting of most non-
|
·
|
Forest fragmentation
|
·
|
Forest surface fires
|
|
timber plants products
|
·
|
Recent slash-and-burn
|
·
|
A range of edge-effects
|
·
|
Old-mechanized
|
|
agriculture
|
·
|
Old-slash-and-burn
|
|
selective logging
|
·
|
Major canopy fires
|
|
agriculture
|
·
|
Narrow sub-canopy
|
·
|
Major roads
|
·
|
Small scale mining
|
|
roads (<6m wide)
|
·
|
Conversion to tree
monocultures
|
·
|
Unpaved secondary
roads (6-20m wide)
|
·
|
Understorey thinning and clear cutting
|
·
|
Hydroelectric dams and
other forms of flood disturbances
|
·
|
Selective thinning of
canopy trees
|
·
|
Invasion of exotic
species
|
·
|
Large-scale mining
|
|
|
|
|
Table 3: Forest degradation activities and their degree of
detection using Landsat-type data, Source:
Peres et al.(2006).
The literature indicates that forest canopy changes can be
detected by a variety of analysis methods. Although most methods provide
generally positive results, few studies have compared and evaluated alternative
approaches. Since Singh's 1999 paper, two recent studies have attempted to
determine what change detection method is most appropriate.
Using SPOT multispectral, multitemporal data, Muchoney and
Haack (2004) compared four methods, merged PCA, image differencing, spectral
temporal change classification, and post-classification change differencing,
for identifying changes in hardwood defoliation by gypsy moth. Defoliation was
most accurately detected by the image differencing and PCA approaches.
Collins and Woodcock (1996) have compared three linear change
detection techniques, multitemporal Kauth-Thomas, PCA, and Gramm-Schmidt
orthogonalization. Better and similar results were obtained with the
multitemporal Kauth-Thomas and PCA methods than for the Gramm-Schmidt
technique; however, the authors recommended the Kauth-Thomas approach because
it identifies change in a more consistent and interpretable manner. These
authors also examined to what extent the digital images should be
preprocessed.
3.3. Direct methods of mapping forest degradation
Visual interpretation of high resolution data can detect
canopy damage in some cases (Saatchi et al., 2007). Spatial patterns of log
landings (patios for logging trucks and river landings) and identification of
other infrastructure (e.g. roads and rivers used for transportation) has been a
successful approach for identifying degradation (Asner et al., 2005). Likewise
deforestation and forest degradation can be mapped with different techniques,
varying from visual interpretation to advanced image processing algorithms.
13
Among the most classically used techniques Herald et al.,2009;
related some of them Visual interpretation, which can easily detect canopy
damage areas in very high spatial resolution imagery; ii) automate
segmentation; iii) spectral mixing analysis for logging disturbances (Asner
et al. 2005, Oliveira et al. 2007) and fire (Souza et
al. 2005); iv) lacunarity indices for canopy structural characterization
(Malhi and Román-Cuesta 2008); vi) Hyperspectral automated canopy
identification (Palace et al. 2008).
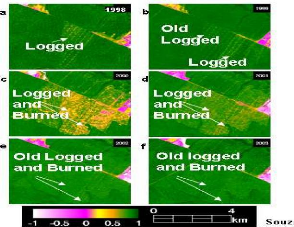
Figure 5: Spectral mixing analysis (SMA) as a way to follow the
degradation dynamics of Amazonian lowland forests using Ikonos sensors
(Souza et al. 2005),
For example Asner et al. (2005) developed automated algorithms
to identify logging activity with Landsat data. Detection of active fires with
thermal data can also indicate presence of subsequent burn scars (Roy et al.,
2005).An effective solution for identifying degraded forests from proximity to
infrastructure has recently been proposed to take advantage of existing
observational approaches given the current limitation in knowledge on the
spatial distribution of biomass (Mollicone et al., 2007).
3.4. Indirect methods of forest degradation mapping
In the study of herald et al. (2009), they tried to explain in
detail this method as The indirect method is useful when degradation intensity
is low and the area to assess is large, when satellite imagery is not easily
accessible, or when the direct approach cannot be applied for whatever other
reason. An example of a useful indirect approach is the «intact
forest» approach where the spatial distribution of human infrastructures
(i.e. roads, population centres) are used as proxies, so that the absence of
these are used to identify forest land without anthropogenic disturbance
14
(intact forests) so as to assess the carbon content present in
the disturbed and non-disturbed forest lands (Mollicone et al. 2007;
Potopov et al. 2008 in Herald et al, 2009):
According to this previeous study of herald, Scenario modelling
for forest degradation would be another indirect method which could be applied
to estimate both future and historical forest degradation dynamics..
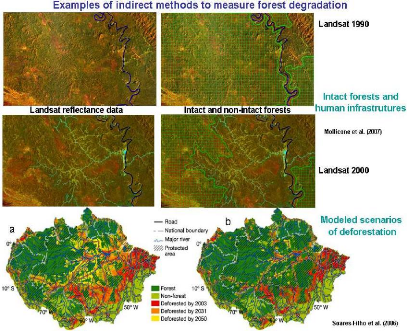
Figure 6: Estimation of intact and non-intact
forests based on areas of influence (buffers) from human infrastructures
Soares-Filho et al. 2006
3.5. Relevancy of different forest degradation
approach
In the study of Achanya and Dangi, 2009 they tried to develop
a relevancy of different forest degradation assessment in Nepal. This method
can also be applied in the tropical region of Africa.
15
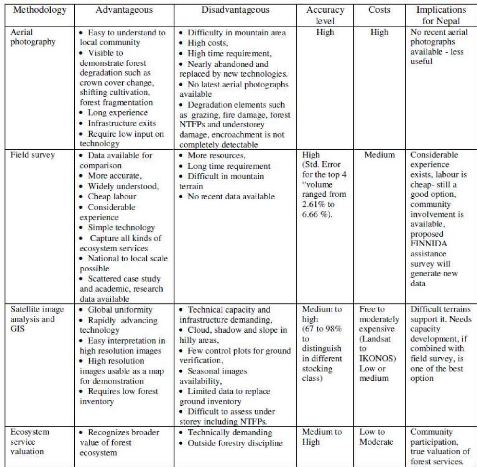
Table 3 : Relevance of different forest degradation approach,
source: Acharya and Dangi, 2009
3.6. The use of vegetation indices as NDVI concept to
assess forest degradation
Vegetation indices are the quantitative measure of measuring
biomass or vegetation vigour, usually formed by a combination of several
spectral bands; whose values are added, divided or multiplied in order to yield
a single value that indicates the amount or vigour of vegetation. A variety of
vegetation indices have been developed, with most commonly using red and near
infrared regions of the spectrum to emphasize the difference between strong
absorption of red
16
electromagnetic radiation and the strong scatter of near infrared
radiation. The simplest form of vegetation index is a ratio between near
infrared and red reflectance and it is high for healthy living vegetation.
Literature survey revealed wide disagreement regarding the biomass and
vegetation indices relationship. Many studies report a significant positive
relationship ( Boyad et al., 1999 ) while some results showed poor relationship
( Foody et al.,2003,schlerf et al.,2005).
The normalized difference index is one of the most commonly
used vegetation indices in many applications relevant to analysis of
biophysical parameter of forests. Over past two decades its utility has been
well demonstrated in satellite assessment and monitoring of global vegetation
cover (Huete and Liu,1994,leprieur et al 2000). The strength of NDVI is in its
rationing concept which reduces many form of multiplicative noise present in
multiple bands. However, conclusions about its value vary depending on the use
of specific biophysical parameters and characteristics of the study area. (Deo,
2008).It is computed by the product of the ratio of two electro-magnetic
wavelengths (near infrared- red )/(near infrared+red). Vegetation has a high
near chlorophyll pigments and the value of NDVI tends to one. In contrast of
this, clouds, water, snow etc. have a high red reflectance than near-infrared
and these features yield negatives NDVI value. Rocks and bare soil also have
similar reflectance and usually zero value of NDVI;
The saturation of the relationship between biomass and NDVI is
also a well known problem. This can be explained by the fact that as canopy
increases, the amount of red light that can be absorbed by leaves reaches a
peak while near-infrared (NIR) reflectance increases because of multiples
scattering with leaves. The imbalance between a slight decrease in the red and
high NIR reflectance results in a slight change in the NDVI ratio and thus,
yield poor relationship with biomass (Tenkabail et al., 2000). Further, Rauste
(2005) observed that saturation level is also dependent on the tree species,
forest types as well as the ground surface types. Therefore, a suitable
relationship of vegetation indices and biomass is crucial in assessment of
biomass in different circumstances and a matter of more research work. The
usefulness of remote sensing in such work depends on the strength of the
relationships developed with respect to a particular type of forests and its
geographical location.
3.7. Forest canopy change and remote sensing
Researchers have found relationships between vegetation
properties and remotely sensed variables. In order to summarize these diverse
experiments, basal area and canopy cover, and the volume and productivity
variable includes age, height, volume, diameter and density. Brockhaus et al.,
(1992) found a significant relationship between green TM band (2) with basal
area of trees.
More recent work by Fiorella et al. (2003) found that ratios
of near-infrared/red and near-infrared/middle-infrared correlated with
structural forms. Cook et al (1989) discovered that vegetation productivity is
more strongly related to band ratios than individual bands.
Both the volume and the aboveground biomass (AGB) of forests
can be estimated from allometri c relationships with canopy width, structure,
and/or height, the intensity of SAR backscatter, corr
17
Nelson et al (1984) analyzed (simulated) TM data, and
concluded most information about vegetation was contained in the blue,
near-infrared and middle-infrared. Thermal infrared was used by Holbo and
Luvall (1989) to map broad forest type classes. Vegetation dieback and damage
are best mapped by band ratios.
3.8. Comparing Forest Inventory and Remote Sensing
Measurement for forest degradation mapping
The same forest quantities (e.g., biomass) are estimated
differently by ground forest inventory and by remote sensing . Forest inventory
typically measures tree abundance, diameter, crown width, species, and height
(Song 2007; Chave et al. 2005).
Table 5. How Forest Inventory and Remote Sensing Estimate the
Forest Identity, adapted from Fragn et al,2009.
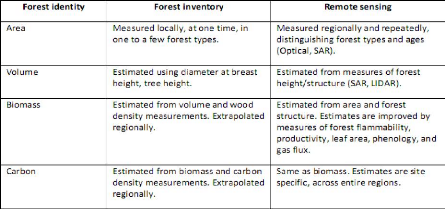
Remote sensing measures reflected spectra, forest area and the
horizontal and vertical structure of forests can be measured directly from
these reflected spectra. Fieldwork or higher resolution imagery can be used to
generate ground-truth data to assess the accuracy of these forest area and
structure measurements (Jensen 2007).
3.9. Estimating Forest Volume Using Remote Sensing
18
elations with passive spectra, and various fusions of the above
(Lu et al. 2006; Balzter et al. 2007; Rosenqvist et al. 2003).
3.10. Estimating forest biomass using remote
sensing
Biomass cannot be directly measured from remote sensing data,
however remotely sensed reflectance can be related to the biomass estimates
based on in situ measurements (Dong et al. 2003). Reflections of the red, green
and near infrared radiances contained considerable information about forest
biomass. Two main approaches predicting biomass using satellite images are (1)
Use of Solar radiation and (2) Use of Reflection Coefficients (Namayanga 2002),
which is primarily determined by the green foliage biomass (Christensen and
Goudriaan, 1993).
Forest height can be measured from a variety of remotely sensed
data and used to estimate biomass (Kellndorfer et al. 2004; Palace et al. 2008,
Pflugmacher et al. 2008). Although diamete, height, and wood density are
central variables, biomass estimates can be improved by using addit ional
forest structure variables (e.g., canopy width, canopy volume) (Dubayah et al.
2000; Palace et al. 2008; Popescu et al. 2003).
Direct biomass estimation may also be possible with vegetation
Light Detection and Ranging (LIDAR) observations (Popescu 2007; Drake et. al
2002). The potential of forest biomass mapping has also been explored using
Radar (Gaveau et al., 2003; Tomppo et al. 2002) along with JAXA ALOS-PALSAR
L-band (24 cm wavelength) which gives lower range of biomass (upto 50-80 t/ha).
The BIOMASS mission, which is expected to launch around 2014 by ESA uses a
longer wavelength (68 cm) and shows potential of estimating higher levels of
biomass (FAO,2008).
3.11. Estimating Forest Carbon Stocks from Remotely
Sensed Data
Satellite imaging can tell us much about global carbon stocks,
but there are limits to its accuracy. Dry biomass is approximately 47.to.55
percent carbon by weight (IPCC 2006), so aboveground b iomass estimates from
remote sensing can be simply converted into aboveground carbon (AGC) stock
estimates (Gibbs et al. 2007).
Carbon emissions from deforestation and degradation depend not
only on the area of forest change but also on the associated biomass loss
(Brown, 2002). The IPCC (Penman et al., 2003a) compiled methods and good
practice guidance for determining changes in carbon stocks in association with
national inventories of greenhouse gas (GHG) emissions (Chapter 3 in Penman et
al., 2003a) for changes in Land Use, Land Use Change, and Forestry (LULUCF) and
with carbon sequestration projects (Penman et al., 2003a) in the first
commitment period. With the updated version of the IPCC guidelines for
conducting national GHG emissions from the
19
LULUCF sector (Penman et al., 2003a; IPCC, 2006), methods are
available for estimating GHG emissions from deforestation at the national and
project scales.
4. Conclusion
As conclusion of this literature review, we can see that a lot
f conclusion can be formulated from this literature review.
Our literature review had three important parts, the first one
helped us to understand the concept of remote sensing, the second one was a
brief explanation of the concept of forest degradation and the last one
explained different methodologies to evaluate the problem of degradation using
remote sensing
As a change in forest structure it is not easy to detect the
problem through remote sensing, the choice of different approaches depends on a
number of factors including the type of degradation process, available
(historical) data, capacities and resources and the potentials and limitations
of various measurement and monitoring approaches.
Mapping forest degradation should not be only a problem of
scientist but a social problem putting together interdisciplinary stakeholders,
they must have information of the problem in order to quantify the extent of
the threat. the preceding discussion the point 3 of this literature review has
explored how advancements in remote sensing techniques would help to gather
information and to answering most of these questions about all sides of the
definition of forest degradation
The big challenge is now how those methodologies especially in
developing country because of the availability of the satellites imagery,
increase in new processing technologies methods, and the cost of spatial
information.
20
5. References
Acharya, K., and Dangi, R. 2009. Forest Degradation in Nepal:
Review of Data and Methods. FAO Forest Resources Assessment Programme, Rome,
Italy
Anderson, J.E., L.C. Plourde, M.E. Martin, B.H. Braswell, M.L.
Smith, R.O. Dubayah, M.A. Hof ton, and J.B. Blair. 2008. Integrating waveform
lidar with hyperspectral imagery for inve ntory of a northern temperate forest.
Remote Sensing of the Environment 112(4): 1856- 1870.
Andersson, K., T.P. Evans, and K.R. Richards. 2009. National
forest carbon inventories: Policy needs and assessment capacity. Climatic
Change 93(1-2): 69-101.
Askne, J., M. Santoro, G. Smith, and J.E.S. Fransson. 2003.
Multitemporal repeat-pass SAR inter ferometry of boreal forests. IEEE
Transactions on Geoscience and Remote Sensing 41(7): 1540-1550.
Asner, G. P., M. Keller, R. Pereira, and J. C. Zweede. 2002.
Remote sensing of selective logging in Amazonia: Assessing limitations based on
detailed field observations, Landsat ETM+, and textural analysis. Remote
Sensing of Environment 80:483-496.
Asner, G. P., M. Keller, R. Pereira, J. C. Zweede, and J. N. M.
Silva. 2004. Canopy damage and recovery after selective logging in Amazonia:
Field and satellite studies. Ecological Applications 14:S280-S298.
Asner, G. P., D. E. Knapp, E. N. Broadbent, P. J. C. Oliveira, M.
Keller, and J. N. M. Silva. 2005. Selective logging in the Brazilian Amazon.
Science 310:480-482.
Asner, G., Rudel, T., Aide, M., Defries, R., Emerson, R. 2009. A
contemporary assessment of change in humid tropical forests. Conservation
Biology 23:1386-1395.
Baccini, A., N. Laporte, S.J. Goetz, M. Sun, and H. Dong. 2008. A
first map of tropical Africa's aboveground biomass derived from satellite
imagery. Environmental Research Letters 3: 1-9
Baikal region. Forest Ecology and Management 257(3): 911-922.
Bicheron, P., P. Defourny, C. Brockmann, L. Schouten, C.
Vancutsem, M. Huc, S. Bontemps, Leroy, F. Achard, M. Herold, F. Ranera, and O.
Arino. 2008. GLOBCOVER Products Description and Validation Report.
MEDIAS-France,
ftp://uranus.esrin.esa.int/pub/globc
over v2/global/.
DeFries, R. 2008. Terrestrial vegetation in the coupled
human-earth system: Contributions of re mote sensing. Annual Review of
Environment and Resources 33: 369-390.
21
Blaschke, T., Lang, S., and G. Hay (Eds.), 2008, Object-Based
Image Analysis, Berlin, Germany: Springer-Verlag, 817 p
Brockhaus, J A and S Khorram. 1992. A comparison of Spot
andLandsat-TM data for use in conducting inventories of forest resources. IJRS
13, 16, pp 3035-3043.
Campbell, J., 2007, Introduction to Remote Sensing, 4th ed., New
York, NY: Guilford Press, 626p.Change Biology 11(6): 945-958.
Chave, J., C. Andalo, S. Brown, M.A. Cairns, J.Q. Chambers, D.
Eamus, H. Folster, et al. 2005.
Comparison of various remote sensing data sources in the
retrieval of forest stand
Congalton, R. and K. Green, 2009, Assessing the Accuracy of
Remotely Sensed Data: Principles and Practices, 2nd ed., Boca Raton, FL:
CRC/Taylor & Francis, 183 p.
Congalton, R., 2010, «How to Assess the Accuracy of Maps
Generated from Remotely Sensed Data,» in Manual of Geospatial Science and
Technology, 2nd ed., Bossler, J. (Ed.), Boca Raton, FL: Taylor & Francis,
403-421.
Cook E A, L R Iverson and R L Graham. 1989. Estimating forest
productivity with Thematic Mapper and biogeographical data.Remote Sens of
Environ 28, pp 131-141.
Cramer, W., A. Bondeau, S. Schaphoff, W. Lucht, B Smith and S.
Sitch 2004. Tropical forests and the global carbon cycle: impacts of
atmospheric carbon dioxide, climate change and rate of deforestation. Phil.
Trans. Roy. Soc. Lond. B 359: 331-343.
Danson, F M and P J Curran. 1993. Factors affecting the remotely
sensed response of coniferous forest plantations. Remote Sensing of Environ 43,
pp 55-65.
De Wulf, R R, R E Goosens, B P De Roover and F C Borry.
1990.Extraction of forest stand parameters from panchromatic and multi-spectral
Spot-1 data. IJRS 11, 9, pp 1571-1588.
DeFries, R., F. Achard, S. Brown, M. Herold, D. Murdiyarso, B.
Schlamadinger, and C. de Souz a. 2007. Earth observations for estimating
greenhouse gas emissions from deforestation in developing countries.
Environmental Science and Policy 10(4): 385-394.
GOFC-GOLD. 2009. A sourcebook of methods and procedures for
monitoring and reporting anthropogenic greenhouse gas emissions and removals
caused by deforestation, gains and
22
Donnellan, A., P. Rosen, J. Graf, A. Loverro, A. Freeman, R.
Treuhaft, R. Oberto, et al. 2008. Deformation, ecosystem structure, and
dynamics of ice (DESDynI). Paper presented at th e ESRI International User
Conference. April 2008, Washington, DC.
FAO. 2002. Food and Agriculture Organization. Proceedings:
Second Expert Meeting on Harmonizing Forest-related Definitions for Use by
Various Stakeholders. Rome, 11-13 September 2002. Rome.
http://www.fao.org/docrep/005/y4171e/y4171e00.htm
FAO. 2006a. Food and Agriculture Organization .Global Forest
Resources Assessment
FAO.2006b.Summaries of FAO's work in forestry. Rome, Italy.
http://www.fao.org/forestry/foris/webview/forestry2
FAO. 2007. Food and Agriculture Organization. State of the
World's Forests. United Nations, Rome. Available:
http://www.fao.org/docrep/009/a0773e/a0773e00.htm.
Fiorella, M and W J Ripple. 1993. Determining successional stage
of temperate coniferous forests with Landsat satellite data. PE&RS59, 2, pp
239-246.
Foody, G.M. 2002. Status of land cover classification accuracy
assessment. Remote Sensing of Environment 80(1): 185-201
Franklin J F, F W Davis and P Lefebvre. 1991. Thematic Mapper
analaysis of tree cover in semiarid woodlands using a model of canopy
shadowing. Remote Sens of Environ 36, pp 189-202.
Foody,G.M.,Boyad,D.S.,Cutler M.E.J.,2003.Predictive relations
of tropical forest biomass from landsat TM data and their transferability
between regions.Remote Sensing of environment 84(4):463-474.
Gao, X., A.R. Huete, W.G. Ni, and T. Miura. 2000.
Optical-biophysical relationships of vegetation spectra without background
contamination. Remote Sensing of Environment 7 4(3): 609-620
Gibbs, H.K., S. Brown, J.O. Niles, and J.A. Foley. 2007.
Monitoring and estimating tropical
forest carbon stocks: Making REDD a reality. Environmental
Research Letters 2(4): 045023.
Jensen, J., 2005, Introductory Digital Image Processing: A Remote
Sensing Perspective, 3rd ed., Upper Saddle River, NJ: Pearson Prentice Hall,
526 p.
23
losses of carbon stocks in forest remaining forests, and
forestation. GOFC-GOLD Report version COP15-1.Available at
www.gofc-gold.uni-jena.de/redd/.
Hame, T, E Tomppo and E Parmes. 1988. Stand based forest
inventory from Spot Image. Symp proc: Spot-1, Image Utilisation,Assessment,
Results. CNES, Cepadues Editions, Toulouse, France,pp 971-976.
Herold, M., Yasumasa H., Patrick V.,Asner G., Victoria
Heymell5, Rosa María Román-Cuesta6
Houghton, R.A. 2005. Aboveground forest biomass and the global
carbon balance. Global Change Biology 11(6): 945-958
Huete, A. R., Miura, T., & Gao, X. (2003). Land cover
conversion and degradation analyses
through coupled soil-plant biophysical parameters derived from
hyperspectral EO-1 Hyperion. IEEE Transactions on Geoscience and Remote
Sensing, 41(6), 1268-1276.
Hyyppa, J., H. Hyyppa, M. Inkinen, M. Engdahl, S. Linko, and Y.H.
Zhu. 2000. Accuracy comparison of various remote sensing data sources in the
retrieval of forest stand attributes . Forest Ecology and Management 128(1-2):
109-120.
Hyyppa, J., H. Hyyppa, D. Leckie, F. Gougeon, X. Yu, and M.
Maltamo. 2008. Review of methods of small-footprint airborne laser scanning for
extracting forest inventory data in b oreal forests. International Journal of
Remote Sensing 29(5): 1339-1366
IPCC. Intergovernmental Panel on Climate Change. 2003. Good
Practice Guidance on Land Use, Land-Use Change and Forestry. Eggleston,
H.S.,Buendia, L., Miwa, K., Ngara, T. and Tanabe, K. (eds.). National
Greenhouse Gas Inventories Programme. Institute for Global
Environmental Strategies (IGES). Japan.
http://www.ipcc-
nggip.iges.or.jp/public/gpglulucf/gpglulucf_contents.html
ITTO. 2005. International Tropical Timber Organization. 2005.
Status of tropical forest
management 2005. Available:
http://www.itto.or.jp/live/PageDisplayHandler?pageId=270.
Jensen, J. R., Im, J., Jensen, R., and P. Hardin, 2009,
«Image Classification,» in Handbook of Remote Sensing, Nellis, D. and
T. Warner (Eds.), Boca Raton, FL: CRC Press, 82-102 (Chapter 19).
24
Jensen, J., 2007, Remote Sensing of Environment: An Earth
Resource Perspective, 2nd ed., Upper Saddle River, NJ: Pearson Prentice Hall,
592 p.
Kauppi, P.E., J.H. Ausubel, J.Y. Fang, A.S. Mather, R.A. Sedjo,
and P.E. Waggoner. 2006. Returning forests analyzed with the forest identity.
Proceedings of the National Academy of Sciences of the United States 103(46):
17574-17579.
Kayitakire, F., C. Hamel, and P. Defourny. 2006. Retrieving
forest structure variables based on image texture analysis and IKONOS-2
imagery. Remote Sensing of Environment 102(3- 4): 390-401.
Kellndorfer, J., W. Walker, D. Nepstad, C. Stickler, P. Brando,
P. Lefebvre, A. Rosenqvist, and M. Shimada. 2008. Implementing REDD: The
potential of ALOS/PALSAR for forest mapping and monitoring. Paper presented at
the Second GEOSS Asia-Pacific
Symposium. April 2008, Tokyo, Japan.
Kimes, D.S., K.J. Ranson, G. Sun, and J.B. Blair. 2006.
Predicting lidar measured forest
vertical structure from multi-angle spectral data. Remote Sensing
of Environment 100(4): 503-511.
Leprieur,P.E. ;Kerr ?Y.H.,Mastorchio,S.,Meunier,J .C.,2000
.Monitoring vegetation cover across semi-arid regions:comparision of remote
observations from various scales.International journal of remote
sensing21:281-300.
Lu, D.S. 2006. The potential and challenge of remote
sensing-based biomass estimation. International Journal of Remote Sensing
27(7): 1,297-1,328
Lund, H. 2009. What is a degraded forest. Forest Information
Services. Gainesville, VA. USA.
http://home.comcast.net/~gyde/2009forestdegrade.doc
Luus, K.A., and R.E.J. Kelly. 2008. Assessing productivity of
vegetation in the Amazon using M. Shimada. 2008. Implementing REDD: The
potential of ALOS/PALSAR for forest
Malhi, Y., J.T. Roberts, R.A. Betts, T.J. Killeen, W.H. Li and
C.A. Nobre. 2008. Climate change, deforestation, and the fate of the Amazon.
Science 319: 169-172.
Maltamo, M., K. Eerikainen, P. Packalen, and J. Hyyppa. 2006.
Estimation of stem volume mapping and monitoring. Paper presented at the Second
GEOSS Asia-Pacific Marrakech Accords. Bonn, Germany.
25
Means, J.E., S.A. Acker, D.J. Harding, J.B. Blair, M.A. Lefsky,
W.B. Cohen, M.E. Harmon, and methods of small-footprint airborne laser
scanning for extracting forest inventory
Mollicone D., Achard F., Federici S., Eva H.D., Grassi G.,
Belward A., Raes F., Seufert G., Stibig H.-J., Matteucci G., Schulze E.-D.
2007. An incentive mechanism for reducing emissions from conversion of intact
and non-intact forests. Climatic Change 83: 477- 493.
Nelson, R F, R S Latty and G Mott. 1984. Classifying northern
forests using thematic mapper simulator data. PE&RS 50, 5, pp 607-617.
Nemani, R, P S Running and L Band. 1993. Forest ecosystem
processes at the watershed scale: sensitivity to remotely-sensed LeafArea Index
estimates. IJRS 14, 13, pp 2519-2534.
Oliveira, P. J. C., G. P. Asner, D. E. Knapp, A. Almeyda, R.
Galvan-Gildemeister, S. Keene, R. Raybin, and R. C. Smith. 2007. Land-use
allocation protects the Peruvian Amazon. Science 317:1233-1236.
Page, S.E., F. Siegert, J.O. Rieley, H.D.V. Boehm, A. Jaya, and
S. Limin. 2002. The amount of c arbon released from peat and forest fires in
Indonesia during 1997. Nature 420(6911): 61 -65.
Palace, M., Keller, M., Asner, G., Hagen, S., and B. Braswel.
2008. Amazon Forest Structure from IKONOS Satellite Data and the Automated
Characterization of Forest Canopy Properties. Biotropica 40: 141-150
Patenaude, G., R. Milne, and T.P. Dawson. 2005. Synthesis of
remote sensing approaches for
forest carbon estimation: Reporting to the Kyoto Protocol.
Environmental Science and Policy 8(2): 161-178.
Peres, C., Barlow, J., and Laurance, W. 2006. Detecting
anthropogenic disturbance in tropical forests. TRENDS in Ecology and Evolution
21, 227-229.
Peterson, L.K., K.M. Bergen, D.G. Brown, L. Vashchuk, and Y.
Blam. 2009. Forested land-cover patterns and trends over changing forest
management eras in the Siberian Baikal region. Forest Ecology and Management
257(3): 911-922
Popescu, S.C., R.H. Wynne, and R.F. Nelson. 2003. Measuring
individual tree crown diameter with lidar and assessing its influence on
estimating forest volume and biomass. Canadian Journal of Remote Sensing 29(5):
564-577.
26
Rosenqvist, A., A. Milne, R. Lucas, M. Imhoff, and C. Dobson.
2003. A review of remote sensing technology in support of the Kyoto Protocol.
Environmental Science and Policy 6(5): 441-455
Simula, M. 2009. Towards defining forest degradation: comparative
analysis of existing definitions. Forest Resources Assessment. Pp 57. Working
Paper 154. FAO, Rome.
ftp://ftp.fao.org/docrep/fao/012/k6217e/k6217e00.pdf
Smith, J A, T L Lin et al. 1980. The Lambertian assumption
andLandsat data. PE&RS 46, 9, pp 1183-1189.
Song, C. 2007. Estimating tree crown size with spatial
information of high resolution optical remotely sensed imagery. International
Journal of Remote Sensing 28(15): 3305- 3322
Song, C., T.A. Schroeder, and W.B. Cohen. 2007. Predicting
temperate conifer forest successional stage distributions with multitemporal
Landsat Thematic Mapper imagery. Remote Sensing of Environment 106(2):
228-237
Souza, C., L. Firestone, L. M. Silva, and D. Roberts. 2003.
Mapping forest degradation in the Eastern Amazon from SPOT 4 through spectral
mixture models. Remote Sensing of Environment 87:494-506.
Souza, C., D. A. Roberts, and M. A. Cochrane. 2005. Combining
spectral and spatial information to map canopy damages from selective logging
and forest fires. Remote Sensing of Environment 98:329-343.
Souza, C., Cochrane, M., Sales, M., Monteiro, A., Mollicone,
D. 2009. Integrating forest transects and remote sensing data to quantify
carbon loss due to forest degradation in the Brazilian Amazon, FRA Working
Paper 161
Tucker, C J. 1979. Red and photographic infrared linear
combinations for monitoring vegetation. Rem Sens of Environ 8, pp 127-150.
Tucker, C.J., J.R. Townshend, and T.E. Goff. 1985. African
land-cover classification using satellite data. Science 227: 369-375.
UNFCCC (United Nations Framework Convention on Climate
Change). 2001. COP-7: The Marrakech Accords. Bonn, Germany.
|