UNIVERSITY OF BOTSWANA

Faculty of Sciences Geology Department MASTERS
PROGRAMME OF HYDROGEOLOGY
HYDROLOGICAL MODELLING OF THE CONGO RIVER BASIN: A
SOIL-WATER BALANCE APPROACH
Josué BAHATI CHISHUGI
A Dissertation submitted to the School of Graduate
Studies in partial fulfilment of the requirements for the degree of Master of
Science (MSc) in Hydrogeology
SUPERVISOR: Dr B.F. Alemaw 2008
DECLARATIONS
I solemnly declare that this work is the result of my own toiling
and has never been submitted anywhere for any award.
Signature of Author
Date
Josue BAHATI CHISHUGI
This dissertation has been submitted for examination with my
authority as a University supervisor.
Signature of the Supervisor
Date
Dr B.F. ALEMAW
STATEMENT OF COPYRIGHT
No part of this dissertation may be reproduced, stored in any
retrieval system, or transmitted in any form or by any means: electronic,
mechanical, photocopying, recording, or otherwise, without prior written
permission of the Author or the University of Botswana.
ACKNOWLEDGEMENT
To my Parents, brothers and sisters in D.R.Congo for their
unlimited love and support through the many years of school;
To my fiancée, Rachel Moiza Maline, for her Love and
endurance,
To the German Academic Exchange (DAAD) for the fellowship which
enabled us to complete this M.Sc. degree in Hydrogeology;
To my supervisor Dr B.F. Alemaw, for his invaluable help and
encouragement, fruitful advice and patience during my introduction to Water
Balance Modelling;
To Dr. T.R. Chaoka, the Head of Geology Department, University of
Botswana, for his advices and financial support that regenerated my efforts;
To all the Staff member of Geology Department for their advises
and supports;
To my Congolese family in Botswana, in particular Madam P.
Kampunzu, Dr. Lukusa and His wife, Papa and Maman Mihigo, Prof. Kitenge's
family, V. L. Basira, A. Ibrahim, S. Loly, S.M. Oscar, L. Wani, Dr Chantal and
Asina;
To Neovitus Shayio, K. Justin and Kaniki, Tina, Adjoa, and many
good people I met in Botswana, Geramny and Malawi;
To my graduate colleagues in Batswana, Z. Chiyapo, M. Brighton
«The useless Boy», Oteng, Obone, Pricila, Lynette, Haward, Defaru,
Lintwe;
I am truthfully grateful and express my thanks.
EPIGRAPHS
Oh God! Make me strong to overcome my weaknesses,
and Blessed be Your Name forever and ever. Amen
Dear Parents, My gods! Despite your insufficiencies, You
showed me the way to School. I have understood your divinity! Thanks for
your Love and Sacrifices.
Josué B. Chishugi
ABSTRACT
During the last decade African continent has been
characterised by a shortage of water, electricity and food due probably to
climatic change and mismanagement of the natural resources. The political
stability in Central Africa region, the progress in industrial development in
southern and the northern Africa gradually increase the need in water for
domestic, agricultural, industrial and environmental uses. The second longest
river in Africa, contributing with 30% to the African discharge to the Atlantic
Ocean, the Congo contains the second largest forest in the world, after the
Amazon, sustains the global climate change regulation and ecosystem stability;
therefore, the understanding of its hydrology is of grand importance.
In order to understand and evaluate the spatial and temporal
distribution of the Congo River Basin (CRB) water balance, a distributed
GIS-based hydrological model, namely Hybrid Atmospheric and Terrestrial Water
Balance (HATWAB) initially developed by Alemaw (2006) was parameterised and
applied to the Congo basin using Rainfall, Potential Evapotranspiration, soils
and vegetation information. The model simulates the Soil-water balance model
component, namely the Integrated Vertical Moisture Convergence (C), soil
moisture (SM), Actual Evapotranspiration (AET) and Runoff (ROF). The spatial
distribution of the simulated components correlate strongly with Rainfall
patterns, especially in the high rain fed region (Effective Rainfall >1100
mm/year), corresponding to the central part of the equatorial forest, and
extending between 5 and -5 degrees of latitude; whereas some disturbances are
observed in the lowest rain fed (Effective Rainfall <1000 mm/year) regions
of the basin located the south-eastern and the up-north part of the CRB. The
Evapotranspiration Ratio (ETR) shows two main climatic regions, ETR close to 1
for region 1 and ETR<7 for region 2, with an intermediate zone between
(07<ETR<0.8). The Annual average SM varies between 0 and 400 mm while the
actual Evapotranspiration (AET) varies between 400 and 1700 mm/year with
highest values on the water bodies. The based-wide gridded runoff (ROF) varies
between 0 and 1400 mm/annum with a annual average of 324 mm/a. Flooded wetland
areas and swamps are characterised by 0 to 40 mm/a values while highest runoff
(> 900mm/a) are computed on the rivers and lakes. The inland grid ROF varies
between 50 and 900 mm per year with an average of 324 mm/year. The accumulated
ROF computed at the CRB outlet reaches 44700m3/sec, which is within 5% of
marginal error compared to the observed discharge of the Congo River.
A supporting script tool (DEMHydro) was developed to extracts
the topographic, topologic and hydrologic characteristics of the basin, using
Digital Elevation Model (DEM) information.
TABLE OF CONTENTS
DECLARATIONS II
STATEMENT OF COPYRIGHT III
ACKNOWLEDGEMENT IV
EPIGRAPHS V
ABSTRACT VI
TABLE OF CONTENTS VII
LIST OF FIGURES XI
LIST OF TABLES XIII
LIST OF TABLES XIII
LIST OF ABBREVIATIONS, ACRONYMS AND SYMBOLS
XIV
LIST OF ABBREVIATIONS, ACRONYMS AND SYMBOLS
XIV
CHAPTER ONE 1
1.0
|
INTRODUCTION OF THE STUDY
|
1
|
1.1
|
Introduction
|
1
|
1.2
|
Statement of problem
|
1
|
1.3
|
Research objectives
|
2
|
1.4
|
Importance of study
|
2
|
1.5
|
Organisation of the thesis
|
3
|
CHAPTER TWO 4
2.0 OVERVIEW OF THE STUDY AREA 4
2.1 The Study Area 4
2.2 Physiography 5
2.3 Hydrology 6
2.4 Climate 8
2.5 Soils 10
2.6 Land cover/use and Population density 11
2.7 Geology 13
2.7.1 Basement formation 13
2.7.2 Surface formations 13
CHAPTER THREE 14
3.0 LITERATURE REVIEW 14
3.1 Hydrological models 14
3.2 Water Balance Model approaches 15
3.2.1 Atmospheric Water Balance Studies 16
3.2.2 Soil Water Balance Studies 16
3.2.3 Surface Water Balance Studies 17
3.2.3.1 Water Balances 17
3.2.3.2 Runoff Mapping 18
3.3 Potential evapotranspiration (ETp) and Effective
rainfall determination 18
3.3.1 Estimation of Potential Evapotranspiration 18
3.3.1.1 Net radiation 19
3.3.1.2 Mean Relative Humidity 20
3.3.1.3 Wind speed 20
3.3.1.4 Solar radiation 20
3.3.2 Estimation of Effective Rainfall 21
CHAPTER FOUR 23
4.0 METHODOLOGY 23
4.1 Watershed and streams characteristics 23
4.2 Watershed and drainage network Processing Method
23
4.3 DEM-Hydro processing output maps 25
4.3.1 DEM Visualization and areal distribution over elevation
25
4.3.2 Flow direction map 27
4.3.3 Flow accumulation 28
4.3.4 Drainage network extraction and ordering 29
4.3.5 Catchment and Sub-Catchments extraction 30
4.3.6 Overland Flow map 32
4.4 Watershed characteristics 33
4.4.1 Watershed Geomorphology 33
4.4.1.1 Area and length 33
4.4.1.2 Watershed Shape 35
4.4.2 Morphometric Analysis 35
4.4.2.1 Morphometric network topology
35
4.4.2.2 Horton morphometric parameters
36
4.5 GIS-Based Hydrological Model Development
41
4.5.1 Introduction 41
4.5.2 Water Balance Model development procedure 43
4.5.3 Water Balance Model Development 43
4.5.3.1 Atmospheric water balance 43
4.5.3.2 Terrestrial water balance 44
4.5.3.3 Imbalance estimation 45
4.5.3.4 Rainfall-Actual Evapotranspiration-Soil
moisture-Runoff modelling 45
4.5.4 Data sets and software 48
4.5.4.1 GIS and geo-referencing procedure 48
4.5.4.2 Meteorological data sets 48
4.5.4.3 Discharge data 50
4.5.4.4 Digital Elevation Model (DEM) and Mask files
50
4.5.4.5 NDVI and vegetation database 51
4.5.4.6 Soil properties 51
4.5.4.7 Software resources 55
CHAPTER FIVE 56
5.0 MODEL APPLICATION, DATA PRESENATTION AND
INTERPRE-TATION RESULTS 56
5.1 Generalities on the Model application 56
5.2 Initial soil moisture 56
5.3 Data presentation and Interpretation results
57
5.3.1 Soil moisture (SM) 57
5.3.2 Actual Evapotranspiration (AET) 59
5.3.3 Runoff 60
5.3.4 Simulated sub-watershed and basin-wide runoff 62
5.3.5 Vertical Integrated Moisture Convergence 65
CHAPTER SIX 67
6.0 CONCLUSIONS AND RECOMMENDATIONS 67
6.1 Conclusions 67
6.2 Recommendations 68
REFERENCES 69
APPENDICES 74
APPENDIX 1: METEOROLOGICAL STATIONS COVERING THE STUDY
AREA (FROM FAO/UNESCO CLIMWAT DATBASE) 75
APPENDIX 2: SPREADSHEET MODEL FOR THE PENMAN-MONTEITH
CALCULATION METHOD OF ETO (AFTER ALLEN ET AL, 1998) 79
APPENDIX 3: CONGO RIVER DISCHARGE DATA AT KINSHASA
82
APPENDIX 4: ATTRIBUTE TABLE FOR DRAINAGE NETWORK ORDERING
84
APPENDIX 5: HORTON STATISTICS FUNCTIONALITY: DEFINITION
OF PARAMETERS 85
APPENDIX 6: HORTON MORPHOLOGICAL PARAMETERS AND
STATISTICS FOR SUBSEQUENT STRAHLER ORDER 86
APPENDIX 7 PEARSON PRODUCT MOMENT CORRELATION BETWEEN
HORTON AND GIUH 87
APPENDIX 8.A. LOCAL WATER BALANCE FOR SELECTED GRID CELLS
IN THE CONGO RIVER BASIN (TABLE) 88
APPENDIX 8.B. LOCAL WATER BALANCE FOR SELECTED GRID CELLS
IN THE CONGO RIVER BASIN (GRAPHS) 90
APPENDIX 9: SEASONAL DISTRIBUTION OF THE CONVERGENCE
MOISTURE SAMPLES FOR SELECTED GRID CELLES OVER THE CONGO BASIN (C)
91
LIST OF FIGURES
Figure 1 General Location Map: Position of the study area in
Africa 4
Figure 2 The Congo River Basin Elevation System. The high est
station elevation is located in the Tanzanian region while the lowest, at the
Atlantic Ocean (Note: this elevation grid is derived
from the elevation of the selected 145 meteorological
stations falling inside the study area) 6
Figure 3 Mean Discharge Regime of the Congo River Basin at
the Kinshasa gauge 7
Figure 4 Monthly discharge of the Congo River (Kinshasa
gauge). Mean 1960-1990 7
Figure 5 Meteorological profile of D.R.Congo 9
Figure 6 Long-term mon thly average of Effective Rainfall
(1961-1990) at grid cell. 9
Figure 7 Effective rainfall distribution for three selected
grids in the Area 10
Figure 8 Congo Basin Agronomic Soils Map. The polygon limit
the Congo watershed 11
Figure 9 Vegetation and Land cover and uses over the Congo
River Watershed (after World river resources, 2003) 12 Figure 10
Population density distribution over the Congo River Watershed: Basin area
3,730,881 sq.Km, Average Population Density (people per
sq.km): 15, Number of large
cities (100,000
people). 12
Figure 11 Hydrological Model Classification 14
Figure 12 DEM Processing flow chart: Extraction of Drainage
network, Catchment and Horton
Parameters. 24
Figure 13 Areal distribution at different altitude (The area
in a logarithmic scale) 25
Figure 14 DEM visualization map for Cental Africa. The
defined colored polygone delineated the Congo River basin.
26 Figure 15 D-8 algorithm: Based on the output Flow direction map,
the Flow accumulation operation counts the total number of pixels that will
drain into outlets (after ILWIS 3.4 Manual)
27
Figure 16 Flow direction map 27
Figure 17 Histogram of Flow Direction for Central Africa
28
Figure 18 Flow Accumulation map; on top: Entire basin, on
bottom: A selected area 29
Figure 19 Stream network map masked by the boundary of the
Congo River Basin 30
Figure 20 Extracted sub-catchment map in the Congo Basin
31
Figure 21 Merged sub-watershed with stream network and majors
outlet of the CRB 31
Figure 22 Longest flow path map overlayed on the
sub-watersheds of the CRB 32
Figure 23 Overland flow distribution in the study area
32
Figure 24 Overland flow distribution in the Ouesso
sub-watershed 33
Figure 25 Horton morphometric parameters for 4 selected
sub-watersheds in the Congo River
37
Figure 26 Strahler order vs. Stream length map 39
Figure 27 General terrestrial Water Balance model structure
42
Figure 28 Rainfall-Runoff simulation model for a single grid
cell 42
Figure 29 Functional relationship between soil moisture and
Evapotranspiration (ETa is the actual Evapotranspiration, ETp is the potential
Evapotranspiration, SM is the soil moisture, FC is the field capacity and WP,
the Wilting point 47 Figure 30: Distribution of Clima tic stations
in the study area. The study area covers more than
145 stations 49 Figure 31 Rainfall averaged
(1961-190) data from 145 stations. 1. Rainfall, 2. Effective Rainfall
49
Figure 32 Mean Annual Potential Evapotranspiration
(1961-1990) map 50 Figure 33 Available soil water vs. soil texture
showing estimates of field capacity, permanent wilting point and Available
water content. S-Sand, SI-Silt, CL-Clay, F-Fine, VF-Very Fine, L-
Loamy (after Levy et al, online) 53
Figure 34 Hydrological Soil types over the basin 54
Figure 35 Soil Field Capacity in the root zone. 54
Figure 36 Hydrological Soil types over the basin 55
Figure 37 Soil moisture correlation with the latitude
57
Figure 38 Mean annual moisture (in mm) over the Congo basin.
58
Figure 39 Season Soil moisture (in mm per season) over the
basin. 58
Figure 40 Mean Annual Actual Evapotranspiration over the
Congo River basin 59
Figure 41 Season Actual Evapotranspiration over the Congo
basin 60
Figure 42 Mean annual runoff over Congo basin (mm/year)
61
Figure 43 The relationship between precipitation and drigged
simulated runoff in the CRB 61
Figure 44 Seasonal Runoff grid runoff maps. Top left:
December-February, Top right: MarchMay, Bottom Left: June-August, Bottom right:
September-November 62 Figure 46 Seasonal and Spatial distribution
maps for Vertical Integrated Moisture Convergence (in mm/month) over the Congo
River basin. A: December-February, B: March-May, C: JuneAugust, D:
Septembre-November (Negative values correspond to the moisture
convergence,
positive values correspond to the moisture divergence).
65
LIST OF TABLES
Table 1 River discharge at KINSHASA gauge (after Vorosmarty
et al, 1998) 7
Table 2 Effective Rainfall distribution in the Congo Basin
9 Table 3 Land cover distribution in the Congo River basin (after
World river resources, 2003).... 11
Table 4 Summarised Statistics for the DEM 26
Table 5 Summarised statistics for the Flow direction grid map
in the area of study. 28
Table 6 Sub-wateshed characteristics of the CRB 34
Table 7 Extra cted sub-watersheds areas of the CRB 34
Table 8 Stream numbers and Bifurcation Ratio for
sub-watersheds of the Congo River 36
Table 9 Horton Morphometric Parameters for the sub-catchments
in the Congo River 38
Table 10 Stream Length Ration for the different
sub-catchments in the Congo River 38
Table 11 Stream Ration for the selected subwatershed
39
Table 12 Drainage density for watersheds of the Congo River
40
Table 13 Rooting depth assigned for various soil textures and
SCS soil groupings 51
Table 14 Soil texture distribution in the Congo River basin
51
Table 15 Relationship linking vegetation class, soil texture,
rooting depth and moisture
capacities of various soil groups in Central Africa (Source:
Alemaw and Chaoka, 2003) 53
Table 16 Subwatershed runoff averages 63
LIST OF ABBREVIATIONS, ACRONYMS AND SYMBOLS
AET actual evapotranspiration
(ea-ed) Vapour pressure deficit (kpa)
AfDB African Development Bank
ASCE American Society of Civil Engineering
AWC Democratic Republic of the Congo
AWF African Water Facility
C Vertical Integrated Moisture Convergence
C.R.B Congo River Basin
C.V.I Vertical Integrated Moisture Convergence
CICOS International Commission of the Congo-Oubangi-Sangha
River Basin
CLIMWAT Cliamtic Database
Slope vapour pressure curve (kPa oc-1)
D.R.C. Democratic Republic of the Congo
DEM Digital Elevation Model
DRO Direct Runoff
dS/dt Change of storage with time
ed actual vapour pressure (Kpa)
EPPT Effective Precipitation
EROS Earth Resources Observation Systems
ET Evapotranspiration
ETo Reference crop evapotranspiration
ETp Potential Evapotranspiration
ETR Evaptranspiration ratio
ETref Reference Evapotranspiration
FAO Food Agricultural Organization
FC Field Capacity
G soil heat flux (MJ m-2 d-1)
GCM General Climate Model
GIS Geographic Information System
GIUH Geomorphological Instantaneous Unit Hydrograph
GW Giga Watt
HATWAB Hybrid Atmospheric and Terrestrial Water Balance
HYDROSHEDS Hydrological data and maps based on SHuttle
Elevation
Derivatives at multiple Scales
ILWIS Integrated Land and Water System
Imb Imbalance
IRD International Research Development
ITCZ Inter-Tropical Convergence Zone
L T-1 Length over Time
LAI Leaf Area Index
LDP Longest Drainage Path
LHSOr Lengnth of the Hisghest Strahler Order
LFP Longest flow path
mm millimeter
N Day length
n Day sunshine
n /N relative sunshine fraction
n/a Non applicable
NDVI Normalized Difference Vegetation Index
NEPAD New Partnership for Africa's Development
NOAA-AVHRR National Oceanic and Atmospheric Administration-
Advanced Very High Resolution Radiometer
npix Number of Pixels
npixcum Cumulative Nmber of Pixels
npixpct percentage of number of pixels
P Precipitation
PET Potential evapotranspiration
Q Discharge
Q Water Vapour flux
Qpg Peak discharge
R Runoff
Rn Net radiation at crop surface (MJ
m-2 d-1)
Rnl net longwave radiation (MJ m-2
d-1)
Rns net short wave radiation (MJ m-2
d-1)
RO Runoff
ROF Runoff
SADC Souther Africa Development Community
SCS-CN Soil Conservation Service - Curve Numbers
SM Soil moisture
T Average temperature (oc)
Tkn Minimum temperature (K)
Tkx maximum temperature (K)
Tmonth n Mean temperature in month n ( 0C)
Tmonth n Mean temperature in month n-1 (
0C)
Tpg Time to Peak Discharge
TRO Total Runoff
U2 Windspeed measured at 2m height (m s-1)
U2 Windspeed measured at 2m height (m/s)
UFC Unit field Capacity per unit volume of Soil
UH Unit Hydrograph
UNEP Uneted Nations Environment Programme
USGS Unit field Capacity per unit volume of Soil
UWP Unit Wilting Point per unit volume of Soil
WHC Water Holding Capacity
WP Wilting Point
? Psychometric constant (kPa oC-1)
CHAPTER ONE
1.0 INTRODUCTION OF THE STUDY
1.1 Introduction
The Congo drainage basin is situated in Central Africa. Its
hydrological system straddles several countries; Congo and the Democratic
Republic of Congo for the most part, but also Angola, Cameroon, the Central
African Republic, Zambia and Tanzania, stretching through Lake Tanganyika. The
River Congo, the second longest African river after the Nile, second in the
world, after the Amazon, in terms of discharge, itself accounts for half the
total volume of waters which pour into the Atlantic from the Africa continent.
An understanding of how the hydrological system works is indispensable now at
the start of a new century when water is such a crucial issue, especially in
Africa.
1.2 Statement of problem
The Congo basin, just like in the whole of Africa, and
especially in the North of the continent, has been hit by a period of drought
which started to bite in the second half of the 20th century. The
drop in rainfall appeared first in a sub-basin of the Congo, that of its main
tributary the Oubangui, since 1960. Precipitation decreased by 3% between the
two periods 195 1-1959 and 1960-1989. In other sub-catchments (Shangha, Kouyou,
further South), rainfall Figures began to decrease 10 to 13 years later. For
the Congo Basin as a whole, comparison of data for the periods 1951-1969 and
1970-1989 revealed a rainfall loss of 4.5% (IRD, 2002).
As for discharge, data indicated a series of four distinct
phases in the Congo and the Oubangui since the beginning of the 20th
century. During the 1960s they increased, overtaking their average over a
century. The Congo discharge then fell, returning in 1970 to what had been the
normal level, whereas the Oubangui entered a drought phase. This trend
accentuated from 1980 and, until 1996, the Congo discharge decreased by 10% (37
400 m3/s in 1992 compared with an average of 40 600 m3 /s
over that period as a whole), which was the most dramatic decrease of the
century. This fall is much stronger in the Oubangui (- 29%), yet negligible (-
0.2 %) in the Kouyou sub-basin. Overall, whereas discharge decrease in the
Congo Basin is between two and four times the drop in rainfall, it is nine
times that in the Oubangui (IRD, 2002).
Great differences in discharge reduction are therefore evident
between the different rivers in the Congo system, in spite of a generalized
drought which has been prevailing over the entire region. What can explain
this? The researchers have brought evidence here of the strong influence of
soil geology on the effect a change of rainfall has on river discharge. The
various sub-catchments here indeed differ widely in their geology. In the
North, the Oubangui subbasin is a ferruginous cuirassed peneplain which favours
water runoff. Further South, the Shangha sub-basin has a sandy soil where the
land becomes partly flooded during periods of heavy rainfall. The third, nearer
the river mouth, is where the Kouyou sub-basin borders the Bak't's plateaux
consisting of sandstones. These are porous and permeable, aquifers capable of
storing an excess of water. The drought has not had the same impact in the
three regimes. The nature of the geological substrate of the Oubangui sub-basin
amplifies considerably any
variation in rainfall; between 1982 and 1993, a 3% drop in
rainfall induced a 29% loss of discharge. Conversely, the sandy soils of the
Kouyou sub-catchment have a stabilizing effect, in that they store or release
water. During the humid period of the 1960s, excess water from heavy
precipitation entered them and was held there. When drought followed, the water
was released. The decrease in rainfall in this area is about 5.3% but the
effect on the discharge is 26 times smaller: it has diminished by only 0.2%.
The Congo River history shows the emphasis of conducting
details researches since its behaviour can vary in the future while the whole
world is showing its interest the benefit from the Congo Rivers resources in
different domains like hydroelectric power, agriculture, water supply, trade of
wood and other green businesses, and so on. The World Bank, NEPAD, SADC and
many other international organizations are showing their interest to invest in
the hydroelectric power sector, agriculture and water supply in this area.
Its size and the diversity of its tributaries provide the
Congo Basin with an overall stability against variations in rainfall. If the
balance of the hydrological regime of the main river was as delicate as that in
the Oubangui sub-basin, it is easy to imagine the consequences the recent
drought would be having on this region of Africa in particular and the whole
world in general. Therefore, the water balance study of the individual
watershed is requested for the better use of these resources. This study will
bring a more accurate picture of water availability in the Congo River Basin
(CRB) as well as its spatial and temporal distribution through modelling of the
water budget.
1.3 Research objectives
The main objective of is study is to understand and to model the
hydrological budget and processes in the Congo River Basin.
The specific objectives are as follows:
· To study the hydromorphologic characteristics of the
Congo basin based on Digital Elevation Model (DEM) processing techniques
· To determine the available spatial and temporal
hydro-climatic information and data gaps in order to undertake a GIS-based
water budget study in the Congo River Basin.
· To assess the spatial and temporal variability of water
balance components namely, soil moisture, actual evapotranspiration and runoff
in the Congo river basin.
· To map the spatial and temporal variability of
rainfall, effective rainfall, potential and actual evapotranspiration, soil
moisture, runoff and Vertical Integrated Moisture Convergence in Congo River
Basin
1.4 Importance of study
The hydrological cycle of the Congo River Basin is of great
importance as the region plays an important role in the functioning of
regional and global climate. Variations in regional water and energy balance
at year-to-year and longer time scales are of special interest, because
alterations in circulation and precipitation can ultimately
translate changes in the streamflow of the Congo River Basin. In addition,
these changes can also affect the atmospheric moisture transport from the Congo
River Basin to adjacent regions.
With a discharge of be 41,800m3/s; the Congo River
contributes for itself with about 30 % of the water inflow to the Atlantic
Ocean from the African continent. In 1980, its contribution was estimated to
41.1% (Olivry et al, 1993).
The Congo is the biggest means of transportation in Central
Africa with more than 14,500 km of navigable channel/rivers across Central
Africa. The Congo River has enormous hydroelectricity potential that can supply
the whole African continent. It represents more than one-sixth of the world's
known resources and remains un-exploited.
In February 2005, South Africa's state-owned power company,
Eskom, announced a proposal to increase the capacity of the Inga Dam
dramatically through improvements and the construction of a new dam and
hydropower plant. The project would bring the maximum output of the facility to
40 GW, twice that of China's Three Gorges Dam (UNEP, 2006).
In June 2007, the African Development Bank (AfDB) signed two
agreements with the International Commission of the Congo-Oubangi-Sangha River
Basin (CICOS) amounting to 2.44 million euros from the African Water Facility
(AWF) to finance programmes aimed at improving the integrated management of
Congo River Basin. The two agreements were hailed as a significant event of
engagement of the African Water Facility to support the objectives of creating
an enabling environment for sustainable water resources management of the Congo
River Basin with a view to bringing about socio-economic development and
environmental wellbeing for the benefit of countries sharing the water
resources in particular, Africa in general (Allafrica, 2007).
The water balance model to be developed will provide a reasonable
solution to large scale hydrological problems associated with planning and
optimal management of the resources in the catchment.
1.5 Organisation of the thesis
Including the introductory chapter one, this thesis is subdivided
in eight Chapters. General overviews of each chapter are given below.
Chapter two details the overview of the study area, mainly
including the location of the study area, physiography, hydrology, climate,
vegetation, geology and soils, geology, soils, and land cover-uses and
population density.
Chapter three summarises literature review of the study.
Chapter four concerns the methodology: watershed and streams
extraction method and the soil-water balance method are intensively
detailed.
Chapter five details the model application, data presentation and
interpretation of the results; and finally, conclusions and recommendations are
presented in chapter six.
CHAPTER TWO
2.0 OVERVIEW OF THE STUDY AREA
2.1 The Study Area
The study area comprises The Congo River Basin, bounded
between Latitude 100 and 150S and between longitude
100 and 350 degree East (Figure 1). This area covers
several countries in Africa: Democratic Republic of the Congo (DRC), the
People's Republic of the Congo, the Central African Republic, and partially
through Zambia, Angola, Cameroon, and Tanzania. The Congo River (also known as
the Zaire) is over 4,375 km long. It is the fifth-longest river in the world,
and the second longest in Africa - second only to the Nile River in
North-eastern Africa. The Congo ranges in width from 0.8 to 16 km depending on
the location and time of year (The Living Africa, 1998).
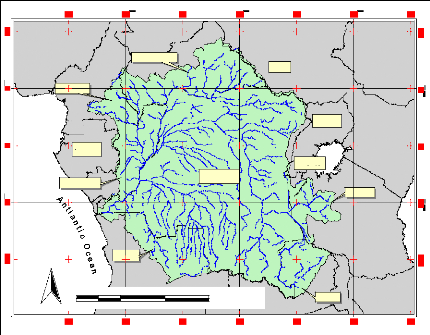
N
Cameroon
Congo Rep.
500 0 500 1000 Kilometers
Gabon
Angola
Central Africa
D.R.Congo
Sudan
Rwanda
Uganda
Zambia
Ta
nzania
Figure 1 General Location Map: Position of the study
area in Africa
The Congo River forms in the southern-most part of the DRC
where the Lualaba and Luvua Rivers meet, then flows to Stanley Falls, near
Kisangani, a point just north of the Equator before taking on a counter
clockwise course. The Congo loops first to the northeast, then to the west, and
then to the south before reaching an outlet into the Atlantic Ocean, feeding a
river
basin that covers over 4.1 million km2. At the outlet
into the Atlantic Ocean, the Congo discharge up to 34,000 m3/s of
water per second (The Living Africa, 1998).
Within the Congo's banks can be found over 4,000 islands, more
than 50 of which are at least 10 miles (16 km) in length. It is because of
these islands that some stretches of the Congo are not navigable. It has been
estimated that almost 400 km of the Congo are not navigable due to these
islands plus a number of cataracts, in particular at Livingstone Falls.
2.2 Physiography
The Congo basin is the most clearly bounded by various
geographic depressions situated between the Sahara to the north, the Atlantic
Ocean to the south and west, and the region of the East African lakes to the
east (Britanica, 2007). Tributaries flow down slopes that vary from 274.3 m to
457.2 m into a central depression that forms the basin. It measures more than
193 1.2 km north to south (from the Congo-Lake Chad watershed to the Angolan
plateaus). West to east - from the Atlantic to the Nile-Congo watershed - it
also measures 193 1.2 Km (Butler, 2006).
The Congo basin has a large depression in the central portion.
Referred to as a "cuvette", it is a large, shallow, saucer-shaped area. This
depression contains Quaternary alluvial deposits which rest on thick sand and
sandstone sediment of continental origin. Along the eastern edge of the cuvette
outcrops of sandstone are formed. The cuvette has a filling that dates to
Precambrian times (570 million years ago). Studies have shown that the sediment
has built up over time from the erosion of the formations that surround the
cuvette. The Congo River system is composed of three distinct sections - the
upper Congo, the middle Congo and the lower Congo.
Kisangani is situated downstream from the Boyoma Falls and is
at the beginning of where the Congo River becomes navigable. For 1000 miles
(1610 km) the river flows towards Kinshasa. At first the river is narrow but
soon widens as it enters the alluvial plain. From the point where the river
widens, strings of islands occur which divide the river into different forms.
The width of the Congo River can vary from 3.5 miles to 7 miles, reaching up to
8 miles at the mouth of the Mongala River. Along the banks of the river are
natural levees which have been formed by deposits of silt. When the river
floods these levees are washed away and the river banks increased in width.
The middle Congo is characterized by the narrowing of the
river. The banks are a half-mile to a mile apart, the river is much deeper and
its current is high. This section of the Congo is referred to as the Chenal
(Channel) or Couloir (Corridor). It is along this stretch of the river that its
principal tributaries flow into the Congo. They include the Ubangi River,
Sangha River and the Kwa River.
This results in a tremendous increase in the flow of water from
7079 m3/s at Kisangani to its maximum when it reaches Kinshasa
NE
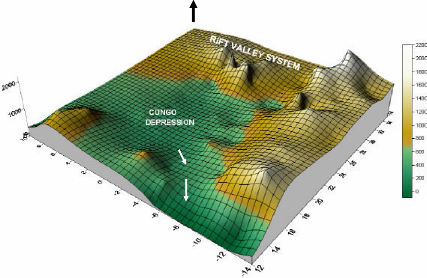
Figure 2 The Congo River Basin Elevation System. The highest
station elevation is located in the Tanzanian region while the lowest, at the
Atlantic Ocean (Note: this elevation grid is derived from the elevation of the
selected 145 meteorological stations falling inside the study area)
From the middle Congo (Chenal) the river divides into two. One
branch forms Malebo Pool, which is 24 mile by 27.3 miles large. This is the end
of the middle Congo. Just downstream are the first of 30 waterfalls as the
river continues to flow towards Matadi. At Matadi, the Congo's estuary begins
in a narrow channel only half a mile to a mile wide. Eventually it widens below
Boma but islands are once again a factor, dividing the river into several
forms. The Congo now flows freely into the Atlantic Ocean.
2.3 Hydrology
The Congo has a regular flow, which is fed by rains throughout
the year. As recorded at Kinshasa, the flow has for years remained between the
high level of 65411.92 m3/s, recorded during the flood of 1908, and
the low level of 21407.54 m3/s, recorded in 1905. During the unusual
flood of 1962, however, by far the highest for a century, the flow probably
exceeded 73623.8 m3/s (Encyclopedia Britannica, 2007). At Kinshasa,
the river's regime is characterized by a main maximum at the end of the year
and a secondary maximum in May, as well as by a major low level during July and
a secondary low level during March and April (Figure 4, Table 1). In reality,
the downstream regime of the Congo represents climatic influence extending over
20° of latitude on both sides of the equator, a distance of some
2253km.
The Congo River's flow and water levels are affected by the
rains all year round. It is the effects of rainfall throughout the regions
whose rivers and tributaries contribute to the Congo River that influence the
fluctuations in the flow of the river. However, because the Congo basin has an
immense area, the weather pattern in one particular region will not have much
effect on the river's overall levels. For example, heavy rainfall in the
northern areas that contribute to the
Table 1 River discharge at KINSHASA gauge (after
Vorosmarty et al, 1998) Station: Kinshasa, Latitude: 4.3o S/
Longitude: 15.3o E, Elevation: River: Zaire, Country: Congo D.R., Area:
3475000 km2
-
|
Jan
|
Feb
|
Mar
|
Apr
|
May
|
Jun
|
Jul
|
Aug
|
Sep
|
Oct
|
Nov
|
Dec
|
Ann
|
m 3 /s
|
47494
|
37649
|
34713
|
37172
|
39150
|
36717
|
31703
|
31087
|
36366
|
43172
|
51708
|
56082
|
40251
|
mm
|
36.6
|
26.4
|
26.8
|
27.7
|
30.2
|
27.4
|
24.4
|
24
|
27.1
|
33.3
|
38.6
|
43.2
|
366
|
km 3
|
127
|
91.9
|
93
|
96.3
|
105
|
95.2
|
84.9
|
83.3
|
94.3
|
116
|
134
|
150
|
1270
|
l/s/km 2
|
13.7
|
10.8
|
9.99
|
10.7
|
11.3
|
10.6
|
9.12
|
8.95
|
10.5
|
12.4
|
14.9
|
16.1
|
11.6
|
%
|
9.83
|
7.79
|
7.19
|
7.7
|
8.11
|
7.6
|
6.56
|
6.44
|
7.53
|
8.94
|
10.7
|
11.6
|
100
|
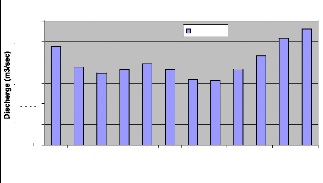
40000
60000
20000
50000
30000
10000
0
Jan Feb Mar Apr May Jun Jul Aug Sep Oct Nov Dec
Month
Mean Discharge Regime (1903-1983)
Discharge
Figure 3 Mean Discharge Regime of the Congo River Basin
at the Kinshasa gauge
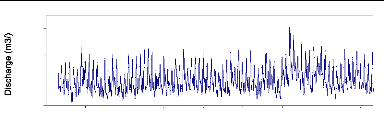
40000
20000
80000
60000
Jan-00 Jan-10 Jan-20 Jan-30 Jan-40 Jan-50 Jan-60 Jan-70 Jan-80
Time
Monthly Discharge at Kinshasa gauge (Mean 1903-1
983)
Figure 4 Monthly discharge of the Congo River (Kinshasa
gauge). Mean 1960-1990
Patterns have been established in the past and the river can be
expected to have higher levels in December and May due to the rainy season.
The levels are expected to be low in March and
April and even lower in July in response to the dry season. If
some of the weather patterns change drastically, resulting in floodwaters
arriving at the same or different times, then the anticipated water levels are
affected accordingly.
2.4 Climate
The Congo basin is located in the equatorial belt. This
location ensures that different parts of the Congo basin receive substantial
rainfall throughout the year; with a decreasing trend of rainfall with
latitude. The northern and central portions of the basin have two major
rainfall seasons which begin in March and October each year (Kazadi, 1996). The
northernmost points of the basin, situated in the Central African Republic,
receive 8 to 406.4 mm during the course of a year, which is less than the
average near the equator; the dry season, however, lasts for four or five
months, and there is only one annual rainfall maximum, which occurs in
summer.
In the south, the two rainfall seasons gradually merge into a
single season beginning in December and lasting for six months each year. In
the far southern part of the basin -- at a latitude of 12° S, in the
Katanga region -- the climate becomes definitely Sudanic in character, with
marked dry and wet seasons of approximately equal length and with mean rainfall
of about 1245 mm a year.
The rainfall peaks are associated with the passage of the
Inter-Tropical Convergence Zone (ITCZ), which is a large zone of low pressure
caused by excessive heating from an overhead sun. During the northern summer,
the midday sun is directly overhead in the tropical regions of the Northern
Hemisphere. This results in higher temperatures and consequently, lower air
pressures at the surface. Moist air flows from the oceans towards these low
pressure areas. The moisture is released as rainfall on the land surface when
the air is forced to rise on entering the convergence zone or by orographic
effects. The ITCZ causes heavy rainfall in the areas it passes over as it moves
north and south between the tropics during the respect northern and southern
summers. The Congo basin is thus representative of a large river basin in which
the spatial distribution of input varies significantly with time.
Figures 6 bellow shows the monthly distribution of rainfall,
Evapotranspiration and temperature for 8 stations over the Conog Basin. Figure
7 and Table 2 give the evective rainfall for three virtual stations located in
the southern hemisphere, northern and the center of the basin.
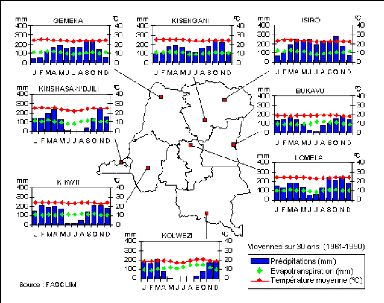
Figure 5 Meteorological profile of
D.R.Congo
Table 2 Effective Rainfall distribution in the Congo Basin
Long
|
Lat
|
Dec
|
Jan
|
Feb
|
Mar
|
Apr
|
May
|
Jun
|
Jul
|
Aug
|
Sep
|
Oct
|
Nov
|
12
|
-14
|
32
|
30.1
|
40.4
|
75.6
|
44.6
|
1.8
|
-0.5
|
-1.2
|
-2.8
|
-5.1
|
0.7
|
32
|
23.58
|
-2
|
131.3
|
113.6
|
104.2
|
129.9
|
126.3
|
106
|
57.1
|
61.7
|
99.4
|
137.6
|
139.2
|
131.3
|
35
|
10
|
5.8
|
11.9
|
12.7
|
43.5
|
92.7
|
114.8
|
84.7
|
115.5
|
129.8
|
121.1
|
98.7
|
5.8
|
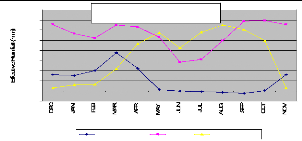
160
140
120
100
-20
40
80
60
20
0
Lowest station Center Upper Station
Grid: Long. 23.58, Lat. -2.00
Months
Figure 6 Long-term monthly average of Effective
Rainfall (1961-1990) at grid cell.
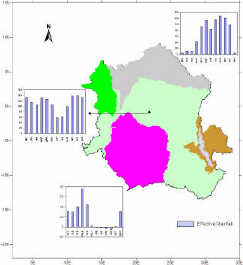
Figure 7 Effective rainfall distribution for three
selected grids in the Area
2.5 Soils
Generally, there are two types of soils in the study area:
those of the equatorial areas and those of the drier savanna (grassland)
regions. The equatorial soils occur in the warm, humid lowlands of the central
basin, which receive abundant rainfall throughout the year and are covered
mainly with thick forests. This soil is almost fixed in place because of the
lack of erosive forces in the forests. In the shore areas, however, swamp
vegetation has built up a remarkably thick soil that is constantly nourished by
humus, the organic material resulting from the decomposition of plant or animal
matter. Although in the savanna regions the soils are constantly endangered by
erosion, the river valleys contain rich and fertile alluvial soils. Special
note should be made of the highlands of eastern Congo in the Great Lakes
region, which are partly covered with volcanic lava that has been transformed
into exceptionally rich soil.
An agronomic soil map at 10X10 spatial resolutions (Figure 1
1) is available at FAO-UNESCO database (1984). This map can be resampled and
reclassified according to the objectives of the research. In Hydrological
modelling, a textural Soil map is required to derive soil retention properties
such as field capacity, wilting point, available water content, etc. For the
southern Africa, Alemaw and Chaoka (2003) demonstrate the usefulness of the
agronomic soil data in deriving soil texture classes for hydrological
modelling.
2.6 Land cover/use and Population density
The land cover pattern of the Congo basin is viewed in Figure9
and summarised in Table 3. Mostly, the basin is coverd by green and dense
forest. Dryer regions occupy less then 0.2 % of the basin. Figure 10 below
shows the population density distribution over the basin.
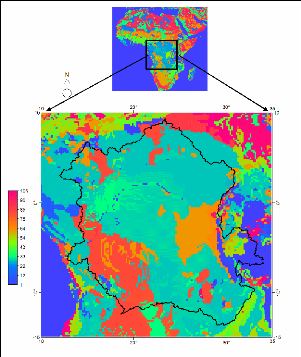
Figure 8 Congo Basin Agronomic Soils Map. The polygon
limit the Congo watershed Table 3 Land cover distribution in the Congo River
basin (after World river resources, 2003)
Percent Forest cover
|
44.0
|
Percent Grassland, Savanna and Shrubland
|
45.4
|
Percent Croplands
|
7.2
|
Percent Irrigated Cropland
|
0.0
|
Percent Dryland Area
|
0.2
|
Percent Urban and Industrial Area
|
0.2
|
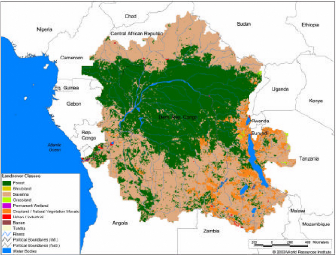
Figure 9 Vegetation and Land cover and uses over the
Congo River Watershed (after World river resources, 2003)
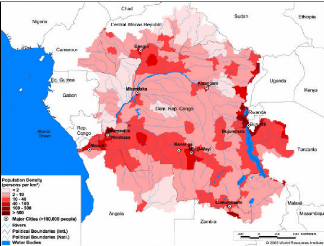
Figure 10 Population density distribution over the Congo
River Watershed: Basin area 3,730,881 sq.Km, Average Population Density (people
per
sq.km): 15, Number
of large cities (100,000 people).
2.7 Geology
The Geology of the Democratic Republic of Congo is
characterized by two large structural units separated by discordance and/or a
significant gap: The Formations of covers (Phanerozoic), not metamorphosed,
generally fossiliferous, and of age ranging between the Upper carboniferous and
the Holocene; and the Basement terrains (Precambrian shield): Highly
metamorphosed + shielded contouring continuously the basin.
2.7.1 Basement formation
The Basement terrains are subdivided in "tectostratigraphic"
units:
a) Archean shields of age equal to or higher than 2500 MA
levelling to the northern Congo and Kasaï;
b) Lower and middle Precambrian Belts (2.500 to 1.300 MA)
whose sediments settled in meridian mobile zones located on the Eastern and
Western edges of the Craton and in the intra - cratonic valleys;
c) Upper Precambrian called Katangien whose sediments settled
on the epicontinental platforms and in the «subsiding surfaces» of
the craton of Congo (Katanga folded and tabular).
2.7.2 Surface formations
The surface formations are grouped into four zones as follows:
(i) A littoral zone, ranging between the Atlantic Ocean and the
Mayumbe mounts (Crystal Mounts);marine formations tertiary and Cretacic age are
well developed there;
(ii) The central basin where the deposits of Mezoic and Cenozoic
ages spread out; vast terrains level on the circumference of the Basin;
(iii) The edge of old grounds subdivided in six unconnected
areas ;
(iv) The tectonic valley of the East of Congo occupied by
particular Cenozoic formations and characterized by recent volcanic
activities.
The formations of each one of these 4 great zones are covered
indifferently by recent formations, the ochre series of sands and the series of
the polymorphic sandstones.
CHAPTER THREE
3.0 LITERATURE REVIEW
3.1 Hydrological models
Hydrological model have been largely detailed and classified
(Figure 1) based on multiple parameters such as the model input data sets
types, and the physical characteristic of the model among others. However,
Hydrological models have, traditionally, been modelled as physically-based or
conceptual depending on the complexity and extent of completeness of the
structure of the model (Beven, 1989; Refsgaard et al., 1989;
Bergstrom, 1990; Refsgaard, 1996, 1998).
Hydrological Models
|
|
|
|
|
|
|
|
|
|
|
Deterministic
|
|
|
|
Stochastic
|
|
|
|
|
|
|
|
|
|
|
|
|
|
|
|
|
|
|
|
|
Grid based
|
|
Subwatershed
|
|
No Distribution
|
|
|
|
|
Figure 11 Hydrological Model Classification
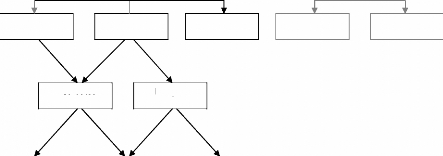
Probabilistic
Time Series
Physical Based
Distributed
Conceptual
Lumped
Empirical
In physically-based water balance models, all the physical
phenomena like precipitation, evapotranspiration, ground water inflow, ground
water outflow and storage need to be quantified and modelled. Models are
further classified into lumped or distributed, based on basin terrain
(Bergstrom and Graham, 1998). In lumped models, spatial variability in
hydrologic parameters or meteorological related data are not accounted for,
meaning that they are averaged or assumed uniform over the system, whereas, in
distributed models spatial variability is explicitly accounted for by assuming
uniformity over smaller modelling units by sub dividing the bigger system based
on physical properties. In most of the distributed hydrologic models, these
units are delineated by combining climatic components, topography, soil
properties, land use properties and other pertinent properties. Distributed
models are especially useful, for example, when impacts of land use change are
to be studied or for analyzing spatially varying flood responses (Koka,
2004). The Lumped models are easy to implement, but do not
account for terrain variability whereas Spatially-distributed models require
sophisticated tools to implement, and account for terrain variability
(Oliveira, 2002).
A statistical model derives an empirical relationship between
precipitation, infiltration, flow and any other parameters that are included in
the model. The relationship is derived based on observed data for all the
dependent and independent parameters in the model. The best relationship is
identified using suitable statistical parameters (Sukheswalla, 2003).
Large scale modeling of streamflow can be done efficiently
using simple models (Becker and Braun, 1999; and Wolock and McCabe, 1999).
Distributed models require high resolutions for efficient modeling like the
MIKE SHE model (Ewen et al., 1999) and the TOPMODEL (Beven et
al., 1994). However, for large scales such high resolution is not always
available. Also, distributed models are generally not practical and efficient
for large-scale modelling (Becker and Braun., 1999), while statistical
lumped models that fulfil large scale modelling requirements of resolution and
computation time are better (Becker and Pfützner, 1987).
Using the cell-to-cell model, a watershed can be represented
as a single cell, a cascade of n equal cells, or a network of n equal cells
(Singh, 1989). The storage in the cells is calculated as given below:
(1)
dS= -
dt
t I O
t t
where, St is the time-variant storage in a
grid cell,
It is the summation of input coming into the
cell from
upstream cells and the runoff generated in the cell, and
Qt is the outflow from the cell which is
calculated by
various methods, e.g. the linear reservoir method.
Equation (1) is a generalized water balance model which can be
applied in different situations: atmospheric, surface, soil-water, groundwater
models. The types of input variables are defined by the researcher according to
the problem. Several books, papers ad reports describing the application of
equation (1) are available, viz Thornthwaite and Mather (1957), Chow
et al (1988), Reed et al (1997) and Rasmusson (1997).
3.2 Water Balance Model approaches
In order to understand water balances, complex hydrologic
systems have always been simplified. Thornthwaite and Mather (1957)
conceptualized a Catchment water balance model for long term monthly climatic
conditions; which then led to many researches on water balance models for
similar conditions on a catchment, region or a continent. Typical examples for
these models are the GIS-based water balance model for the Southern Africa of
Alemaw and Chaoka (2003), the Grid-based model for Latin America of Vorosmarty
et al. (1989), Reed et al. (1998), the Grid -based model for Amazone
basin of Marengo (2005), the Rhine flow model of Van Deursch
and Kwadijk (1993); and the Large-scale water balance model for the upper Blue
Nile in Ethiopia of Conway (1997), and the spatial water balance of Texas (Reed
et al., 1998).
Hydrological water balance models can be based on the
interactions between the water, atmosphere, and land surface; which is a
combination of the atmospheric water balance, Surface Water Balance and the
soil Water Balance Studies.
3.2.1 Atmospheric Water Balance Studies
The estimation of hydrologic flux using Atmospheric water
balance has been studied by a number of researchers (Reed et al., 1998;
Rasmusson, 1967; Brubaker et al., 1994; and Oki et al., 1995; Marengo, 2005),
among them. Rasmusson (1967), Brubaker et al. (1994) and Oki et al. (1995)
describe atmospheric water balance at river basin, continental, and global
scales. Rasmusson (1967) analyzes the characteristics of total water vapor flux
fields over North America and the Central American Sea. Reed et al (1998)
presents the atmospheric water balance of Texas and Marengo (2005), that of the
Amazone basin.
The atmospheric branch of the water balance has always been
expressed in the form of a

simple equation of vertically integrated terms: Q
(2)
dW Q P E
r
= -?× - +
t
d
|
|
where -?×Q=C
|
expresses the vertically integrated moisture convergence,
Q is the
|
water-vapor fluxe, P is the precipitation, E is the
Evapotranspiration, and dW/dt represents the atmospheric storage
(water vapour) change term which is generally negligible for averages over a
month or more (Roads et al., 1994; Eltahir and Bras, 1994; Reed et al., 1998;
Curtis and Hastenrath, 1999; Costa and Foley, 1999; Zeng, 1999, Marengo, 2005).
Given adequate data, the atmospheric water balance is a promising method for
estimating regional evaporation, runoff, and changes in basin storage (Reed et
al, 1998).
Although the atmospheric water balance model is efficient to
estimate hydrologic flux within an area, significant uncertainties in runoff
estimation using atmospheric data exist even at the continental scale (Reed et
al., 1998). Comparing their estimated continental runoff with those given by
Baumgartner and Reichel (1975) in North America river runoff, Brubaker et
al. (1994) and Oki et al. (1995) note the fact that poorly
defined continental or basin boundaries may contribute to inaccuracies in
runoff estimation. To obtain an accurate runoff form vertically integrated
vapour flux convergence, the annual change in atmospheric water storage and
surface water storage should be negligible (Reed et al, 1998).
3.2.2 Soil Water Balance Studies
The simple bucket models have been developed by hydrologist to
simulate near-surface hydrological model in the conditions where detailed
data about soil layers, depth to
groundwater, and vegetation are not available (Reed et al,
1998). Despite numerous uncertainties associated with the simple soil-water
budget model many researchers have applied this type of model to problems
ranging from catchment scale studies to the global water balance and climate
change scenarios (Thornthwaite, 1948; Thornwaite and Mather, 1957; Manabe,
1969; Mather, 1978; Dunne and Leopold, 1978, Shiklomanov, 1983; Alley, 1984;
Willmott et al., 1985; Mintz and Serafini, 1992; Mintz and Walker, 1993, Alemaw
and Chaoka, 2003, Sen and Ambro Gieske, 2005, Marengo, 2005).
The "bucket" model approach is attractive because of its
simplicity and requires minimal input data: precipitation, potential
evapotranspiration, and soil-water holding capacity (Reed et al, 1998). The
studies by Willmott et al. (1985), Mintz and Walker (1993), and Mintz and
Serafini (1992), are climatology studies that present the global distributions
of precipitation, evapotranspiration, and soil moisture. Mintz and Serafini
(1992) compare their evapotranspiration estimates for sixteen major river
basins throughout the world with those derived from river runoff analysis made
by Baumgartner and Reichel (1975) and the values show reasonable agreement
(Reed et al, 1998).
At a smaller scale, Mather (1978), quoted by Reed et al
(1998), describes the application of a soil-water budget model to several
watersheds in the coastal plains of Delaware, Maryland, and Virginia.
Comparisons between measured and computed runoff values are rather poor for
monthly data, but better for annual data.
In its simplest form, the soil-water budget model does not
account for situations where the precipitation rate is greater than the
infiltration capacity of the soil. Mather (1978) describes one approach to
remedy this problem, that is, to first use the SCS method to estimate direct
overland runoff and substract this amount from the precipitation before it is
allowed to enter the soil "bucket." This approach appears to yield better
results. A similar approach of taking an initial rainfall abstraction before
allowing precipitation to enter the soil column for climatological budgeting
was used in a study of the Niger Basin (Maidment et al., 1996), in
southern Africa region (Alemaw and Chaoka, 2003) and in the Limpopo basin
(Alemaw, 2006).
3.2.3 Surface Water Balance Studies 3.2.3.1 Water
Balances
The commonly used method in hydrologic studies, namely the
surface water balance, relies on the fact that with the exception of coastal
areas, the landscape can often be divided into watershed units from which there
is only one surface water outflow point (Reed et al, 1998). If assumed that
change in storage is negligible and that there are no significant
inter-watershed transfers via groundwater or man-made conveyance structures,
providing that the average watershed precipitation and runoff can be measured
with reasonable accuracy, the annual evaporative losses from a watershed can be
estimated by Empirical relationships which are often used to estimate mean
annual or mean monthly flows in ungaged areas; this approach is used in this
study (Reed et al, 1998).
3.2.3.2 Runoff Mapping
Arnell (1995), Lullwitz and Helbig (1995), Reed et al (1998),
Alemaw and Chaoka (2003) and Alemaw (2006) describe studies of runoff mapping
using a geographic information system (GIS) to manage spatial data at a
regional or continental scale.
Arnell (1995) presents five approaches for deriving gridded
runoff maps at a 0.5 degree grid resolution; they include: (1) simply averaging
the runoff from all stations within each grid cell, (2) statistically
interpolating runoff between gages, (3) using an empirical relationship that
relates runoff to precipitation, potential evaporation, and temperature, (4)
using a soil-water balance type model, and (5) overlaying grid cells onto
catchment runoff maps to derive area-weighted runoff estimates. In a study
using the 5 approaches to map runoff over a large portion of Western Europe,
the results show that method (5) produces the most reasonable estimates. In a
study similar to that of Arnell (1995), Lullwitz and Helbig (1995) created
0.50 grid runoff maps for the Weser River in Germany. In both this
papers, authors noted that 0.5 degree runoff maps can be useful for validating
general circulation models (GCM's). Church et al. (1995), using an
interpolation method to create runoff maps, present maps of evapotranspiration
(ET) and runoff/precipitation (R/P) ratios for the northeastern United States.
A different approach of mapping surface runoff, similar to Arnell's method
(Arnell, 1995), combining an empirical rainfall-runoff relationship and
watershed runoff balancing was used by Reed et al (1998).
Alemaw and Chaoka (2003) developed a GIS based hydrological
model simulating the spatial and temporal distribution of water budget
parameters for the Southern Africa region. This model was slightly modified and
used for the Limpopo basin (Alemaw, 2006), where monthly runoff is generated
from matrix of specific geo-referenced grids.
3.3 Potential evapotranspiration (ETp) and Effective
rainfall determination
Potential runoff is defined the maximum possible rate at which
evapotranspiration would occur from a large area completely and uniformly
covered with growing vegetation which is not short of water under given
atmospheric conditions (Weligepolage, 2005).
3.3.1 Estimation of Potential
Evapotranspiration
A Number of approaches have been developed for estimating the
ETp or ETref based on different theoretical concepts. Most commonly applied
methods for hydrological studies can be classified into four categories on the
basis of their data requirement (Weligepolage, 2005):
a) Temperature based methods- use only daily average air
temperature and some times the day length.
b) Radiation based methods- use both the net radiation and air
temperature data for estimating ET.
c) Combination- use net radiation, air temperature, wind speed
and relative humidity data based on the Penman-Monteith combination
equation.
d) Pan measurement- use pan evaporation with modifications
depending on wind speed, temperature and humidity.
The methods that do not require information about the nature
of the surface, estimate the reference crop evapotranspiration rate where as
others are surface specific and do require information about albedo, vegetation
height, maximum stomatal conductance, leaf area index and other factors.
The American Society of Civil Engineering (ASCE) and
Consortium of European Research Institutes have undertaken major studies to
evaluate the performance of different evapotranspiration estimation procedures
under different climatologic conditions. Both have indicated that the FAO
Penman-Monteith approach of reference crop evapotranspiration as relatively
accurate and consistent performance in evapotranspiration estimation (Allen et
al, 1998).
The following paragraph gives details on the Penman-Montheith
method where the Potential evapotranspiration is considered to be the Reference
evapotranspiration:
ET=
0 Ä + +
(1 0 . 34 )
U 2
(3)
900
0. 40 8Ä
+
+
( )
R G
-
n
U e
2 ( a
- ed)
T
273
where; ETo =
|
Rn
|
=
|
G
|
=
|
T
|
=
|
U2
|
=
|
Reference crop evapotranspiration (mm/day) Net radiation at crop
surface (MJ m-2 d-1) Roil heat flux (MJ m-2
d-1)
Average temperature (oC)
Windspeed measured at 2m height (m s-1)
(ea-ed) = Vapour pressure deficit (kpa)
=? Slope vapour pressure curve (kPa
oC-1)
? = Psychometric constant (kPa oC-1) 900 =
Conversion factor
3.3.1.1 Net radiation
The Net radiation is s determined as follows;
R n =R ns -R nl
(4)
n
R = 0 .77(0 .25 + 0 . 5 ) (5)
ns Ra
N

(6)
n
nl 2.45.10 (0.9 0.1)(0.34 0.14 )( )
= + - +
9 ed T T
4 4
R kx kn
N
G = 0.14(Tmonthn - T monthn-1) (7)
where; Rn = net radiation
Rns = net short wave radiation (MJ m-2
d-1)
Rnl = net longwave radiation (MJ m-2
d-1)
n /N = relative sunshine fraction
Tkx = maximum temperature (K)
Tkn = minimum temperature (K)
ed = actual vapour pressure (kPa)
G = soil heat flux (MJ m-2 d-
1)
Tmonth n = mean temperature in month n
(oC)
Tmonth n-1 = mean temperature in preceding month n-1
(oC)
3.3.1.2 Mean Relative Humidity
The humidity expressed as saturation vapour pressure at
dewpoint temperature (mbar) has been converted to mean daily relative humidity
from maximum and minimum temperatures according to the following
relationship:
RH RH
+ ?
RH e
= =
max min
min 2 d ? ?
50 50
+ (8)
e e ?
a Tmean a T
( ) ( max) ?
Where ed = saturation vapour pressure at dewpoint temperature
(kPa) ea = saturation vapour pressure at minimum
temperature ea(Tmax) = saturation vapour pressure at maximum temperature
The saturation vapour pressure is
determined according to Teten's formula: e a 0.611exp (
17.27 T T 237.3 )
= +
|
(9)
|
Where ea = saturation vapour pressure at temperature T
(oc) 3.3.1.3 Wind speed
The original wind data expressed in m/s are convereted into
km/day according to:
U2=U 2 ×86.4 (10)
*
Where; U2 = wind speed in km/day at 2 m
height
U2 = wind speed in m/s
*
3.3.1.4 Solar radiation
As no measured data on solar radiation are available, solar
radiation has been estimated from measured sunshine hours according to the
following relationships:
n
R )
p
= (0 .25 + 0 . 5
s 100
|
R (11)
a
|
Where; Rs = solar radiation (MJ
m-2d-1)
Ra = extraterrestrial radiation (MJ
m-2d-1)
0.25, 0.5 = Angstrom coefficients
n
n = (12)
* 100
p N
Where; n = daily sunshine hours (hr)
np = daily sunshine percentage (percentage.)
N = day length (hours), depending on latitude and month of the
year.
From the computed ETo series, the 20%
exceedence probability (1 in 5 years-return period) values are estimated.
3.3.2 Estimation of Effective Rainfall
Effective rainfall is defined as that part of the
precipitation which is effectively used for evapotranspiration by the crop.
Four methodologies are given below to determine the effective rainfall:
a) Fixed percentage rainfall: effective
rainfall is calculated according to:
EPPT=a×PPT (13)
Where a, is a fixed percentage to be given by the user to account for
losses from runoff and deep percolation. Normally losses are around 10 to 30%,
thus a = 0.7- 0.9, EPPT is the effective precipitation and PPT, the total
precipitation
b) Dependable rain: based on an analysis
carried out for different arid and subhumid climates an empirical formula was
developed in FAO/AGLW to estimate dependable rainfall, the combined effect of
dependable rainfall (80% prob.exc.) and estimated losses due to runoff and
percolation. This formula may be used for design purposes where 80% probability
of exceedance is required. Calculation according to:
EPPT=0.6PPT-10;
forPPT<70mm (14)
EPPT = 0.8PPT - 24; for PPT >
70mm (15)
c) Empirical formula: The parameters may be
determined from an analysis of local climatic records. An analysis of local
climatic records may allow an estimation of effective rainfall. The
relationship can, in most cases, be simplified by the following equations:
EPPT= aPPT-b;
forPPT<Z(mm) (16)
EPPT=cPPT+d;
forPPT>Z(mm) (17)
Values for a, b, c and z are correlation coefficients.
d) USDA Soil Conservation Service Method: is
a method where monthly effective rainfall (in millimetre) can be calculated
from monthly total rainfall (in millimetre) according to the following
equations (18, 19); this mwthod is adopted whenever daily rainfall data are not
available.
EPPT = PPT(125 - 0.2PPT)/125; for
PPT 250 mm
< (18)
EPPT = 125 + 0.1PPT; forPPT >
250mm (19) The use of these methods depends on the
time scale of the model and the availability of data.
CHAPTER FOUR
4.0 METHODOLOGY
The present chapter comprises two major sections describing the
watershed and streams characteristics method of determination and the water
balance modelling method.
4.1 Watershed and streams characteristics
The concept of a watershed is basic to all hydrologic designs.
Watershed has been defined as an area of land draining into a stream at a given
location (Chow et al, 1988). In other words and usually a watershed is defined
as the area that appears, on the basis of topography, to contribute all the
water that passes through a given cross section of a stream. The surface trace
of the boundary that delimits a watershed is called a divide, and the
horizontal projection of the area of a watershed is called the drainage area of
a stream at that cross section, while the location of the stream cross section
that defines the watershed is determined by the analysis.
Since large watersheds are made up of many smaller watersheds,
it is necessary to define the watershed in terms of a point. This point is
usually the location at which the design is being made and is referred to as
the watershed «outlet». With respect to the outlet, the watershed
consists of all land area that «sheds» water to the outlet during a
rainstorm. Using the concept that «water runs downhill», a watershed
is defined by all points enclosed within an area from which rain falling at
these points will contribute water to the outlet (McCuen, 2005).
To delineate the Congo River Watershed and streams network, a
Digital Elevation Model (DEM), HYDRO 1K with 1 km of spatial resolution, later
on described, was used and processed with Geographical Information System
packages viz Integrated Land and Water System (ILWIS) and ArcGIS.
In the following section are presented different steps
followed for the DEM-Hydro watershed processing. The purpose of this chapter is
to define and hydrogeomorphologically charcterise the watershed and streams
behaviours of the Congo basin, availing useful data sets for hydrological
modellings.
4.2 Watershed and drainage network Processing Method
The detailed processing method presented bellow (Figure 12) is
a combination of techniques used in GIS ILWIS 3.4, ArcINFO 9.2 and ArcView 3.2
packages; it consists in:
1 DEM visualization
2 Flow Determination (Fill sinks, Flow direction, Flow
accumulation, Flow
Modification)
3 Variable threshold computation
4 Network and Catchment Extraction (Drainage network extraction,
Drainage
network ordering, Catchment extraction, Catchment merge
5 Compound Parameter Extraction (Overland flow length, Compound
Index
calculation)
6 Statistical Parameter Extraction (Horton statistics, aggregate
statistics, cumulative
hypsometric curve, class coverage).
DEM 1 kHYDRO 30sec

Statatistical Parameters Extraction
HORTON statistics
Cumulative Hypsometric Curve

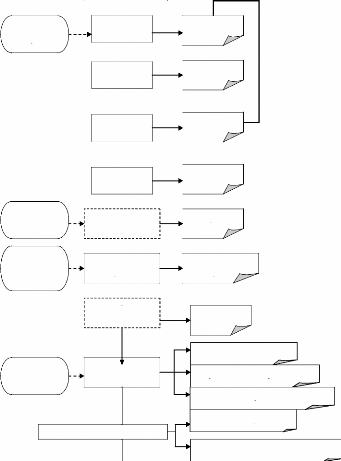
Threshold
Outlets coordinate
Digitized Drainage
Minimum Drainage
Length
Compound Parameters Extraction
Drainage Network Ordering
Catchment Extraction
Catchment Merge
Drainage Network Extraction
DEM Optm
DEM Fill Sinks
Flow Direc
Flow Accu
Drainage Network Ordering
Map
DEM Optm Map
DEM Filled Map
Flow Direct Map
Flow Accu Map
Drainage Map
Overland Flow length Map
Catchment Maps
Extract Stream Segments + Attributes
Wetness/Power/Sediment indexes Maps
Catchment Merged Map
Longest Flow Path Segment Map
Figure 12 DEM Processing flow chart: Extraction of
Drainage network, Catchment and Horton Parameters.
4.3 DEM-Hydro processing output maps
This section presents and describes the findings of the DEM-Hydro
processing technique accordingly to their application in hydrological
modelling.
4.3.1 DEM Visualization and areal distribution over
elevation
The topography is mostly characterised by a more or less flat
are in the centre of the study area (Figure 14). This area is called
«Central cuvette» and is limited by the Great Rift Valley to the
East, mountainous regions in the north-western and south-eastern corner of the
study area.
The altitude varies between -99999 and 4657 m with an average
of 1886 m. In HYDRO1k DEM, pixels with missing data are assigned a negative
value of -99999. Extracting the area covering exclusively the Congo Watershed,
the elevation mean is around 238 m aswl with a minimum of 0 m.
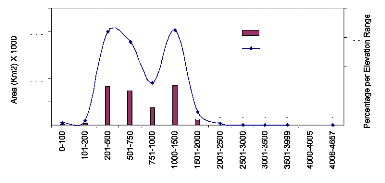
2500
2000
1500
1000
500
0
1 1
26 24
12
Elevation ranges
27
3 0 000 0
% of Elevation Area
40
80
60
20
0
Figure 13 Areal distribution at different altitude (The area in
a logarithmic scale)
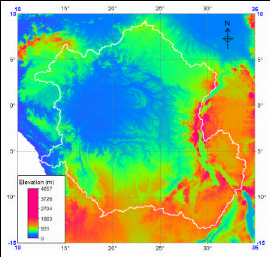
Figure 14 DEM visualization map for Cental Africa. The defined
colored polygone delineated the Congo River basin.
Table 4 Summarised Statistics for the DEM
Elevation
|
npix
|
npixpct
|
npixcum
|
npcumpct
|
Area (Km square)
|
0-100
|
46440
|
0.65
|
41461805
|
553
|
46956
|
101-200
|
88017
|
1.24
|
47682206
|
636
|
88994
|
201-500
|
1984879
|
27.92
|
342086982
|
4563
|
2006916
|
501-750
|
1770620
|
24.91
|
869454897
|
11598
|
1788574
|
751-1000
|
901295
|
12.68
|
1183992072
|
15794
|
911302
|
1000-1500
|
2006891
|
28.23
|
3176337475
|
42371
|
2029172
|
1501-2000
|
274325
|
3.86
|
3693851852
|
49274
|
277371
|
2001-2500
|
28808
|
0.41
|
3738833270
|
49874
|
29128
|
2501-3000
|
6081
|
0.09
|
3716166768
|
49572
|
6149
|
3001-3500
|
1168
|
0.02
|
2840777253
|
37895
|
1181
|
3501-3999
|
467
|
0.01
|
1866541342
|
24899
|
472
|
4000-4005
|
n/a
|
0.00
|
-
|
-
|
|
4006-4657
|
114
|
0.00
|
607211659
|
8100
|
115
|
PS: npix= number of pixels, npixpct= percentage of number of
pixels, Npicum = cumulated percentage of number of pixels. In colone 2, the
pixel numbers with -9999 elevation value are ignored.
4.3.2 Flow direction map
This step comes after fill-sink step. The filled DEM was then
used to find the flow direction map using standard D-8 algorithm (Figure 15).
Flow direction is calculated for every central pixel of input blocks of 3 by 3
pixels, each time comparing the value of the central pixel with the value of
its 8 neighbors. The steepest slope method was used for this study to find the
steepest downhill slope of a central pixel to one of its 8 neighbour pixels and
assign to flow directions.
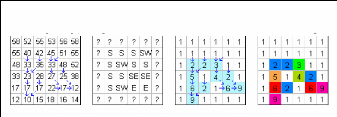
Calculating flow directions from a DEM (steepest slope)
Output flow direction map
Calculating flow accumulation
Output flow accumulation map
Figure 15 D-8 algorithm: Based on the output Flow
direction map, the Flow accumulation operation counts the total number of
pixels that will drain into outlets (after ILWIS 3.4 Manual)
The output map shown in Figure 16 contains flow directions
grids as N (to the North), NW (to the North West), NE (to the North East), SE
(to the South East), S (to the South) and SW (to the South West).
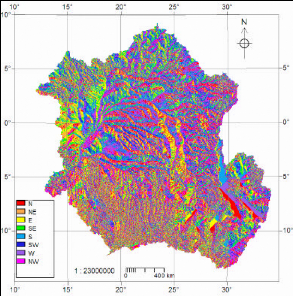
Figure 16 Flow direction map
The histograms (Figure 17) indicate that the flow direction
algorithm tends to favor the cardinal directions (north, south, east and west)
over the diagonal directions (northeast, northwest, southeast and southwest).
For the entire dataset (rectangular area) 63 % of grids cells had flown in a
cardinal direction as compared to 37 % diagonals. This indicates that the flow
direction algorithm used in the model is predisposed in favor of flow through
the cardinal directions. The same observation was done in previous study on the
basin (Kwabena, 2000).
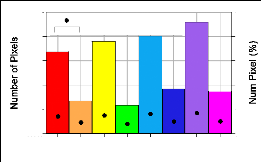
600000
400000
200000
700000
500000
300000
N NE E SE S SW W NW
Flow Direction Orientation
CB_Fdir_filled x Number Pixel Perc CB_Fdirjilled x NPix
60
40
20
80
0
100
Figure 17 Histogram of Flow Direction for Central
Africa
Table 5 Summarised statistics for the Flow direction grid
map in the area of study.
Flow direction Orientation
|
Number Pixel
|
% Number pixels
|
Area (Km2)
|
E
|
579477
|
15
|
579477
|
N
|
536518
|
14
|
536518
|
NE
|
334119
|
9
|
334119
|
NW
|
372838
|
10
|
372838
|
S
|
600426
|
16
|
600426
|
SE
|
314363
|
8
|
314363
|
SW
|
382729
|
10
|
382729
|
W
|
658407
|
17
|
658407
|
Min
|
314363
|
8.3
|
314363
|
Sum
|
3778877
|
100.0
|
3778877
|
4.3.3 Flow accumulation
The flow direction grid developed at the previous step is then
used as input data for Flow Accumulation grid calculations. The flow
accumulation map contains cumulative hydrologic flow values that represent the
number of input pixels which contribute any water to any outlets; the outlets
of the largest streams (drain, river) will have the largest values which is
3778906 for the Congo River basin. The grid generated (Figure 18) has a minimum
of 1 and a maximum of 3778906 pixels values for computed flow accumulation
matrix.
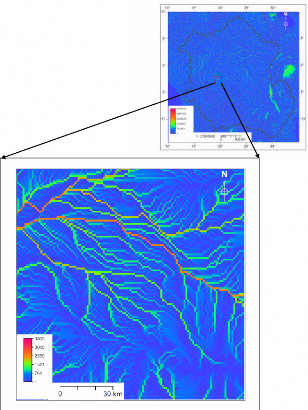
Figure 18 Flow Accumulation map; on top: Entire basin, on
bottom: A selected area
4.3.4 Drainage network extraction and
ordering
The Drainage Network Extraction operation extracts a basic
drainage network (raster map). As input it is required the output raster map of
the Flow Accumulation operation and a defined threshold value. A threshold
value, i.e. a value for the minimum number of pixels that are supposed to drain
into a pixel to let this pixel remain as a drainage in the output map; the
larger is this value, the fewer drainages will remain in the output map.
Depending on the flow accumulation value for a pixel and the threshold value
for this pixel, it is decided whether true or false should be assigned to the
output pixel. If the flow accumulation value of a pixel exceeds the threshold
value, the output pixel value will be true; else, false is assigned. A
threshold value of 1000 (number of pixels) is used in this process and 1752
stream segments are identified in the Congo River Basin masked (Figure 19).
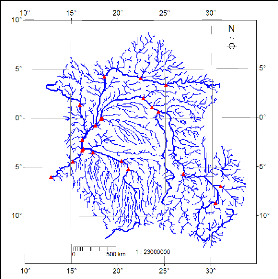
Figure 19 Stream network map masked by the boundary of the Congo
River Basin 4.3.5 Catchment and Sub-Catchments extraction
During the Catchment extraction operation, 3435 sub-catchments
were extracted. Using the Cross operation 1752 sub-catchements only (Figure 20)
were selected; each of them corresponding to a single stream segment from the
Drainage network ordering operation. This operation delivers an output raster
map, an output polygon map and an output attribute table.
The attribute table (appendix 6) andd Table 6 summarises
information for each catchment, such as the area, longest flow path, density,
and perimeter of catchment, the total upstream area. Figures 21-22 show 5
sub-catchments corresponding to 5 defined outlets namely Sangha, Ubangi, Kasai
and Lualaba. The Congo sub-catchment is generated with the residual area.
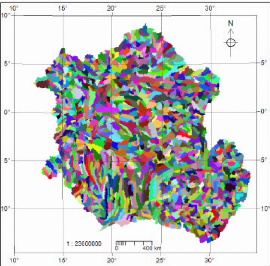
Figure 20 Extracted sub-catchment map in the Congo
Basin
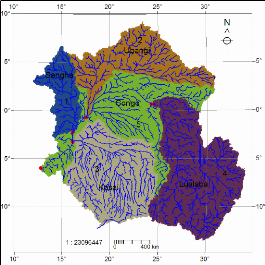
Figure 21 Merged sub-watershed with stream network and
majors outlet of the CRB
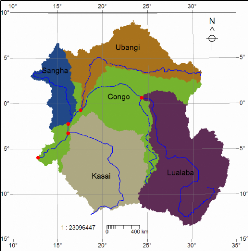
Figure 22 Longest flow path map overlayed on the
sub-watersheds of the CRB
4.3.6 Overland Flow map
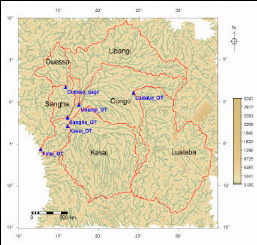
Figure 23 Overland flow distribution in the study
area
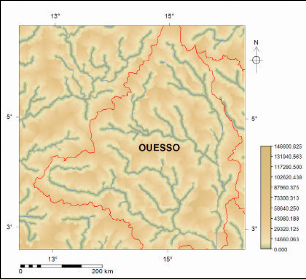
Figure 24 Overland flow distribution in the Ouesso
sub-watershed
4.4 Watershed characteristics
Watershed characteristics are subdivided in 2 major groups:
physiographical and hydrological. The physiographical characteristics of a
watershed influence to a large degree its hydrological responses and especially
the flow regime during floods and periods of drought, hence the discharge, and
the concentration time, which characterizes the speed and intensity of the
watershed's reaction to stress (rainfall), is influenced by the different
morphological characteristics. The analysis of the hydrologic behavior of a
watershed is done in order to study the hydrologic reaction of the watershed in
relation to rainfall. The Horton morphometric parameters will be described
separately.
4.4.1 Watershed Geomorphology 4.4.1.1 Area and
length
The drainage area (A) is probably the single most important
watershed characteristic for hydrologic design and reflects the volume of water
that can be generated from rainfall. It is common in hydrologic design to
assume a constant depth of rainfall occurring uniformly over the watershed.
Under this assumption, the volume of water available for runoff would be the
product of rainfall depth and the drainage area. Thus the drainage area is
required as input to models ranging from simple linear prediction equations to
complex computer models (McCuen, 2005).
The computed watershed area is of 3778879 km2, which
is strongly closer to 3,780,000km2, value estimated by Asante (2000)
using ArcMap.
Table 6 Sub-wateshed characteristics of the
CRB
|
|
|
|
|
|
|
|
|
|
|
1
|
Ouesso
|
2364.2
|
155408
|
3572.4
|
22.73
|
792.9
|
730197.6
|
341
|
762
|
702
|
2
|
Sangha South
|
2186.6
|
130671
|
3117.0
|
23.59
|
697.8
|
625778.7
|
234
|
457
|
343
|
3
|
Ubangi
|
6845.5
|
639564
|
15455.1
|
23.9
|
2538.5
|
2472266
|
291
|
1272
|
1133
|
4
|
Kasai
|
5516.5
|
895179
|
25007.0
|
27.62
|
2102.4
|
2022944
|
234
|
1481
|
1282
|
5
|
Lualaba
|
7264.1
|
1109014
|
27362.9
|
24.4
|
3083.4
|
3020203
|
429
|
1842
|
1446
|
6
|
Congo
|
11000.0
|
849063
|
19436.5
|
22.64
|
3515.9
|
3470018
|
0
|
1128
|
1032
|
|
CRB
|
13475054
|
3778879
|
93953.5
|
24.6
|
5112.7
|
5049.5
|
0
|
1842
|
1446
|
Table 7 Extracted sub-watersheds areas of the
CRB
ID
|
Names
|
Area (km square)
|
Calculated
|
Repported
|
Source
|
1
|
Ouesso
|
155408
|
180418
|
|
2
|
Sangha South
|
130671
|
3
|
Ubangi
|
639564
|
613202
|
|
4
|
Kasai
|
895179
|
925172
|
|
5
|
Lualaba
|
1109014
|
|
|
6
|
Congo
|
849063
|
|
|
Min
|
130671
|
|
|
Avg
|
629817
|
|
|
Sum
|
3778879
|
3755441
|
Asante (2000)
|
For a better and easier use of the DEM in the Congo River
hydrological model, the Congo Basin was subdivided in 6 major sub-watersheds,
namely: LUALABA, OUESSO, UBANGI, CONGO, SANGHA SOUTH and KASAI (Figure 22). The
Congo sub-watershed is a shapeless basin since it is a residue after the
selection of the other 5 major sub-watersheds; therefore it will not be
considered in the water balance process.
4.4.1.2 Watershed Shape
The shape of a watershed influences the shape of its
characteristic hydrograph. For example, a long shape watershed generates, for
the same rainfall, a lower outlet flow, as the concentration time is higher.
A watershed having a fan-shape presents a lower concentration
time, and it generates higher flow.
Different geomorphologic indices can be used for the analysis
of a watershed if its shape is taken into consideration. The most frequently
used index is the Gravelius's index KG, which is defined as the
relation between the perimeter of the watershed and that of a circle having a
surface equal to that of a watershed.
(20)
P P
K =
G
0.28
2 · A A
Where KG is the Gravelius's shape index, A is the watershed area
[km2] and P, watershed perimeter [km].
Musy (2001) presented different values of the Gravelius's
index whose comparison to the Congo Basin (Gravelius index = 1.93) makes it to
be treated like a circular basin. However, the Gravelius Index varies from one
sub-watershed to an other one.
4.4.2 Morphometric Analysis
The morphometric network is defined as the sum of all the
watercourses, natural or artificial, permanent or temporary, which contribute
to the runoff. The characteristics of a hydrographic network of a watershed are
influenced by four main factors: geology, climate, relief and environment.
4.4.2.1 Morphometric network
topology
The classification of the watercourses was introduced by
Strahler (1957). The order of the watercourses reflects the degree of
ramification of the morphometric network from upstream to downstream and it is
based on the following principles: (Musy, 2001)
all watercourses without tributaries are of 1 st order;
the watercourse formed by the confluence of two watercourses of
different order is going to keep the highest order of the two;
the watercourse formed by the confluence of two watercourses of
same order is going to have an order higher with one than the other two.
Seven Sthraler orders and 898 Shreve orders were identified in
the Congo basin (Appendix 6).
4.4.2.2 Horton morphometric
parameters
Based on the Horton's infiltration equation fame, Horton Laws and
ratio were developed in order to describe the geomorphological characteristics
of watershed based on the stream properties.
This ratio can be calculated manually if considering a simple
illustrative model. Considering the sub-continental size of the Congo Basin,
the estimation of Horton morphometric parameter were calculated using the
DEM-Hydrological module of ILWIS version 3.4, if not it would not be possible.
Figure 24 shows different graphs for the derived Horton morphometric parameters
for the Congo Basin and the following section define each of them and present
the results.
4.4.2.2.1 Streams number and bifurcation ratio
(RB)
STREAM NUMBER (or stream order) is a
measure of the degree of stream branching within a watershed. Each length of
stream is indicated by its order. The principal order in the Congo Basin is 7
and can be find only in the Congo subwatershed. The stream number for each
Subwatershed is given in Table 8.
The concept of stream order is used to computer other indicators
of drainage characteristics presented in the following paragraph.
Table 8 Stream numbers and Bifurcation Ratio for
sub-watersheds of the Congo River
Stream Order
|
Ouesso
|
Sangha
|
Ubangi
|
Kasai
|
Lualaba
|
1
|
30
|
26
|
118
|
168
|
261
|
2
|
6
|
8
|
32
|
42
|
54
|
3
|
3
|
1
|
9
|
13
|
14
|
4
|
1
|
1
|
2
|
5
|
3
|
5
|
0
|
0
|
1
|
2
|
1
|
6
|
0
|
0
|
0
|
1
|
|
BIFURCATION RATIO (RB) is defined as
the ratio of the number of streams of any order to the number of streams of the
next highest order. It is calculated as
Where Ni: number of streams of order I.
For the selected subwatershed, values of Rb range between 2.7
and 5.1 (Table 9); which falls into the theoretical interval [2 to 6] and a
typical interval (3 to 5) is reported in the literature (MacCuen, 2005).





CONGO RIVER BASIN SANGHA CATCHMENT
1000000
1000000
500000
500000
|
|
|
|
|
|
|
|
|
|
|
|
|
|
|
|
|
|
|
|
|
|
|
|
|
|
|
|
|
|
|
|
|
|
|
|
|
|
|
|
|
|
|
|
|
|
|
|
|
|
|
|
|
|
|
|
|
|
|
|
|
|
|
|
|
|
|
|
|
|
|
|
|
|
Order x C1A LSq Order x C1A Order x C1LLSq Order x C1L
Order x C1NLSq Order x C1N
|
|
|
|
|
|
|
|
|
|
|
|
|
|
|
|
|
|
|
|
|
|
|
|
|
|
|
|
|
|
|
|
|
|
|
|
|
|
|
|
|
|
|
|
|
|
|
|
|
|
|
|
|
|
|
|
|
|
|
|
|
|
|
|
|
|
|
|
|
|
|
|
|
|
|
|
|
|
|
|
|
|
|
|
|
|
|
|
|
|
|
|
|
|
|
Order x C1ALSq
|
|
|
|
|
Order x C1LLSq Order x C1NLSq
|
|
|
|
Order x C1A Order x C1L
|
|
|
|
|
Order x C1N
|
|
|
|
|
|
|
|
|
|
|
|


500000
500000
200000
200000
200000
200000
100000
100000
100000
100000
50000
50000
50000
50000
20000
20000
20000
20000
10000
10000
10000
10000
5000
5000
5000
5000
2000
2000
2000
2000
1000
1000
1000
1000
500
500
500
500
200
200
200
200
100
100
100
100
50
50
50
50
20
20

20
20

10
10
10
10
5
5

5
5
2
2
2
2
1
1
1
1
1 2 3 4 5 6 7
1 2 3 4 5 6 7 1 2 3 4 5 6 7
1 2 3 4 5 6 7
Order
Order
Order Order
Kasai
Ubangi
Kasai Ubangi
1000000
1000000
100000
100000
|
|
|
|
|
|
|
|
|
|
|
|
|
|
|
|
|
|
|
|
|
|
|
|
|
|
|
|
|
|
|
|
|
|
|
|
|
|
|
|
|
|
|
|
|
|
|
|
|
|
|
|
|
|
|
|
|
|
|
|
|
Order x C3ALSq Order x C3A
Order x C3NLSq Order x C3N
Order x C3LLSq Order x C3L
|
|
|
|
|
|
|
|
|
|
|
|
|
|
|
|
|
|
|
|
|
|
|
|
|
|
|
|
|
|
|
|
|
|
|
|
|
|
|
|
|
|
|
|
|
|
|
|
|
|
|
|
|
|
|
|
|
|
|
|
|
|
|
|
|
|
|
|
|
|
|
|
|
|
|
|
|
|
|
|
|
|
|
|
Order x C4ALSq Order x C4A Order x C4LLSq Order x C4L
Order x C4NLSq Order x C4N
|
|
|
|
|
|
|
|
|
|
|
|
|
|
|
|
|
|
|
|
|
|
|
|
|
|
|
|
|
|
|
|
|


500000
500000
50000
50000
200000
200000
20000
20000
100000
100000
10000
10000
50000
50000
5000
5000
20000
20000
10000
10000
2000
2000
5000
5000
1000
1000
2000
2000
500
500
1000
1000
200
200
500
500
100
100
200
200
50
50
100
100
50
50
20
20
20
20
10
10
10
10
5
5
5
5
2
2
2
2
1
1
1
1
1 2 3 4 5 6 7
1 2 3 4 5 6 7 1 2 3 4 5 6 7
1 2 3 4 5 6 7
Order
Order
Order Order
Lualaba
CONGO SUBWATERSHED
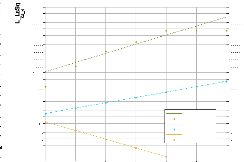
100000
100000
|
|
|
|
|
|
|
|
|
|
|
|
|
|
|
|
|
|
|
|
|
|
|
|
|
|
|
|
|
|
|
|
|
|
|
|
|
|
|
|
|
|
|
|
|
|
|
|
|
|
|
Order x C5ALSq Order x C5A Order x C5LLSq Order x C5L
Order x C5NLSq Order x C5N
|
|
|
|
|
|
|
|
|
|
|
|
|
|
|
|
|
|
|
|
|
|
|
|
|
|
|
|
|

10000000
10000000
50000
50000
5000000
5000000
2000000
2000000
20000
20000
1000000
1000000
10000
10000
500000
500000
5000
5000
200000
200000
100000
100000
2000
2000
50000
50000
1000
1000
20000
20000
10000
10000
500
500
5000
5000
200
200
2000
2000
1000
1000
100
100
500
500
50
50
200
200
Order x C5ALSq Order x C5A Order x C5LLSq Order x C5LLSq Order
x C5NLSq Order x C5NLSq
20
20
100
100
50
50
10
10
20
20
5
5
10
10
5
5
2
2
2
1
1
1
1
1 2 3 4 5 6 7
Order
1 2 3 4 5 6 7
Order
Figure 25 Horton morphometric parameters for 4 selected
sub-watersheds in the Congo River
37
Table 9 Horton Morphometric Parameters for the
sub-catchments in the Congo River.
Sub- watersheds
|
RB
|
RL
|
RA
|
Ouesso
|
3.2
|
2.4
|
3.5
|
Sangha South
|
5.1
|
1.7
|
6.1
|
Ubangi
|
4.0
|
2.2
|
4.3
|
Kasai
|
2.7
|
1.6
|
3.3
|
Lualaba
|
4.2
|
2.3
|
4.6
|
MIN
|
2.7
|
1.6
|
3.1
|
MAX
|
5.1
|
2.5
|
6.1
|
4.4.2.2.2 Law of Stream Lengths and stream length
ratio (RL)
The stream length assumes that the average lengths of the streams
of successive orders are related by a length ratio RL, and given by the
equation:
(22)
L
R thus L L R
= = ×
i
L L +
1
i i L
1
i +
Table 10 Stream Length Ration for the different
sub-catchments in the Congo River
|
Ouesso
|
Sangha
|
Ubangi
|
Kasai
|
Lualaba
|
C1_L
|
C2_L
|
C3_L
|
C4_L
|
C5_L
|
Stream Order
|
1
|
64.74
|
64.76
|
65.19
|
77.44
|
57.51
|
2
|
196.02
|
183.8
|
191.43
|
234.59
|
185.49
|
3
|
384.32
|
189.49
|
340.46
|
476.94
|
346.48
|
4
|
662.01
|
664.74
|
932.09
|
82 1.84
|
98 1.2
|
5
|
0
|
|
2130.4
|
1013.09
|
2265.76
|
6
|
0
|
0
|
0
|
1152.81
|
0
|
7
|
0
|
0
|
0
|
0
|
0
|
Horton Ratio
|
RL
|
2.44
|
1.71
|
2.21
|
1.64
|
2.3
|
The stream length ratio values (Table 10) fall between the
natural limits ranges of 1.6 - 2.5 (MacCuen, 2005).
1 2 3 4 5 6 7
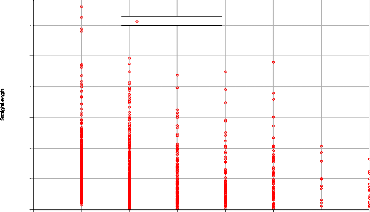
Strahler x StraightLength
300000
250000
200000
150000
100000
50000
0
Strahler
Figure 26 Strahler order vs. Stream length
map
Above (Figure 26) is a plot of Strahler stream order and
stream length. It is evident form the figure that this region has well defined
geomorphic features with stream length of 1 st order streams varying from 3 km
to 335 km, and 7th order streams with length varying from 0 to 90 km. Also, the
stream number in a subwatershed is inversely correlated to the stream order
(FigurF26).
4.4.2.2.3 Stream Area Ratio (RA)
Stream ratio is given by the following equation and the obtained
values from the DEMHydro processing are presented in Table 11 bellow.
RA
A i + 1
= (23)
Ai
Table 11 Stream Ration for the selected
subwatershed
|
Ouesso
|
Sangha
|
Ubangi
|
Kasai
|
Lualaba
|
C1_A
|
C2_A
|
C3_A
|
C4_A
|
C5_A
|
Stream Order
|
1
|
3322.57
|
C2_A
|
3137.12
|
2986.6
|
2591.04
|
2
|
21035.86
|
3258.19
|
15777.09
|
16796.23
|
16541.88
|
3
|
49761.68
|
14855.7
|
61493.83
|
63232.86
|
70292.39
|
4
|
158672.2
|
119366.6
|
296725.4
|
177175
|
358082.7
|
5
|
0
|
132257.5
|
646792.4
|
450879.8
|
1121600
|
6
|
0
|
0
|
0
|
905331.4
|
0
|
7
|
0
|
0
|
0
|
0
|
2445.2
|
Horton Ratio
|
RA
|
3.5
|
6.05
|
4.34
|
3.25
|
4.6
|
4.4.2.2.4 Channel slopes and length
The steep slope of a watercourse favours and accelerates the
runoff, while a small slope gives the water the necessary time to infiltrate
totally or partially into the soil. The calculation of the average slope is
obtained from the longitudinal profile of the main stream and its
tributaries.
The most frequent method used to calculate the longitudinal
slope of a watercourse consists of correlating the difference of altitude of
the extreme points of the stream with its length.
where: S1 longitudinal slope of stream [m/km] or
[%o]
?H: difference of altitude of the extreme points of
the stream [m] L: total length of the stream between its extreme
points [km]
At a spatial resolution of 1 km (Hydrosheds and Hydro1k) the
slope values for each pixel grid seems to be negligible.
4.4.2.2.5 Channel degree of
development
Drainage density, introduced by Horton, is the ratio of the total
length of streams within a watershed to the total watershed area (Equation
24).
where: Dd: degree of development of the hydrographic network
[km/km2], Li: length of the stream [m] and A: watershed surface [km].
The Density values for each subwatershed are given in Table
12. The subwatershed density varies between 22.6 and 27.6 with a mean of 24.2
and a standard error of 0.63. A high value of stream density indicates a
relatively high density of streams and thus a rapid storm response. The
development of stream seems to be uniform all over the basin.
Table 12 Drainage density for watersheds of the Congo
River
ID
|
1
|
2
|
3
|
4
|
5
|
6
|
|
Name
|
Ouesso
|
Sangha South
|
Ubangi
|
Kasai
|
Lualaba
|
Congo
|
CRB
|
A (Km2)
|
155408
|
130671
|
639564
|
895179
|
1109014
|
849063
|
3778879
|
Li (m)
|
3572.4
|
3117
|
15455.1
|
25007
|
27362.9
|
19436.5
|
93953.5
|
Dd (m/Km2)
|
22.73
|
23.59
|
23.9
|
27.62
|
24.4
|
22.64
|
24.6
|
Owing to the fact that all the Horton geomophometric
parameters fall in the accepted natural limits, we assume that the 5
sub-watershed extracted from the DEM are representative for the Congo watershed
and can therefore be used in the GIS-linked hydrological model to develop.
4.5 GIS-Based Hydrological Model Development
4.5.1 Introduction
In order to compute the water resource availability and to
simulate the spatial and temporal distribution of water balances over the Congo
River Basin (CRB), a GIS-based hydrological model, namely HATWAB (Hybrid
Atmospheric and Terrestrial Water Balance) was developed and parameterized for
the Congo basin (Figures 27 and 28), based on the initial model of Alemaw
(2006). Initially, a GIS based hydrological model (Alemaw, 1999) was developed
to computer the spatial and temporal distribution of water balance in southern
Africa reion. This model was modified and used for the same region (Alemaw and
Chaoka, 2003) and for the Limpopo basin (Alemaw, 2006). The approach of this
model is based on the parametersization of input data, viz monthly
temperature, precipitation, land-cover/use, soil texture and rooting depth and
the Digital Elevation Model, to compute temporal and spatial variability of
water budgets at geo-referenced grids cells covering the Congo basin.
The atmospheric component of the water balance model computes
the Integrated Vertical Moisture Convergence (C) based on the precipitation and
evaporation, while its terrestrial component estimates Actual Soil Moisture
(SM), Actual Evapotranspiration (AET), and Runoff (TRO). Surface abstractions
components in term of overland runoff (DRO) and interception are also
accounted. The model estimates the uncertainties on water balance parameters
and as well as the imbalances.
A separate component of the model consists of a DEM
hydrological processing. This component extracts watershed and streams network
(Figure 12), compute their hydrogeomorphometric parametes which characterise
the topography, topology and hydrography of the river basin.
The Spatial and temporal distributed water balance model
requires various types of data from different sources (Melesse et al, 2006)
like Remotely-sensed datasets (NDVI images, DEM), meteorological datasets
(precipitation, air temperature, wind speed, sunshine hours, etc), hydrologic
(river discharge), hydrologic soil maps and other GIS layers ( watershed
boundaries and properties, streams topology, etc) in raster,vector and tabular
formats. Their collection and preparation will be presented in section 5.4.
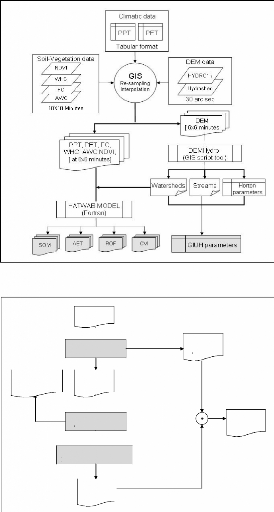
Figure 27 General terrestrial Water Balance model
structure
RAINFALL (PPT)
Surface process
EPPT = PTT -DRO
EFFECTIVE RAINFALL (EPPT)
DIRECT RUNOFF (DRO)
Soil storage
(FC, WP, AWC...)
Excess = EPPT- DRO - AET-? SM
RUNOFF STORAGE (RO)
TOTAL RU NO
FF (GRID)
ACTUAL EAVPO- TRANSPIRATION
Figure 28 Rainfall-Runoff simulation model for a single
grid cell
4.5.2 Water Balance Model development
procedure
The water balance model development comprises the following
steps:
(i) Vertical Integrated Moisture
convergence:
The Vertical Integrated moisture convergence (C) is a component
of the atmospheric water balance and will be computed based on rainfall and
Evapotranspiration data.
(ii) Rainfall-Runoff Development
At this stage, components derived from previous steps will be
used to generate monthly water balance compounds as shown in Figures 27 and 28:
Runoff (TRO), Actual Evapotranspiration (AET), Soil Moisture (SM) and
Percolation (P). The Thornthwaite and Mather (1957) method, slightly modified,
generate a monthly water balance for each grid-pixel as well as for the whole
Congo River Watershed.
(iii)DEM-Hydro processing: Watershed and Streams
delineation
Watershed and sub-watershed boundary maps are required to
delineate the specific area where the soil-water balance model will be applied.
In other words, the model will account for the water balance for the grid cells
falling in the watershed limits. These files, namely `MASKS', are extracted
during the DEM processing.
The DEM-Hydro Processing were developed in section 4 in order
to derive Geomorphometric properties of the catchments and stream network like
flow direction, flow accumulation, catchment, drainage network, overland flow,
masking files, and other hydrologic data using GIS packages (viz ILWIS 4.3 and
ESRI ArcMap 9.2 versions). The Horton statistic parameters were used for
discriminating and characterizing the extracted watershed and streams from the
DEM.
4.5.3 Water Balance Model Development
Supposing the principle of continuity in hydrologic system,
Chow (1998) assumes that the time rate change of storage is equal to the
difference between the input and the output in of the hydrologic model
(equation 26).
where, St is the time-variant storage in a grid cell,
It , the summation of input coming into the cell from upstream cells
and the runoff generated in the cell, and Ot , the outflow from the
cell which is calculated by various methods, e.g. the linear reservoir
method.
4.5.3.1 Atmospheric water balance
The atmospheric component of the water balance model is expressed
as
dWPEC
dt = - + + (27)
C=-?×Q (28)
Where dW/dt is the amospheric storage change, C is the
vertical integrated moisture converence (LT-1) and was expressed as
a function of the water vapour flux (Q) in Equation (28).
? × Q is the divergence or net outflow of water
vapor across the sides of the atmospheric column, Q is the vapor flux, E is
evaporation, and P is precipitation.
The quantity W is also referred to as the precipitable water
and may be expressed in units of mass per unit surface area [M L-2]
or converted to an equivalent depth of liquid water [L] by dividing by the
density of liquid water (1000 kg m-3).
The divergence Q mesures the difference between inflow and
outflow to a region; a positive divergence means that outflow is greater than
inflow, and a negative divergence (or convergence) means that inflow is greater
than outflow. The units of divergence are [M L-2 T-1] but
may also be expressed as depth of water per time [L T-1].
In mean water balance computation like the HATWAB model which
is monthly water balance model, the atmospheric storage change is often assumed
to be negligible (Reed et al, 1998 and Marengo, 2005); thus equation (28) is
reduced to

P-E=C or P-E=-?Q
(29)
|
|
From Equation (30), it is seen that if the divergence
(?Q
|
) in a region is positive, then
|
evaporation is greater than precipitation (P-E < 0), while a
negative divergence or "convergence" indicates that precipitation is greater
than evaporation (P-E > 0).
4.5.3.2 Terrestrial water balance
At monthly time scale a terrestrial water balance model is
written as
ds P E R
dt = - - (30)
Where S is soil moisture storage (L); P is
precipitation (LT-1); E, actual Evapotranspiration
((LT-1); and R, the observed runoff (LT-1).
Linearising Equation (30), the terrestrial water balance has the
following form
SM1 = SM t - 1 + P
t -E t - R t (31)
Where t is the time period, which is a single month is this
model, P is precipitation, E is the Evapotranspiration, and RO is the
runoff.
Once the initial soil moisture (St-1) and the actual
soil moisture (St) are determined, the monthly Actual Evapotranspiration Et and
the Runoff Rt can be calculated.
4.5.3.3 Imbalance estimation
Combining Equations (30) and (31), the vertical integrated
moisture convergence (C) is computed as follows
dS C R
- = (32)
dt
In a steady state P AET =R. Changes in storage could
however lead to P E being different from R. These could also be due to
certain errors in representing rainfall within the Congo River Basin. These
lead to the expression of an imbalance equation
C ds dt R
- ( / + )
Imb = (33)
R
The imbalance in Imb is calculated using the
following formula:
C ds dt
/
Imb (34)
= - + 1
R R
Contrary to the formulation adopted in this study based on
(34), Marengo (2005) assumes that dS/dt is negligible for monthly time
scales and applied it in the Amazon basin, which reported unaccounted residuals
in his water budgets. Whereas in the proposed model in this study, the soil
moisture variation dS/dt is varying from season to season as a
function of the prevailing PET and PPT at a given location
according to Equation (30), in which the imbalance should also cater for this
variability according to Equation (34). One of the contributions of this study
is that, once the imbalance (Equation 34) is kept to a minimum in the water
balance computation at each grid, then the simulated variables and water
balances, PPT, ET, RO, RO/PPT,
ET-PPT, ET/PET, S and C can then be used to
investigate the seasonal and temporal variability of water budget of the Congo
basin.
The amount of precipitation and potential evapotranspiration
determines soil moisture availability, which in turn is controlled by the water
holding capacity of the soils. Solution of the mass balance equation will be
solved for E, R and S (section 4.5.3.4).
4.5.3.4 Rainfall-Actual Evapotranspiration-Soil
moisture-Runoff modelling 4.5.3.4.1 Effective Precipitation (EPPT) and
overland runoff (Direct runoff DRO)
One of the main inputs required in the proposed water balance
model is the effective precipitation (EPPT). At a monthly time scale, the
effective precipitation is calculated as per the formula of the USDA soil
conservation available from FAO (1990) as follows:
125 0.2
- PPR
EPPT PPT for PPT mm
= ( ) 250
< (35)
125
EPPT = 125 + 0.1 PPT forPPT=250 mm
(36)
Where EPPT is effective precipitation and PPT
is the total precipitation
The direct runoff (DRO) is the difference between the
precipitation (PPT) and the Effective precipitation (EPPT).
4.5.3.4.2 Soil moisture estimation
(SM)
Soil moisture is determined from the interaction between
effective PPT and PET. During wet months (when effective PPT in excess of PET),
soil moisture can increase up to a maximum of field capacity determined by soil
texture and rooting depth.
dt
dSM = EPPT - PET when EPPT > PET and SM < FC
(37)
dt
dSM = 0 when SM = FC (38)
dSM ( - )
dt
= a SM PET EPPT when EPPT < PET (39)
Where, FC is the soil moisture at field capacity of the soil
millimetres, PPT is precipitation in mm/month; PET is potential
evapo-transpiration in mm/month.
During the dry periods where the EPPT < PET, the soil
becomes increasingly dry, soil moisture becomes a function of potential soil
loss. Thus, different authors assumed different relationship to find the soil
moisture during the dry periods (Thornthwaite, 1948; Vorosmarty et
al., 1989).
For a particular soil, there is a linear relationship between
Log (SM) and Ó(EPPT-PPT) summed from the start of the dry season to the
current month. Thus, to calculate the rate of change of soil moisture (ÄS)
through the dry season, the soil moisture is directly estimated from the
empirical relation suggested by Vorosmarty et al. (1989). To calculate
ÄSM/dt through Equation (40) for intermediate field capacities, the HATWAB
defines a slope a to the retention function as:
a = ln(FC)/(1.1282 FC)1 . 2756 (40)
where the numerator represents soil moisture (millimetres) with
no net drying. The denominator is the accumulated potential water loss or
APWL ( ? [PET - PPT ]) in mm
at SM=1 mm. With a determined, the model can
calculate dSM/dt as a function of soil dryness and update SM. Figure 29 shows
the relationship between soil moisture and the Evapotranspiration. Between the
plant wilting point and the root zone Field Capacity, Evapotranspiration
increases with the soil moisture.
Calculations commence at the end of the wet season when it is
assumed the soil is at the field capacity. Soil water stocks are then depleted
during the dry season in accordance with the moisture retention function. For
each wet month, soil moisture is determined by incrementing antecedent values
by the excess of the available water over PET. This recharge may or may not be
sufficient to bring the soil to field capacity at the end of subsequent wet
season.
ETa/ETp
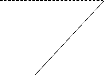

1

WP FC=WH
Figure 29 Functional relationship between soil
moisture and Evapotranspiration (ETa is the actual Evapotranspiration, ETp is
the potential Evapotranspiration, SM is the soil moisture, FC is the field
capacity and WP, the Wilting point
Analysis of the ratio PPT/PET at the various grids in Central
Africa has shown that the region can not be represented by one homogeneous
climatic zone, rather it is a combination of climate influenced by the
diversified hydro-climatic conditions in the region. In this analysis, it has
been observed that the long-term monthly rainfall distribution varies greatly
in the region.
It is assumed that the ratio PPT/PET could be a good indicator
of the seasonal distribution of monthly rainfall, and accordingly the wet
season ends when the ratio PPT/PET just starts to fall below unity. In line
with this, therefore, the soil moisture can be assumed to be at its field
capacity. Consequently, HATWAB fixes a starting month of any grid across the
region according to the aforementioned criterion. The solution of the basic
mass balance equation for the subsequent months can determined once the initial
soil moisture state is obtained.
4.5.3.4.3 Actual Evapotranspiration
(AET)
Once the actual soil moisture is determined, the corresponding
actual evapotranspiration (AET) is calculated for the month. Following the
Thornthwaite and Mather (1957) approach, AET is set equal to PET in wet months,
when EPPT > PET. During this time it is assumed that EPPT is in sufficient
abundance to satisfy all the potential water demands of the resident
vegetation. During dry season when EPPT < PET, the monthly average AET is
modified down wards from its potential value as shown below.
AET = PET when EPPT > PET (41)
dSM
AET = EPPT - when EPPT < PET (42)
dt
where the soil moisture drops below the wilting point, the AET is
equal to the EPPT but if there is no EPPT at all time, AET becomes zero.
4.5.3.4.4 Total runoff (TRO) generated at a grid
spatial scale
Most of the water does not leave the basin as soon as it
becomes available as surplus. The portion that constitutes overland
flow is assumed to flow out of the watershed within the
month it occurs but the portion that infiltrates may take a
number of months to move slowly through upper layer of the soil column to
emerge in the surface water courses as base flow.
The lag or delay factor depends not only the size of the basin
but also on the vegetation cover and the soil type, degree of slope,
characteristics of the soil layers, etc. Thornthwaite suggested that 50 % of
the water surplus could be assumed to runoff each
month from large basins with the remainder being held over and
added to the surplus of the next month. This factor is set according to
Thornthwaite & Mather (1957) which is equal to 0.5.
RO = (D + (EPPT - PET) when SM = FC EPPT > PET
0 . 5 * ) &(43)
RO = 0.5 * D when SM < FC EPPT < PET
& (44)
Where, RO is rainfall-driven runoff or surplus runoff (mm/month)
and D is the amount of detention storage in millimeters that is assumed to
leave each grid cell next month.
4.5.4 Data sets and software
This study requires a set of hydro- meteorological variables
(rainfall, evapotranspiration, and wind speed), topographic data or Digital
Elevation Model (DEM) data set, landcover/use variables, vegetation, soil
data.
4.5.4.1 GIS and geo-referencing procedure
The Congo River basin has a sub-continental extension covering
8 countries in Central Africa region. It is extended between 150S to
100N and 100E - 35 0E. Originally, the area of
study has a spatial resolution of 30x30 minutes but for a better resolution and
accuracy of the results a 6 minutes (averaged to 12km at the equator) was
adopted and forced by the computational techniques.
The Kriging method (Krige, 1966; Stein, 1998, Surfer v.8) was
used to interpolate and fill the missing value, harmonizing different data sets
to a spatial resolution of 6 minutes for a single grid cell, corresponding to
62500 square grids (250 rows and 250 columns).
4.5.4.2 Meteorological data sets
Free meteorological data for 3262 global climatic stations are
available at FAO-UNESCO /CLIMWAT, with a record time of 30 years (1961 -1990)
at a monthly scale; where 145 climatologic stations covering the study area
(Figure 29 and Appandix 1) were extracted to compile the meteorologic database
for the model. These climatologic data sets include monthly averages of maximum
and minimum of temperatures, mean relative humidity, wind speed, sunshine
hours, radiation data as well as rainfall and Reference Crop Evapotranspiration
(ETo) calculated with the Penman-Monteith method (Allen et al, 1998). Below,
Figures 31 and 32 show the Rainfall and Potenital Evapotranspiration
distribution in the basin, respectively.
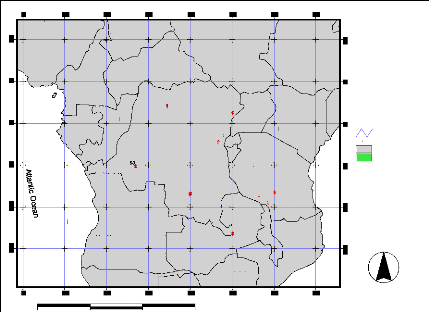
700 0 700 1400 Kilometers
Enugu
%
Kano
Libreville
Pointe Noire
%
Douala
%
%
Malabo
%
Port Gentil
%
Benguela
$
Luanda
Yaounde
%
Brazzaville $
$
$
%
$
$$
M $
atadi $ $
%
$
$
%
$
$
$
$
% %
Huambo
%
Maiduguri
$ $
$
$
$
$
$ % %
%
$
$
$
$
Kinsha
$
$
$
$
$
$
Mbandaka $
$
$
$
$
$
$
Kahemba
%
Bangui
$ %
$
$
$
%
$
$
$
$
$
$ $
$
$
$
$
$
$
$
$
$
%
$
$
$
$
$
Kananga $ $ $
$$
$ $
$
$
$ $
$ Kisangani
$ $ %
Lumumbashi
$ $
Livingstone
$ $
%
$
$
$
$
$
$
$
$
$
$
$ Kigoma
$
%
$
$
$
$
Lusaka
$$ %
$
$
$
$
$ $
%
$
$
$
$ $ $
$
$ Kigali
%
$
$
$
$
%Bujumbura
Harare
$
$
$
%
Kampala
%
$
$ $
$
$
$
$
$ Lilongwe
%
$
$
Dodoma
$
$
$
$
Beira
$
Dar es Salaam
Nairobi
%
Adis Abeba
Mocambique
%
%
Mtwara
%
%
%
Mombas
%
Djib
An
$ Stations zxy.txt
% Cities.shp
Afrbord.shp
Afpolit.shp
Latlong.shp
Figure 30: Distribution of Climatic stations in the study
area. The study area covers more than 145 stations

10
-10
-15
-5
5
0
Annual Rainfall
|
|
|
|
|
mm/year
2100 1900 1700 1500 1300 1100 900 700
|
|
|
|
|
|
|
|
|
|
|
|
|
|
|
|
|
|
|
|
|
Annual Effective
|
Rainfall
|
|
|
|
mm/year
1600 1500 1400 1300 1200 1100 1000 900 800 700 600
|
|
|
|
|
|
|
|
|
|
|
|
|
|
|
|
|
|
|
|
|
10 5 0 -5 -10 -15
10 15 20 25 30 35 10 15 20 25 30 35
10 15 20 25 30 35
10 15 20 25 30 35
Figure 31 Rainfall averaged (1961-190) data from 145
stations. 1. Rainfall, 2. Effective Rainfall
10 5 0 -5 -10 -15
Annual Potential
|
Evapotranspiration
|
|
|
mm/year 1800 1500 1400 1300 1200 1100 1000
|
|
|
|
|
|
|
|
|
|
|
|
|
|
|
|
|
|
|
|
|
|
|
10 15 20 25 30 35
Figure 32 Mean Annual Potential Evapotranspiration
(1961-1990) map
4.5.4.3 Discharge data
There is a lack of discharge data in the Congo Basin although
there is Rainfall gages and data since 1958. The availability of recharge data
is limited to a few numbers of rivers gauges. At the basin final outlet,
discharge has been amounted to an average of 45000 m3/sec (Asante,
2000).
Discharge data indicate a series of four distinct phases in
the Congo and the Oubangui since the beginning of the 20th century.
During the 1 960s they increased, overtaking their average over a century. The
Congo discharge then fell, returning in 1970 to what had been the normal level,
whereas the Oubangui entered a drought phase. This trend accentuated from 1980
and, until 1996, the Congo discharge weakened by 10% (37 400 m3/s in 1992
compared with an average of 40 600 m3 /s over that period as a whole), which
was the most dramatic decrease of the century. This fall is much stronger in
the Oubangui (- 29%), yet negligible (- 0.2 %) in the Kouyou sub-basin.
Overall, whereas discharge decrease in the Congo Basin is between two and four
times the drop in rainfall (IRD, 2002) Table 14 summarises the Congo River
discharge (1903 -1983) at Kinshasa station.
4.5.4.4 Digital Elevation Model (DEM) and Mask
files
The topographic properties of the area were derived from a DEM
raster data namely HYDRO1k. The HYDRO1k is a geographic database developed at
the U.S. Geological Survey's EROS Data Centre to provide comprehensive and
consistent global coverage of topographically derived data sets, including
streams, drainage basins and ancillary layers derived from the USGS' 30
arc-second digital elevation model of the world (GTOPO30).
The HYDRO1k has the advantage of being hydrologically
corrected for calculation of derived hydrologic parameters such flow direction,
flow accumulation, slope (Asmamaw, 2003); and it is freely available at a
standard suite of geo-referenced data sets with a spatial resolution of 1
kilometre, adapted for a large scale water balance model. Figures
14 and 21 show the DEM of the study area and the polygon map of
the entire CRB and sub-watersheds `Mask files».
4.5.4.5 NDVI and vegetation database
The monthly NDVI images for each basin were acquired from the
Global Land Cover Characteristics database. Satellite data have been used
extensively for mapping the land use and for monitoring the seasonal change of
vegetation of river basins (Nemani & Running, 1989).
The Normalized Difference Vegetation Index (NDVI) is commonly
applied to derive the leaf area index (LAI) from channels 1 and 2 of
NOAA-AVHRR data at 1 -km2 resolutions. Monthly twelve NDVI images,
expressed in percentage, for 1987 are acquired from USGS web pages. Based on
NDVI values the LAI for different land use groups can be estimated by empirical
formulae. Table 13 presents rooting depth assigned for various soil textures
and SCS soil groupings
Table 13 Rooting depth assigned for various soil textures
and SCS soil groupings
Veg. Group
|
NDVI
|
Assigned Root Depth (M) For Different Soil Types
|
Sand
|
Sandy Loam
|
Silty Loam
|
Clay Loam
|
Clay
|
Lithosol
|
VEG2
|
>50%
|
2.5
|
2.0
|
2.0
|
1.6
|
1.2
|
0.1
|
VEG1
|
=50%
|
1.0
|
1.0
|
1.3
|
1.0
|
0.7
|
0.1
|
SCS soil group (Singh, 1992)
|
A
|
B
|
D
|
C
|
C
|
B
|
4.5.4.6 Soil properties
A global Soil map at 10x10 minutes resolution is freely
available at FAO/UNESO database (FAO, 1984). This map is used to extract the
submap of the study area and later re-sampled to a spatial resolution of 6x6
minutes so that it can overlay onto the model.
Since the Soil Water available to plants depends on soil water
content, soil texture (Figure 33), and consequently, the rooting depth of
vegetations, the 133 agronomic groups of FAO agronomical soil map were
therefore used to derive and reclassify textural soil classes which are
required as input data for the model. For this matter a FORTRAN algorithm was
developed and 6 hydrological textural classes of soil (Table 14 and 15) were
identified in the study area.
Table 14 Soil texture distribution in the Congo River
basin
Class ID
|
1
|
2
|
3
|
4
|
5
|
6
|
0
|
Total
|
Soil type
|
Sand
|
Sandy Loam
|
Silty Loam
|
Clay loam
|
Clay
|
Lithosol
|
Water body
|
No grids
|
4847
|
0
|
58
|
21867
|
989
|
2374
|
513
|
30640
|
% gris
|
15.8
|
0
|
0.2
|
71.3
|
3.2
|
7.7
|
1.7
|
100
|
The reclassified textural soil and vegetation maps are used to
derive the soil retention parameters such as Field Capacity (FC), Wilting Point
(WP) which determine the soil moisture capacity known as Available Water
Content (AWC) for each soil group.
Based on field measurements, Saxton et al. (1986)
have developed a technique to estimate the matrix potential of different soils
by using multiple regression techniques. Therefore, at a matrix potential
equivalent to field capacity (33 KPa) and wilting point (1500 KPa) for a unit
meter depth of soil, approximate values of FC and WP for the various soil
textures have been extracted (see Table 15). Accordingly, the FC and WP values
as per the rooting depth are then derived for the whole region as summarised in
Table (15). Available water content (AWC) of a soil of given texture is defined
as the difference between the field capacity and wilting point.
The depth of soil water which can be used by the crop, the
Total Available Water (AWC), depending on the root depth of the crop and on the
soil moisture holding properties of the soil (Eilers et al, 2007), Equations
(45, 46) bellow shows the relationship between these soil parameters:
AWC = (UFC-UWP)R (45)
With
|
FC UFC R
= ×
WP UWP R
= ×
|
(46)
|
Where AWC is the available water content per root depth;
FC and WP are the field capacity and wilting point,
respectively; R is the current depth of the roots; UFC and
UWP are the field capacity and the wilting point per unit volume of
the soil, respectively.
Figures 33-36 show the generalised relationship between the
soils parameters, the Textural soil groups, the Field capacity map, the Wilting
Point map and the Available water content map, respectively.
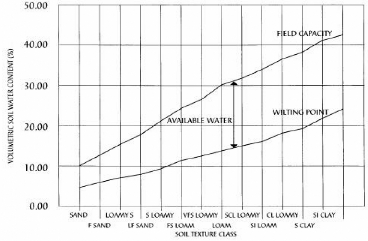
Figure 33 Available soil water vs. soil texture
showing estimates of field capacity, permanent wilting point and Available
water content. S-Sand, SI-Silt, CL-Clay, F-Fine, VF-Very Fine, L-Loamy (after
Levy et al, online)
Table 15 Relationship linking vegetation class, soil
texture, rooting depth and moisture capacities of various soil groups in
Central Africa (Source: Alemaw and Chaoka, 2003)
|
Sand Sandy Loam Silt Loam Clay Loam Clay Lithosol
|
Veg. Group
|
The Root depth (m)
|
GRP1
|
2.5
|
2.0
|
2.0
|
1.6
|
1.2
|
0.1
|
GRP2
|
1.0
|
1.0
|
1.3
|
1.0
|
0.7
|
0.1
|
2.3.2.1.1.1.1.1.1 FC and AWC of soils as % of total volume
of soils / m
|
% (FC)/m %(AWC)/m
|
14.1
6.3
|
20.0
9.1
|
27.3
13.2
|
35.2 35.8
|
48.5 35.8
|
27.3
13.2
|
2.3.2.1.1.1.2 Field Capacity and AWC per root depth of the
plant
|
GRP1 (FC)
(AWC)
|
353 196
|
400 218
|
546 282
|
563 243
|
582 153
|
27 14
|
GRP2 (FC)
(AWC)
|
141
78
|
200 109
|
355 183
|
352 152
|
339
89
|
27 14
|
-10
-15
10 15 20 25 30 35
10
-5
5
0
Textural Soil Types map
|
|
|
|
Soul types
6 Luthosol 5 Clay
4
|
|
|
|
|
|
|
|
|
Clay loam
3 SultLoam 2Sandy Loam 1 Sand
|
|
|
|
|
|
0 Water body
|
|
|
|
|
|
|
|
|
|
|
|
Figure 34 Hydrological Soil types over the
basin
10 5 0 -5 -10 -15
Soil Fiel Capacity in the root zone
|
|
|
mm/root depth
|
|
|
|
|
0
|
550 500 450 400 350 300 250 200 150 100 50
|
|
|
|
|
|
|
|
|
|
|
|
|
|
|
|
|
|
|
|
|
|
10 15 20 25 30 35
Figure 35 Soil Field Capacity in the root
zone.
10 5 0 -5 -10 -15
Soil Available Water Content
|
|
|
mm/root depth
|
|
|
|
|
0
|
240 220 200 180 160 140 120 100 80
60
40
20
|
|
|
|
|
|
|
|
|
|
|
|
|
|
|
|
|
|
|
|
|
|
10 15 20 25 30 35
Figure 36 Hydrological Soil types over the
basin
4.5.4.7 Software resources
The packages used in this research are FORTRAN Power Station,
ILWIS 3.4, ArcGIS 9.2, ArcViewGIS 3.3, Canvas7, Surfer v8.0 and CorelDraw v12,
and Microsoft Excel packages.
CHAPTER FIVE
5.0 MODEL APPLICATION, DATA PRESENATTION AND INTERPRETATION
RESULTS
5.1 Generalities on the Model application
The HATWAB model runs and simulates the water balance for each
of the 62500 grid cells of the study area using inputs datasets previously
developed and presented in GIS dataset formats. The GIS-database includes the
hydro-climatic data sets which are potential precipitation (PPT) and potential
Evapotranspiration (PET). It includes also the soil texture, the Vegetations,
and rooting depth, which are used to derive the Soil moisture capacities per
unit volume: Field capacities (FC), Wilting point (WP), Available water content
(AWC). All the data sets are incorporated in the model at a 6X6 minutes (12X12
km) grid cell spatial resolution.
A raster file having an integer value of 1 inside the basin
area and 0 outside the basin is assigned to the model as masking file
delineating and forcing the basin boundary to the entire output of the model
simulations.
Other important characteristic of the model is the facility to
simulate the water balance for a specified area: a single grid cell, a
sub-watershed (example: Kasai, Ubangi, Bangui, Ouesso, Lualaba sub Congo) or
the entire Congo basin.
For each grid cell, the model run for a time loop of 12
months, using PPT and PET data sets available for a period of the 30 years
(1961-1990) until a dynamic steady state is achieved in SM, AET and TRO with
tolerance error of 0.01% in all these variables. If the steady state is not
achieved the model proceed on a second, third, and so on, until the steady is
achieved.
Finally, monthly and annual averages for Soil moisture (SM),
Actual Evapotranspiration (AET), Runoff (TRO), Vertical Integrated moisture
coverage (C), and the Imbalance (I) are outputted for the entire basin.
A spreadsheet was developed to accumulate the total Runoff for
each sub-watershed, and to average the annual Soil moisture and
Evapotranspiration. These results will be presented in the following
sections.
5.2 Initial soil moisture
In order to solve the water balance differential equation
governing the soil moisture Equation (31), the initial soil moisture is
determined in accordance with Alemaw (2003). In most hydrological water
balance, it is assumed that at the end of a wet season the soil moisture
remains at the state of its field capacity attained during the wet season.
Therefore, the simulation starts at the end of the wet season when it is
assumes that the soil is at field capacity. Soil water stocks are then depleted
during the dry season in accordance with the moisture retention function.
For a large watershed, the ratio PPT/PET can be used as a good
indicator of seasonal distribution of the wetness of the dryness at a
specific geographic location in a region: a wet season is characterised by a
ratio PPT/PET greater than the unity, while a value less
than the unity corresponds to the dry season. The month where
PPT/PET just starts to fall under the unity is selected by the model as the
starting month of the simulation.
5.3 Data presentation and Interpretation results
5.3.1 Soil moisture (SM)
Annual average Soil moisture and seasonal soil moisture maps
are shown in Figures 38 and 39, respectively. The mean annual soil moisture for
the entire basin ranges between 0.7 and 431.4 mm, with highest mean monthly
soil moisture of 546.2 mm during November and lowest, in July (146.2mm). A
general trend is observed in the spatial distribution of the moisture: the
regions around the equator are characterised by high soil moisture and this is
reduced as the distance to equator is increased (Figure 37).
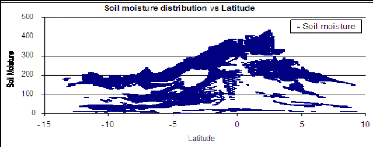
Figure 37 Soil moisture correlation with the
latitude
Spatially, the highest values are located in the centre of the
basin which coincides with the core of the dense equatorial forest. These
values range between 200 and 432 mm (mean annual). The lowest value ranging
between 0 and 50 mm are located in the western part on Tanzania (eastern part
of Tanganyika Lake). This region is also characterised by the small amount of
annual rainfall (< 800) compared to other region inside the basin,
«Lithosol» as type of soil Available Water content (<120 mm/root
depth).
Statistically, the soil moisture distribution over the basin
correlates strongly with the hydrological soil group and the climatic
parameters such as rainfall and Evapotranspiration. The computed soil moisture
values would be better interpreted as indexes of relative wetness rather than
absolute estimates because none are calibrated against measured values in the
field.
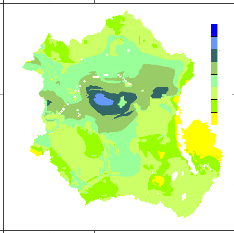
Soil Moisture
mean ann (mm)
250
200
350
300
50
0
150
100
10
5
0
-5
-10
-15
10 15 20 25 30 35
Figure 38 Mean annual moisture (in mm) over the Congo
basin.
10 15 20 25 30 35
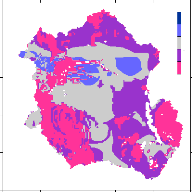
Total Soil Moisture- June-July-August (mm)
400
300
200
100
0
10 15 20 25 30 35
10 15 20 25 30 35
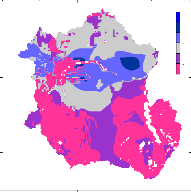
Total Soil Moisture- September-October-November
(mm)
500
400
300
200
100
0
10 15 20 25 30 35
10
5
0
-5
-10
-15
10
5 0
-5
-10 -15
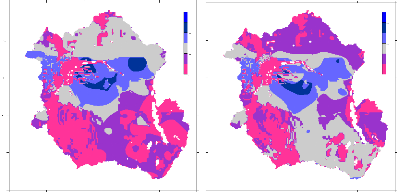
-10
-15
10
-5
5
0
-10
-15
10
-5
5
0
Total Soil Moisture- March-April-May (mm)
500
400
300
200
0
100
Total Soil Moisture- December-January-February
(mm)
500
400
300
200
0
100
Figure 39 Season Soil moisture (in mm per season) over the
basin.
5.3.2 Actual Evapotranspiration (AET)
The simulated results for the Actual Evapotranspiration (AET)
are monthly estimates for each 6 minutes grid cell covering the Congo basin.
Figures 40 and 41 show the mean annual AET and seasonal AET, respectively. The
mean annual AET ranges between 564.13 and 1576.8 mm/year with a mean of 1098
mm/year. It is well observed that the AET trends similarly with the Rainfall
and the existing land cover map of the basin. Consequntly, the area with lowest
AET is located in the south-eastern region of the basin which coincides with
low rain feed region of the area.
-10
-15
10
-5
5
0
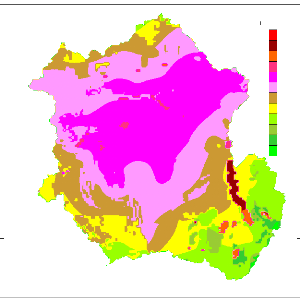
Total An nual Actual Evapotranspiration
mm/year
400
900
800
700
600
1600
1500
1400
1300
1200
1100
1000
10 15 20 25 30 35
Figure 40 Mean Annual Actual Evapotranspiration over the
Congo River basin
High annual averaged AET values are sequentially observed over
the water bodies such as the Tanganyika with a maximum of 1576.8 mm/year,
followed by Upemba, Rukwa, Mweru, Delcommune, Bangwelungu Lakes (1300 - 1531
mm/year) and Kivu, Mai-Ndombe Lakes (>1250 mm/year). Some portions of the
Congo River inside the heart of the Tropical forest present also high annual
values of AET. Excluding the water bodies, the density of the equatorial forest
varies positively with AET distribution.
The seasonal distributions of AET (FigurF41) are characterised
by alternation of high values in the northern hemisphere during the two seasons
of December-February and March-May and low values in the southern hemisphere;
inversely during the other 2 seasons ( June-August and September-November).
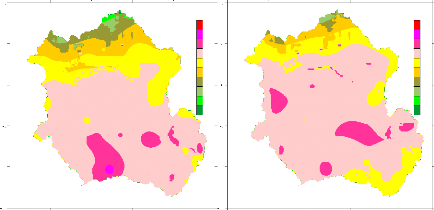
-10
10
15
5
0
5
-10
10
15
5
0
5
Actual Evapotranspiration: March-April-May
[mm]
400
250
200
500
350
300
150
100
50
0
Actual Evapotranspiration:
December-January-February
[mm]
500
400
350
300
250
200
50
0
150
100
10 15 20 25 30 35 10 15 20 25 30 35
10
5
0
5
-10
15
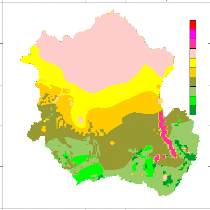
Actual Evapotranspiration: Jun-July-August
[mm]
500
400
350
300
250
200
50
0
150
100
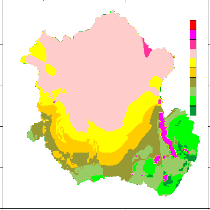
Actual Evapotranspiration:
September-October-November
[mm]
400
250
200
500
350
300
150
100
50
0
10
5 0 5
-10
15
10 15 20 25 30 35 10 15 20 25 30 35
Figure 41 Season Actual Evapotranspiration over the Congo
basin
5.3.3 Runoff
The simulated annual Total runoff is shown in Figure42. The
mean annual Runoff for for the Congo Basin varies between 1 and 1945 mm with a
mean annual runoff of 342 mm. The highest values are concentrated in the heart
of the equatorial forest along the Middle Congo river branch. This area records
higher rainfalls in the whole basin. The lowest value is simulated in the
southern hemisphere around the grid of coordinate 31 0E and and
6.730S (western part of Tanzania). A part the lakes, the highest
values of runoff are simulated in the heart of the equatorial forest across the
equator, and decrease progressively towards the tropics. This trend is
relatively disturbed with the soil types especially in the south-eastern, the
extreme north regiond and along the main Congo River. The annual simulated
runoff show a general trend strongly influenced by the distribution pattern of
precipitation (Figure 43) in the basin, while seasonal and monthly runoff
correlate strongly with both rainfall and soil type during dryer seasons. The
area with zero runoff values correspond to swamps and some inland lakes where
there is negligible or nil flow to the river system.
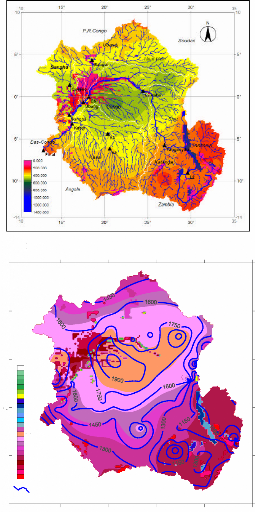
Figure 42 Mean annual runoff over Congo basin
(mm/year)
10 15 20 25 30 35
10
5
Runoff
0
[mm/year]
1350
1250
1050
850
-5
750
650
525
400
350
-10
200
100
0
Rainfall
-15
Total Annual Rainfall and Runoff
Figure 43 The relationship between precipitation and
drigged simulated runoff in the CRB
Seasonally, September-November records the highest amount
(658432 mm. /season) of runoff whereas the lowest (358223 mm/season) is
generated during June-August. This shows again the influence of rainfall on the
generated runoff pattern in the Congo basin where, in general, the period
June-July season records the lowest rainfall in the southern hemisphere which
occupies more than 55% of the basin area. Two picks runoff are observed in
March-May and September-November (658432 mm.) seasons which record higher
rainfall during the year in the southern region.
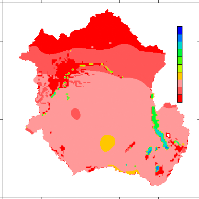
Runoff: December-January-February
[mm]
450
400
350
300
250
200
50
0
150
100
10 15 20 25 30 35
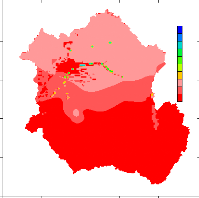
10 15 20 25 30 35
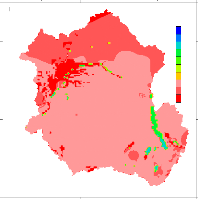
Runoff: March-April-May
[mm]
450
400
350
300
250
200
50
0
150
100
10 15 20 25 30 35
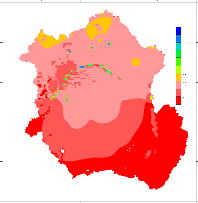
Runoff: September-October-November
[mm]
450
400
350
300
250
200
50
0
150
100
10 15 20 25 30 35
10
5 0
-5
-10
-15
10
-10
-15
10
-10
-15
10
-5
-5
5
0
5
0
Runoff: Jun-July-August
[mm]
450
400
350
300
250
200
150
100
50
0
Figure 44 Seasonal Runoff grid runoff maps. Top left:
December-February, Top right: March-May, Bottom Left: June-August, Bottom
right: September-November
5.3.4 Simulated sub-watershed and basin-wide
runoff
The runoff averages for each subwateshed are given in table 18
below. The Lualaba subcatchement knows the highest runoff than others. The
higher amount accumulated runoff was expected in the Congo but this is not the
case due to the presence of swamps in the subcachements where are simulated
small values averaged to 0 mm per year.
At the basin scale, the total annual runoff is about 47,418
m3/sec. This amount is comparable to those reported in literature
(45,000 m3/sec, Asante, 2000) with a marginal error of 5.1%.
Table 16 Subwatershed runoff averages
Area name
|
ROF ROF Simulated
Mean Ann (m3/sec) (mm/year)
|
ROF Observed (m3/sec)
|
Error (%)
|
Sangha
|
335.5 3,565
|
No data
|
-
|
Ubangi
|
348.6 8,274
|
No data
|
-
|
Kasai
|
344.7 11,545
|
No data
|
-
|
Lualaba
|
297.3 12,3 14
|
No data
|
-
|
Congo
|
385.5 12,177
|
No data
|
-
|
CRB
|
342.0 47,418
|
45,000
|
+5.1
|
Figure 45 shows the local water balance graphs for 11 selected
grid cells around selected rainfall stations used in the model. The
hydrological behavior correlates strongly with the rainfall betwenn -5 and 5
degree latitude zone. In the South-eastern region (Lubumbashi, Urambo,
South-westen) and the Up-North sation, runoff starts to increase in the
mid-year as well as the soil moisture. An inverse situation is observed in the
high rain fed region.
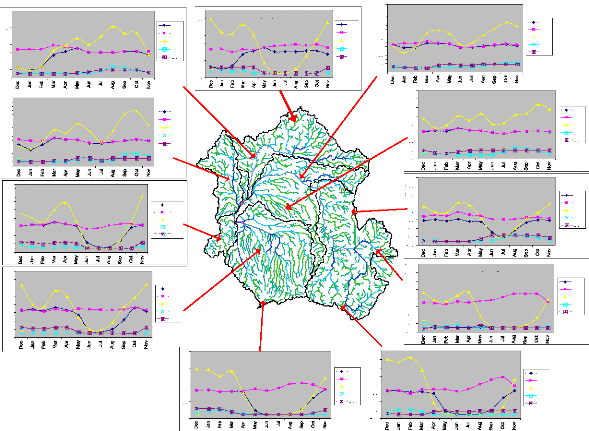
300
260
220
180
140
100
300
|
260 220 180 140 100 60 20 -20
|
OUESSO
|
|
AET PET PPT ROF SOM
|
|
|
300
|
260 220 180 140 100 60 20 -20
|
KINSHASA
|
|
AET PET PPT ROF SOM
|
|
|
300
|
260 220 180 140 100 60 20 -20
|
KANANGA
|
|
|
AET PET PPT
|
|
ROF SOM
|
|
|
|
300
|
-20
60
20
BANGUI
AET PET PPT ROF SOM
260
220
180
140
100
-20
60
20
Sangh
260
220
300
180
140
100
-20
20
60
SOUTH-WEST
Kasa
UP-NORTH
Ubangi
Cong
Lualab
AET PET PPT ROF SOM
AET PET PPT ROF SOM
300
260
220
180
140
100
60
20
20
300
260
220
180
140
100
-20
60
20
300
260
220
300
260
220
300
260
220
180
140
100
180
140
100
180
140
100
-20
60
20
60
20
20
60
20
20
LUBUMBASHI(-14, 27)
KISANGANI
BUKAVU (2852, -230)
BOYENDE
URAMBO
AET PET PPT ROF SOM
AET PET PPT ROF SOM
AET PET PPT ROF SOM
AET PET PPT ROF SOM
AET PET PPT ROF SOM
Figure 45 Local water balance for selected grid cells in
the Congo River Basin.
5.3.5 Vertical Integrated Moisture
Convergence
Figures 46 (A-D) show the Mean seasonal distribution of the
Vertically Integrated Atmospheric Moisture Convergence (C) over the Congo
Basin. The moisture convergence corresponds to the negative values whereas the
positive values correspond to the moisture divergence.
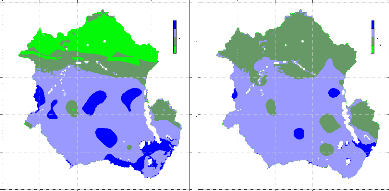
A
Vertical Convergence Moisture
CV' Dec - Feb
2
0 0
-5
-5-5
-10
-15
10
-5
5
0
Vertical Convergence Moisture
B
CV' Mar - May
-
-5-5
0 0
10
5
0
-5
-10
-15
10 15 20 25 30 35
10 15 20 25 30 35
10 15 20 25 30 35
10 15 20 25 30 35
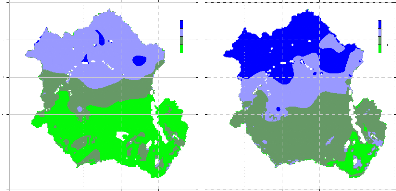
-10
-15
10
-5
5
0
Vertical Convergence Moisture
C
CV' Jun - Aug
2
0 0
-5
-5-5
-10
-15
10
-5
5
0
Vertical Convergence Moisture
D
CV' Sep - Nov
-
-5-5
0 0
Figure 46 Seasonal and Spatial distribution maps for
Vertical Integrated Moisture Convergence (in mm/month) over the Congo River
basin. A: December-February, B: March-May, C: June-August, D:
Septembre-November (Negative values correspond to the moisture convergence,
positive values correspond to the moisture divergence).
The basin is well separated into two majors and disctintive
zones based on the C parameter: The northern and the southern zones,
corresponding exactelly to the northern and the southern hemisphere,
respectively (Figures 46, A-D). Large values of Convergence (negative values)
are simulated during June-August season (in the southern hemisphere) and
December-February season (in the northern hemisphere), whereas during the same
season, the northern and southern hemispheres are characterised by moisture
divergence. During March-May season, the northern hemisphere sees its
convergence moisture moving gradually towards the southern hemisphere, and a
divergence moisture zone completely takes place at the end of June-August
season. At the same time, the southern hemisphere which was characterised by
the highest values of C during December-February starts to loose its C and
falls into a highest convergence moisture zone during
June-August. This behaviour is clearly explained from monthly observations of
C.
CHAPTER SIX
6.0 CONCLUSIONS AND RECOMMENDATIONS 6.1
Conclusions
The hydrogeomorphologic model has caracterised the Congo Basin
in term of its topography, hydrography and hydrology. The major subwatershed
(Sangha, Ubangi, Ouesso, Congo and Lualaba) are identical if considering the
stream development characteristics. Slopes values are generally more or less
negligible all over the basin. As observed from both the Hydrosheds and Hydro1k
DEM maps used, more than 60 percent of the basin is covered by a flat area
where slopes are obviously derived negligible. This flat area is surrounded by
mountainous zones in the eastern, southern and northern part of the basin. The
spatial resolution of 1000 meters used will definitely hide some ground
realities such as hill slopes of a length less than 1000 metres, surrounded by
flat area, will not be observed on the map.
Furthermore, a distributed GIS-based hydrological model,
namely Hybrid Atmospheric Water Balance (HATWAB) was developed using GIS and
computational hydrology techniques. The model is based on the combined,
NDVI-based interception abstraction of precipitation, CN method of overland
runoff and soil-moisture accounting technique for runoff generation for
specific geo-referenced grids.
Besides, rainfall and climatological data, the inputs to the
model are the GIS based agronomic soils and vegetation cover information over
the region has been reclassified into appropriate textural soils and rooting
depth and used to derive the soil moisture parameters such as field capacity
(FC), wilting point (WP) and available water content (AWC).
This enables one first to establish the hydrologic regimes of
the region, and for various drainage basins (subwatershed). It ultimately
permits a through assessment of the region's water resources availability.
The simulated annual runoff using HATWAB for the Congo basin
is found to be within 5% error margin compared to the observed mean annual
runoff, which is quite a commending outcome of the study.
Developed for the water balance studies of drainage basins in
the various climatic regimes of Africa, which is being successfully tested in
different basins, HATWAB model is a regional scale hydrological model which is
capable of estimating the spatial and temporal variation of the major
components of water balance such as the soil moisture, the actual
evapotranspiration and runoff.
First, the model has been used to create a GIS-based high
resolution data sets of soil moisture, evapotranspiratiom, grid runoff for
grids that represent the land catchment of the region at 12 km (6 minutes)
spatial resolution.
This work is the first comprehensive distributed hydrological
model in the region that utilises the hydro-climatology and hydrologic soil
information using the state of the art GIS technique computing the water
balance for the whole Congo River basin. The model and the findings of the
study reveal important information to assess the water resources potential of
the various sub-catchments and the whole Congo basin in one environment.
6.2 Recommendations
Even though the HATWAB model has simulated some realistic
parameters for the Congo basin water balance, the following recommendations are
made:
1. The soil texture data was not verified on the field. For
the entire basin, only 6 textural soil groups were classified from 133
agronomical FAO soil groups. This may also introduced some error in estimation
hydrological soil properties and therefore, in the simulation of water balance
components.
2. The NDVI data was extracted from a map of 1987 while the
climate change is affecting the Africa continent. There is a possible change in
land cover/use distribution over the area.
3. Although the above mentioned deficiencies, one will need
to point out that their influence would be negligible for such a HATWAB Model
applied simulating the water balance for a large watershed such the Congo River
basin. However, for further calibration of HATWAB model, observed or recoded
discharge data for the various sub-catchment outlets as well as the main Congo
basin outlet point need to be used.
4. With the advent of increasing computing power of the
current computers, HATWAB can give an impetus to and can throw light towards
development of refined and data intensive hydrological model as high resolution
spatial and temporal hydroclimatic information are becoming increasingly
affordable from satellite-data sources. Furthermore, this approach provides an
operational hydrological tool to deal with national, or regional planning and
developing decision support systems, with possible linkage with the
inter-related multi-sectoral nature of the natural resources and socioeconomy
of the region or the globe at large.
REFERENCES
Alemaw, B.F. and Chaoka, T.R. (2003). A continental scale water
balance model: a GISapproach for the Southern Africa. Physics and Chemistry
of the Earth, 28: 957-966.
Alemaw, B.F. (2006). A Hybrid Atmospheric and Terrestrial
Water Balance Model. A GIS based approach for large drainage basins.
Unpublished Research Report. University of Botswana.
AllAfrica, Central Africa: Congo River Basin Gets 2.44 Million
Euros Grants From the African Water Facility.
http://allafrica.com/stories/200706070044.html
(accessed: June 2007)
Allen R. G., Pereira, L.S., Raes, D. and Smith, M. (1998).
Crop evapotranspirationGuidelines for computing crop water requirements - FAO
Irrigation and drainage. Paper 56. FAO, Rome
Arnell, N.W. (1995). Grid Mapping of River Discharge. Journal
of Hydrology, 167: 39-56
Asmamaw A. (2003). Regional Scale Assessment and Modelling of
Water Balance and Soil Erosion using global climate and Internet geodata sets:
Case study of the upper Rio Grande System, Bolivia. M.Sc. thesis, ITC,
Netherlands
Baumgartner, A., and Reichel, E. (1975). The World Water Balance.
Elsevier, New York, 179 p.
Bergstrom, S., and Graham, L.P. (1998). On the scale problem in
hydrological modelling, Journal of Hydrology, 211
(1-4): 253-265.
Beven, K. (1989). Changing ideas in hydrology-The case of
physically-based models, Journal of Hydrology, 105:
157-172
Beven, K.J., Quinn, P.F., Romanowicz, R., Freer, J., Fisher,
J., and Lamp, R. (1994). TOPMODEL. Computer Models of Watershed Hydrology. V.P.
Singh (ed.), Water Resources Publications, Highlands Ranch, Colorado
BRICQUET, J.P. (1990) Régime et bilan hydrologique de
l'Afrique Centrale. In : Paysages quaternaires de l'Afrique Centrale
Atlantique. R. Lanfranchi et D. Schwartz (Eds). Publ. ORSTOM - Collection
Didactiques. Paris p. 42-51.
Brubaker, K.L., Entekhabi, D. and Eagleson, P.S. (1994).
Atmospheric Water Vapor Transport and Continental Hydrology over the Americas,
Journal of Hydrology, 155: 407- 428
Butler and Rhett A. The Congo River- Rainforest Biodiversity.
A Place Out of Time: Tropical Rainforests and the Perils They Face. (Accessed:
January 2006 from
http://rainforestsmongabay.com/0305.htm).
Chow V.T., Maidment, D.R. and Mays, L.W. (1988). Applied
hydrology, MacGraw-Hill. pp.572.
Church, M. R., Bishop, G.D. and Cassell, D.L. (1995). Maps of
Regional Evapotranspiration and Runoff/Precipitation Ratios in the Northeast
United States, Journal of Hydrology, 168: 283-298.
Congo (2008). In Encyclopædia Britannica.
(Accessed: March 2007, from Encyclopædia Britannica Online:
http://www.britannica.com/EBchecked/topic/132363/Congo-Kinshasa)
Conway, D. (1997). A water balance model of the Upper Blue Nile
in Ethiopia. Hydrologic Sciences Journal. 42(2): 265-286.
Costa, M. and Foley, J. (1999). Trends in the hydrologic cycle of
the Amazon basin. Journal of Geophysical Research,
104:14189-14198
Curtis, S., Hastenrath, S. (1999). Trends of upper-air
circulation and water vapor over equatorial South America and adjacent oceans.
International Journal of Climatology, 19:863- 876.
Daly, C., Neilson, R.P. and Phillips, D.L. (1994). A
Statistical Model for Mapping. Climatological Precipitation over Mountainous
Terrain. Journal of Applied Meteorology, 33:
140-158.
Dunne, T. and Leopold, L. B. (1978). Water in Environmental
Planning. W. H. Freeman and Company, New York, USA.
Eastman, J.R. (1997). IDRISI for windows user's guide. Clark
University, Graduate School of Geography, Worcester, Massachusetts 06610,
USA.
FAO (1984). FAO-UNESO soils map of the world revised legend,
world soils resources report, series No. 60 Rome
Eilers, V.H.M., Carter, R.C. and Rushton, K.R. (2007). A
single layer soil water balance model for estimating deep drainage (potential
recharge): An Application to cropped land in semi-arid North-east Nigeria.
Geoderma, 140:119-131.
Eltahir, E and Bras, R. (1994). Precipitation recycling in the
Amazon basin. Quaternary Journal of Royal Meteorological
Society. 120 (158):861-880.
Ewen, J., Sloan, W.T., Kilsby, C.G., and O'Connell, P.E.
(1999). UP modeling system for large scale hydrology: deriving large scale
physically-based parameters for the Arkansas-Red River basin. Hydrology and
Earth Sciences System 3.1:125-136.
FAO (of United Nations) (1988) World Soil Resources, An
explanatory Notes on the FAO world soil reference map, World Soils Resources
Report, No. 66.
Gieske A. (2002). Viewer of ASC temperature and rainfall files
per basin (CRU 1961-1990), ITC, Netherlands
Hay, L.E., and McCabe, G.J. (2002). Spatial variability in
water-balance model performance in the conterminous United States. Journal
of the American Water Resources Association, AWRA Paper 00086.
ILWIS, The integrated Land and Water Information System.
http://www.itc.nl/ilwis (accessed
10 October 2007)
IRD, 2002, Congo River Basin: Geology and Soil type influence
drought impact
Kazadi, S. and Kaoru, F. (1996). Interannual and long-term
climate variability over the Zaire River Basin during the last 30 years,
Journal of Geophysical Research, 101:351-360.
Koka, S. (2004). Integrated stream and watershed data for
hydrologic modeling. Unpublished M.Sc. thesis, Texas A&M University.
Krige, D.G. (1966). Two-dimensional weighted moving average
trend surfaces for orevaluation. Procedings of the Symposium on Mathematical
Statistics and Computer Applications in Ore Valuation. Journal of South
African Institute. Mining and Metallurgy, Johannesbur; 7-8 March 1966,
13-38.
Kwadijk, J.C.J. (1993). The impact of climate change on the
discharge of the River Rhine. Unpublished PhD thesis, Dept. of Physical
Geography, University of Utrecht.
Levy et al ( ) Soil Water Monitoring and Measurement. Pacific
Northwest Publication, Washington.
http://cru.cahe.wsu.edu/CEPublications/pnw0475/pnw0475
(accessed: December 2007).
Lullwitz, T. and Helbig, A. (1995). Grid Related Estimates of
Streamflow within the Weser River Basin, Germany. Modeling and Management of
Sustainable Basin-scale Water Resource Systems, Symposium Proceedings, Boulder,
Colorado, IAHS Publ. No. 231, 1995.
Maathuis, B. (2006). DEM based Hydro-Processing: Introduction
to the tools developed, tutorial with exercises (Version 1). International
Institute for Geo-information Sciences and Earth Observation (ITC), Enschede,
71 pp
Marengo, J.A. (2005). Characteristics and spatio-temporal
variability of the Amazon River Basin Water Budget Climate. Dynamics,
24: 11 -22.
McCuen, R.H. (2005). Hydrologic Analysis and Design.
3rd Ed., Pearson Prentice Hall, New Jersey
Musy, A. (2001). e-drologie. Ecole Polytechnique
Fédérale, Lausanne, Suisse
Moteva, M., Kazadjiev, V. and Georgieva, V. (2007). Investigation
of FAO Penman-Monteith Reference Evapotranspiration over the Territory of
Bulgaria, 7th EMS Annual Meeting.
New, M., Hulme, M. and Jones, P. (2000). Representing
twentieth-century space-time climate variability. Part II: development of
1901-1996 monthly grids of terrestrial surface climate. Journal of
Climatology, 13:2217-2238.
Oki, T., Musiake, K. Matsuyama, H. and Masuda, K. (1994).
Global Atmospheric Water Balance and Runoff from Large River Basins. Scale
Issues in Hydrological Modeling, Chapter 24, 411-434.
Olivera, F. (2002). Applications of GIS to Water Resources
Engineering, University of Texas at Austin. Online document:
http://civil.ce.utexas.edu
(Accessed: July 2007)
Olivry, J.C., Bricquet J.P. et MAHE, G. (1993). Les
études du PEGI sur le Bassin du Congo- Zaïre dans le Contexte
Déficitaire des Ressources en Eau de l'Afrique Humide. In Grands
Bassins Fluviaux, Paris, 22-24 november 1993.
Reed, S.M., J. Patoux, D.R. Maidment (1997). CRWR Online Report
97-1: Spatial Water Balance of Texas, CRWR, University of Texas at
Austin.
www.crwr.utexas.edu/reports1997/rpt97_1/sect3.htm (Accessed: January
2008).
Refsgaard, J.C. (1996). Terminology, modeling protocol and
classification of hydrological model codes. In Abbott, M.B. and Refsgaard, J.C.
(eds,): Distributed hydrological modelling. Kluwer Academic Publishers,
Dordrecht, pp. 17-3 9.
Refsgaard, J.C. (1998). Conceptual versus physically-based
hydrological models: Which models to be used for BALTEX purposes?: Proceedings
of the 2nd Study Conference on BALTEX, Rügen, Germany, 25-29 May,
180-184.
Refsgaard, J.C., Alley, W.M., and Vuglinsky, V.S. (1989).
Methodology for distinguishing between man's influence and climate effects on
the hydrological cycle. IHP-III Project 6.3, Technical Documents in Hydrology,
UNESCO, Paris.
Roads J., Chen S., Guetter A. and Georgakakos K. (1994).
Large-scale aspects of the United States Hydrologic Cycle. Bulletin of
American Meteorological Society, 75:1589-1610
Saxton, K.E., Rawls, W.J., Romberger, J.S. and Papendick, R.I.
(1986). Estimating generalized Soil-Water Characterstics from Texture. Soil
SCI. SOC. AM. J., Vol. 50
SCS (1986). Urban Hydrology for Small Watersheds. Soil
Conservation Society of the US Department of Agriculture (USDA). Technical
Release 55.
Sen, K.P and A. Gieske (2005). Use of GIS and Remote Sensing
in identifying recharge zones in an arid catchment: a case study of Roxo River
basin, Portugal. Journal of Nepal Geological Society, 31: 25-32.
Singh, V. P. (1988). Hydrologic systems: Rainfall-runoff
modelling. Volume 1. Prentice Hall, Englewood cliffs, New Jersey, pp
480.
Stein, A. (1998). Spatial statistics for soils and the
environment. Unpublished lecture notes. ITC, 47 pp.
Sukheswalla, Z.R. (2003). A statistical model for estimating mean
annual and mean monthly flows at ungaged locations. Unpublished M.Sc. thesis,
Texas A&M University.
The Living Africa, 1998.
http://library.advanced.org/16645/the_land/congo_river.html
(Accessed June 2007)
Thakur, P. K., Bhardwaj A. and Aggarwal. S.P. Hydrological
parameter extraction and analysis of Mangala Valles of Mars in the
Seventh International Conference on Mars
Thornthwaite, C.W. and Mather, J.R. (1955). The Water Balance.
Drexel Institute of Technology, Laboratory of Climatology, USA.
Thornthwaite, C.W. and Mather, J.R. (1957). Instructions and
Tables for computing potential evapotranspiration and the water balance, Drexel
Inst. Techno. Publ. Clim., X(3)
UNEP (2006). Congo River to Power Africa Out of Poverty.
United Nations Environment Programme (UNEP) online document:
http://www.unep.org/Documents.Multilingual
(Accessed: June 2007)
Vorosmarty, C.J., Fekete, B.M. and Tucker, B.A. (1998). Global
River Discharge Database (RivDis). V. 1.1.
http://www-eosdis.ornl.gov
(Accessed: June 2007)
Willmott, C.J., Rowe, C.M. and Mintz, Y. (1985). Climatology of
the Terrestrial Seasonal Water Cycle. Journal of Climatology,
5: 589-606,
Wolock, D. M., and McCabe, G. J. (1999). Explaining spatial
variability in mean annual runoff in the conterminous United States.
Climate Research, 11: 149-159.
Zapata M.A.V. (2003). Development of an ArcGIS interface and
design of a Geodatabase for the Soil and Water assessment Toll. Unpublished
M.Sc. thesis, Texas A&M University.
Zeng N. (1999). Seasonal cycle and interannual variability in the
Amazon hydrologic cycle. Journal of Geophysical Research,
104:9097-9106
www.itc.pub\adapt (visited on 10 June 2007)
www.libraries.psu.edu/crsweb/maps/
(visited on 15 June 2007)
www.wikipedia.com (Wikipedia the
Free Encyclopedia, Accessed, June 2007)
APPENDICES
APPENDIX 1: METEOROLOGICAL STATIONS COVERING THE STUDY
AREA (FROM FAO/UNESCO CLIMWAT DATBASE)
ANG: Angola, CAF: Cantral Africa, CMR, Cameroon, PRC: People
Republic of the Congo, URT: United Republic of Tanzania ZAI = Zaire
(D.R.Congo), ZAM: Zambia .
COUNTRY
|
STATION NAME
|
LONGITUDE (degree)
|
LATITUDE (degree)
|
ELEVATION (m)
|
ZAI.
|
Bafwasende
|
27.28
|
1.05
|
524
|
ZAI.
|
Bambesa
|
25.43
|
3.27
|
621
|
ZAI.
|
Banana
|
12.35
|
-6
|
2
|
ZAI.
|
Bandundu
|
17.21
|
-3.18
|
324
|
ZAI.
|
Basankusu
|
19.48
|
1.13
|
353
|
ZAI.
|
Basoko
|
23.36
|
1.15
|
366
|
ZAI.
|
Bena-Longo
|
21.4
|
-4.51
|
578
|
ZAI.
|
Binga
|
20.3
|
2.18
|
400
|
ZAI.
|
Boende
|
20.51
|
-0.13
|
351
|
ZAI.
|
Boketa
|
19.46
|
3.11
|
475
|
ZAI.
|
Bokondji
|
21.26
|
-0.43
|
365
|
ZAI.
|
Bongabo
|
20.32
|
3.06
|
450
|
ZAI.
|
Bukavu
|
28.52
|
-2.3 1
|
1612
|
ZAI.
|
Bumba
|
22.33
|
2.11
|
361
|
ZAI.
|
Bunia
|
30.13
|
1.3
|
1239
|
ZAI.
|
Buta
|
24.47
|
2.5
|
410
|
ZAI.
|
Butembo
|
29.16
|
0.08
|
1840
|
ZAI.
|
Eala
|
18.18
|
0.03
|
350
|
ZAI.
|
Gandajika
|
23.57
|
-6.45
|
780
|
ZAI.
|
Gemena
|
19.47
|
3.17
|
446
|
ZAI.
|
Gimbi-Plateau
|
13.22
|
-5.3 1
|
480
|
ZAI.
|
Goma
|
29.14
|
-1.41
|
1552
|
ZAI.
|
Ilebo
|
20.35
|
-4.2
|
420
|
ZAI.
|
Inongo
|
18.16
|
-1.58
|
299
|
ZAI.
|
Irumu
|
29.52
|
1.27
|
955
|
ZAI.
|
Isiro
|
27.39
|
2.46
|
764
|
ZAI.
|
Kalemie
|
29.11
|
-5.53
|
776
|
ZAI.
|
Kamina
|
25
|
-8.44
|
1105
|
ZAI.
|
Kamina - Base
|
25.15
|
-8.38
|
1106
|
ZAI.
|
Kananga
|
22.25
|
-5.53
|
657
|
ZAI.
|
Kaniama
|
24.09
|
-7.25
|
949
|
ZAI.
|
Kibangula
|
27.04
|
-4.52
|
685
|
ZAI.
|
Kikwit
|
18.48
|
-5.02
|
449
|
ZAI.
|
Kindu
|
25.55
|
-2.57
|
497
|
ZAI.
|
Kinshasa
|
15.22
|
-4.22
|
310
|
ZAI.
|
Kisanga-Plateau
|
27.25
|
-11.44
|
1187
|
ZAI.
|
Kisangani
|
25.11
|
0.31
|
396
|
ZAI.
|
Kiyaka-Plateau
|
18.57
|
-5.16
|
739
|
ZAI.
|
Kolwezi
|
25.28
|
-10.45
|
1526
|
ZAI.
|
Kondo
|
12.58
|
-5.34
|
230
|
ZAI.
|
Kongolo
|
27
|
-5.2 1
|
561
|
ZAI.
|
Kutubongo
|
19.53
|
-4.37
|
550
|
ZAI.
|
Libenge
|
18.38
|
3.38
|
359
|
ZAI.
|
Lisala
|
21.34
|
2.19
|
463
|
ZAI.
|
Lodja
|
23.28
|
-3.29
|
479
|
ZAI.
|
Loeka
|
22.42
|
2.12
|
380
|
ZAI.
|
Lomela
|
23.17
|
-2.18
|
427
|
ZAI.
|
Lubao
|
25.45
|
-5.18
|
694
|
ZAI.
|
Lubarika
|
28.57
|
-2.5
|
980
|
ZAI.
|
Lubumbashi Luano
|
27.29
|
-11.4
|
1298
|
ZAI.
|
Luki-Plateau
|
13.06
|
-5.37
|
350
|
ZAI.
|
Lukolela
|
17.1
|
-1.04
|
318
|
ZAI.
|
Luputa
|
23.44
|
-7.08
|
877
|
ZAI.
|
Lusambo
|
23.26
|
-4.58
|
424
|
ZAI.
|
M'Vuazi-Poste
|
14.54
|
-5.27
|
505
|
ZAI.
|
Magombo
|
27.54
|
3.42
|
705
|
ZAI.
|
Manono
|
27.26
|
-7.17
|
633
|
ZAI.
|
Mbandaka
|
18.16
|
0.03
|
317
|
ZAI.
|
Mbuji-Mayi
|
23.37
|
-6.1
|
677
|
ZAI.
|
Mitwaba
|
27.2
|
-8.56
|
1579
|
ZAI.
|
Mont Delele
|
29.47
|
4.22
|
900
|
ZAI.
|
Mont Hawa
|
30.45
|
2.49
|
1350
|
ZAI.
|
Mukumari
|
23.11
|
-2.5
|
535
|
ZAI.
|
Mulungu Bukulumisa
|
28.43
|
-2.2
|
2378
|
ZAI.
|
Mutombo - Yamfu
|
16.22
|
-5.19
|
700
|
ZAI.
|
Mutsora
|
29.44
|
0.19
|
1330
|
ZAI.
|
N'Dihira
|
29.1
|
-0.16
|
2190
|
ZAI.
|
Nioka-Drusi
|
30.39
|
2.09
|
1678
|
ZAI.
|
Opala
|
24.21
|
-0.35
|
398
|
ZAI.
|
Rumangabo
|
29.22
|
-1.21
|
1620
|
ZAI.
|
Rwindi
|
29.17
|
-0.47
|
1040
|
ZAI.
|
Simama
|
27.01
|
-9.37
|
852
|
ZAI.
|
Tshikapa
|
20.5 1
|
-6.25
|
481
|
ZAI.
|
Tukpwo
|
25.55
|
4.27
|
704
|
ZAI.
|
Watsa
|
29.3
|
3.04
|
1008
|
ZAI.
|
Yaligimba
|
22.51
|
2.17
|
435
|
ZAI.
|
Yangambi E.C.P.
|
24.31
|
0.53
|
491
|
ZAI.
|
Yangambi Km 5
|
24.29
|
0.49
|
470
|
ZAM.
|
Chipata
|
32.35
|
-13.33
|
1032
|
ZAM.
|
Kabompo
|
24.12
|
-13.36
|
1075
|
ZAM.
|
Kabwe
|
28.28
|
-14.27
|
1207
|
ZAM.
|
Kaoma
|
24.48
|
-14.48
|
1213
|
ZAM.
|
Kasama
|
31.08
|
-10.13
|
1384
|
ZAM.
|
Kasempa
|
25.51
|
-13.32
|
1234
|
ZAM.
|
Kawambwa
|
29.05
|
-9.48
|
1324
|
ZAM.
|
Lundazi
|
33.12
|
-12.17
|
1143
|
ZAM.
|
Mansa
|
28.51
|
-11.06
|
1259
|
ZAM.
|
Mbala
|
31.2
|
-8.51
|
1673
|
ZAM.
|
Mpika
|
31.36
|
-11.54
|
1402
|
ZAM.
|
Mwinilunga
|
24.26
|
-11.45
|
1363
|
ZAM.
|
Ndola
|
28.39
|
-13
|
1270
|
ZAM.
|
Petauke
|
31.17
|
-14.15
|
1036
|
ZAM.
|
Samfya
|
29.32
|
-11.21
|
1172
|
ZAM.
|
Serentje
|
30.13
|
-13.14
|
1384
|
ZAM.
|
Solwezi
|
26.23
|
-12.11
|
1386
|
ZAM.
|
Zambezi
|
23.07
|
-13.32
|
1078
|
ANG.
|
Cabinda
|
12.11
|
-5.33
|
20
|
ANG.
|
Chitembo
|
16.46
|
-13.32
|
1500
|
ANG.
|
Cuemba
|
18.05
|
-12.08
|
1300
|
ANG.
|
Huambo
|
15.45
|
-12.48
|
1700
|
ANG.
|
Lobito
|
13.32
|
-12.22
|
3
|
ANG.
|
Luanda
|
13.14
|
-8.51
|
70
|
ANG.
|
Luau
|
22.15
|
-10.43
|
1100
|
ANG.
|
Lubango
|
13.34
|
-14.56
|
1758
|
ANG.
|
Luena
|
19.55
|
-11.47
|
1357
|
ANG.
|
Malanje
|
16.22
|
-9.33
|
1142
|
ANG.
|
Menongue
|
17.41
|
-14.39
|
1343
|
ANG.
|
Ndalatando
|
14.57
|
-9.19
|
690
|
ANG.
|
Nova Gaia
|
17.33
|
-10.04
|
1260
|
ANG.
|
Saurimo
|
20.24
|
-9.39
|
1080
|
ANG.
|
Soyo
|
12.21
|
-6.07
|
1
|
ANG.
|
Sunginge
|
16.47
|
-8.46
|
630
|
PRC.
|
Brazzaville
|
15.15
|
-4.15
|
316
|
PRC.
|
Djambala
|
14.46
|
-2.32
|
790
|
PRC.
|
Impfondo
|
18.04
|
1.37
|
326
|
PRC.
|
Loubomo
|
12.42
|
-4.12
|
330
|
PRC.
|
M'Pouya
|
16.13
|
-2.37
|
312
|
PRC.
|
Makoua
|
15.35
|
0.01
|
379
|
PRC.
|
Mouyondzi
|
13.55
|
-3.59
|
512
|
PRC.
|
Ouesso
|
16.03
|
1.37
|
352
|
PRC.
|
Pointe Noire
|
11.54
|
-4.49
|
17
|
URT.
|
Biharamulo
|
31.19
|
-2.38
|
1480
|
URT.
|
Bukoba
|
31.49
|
-1.2
|
1137
|
URT.
|
Chunya
|
33.26
|
-8.32
|
1500
|
URT.
|
Igawa
|
34.23
|
-8.46
|
1070
|
URT.
|
Igeri
|
34.4
|
-9.4
|
2250
|
URT.
|
Kigoma
|
29.38
|
-4.53
|
885
|
URT.
|
Mbeya Airfield
|
33.28
|
-8.56
|
1707
|
URT.
|
Musoma
|
33.48
|
-1.3
|
1147
|
URT.
|
Muze
|
3 1.33
|
-7.4
|
810
|
URT.
|
Mwanza Airfield
|
32.55
|
-2.28
|
1140
|
URT.
|
Njombe
|
34.45
|
-9.25
|
1890
|
URT.
|
Sumbawanga
|
31.36
|
-7.57
|
1710
|
URT.
|
Tabora Airport
|
32.5
|
-5.05
|
1190
|
URT.
|
Tabora Observatory
|
32.49
|
-5.02
|
1265
|
URT.
|
Urambo
|
32.03
|
-5.04
|
1106
|
CAF.
|
Bambari
|
20.39
|
5.51
|
475
|
CAF.
|
Bangassou
|
22.5
|
4.44
|
500
|
CAF.
|
Bangui
|
18.3 1
|
4.24
|
366
|
CAF.
|
Berberati
|
15.48
|
4.15
|
583
|
CAF.
|
Bossangoa
|
17.26
|
6.29
|
465
|
CAF.
|
Bouar
|
15.38
|
5.58
|
1020
|
CAF.
|
Bria
|
21.59
|
6.32
|
584
|
CAF.
|
N'Dele
|
20.39
|
8.24
|
510
|
CMR.
|
Batouri
|
14.22
|
4.28
|
656
|
CMR.
|
Garoua
|
13.23
|
9.2
|
244
|
CMR.
|
Koundja
|
10.45
|
5.39
|
1210
|
CMR.
|
Lomie
|
13.37
|
3.09
|
624
|
CMR.
|
Ngaoundere
|
13.34
|
7.21
|
1104
|
CMR.
|
Yaounde
|
11.31
|
3.5
|
760
|
APPENDIX 2: SPREADSHEET MODEL FOR THE PENMAN-MONTEITH
CALCULATION METHOD OF ETo (After Allen et al, 1998)
PENMAN-MONTEITH CALCULATIONS
|
|
Grass
|
Alfalfa
|
Give :
|
Station name :
|
|
Gamma* Cff
|
Gamma*Cff
|
|
Latitude :
|
|
Lonitude
|
0.34
|
0.42
|
|
Altitude :
|
|
|
|
Parameters :
|
Short Wave Rad
|
|
a =
|
0.25
|
b = 0.50
|
alpha=
|
Albedo
|
|
|
alpha =
|
0.23
|
|
|
|
Long Wave Rad.
|
|
a =
|
0.90
|
b = 0.10
|
|
|
al =
|
0.34
|
bl = -0.139
|
|
|
|
Instrument height
|
|
|
wind
|
temp
|
Cropheight
|
AeroT Cff
|
|
AerDyn Resistance
|
ra * U =
|
206
|
200
|
190
|
12
|
900
|
|
|
|
Grass
|
Alfalfa
|
|
|
|
Canopy resistance
|
rc =
|
70
|
86
|
|
12
|
|
|
|
JAN
|
FEB
|
MAR
|
APR
|
MAY
|
JUN
|
JUL
|
AUG
|
SEP
|
OCT
|
NOV
|
DEC
|
YEAR
|
Tmax
|
12.3
|
13.6
|
15.6
|
19.8
|
23.0
|
28.0
|
31.0
|
31.2
|
28.1
|
22.7
|
17.1
|
4.8
|
|
Tmin
|
3.7
|
4.0
|
5.6
|
7.6
|
12.6
|
15.4
|
18.0
|
18.0
|
15.6
|
11.6
|
7.7
|
82
|
|
RHmean
|
81
|
77
|
72
|
74
|
69
|
65
|
59
|
61
|
70
|
77
|
81
|
58
|
|
RHmin
|
58
|
53
|
49
|
46
|
47
|
41
|
37
|
38
|
45
|
51
|
57
|
190
|
|
Wind (km/d)
|
190
|
190
|
190
|
156
|
156
|
156
|
156
|
121
|
121
|
121
|
190
|
3.50
|
|
Sunhours
|
4.20
|
4.30
|
5.80
|
7.00
|
8.40
|
9.00
|
10.60
|
9.70
|
8.00
|
6.00
|
4.00
|
1.47
|
|
ET fao
|
1.47
|
1.87
|
2.57
|
3.26
|
4.08
|
4.94
|
5.77
|
5.21
|
4.10
|
2.76
|
1.91
|
9.20
|
|
Avg Temp
|
8.00
|
8.80
|
10.60
|
13.70
|
17.80
|
21.70
|
24.50
|
24.60
|
21.85
|
17.15
|
12.40
|
34%
|
|
n/N
|
40%
|
39%
|
49%
|
55%
|
63%
|
65%
|
78%
|
75%
|
66%
|
53%
|
38%
|
2.20
|
|
Wind (m/s)
|
2.20
|
2.20
|
2.20
|
1.81
|
1.81
|
1.81
|
1.81
|
1.40
|
1.40
|
1.40
|
2.20
|
1.56
|
|
Ea(Tmax)
|
1.43
|
1.56
|
1.77
|
2.31
|
2.81
|
3.78
|
4.49
|
4.54
|
3.80
|
2.76
|
1.95
|
0.86
|
|
Ea(Tmin)
|
0.80
|
0.81
|
0.91
|
1.04
|
1.46
|
1.75
|
2.06
|
2.06
|
1.77
|
1.37
|
1.05
|
1.21
|
|
Ea(Tx)-Ea(Tn)
|
1.11
|
1.19
|
1.34
|
1.68
|
2.13
|
2.76
|
3.28
|
3.30
|
2.79
|
2.06
|
1.50
|
0.91
|
|
Edew
|
0.83
|
0.82
|
0.87
|
1.06
|
1.33
|
1.55
|
1.67
|
1.73
|
1.69
|
1.41
|
1.11
|
82%
|
|
RH(max-min)
|
81%
|
77%
|
72%
|
74%
|
69%
|
65%
|
59%
|
61%
|
70%
|
77%
|
81%
|
0.08
|
|
Dlt(ETx-ETn)
|
0.08
|
0.08
|
0.09
|
0.11
|
0.13
|
0.17
|
0.19
|
0.19
|
0.17
|
0.13
|
0.10
|
101.3
|
|
P-atm.
|
101.3
|
101.3
|
101.3
|
101.3
|
101.3
|
101.3
|
101.3
|
101.3
|
101.3
|
101.3
|
101.3
|
2.48
|
|
lambda
|
2.48
|
2.48
|
2.48
|
2.47
|
2.46
|
2.45
|
2.44
|
2.44
|
2.45
|
2.46
|
2.47
|
0.07
|
|
gamma
|
0.07
|
0.07
|
0.07
|
0.07
|
0.07
|
0.07
|
0.07
|
0.07
|
0.07
|
0.07
|
0.07
|
70
|
|
rc
|
70
|
70
|
70
|
70
|
70
|
70
|
70
|
70
|
70
|
70
|
70
|
94
|
|
ra
|
94
|
94
|
94
|
114
|
114
|
114
|
114
|
147
|
147
|
147
|
94
|
0.12
|
|
gamma*
|
0.12
|
0.12
|
0.12
|
0.11
|
0.11
|
0.11
|
0.11
|
0.10
|
0.10
|
0.10
|
0.12
|
0.41
|
|
dl/dl+gm*
|
0.39
|
0.41
|
0.43
|
0.50
|
0.55
|
0.61
|
0.64
|
0.66
|
0.63
|
0.57
|
0.46
|
0.34
|
|
gm/dl+gm*
|
0.35
|
0.34
|
0.33
|
0.31
|
0.28
|
0.25
|
0.22
|
0.23
|
0.25
|
0.29
|
0.31
|
0.71
|
|
Aeroterm
|
0.70
|
0.87
|
1.08
|
1.08
|
1.26
|
1.64
|
1.97
|
1.53
|
1.18
|
0.84
|
0.85
|
12
|
|
Month
|
1
|
2
|
3
|
4
|
5
|
6
|
7
|
8
|
9
|
10
|
11
|
351
|
|
dayno
|
15
|
46
|
76
|
107
|
137
|
168
|
198
|
229
|
259
|
290
|
320
|
-0.408
|
|
soldeclin
|
-0.370
|
-0.230
|
-0.033
|
0.179
|
0.334
|
0.408
|
0.372
|
0.233
|
0.036
|
-0.176
|
-0.336
|
-0.186
|
|
xx
|
-0.170
|
-0.107
|
-0.016
|
0.083
|
0.154
|
0.186
|
0.170
|
0.108
|
0.017
|
-0.082
|
-0.155
|
0.810
|
|
yy
|
0.823
|
0.860
|
0.882
|
0.869
|
0.834
|
0.811
|
0.823
|
0.859
|
0.882
|
0.869
|
0.834
|
1.34
|
|
omega
|
1.36
|
1.45
|
1.55
|
1.67
|
1.76
|
1.80
|
1.78
|
1.70
|
1.59
|
1.48
|
1.38
|
1.03
|
|
dr
|
1.03
|
1.02
|
1.01
|
0.99
|
0.98
|
0.97
|
0.97
|
0.98
|
0.99
|
1.01
|
1.02
|
20.92
|
|
Ra
|
22.25
|
26.84
|
32.52
|
37.40
|
40.01
|
40.91
|
40.33
|
38.04
|
33.89
|
28.21
|
23.28
|
10.23
|
|
N
|
10.41
|
11.04
|
11.86
|
12.73
|
13.42
|
13.77
|
13.59
|
12.96
|
12.15
|
11.27
|
10.57
|
6.8
|
|
Rns
|
7.7
|
9.2
|
12.4
|
15.1
|
17.3
|
18.2
|
19.9
|
18.3
|
15.1
|
11.2
|
7.9
|
0.41
|
|
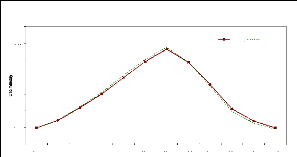
4.oe
2.oe
7.00
6.00
5.oe
3.oe
0.00
1.oe
JAN FEB MAR APR MAY JUN JUL AUG SEP OCT NOV DEC
FAO Penman-Monteith - ETo Rei. Evapotranspiration Rome
ETo (-G) ETo
f(n/N)
|
0.46
|
0.45
|
0.54
|
0.59
|
0.66
|
0.69
|
0.80
|
0.77
|
0.69
|
0.58
|
0.44
|
31.19
|
|
sigma(TxTn)
|
30.66
|
31.02
|
31.83
|
33.27
|
35.19
|
37.14
|
38.58
|
38.63
|
37.21
|
34.88
|
32.64
|
0.21
|
|
emissivity
|
0.21
|
0.21
|
0.21
|
0.20
|
0.18
|
0.17
|
0.16
|
0.16
|
0.16
|
0.18
|
0.19
|
6.46
|
|
Rbo
|
6.53
|
6.62
|
6.69
|
6.53
|
6.32
|
6.18
|
6.18
|
6.06
|
5.91
|
6.10
|
6.31
|
2.64
|
|
LWR
|
3.03
|
2.99
|
3.62
|
3.89
|
4.20
|
4.26
|
4.96
|
4.69
|
4.10
|
3.54
|
2.79
|
4.14
|
|
Rn (Rns-Rl)
|
4.71
|
6.20
|
8.76
|
11.23
|
13.14
|
13.91
|
14.91
|
13.59
|
11.01
|
7.67
|
5.09
|
-0.45
|
|
G
|
-0.17
|
0.11
|
0.25
|
0.43
|
0.57
|
0.55
|
0.39
|
0.01
|
-0.39
|
-0.66
|
-0.67
|
4.59
|
|
Rn-G
|
4.88
|
6.09
|
8.51
|
10.79
|
12.57
|
13.36
|
14.51
|
13.57
|
11.40
|
8.33
|
5.75
|
0.69
|
|
Rad Term
|
0.75
|
1.02
|
1.53
|
2.27
|
2.95
|
3.44
|
3.90
|
3.68
|
2.82
|
1.77
|
0.94
|
0.76
|
|
Rad Term(-G)
|
0.77
|
1.00
|
1.49
|
2.18
|
2.82
|
3.30
|
3.80
|
3.67
|
2.92
|
1.92
|
1.06
|
1.40
|
|
ETcomb
|
1.44
|
1.88
|
2.61
|
3.35
|
4.21
|
5.07
|
5.87
|
5.21
|
4.01
|
2.60
|
1.79
|
-5.3%
|
|
|
-1.8%
|
1.0%
|
1.7%
|
2.6%
|
3.1%
|
2.7%
|
1.7%
|
0.1%
|
-2.5%
|
-5.8%
|
-6.9%
|
1.47
|
|
ET (-G)
|
1.47
|
1.87
|
2.57
|
3.26
|
4.08
|
4.94
|
5.77
|
5.21
|
4.10
|
2.76
|
1.91
|
13.6
|
|
APPENDIX 3: CONGO RIVER DISCHARGE DATA AT
KINSHASA
Station: Kinshasa
Latitude: 4.3o S/ Longitude: 15.3o E River:
Zaire
Country: Zaire
Elevation: m
Area: 3475000 km2
-
|
Jan
|
Feb
|
Mar
|
Apr
|
May
|
Jun
|
Jul
|
Aug
|
Sep
|
Oct
|
Nov
|
Dec
|
Ann
|
m 3 /c
|
47494
|
37649
|
34713
|
37172
|
39150
|
36717
|
31703
|
31087
|
36366
|
43172
|
51708
|
56082
|
40251
|
mm
|
36.6
|
26.4
|
26.8
|
27.7
|
30.2
|
27.4
|
24.4
|
24
|
27.1
|
33.3
|
38.6
|
43.2
|
366
|
km 3
|
127
|
91.9
|
93
|
96.3
|
105
|
95.2
|
84.9
|
83.3
|
94.3
|
116
|
134
|
150
|
1270
|
l/s/km 2
|
13.7
|
10.8
|
9.99
|
10.7
|
11.3
|
10.6
|
9.12
|
8.95
|
10.5
|
12.4
|
14.9
|
16.1
|
11.6
|
%%
|
9.83
|
7.79
|
7.19
|
7.7
|
8.11
|
7.6
|
6.56
|
6.44
|
7.53
|
8.94
|
10.7
|
11.6
|
100
|
Year
|
Jan
|
Feb
|
Mar
|
Apr
|
May
|
Jun
|
Jul
|
Aug
|
Sep
|
Oct
|
Nov
|
Dec
|
Annual
|
1903
|
45250
|
34860
|
32410
|
32820
|
34970
|
34060
|
30850
|
31160
|
36900
|
41280
|
48300
|
51020
|
37823
|
1904
|
42390
|
34510
|
33760
|
39600
|
40510
|
36810
|
28700
|
31960
|
38950
|
45330
|
52340
|
53850
|
39893
|
1905
|
46230
|
36340
|
33320
|
33460
|
29970
|
26080
|
22350
|
24180
|
28120
|
34450
|
42420
|
49960
|
33907
|
1906
|
47700
|
37050
|
36550
|
37620
|
36910
|
34410
|
30660
|
27280
|
30910
|
40750
|
46980
|
48310
|
37928
|
1907
|
37680
|
33130
|
31490
|
31900
|
35110
|
30730
|
24100
|
23980
|
29390
|
35610
|
42180
|
45680
|
33415
|
1908
|
38270
|
30120
|
28350
|
31350
|
34390
|
33840
|
31320
|
30410
|
37370
|
44340
|
55350
|
65760
|
38406
|
1909
|
53560
|
38810
|
35460
|
42050
|
50530
|
48140
|
37290
|
36560
|
37940
|
48170
|
53600
|
54350
|
44705
|
1910
|
49470
|
39480
|
34890
|
34120
|
41150
|
37400
|
29960
|
33800
|
36260
|
44380
|
53550
|
60160
|
41218
|
1911
|
47110
|
34390
|
30640
|
34150
|
36560
|
39260
|
30290
|
26080
|
31460
|
37200
|
46500
|
55440
|
37423
|
1912
|
47720
|
38260
|
34750
|
35870
|
33930
|
30660
|
30930
|
31320
|
37580
|
46680
|
52490
|
53260
|
39454
|
1913
|
42280
|
34110
|
34170
|
34160
|
39650
|
37930
|
29690
|
27730
|
29040
|
35120
|
42220
|
40930
|
35586
|
1914
|
33330
|
26910
|
30320
|
34750
|
32310
|
31780
|
28950
|
26280
|
29980
|
40460
|
49340
|
56810
|
35102
|
1915
|
47450
|
31100
|
28730
|
29230
|
29470
|
28440
|
25220
|
25360
|
30420
|
35730
|
41010
|
47170
|
33278
|
1916
|
41840
|
31490
|
30370
|
31980
|
37570
|
32240
|
29100
|
27760
|
33810
|
43340
|
54960
|
59680
|
37845
|
1917
|
49120
|
38390
|
35010
|
37150
|
42470
|
41220
|
32990
|
29270
|
35990
|
44720
|
55480
|
55920
|
41477
|
1918
|
39130
|
29280
|
27900
|
33170
|
34090
|
29790
|
27440
|
29550
|
35470
|
37520
|
41500
|
43840
|
34057
|
1919
|
39500
|
33620
|
30380
|
30890
|
30240
|
26380
|
23740
|
26810
|
30550
|
36220
|
41510
|
44630
|
32873
|
1920
|
39060
|
30780
|
27230
|
29740
|
34670
|
32090
|
29370
|
32750
|
38460
|
42530
|
50110
|
59540
|
37194
|
1921
|
52780
|
37570
|
32740
|
32900
|
37460
|
34180
|
32650
|
30590
|
38200
|
44720
|
53430
|
55850
|
40256
|
1922
|
43250
|
36260
|
33300
|
33260
|
32760
|
29720
|
26750
|
25830
|
33390
|
41970
|
53000
|
58140
|
37303
|
1923
|
44370
|
33990
|
29540
|
34370
|
41650
|
40550
|
32370
|
33700
|
39390
|
44010
|
50440
|
59870
|
40354
|
1924
|
49770
|
38820
|
30880
|
36310
|
42360
|
41450
|
30620
|
30610
|
37450
|
44910
|
57970
|
63370
|
42043
|
1925
|
49950
|
34770
|
32720
|
34610
|
34580
|
34160
|
34020
|
32180
|
37050
|
44900
|
55210
|
64170
|
40693
|
1926
|
60480
|
40870
|
34900
|
39780
|
43090
|
38090
|
31770
|
31820
|
38150
|
44480
|
55730
|
61660
|
43402
|
1927
|
46500
|
34440
|
32250
|
38020
|
38370
|
33660
|
27000
|
27650
|
31860
|
38480
|
46720
|
47350
|
36858
|
1928
|
39920
|
34680
|
31430
|
35800
|
40480
|
42290
|
37830
|
33530
|
40740
|
45280
|
49460
|
51730
|
40264
|
1929
|
42270
|
35310
|
34090
|
34930
|
36640
|
32940
|
29080
|
30200
|
36110
|
41530
|
51730
|
57930
|
38563
|
1930
|
47420
|
37460
|
33870
|
40460
|
44480
|
40740
|
31160
|
27860
|
31850
|
40360
|
48210
|
47100
|
39248
|
1931
|
40220
|
35530
|
34170
|
36940
|
37550
|
34530
|
29020
|
28850
|
31750
|
36970
|
44480
|
49130
|
36595
|
1932
|
45380
|
32600
|
29860
|
34370
|
35470
|
38280
|
35610
|
30820
|
38200
|
46820
|
56200
|
56380
|
39999
|
1933
|
49740
|
46510
|
42730
|
42240
|
37350
|
32560
|
27700
|
28720
|
34020
|
41630
|
46820
|
50950
|
40081
|
1934
|
47320
|
33860
|
31090
|
34180
|
35870
|
33330
|
31160
|
32580
|
39030
|
43950
|
54800
|
63670
|
40070
|
1935
|
57310
|
43300
|
40510
|
43650
|
44760
|
42300
|
38350
|
31830
|
35580
|
40450
|
45820
|
49040
|
42742
|
1936
|
44870
|
37780
|
35270
|
36520
|
37890
|
35490
|
32900
|
32640
|
39350
|
47430
|
53580
|
56770
|
40874
|
1937
|
45880
|
37740
|
37620
|
40810
|
44140
|
41460
|
31740
|
31400
|
34770
|
40660
|
52490
|
57900
|
41384
|
1938
|
49140
|
34130
|
34410
|
34580
|
35920
|
32880
|
30100
|
32250
|
36710
|
42420
|
52350
|
56790
|
39307
|
1939
|
45860
|
39080
|
37250
|
38080
|
40880
|
37470
|
29990
|
29040
|
32020
|
42340
|
52950
|
62380
|
40612
|
1940
|
49580
|
41420
|
38640
|
40380
|
42230
|
38920
|
30210
|
32480
|
36500
|
42110
|
50240
|
52780
|
41291
|
1941
|
44440
|
34870
|
34620
|
35000
|
34180
|
35270
|
34730
|
31370
|
38020
|
41360
|
47720
|
57110
|
39058
|
1942
|
55200
|
45070
|
38440
|
37180
|
36650
|
36500
|
31190
|
33170
|
41280
|
46570
|
47860
|
46450
|
41297
|
1943
|
39330
|
32430
|
29690
|
30200
|
32200
|
32050
|
27340
|
28170
|
33840
|
41060
|
45800
|
43730
|
34653
|
1944
|
39590
|
35670
|
31670
|
39990
|
40790
|
36460
|
27090
|
28520
|
36420
|
43840
|
50270
|
52030
|
38528
|
1945
|
42080
|
32720
|
31840
|
31010
|
31360
|
34560
|
30960
|
30080
|
36830
|
46180
|
54580
|
56250
|
38204
|
1946
|
43730
|
31860
|
29340
|
30580
|
34250
|
31740
|
28120
|
29270
|
36840
|
46120
|
54850
|
61300
|
38167
|
1947
|
50350
|
39850
|
36080
|
39060
|
45990
|
44510
|
36910
|
34900
|
36730
|
44220
|
49820
|
49540
|
42330
|
1948
|
46590
|
39350
|
37990
|
36180
|
37100
|
35080
|
33560
|
34630
|
42040
|
50620
|
60170
|
62740
|
43004
|
1949
|
47360
|
34630
|
31920
|
33970
|
32800
|
29350
|
29080
|
32870
|
38140
|
46990
|
53960
|
51030
|
38508
|
1950
|
39860
|
33800
|
31250
|
37410
|
36820
|
32500
|
29480
|
32830
|
38270
|
45720
|
56940
|
56150
|
39253
|
1951
|
44720
|
36500
|
34040
|
41700
|
37730
|
34170
|
31650
|
28150
|
34240
|
39310
|
53840
|
62780
|
39903
|
1952
|
55020
|
38160
|
33000
|
33900
|
39350
|
38650
|
32250
|
31900
|
37050
|
43150
|
51020
|
51520
|
40414
|
1953
|
42748
|
34920
|
34230
|
35760
|
36900
|
33800
|
28840
|
25850
|
31910
|
37540
|
45250
|
49370
|
36427
|
1954
|
36920
|
29160
|
31780
|
36470
|
39280
|
35860
|
31830
|
27690
|
33620
|
40560
|
50180
|
51450
|
37067
|
1955
|
46760
|
41590
|
37680
|
41340
|
45350
|
39190
|
32220
|
32570
|
37440
|
47740
|
59470
|
60790
|
43512
|
1956
|
48930
|
38300
|
34460
|
38850
|
46620
|
41840
|
34750
|
30490
|
35440
|
42630
|
50390
|
55490
|
41516
|
1957
|
49770
|
37530
|
36150
|
44050
|
44230
|
40840
|
35120
|
32840
|
35380
|
39480
|
48280
|
54520
|
41516
|
1958
|
50870
|
37780
|
29820
|
29960
|
34110
|
30660
|
26130
|
26010
|
29880
|
35000
|
42100
|
42900
|
34602
|
1959
|
38510
|
34590
|
30180
|
30660
|
31260
|
29920
|
25440
|
25940
|
33870
|
42700
|
49590
|
57720
|
35865
|
1960
|
51820
|
40650
|
41510
|
45450
|
48730
|
43540
|
37560
|
35380
|
38900
|
46860
|
56680
|
59540
|
45552
|
1961
|
48220
|
41240
|
39900
|
42650
|
44510
|
37530
|
35060
|
36700
|
41410
|
52190
|
65900
|
80830
|
47178
|
1962
|
76300
|
61200
|
47280
|
51290
|
53230
|
48710
|
44760
|
40420
|
44240
|
52930
|
64320
|
74890
|
54964
|
1963
|
67950
|
55860
|
47560
|
47220
|
53230
|
51800
|
44830
|
39630
|
40690
|
44010
|
51180
|
58540
|
50208
|
1964
|
57750
|
47440
|
41830
|
43990
|
47130
|
46750
|
38790
|
32930
|
38810
|
47700
|
61390
|
69410
|
47827
|
1965
|
54640
|
40590
|
37580
|
43360
|
43370
|
35590
|
28370
|
30020
|
35980
|
41670
|
51380
|
62350
|
42075
|
1966
|
55580
|
43690
|
41880
|
46980
|
50550
|
47930
|
39300
|
36670
|
41690
|
48190
|
55550
|
63040
|
47588
|
1967
|
49130
|
35900
|
33130
|
35030
|
35220
|
33030
|
30550
|
31420
|
38050
|
45900
|
60160
|
65540
|
41088
|
1968
|
57600
|
44140
|
42800
|
44630
|
46590
|
46150
|
41570
|
36970
|
39700
|
43620
|
50420
|
58560
|
46063
|
1969
|
56690
|
48930
|
45360
|
52690
|
53070
|
50700
|
44510
|
43170
|
45980
|
50840
|
59180
|
66420
|
51462
|
1970
|
54910
|
44920
|
44300
|
45370
|
45020
|
42310
|
34480
|
36200
|
43330
|
51990
|
62820
|
62540
|
47349
|
1971
|
47930
|
38010
|
33630
|
35860
|
37540
|
33050
|
28180
|
32730
|
38690
|
44660
|
50590
|
54330
|
39600
|
1972
|
43510
|
34770
|
33130
|
35210
|
36450
|
33400
|
29580
|
29380
|
33000
|
36990
|
50140
|
55880
|
37620
|
1973
|
47060
|
37530
|
32340
|
32180
|
34650
|
32240
|
25760
|
26080
|
33170
|
40230
|
47200
|
50400
|
36570
|
1974
|
40840
|
34630
|
31530
|
34210
|
36700
|
35790
|
31530
|
33150
|
37410
|
44970
|
52150
|
52180
|
38757
|
1975
|
43490
|
37530
|
34490
|
34650
|
36450
|
32560
|
29970
|
29960
|
39110
|
49880
|
60170
|
63650
|
40993
|
1976
|
46450
|
38470
|
38300
|
40480
|
42240
|
38600
|
33940
|
32410
|
38950
|
44110
|
52040
|
59240
|
42103
|
1977
|
56190
|
46680
|
39680
|
43040
|
45650
|
38580
|
34210
|
33390
|
39260
|
48380
|
55690
|
57770
|
44877
|
1978
|
51640
|
37760
|
35800
|
37980
|
42250
|
41950
|
35860
|
33150
|
39440
|
44410
|
53640
|
62440
|
43027
|
1979
|
51920
|
45540
|
43540
|
45930
|
46870
|
47780
|
40980
|
33570
|
37600
|
39450
|
47430
|
52280
|
44408
|
1980
|
42440
|
35070
|
33340
|
35850
|
38170
|
37170
|
33780
|
34980
|
38050
|
45340
|
53790
|
59530
|
40626
|
1981
|
50000
|
39460
|
32940
|
36290
|
35540
|
38330
|
29680
|
30770
|
38900
|
44920
|
51040
|
52160
|
40003
|
1982
|
44050
|
35070
|
31400
|
29490
|
32610
|
34340
|
30680
|
29270
|
34040
|
41540
|
56690
|
61230
|
38367
|
1983
|
49020
|
36960
|
31360
|
31610
|
29980
|
27060
|
24360
|
25620
|
31250
|
36080
|
45260
|
47720
|
34690
|
APPENDIX 4: ATTRIBUTE TABLE FOR DRAINAGE NETWORK
ORDERING
Domain
|
The IDs of the Table's domain, every record (ID) represents a
certain stream.
|
UpstreamLinkID
|
The IDs of the streams that directly contribute to the current
stream, e.g. when streams 5 and 7 flow together into stream 12, then the
UpstreamLinkID column will read for the record with ID 12: {5,7}
|
UpstreamCoord
|
The XY-coordinate of the beginning of a stream segment
(down-flow); these coordinates are shown as points in the third picture above.
This column is a coordinate column.
|
UpstreamElevation
|
The elevation, as extracted from the DEM, at the position of the
upstream coordinate. This column is a value column.
|
DownstreamLinkID
|
The ID of the stream segment into which the current stream will
flow (down-flow). This column is a value column.
|
DownstreamCoord
|
The XY-coordinate of the end of a stream segment (down-flow).
|
DownstreamElevation
|
A column containing the elevation, as extracted from the DEM, at
the position of the downstream coordinate.
|
ElevationDifference
|
Height difference between upstream coordinate and downstream
coordinate, i.e. UpstreamElevation - DownstreamElevation.
|
Strahler
|
Strahler ordering number; the Strahler ordering number for a
stream is calculated as follows: when streams of equal order join each other:
the Strahler ordering number of one of the streams that directly contributes to
this stream + 1 is assigned;
when streams of unequal order join each other: the largest
Strahler ordering number of the streams that directly contribute to this stream
is assigned;
In other words: the Strahler order values of the UpstreamLinkIDs
are found, when these order values are equal, the value is increased by 1;
when these order values are unequal, the maximum value is
assigned. The Strahler column is a value column.
|
Shreve
|
Shreve ordering number; the Shreve ordering number for a stream
is calculated as the sum of the Shreve ordering numbers of the streams that
directly contribute to this stream, i.e. the sum of the Shreve order values of
the UpstreamLinkIDs. The Shreve column is a value column.
|
Length
|
The length of a stream measured along the drainage.
|
StraightLength
|
The length of a stream calculated as the difference between the
upstream coordinate and the downstream coordinate, i.e. | UpstreamCoord -
DownstreamCoord |
|
SlopeAlongDrainage%
|
The average slope in percentages between the upstream coordinate
and the downstream coordinate, when the length is measured along the drainage,
i.e. (ElevationDifference/Length)* 100%
|
SlopeAlongDrainageDegree
|
The average slope angle in degrees between the upstream
coordinate and the downstream coordinate, when the length is measured along the
drainage, i.e. tan- 1(ElevationDifference/Length)
|
SlopeDrainageStraight%
|
The average slope in percentages between the upstream coordinate
and the downstream coordinate, when the length is the straight length between
the upstream coordinate and the downstream coordinate, i.e.
(ElevationDifference/StraightLength)* 100%
|
SlopeDrainageStraightDegree
|
The average slope angle in degrees between the upstream
coordinate and the downstream coordinate, when the length is the straight
length between the upstream coordinate and the downstream coordinate, i.e.
tan1(ElevationDifference/StraightLength)
|
Sinuosity
|
A measure for meandering of the stream, i.e. Length /
StraightLength
|
TotalUpstreamAlongDrainageLength
|
The total length of all (upstream) streams that contribute to the
current stream
|
StrahlerClass
|
An additional class column to be able to display the Strahler
ordering numbers as classes.
|
APPENDIX 5: HORTON STATISTICS FUNCTIONALITY: DEFINITION
OF PARAMETERS
The Horton Statistics operation calculates for all streams with a
certain (Strahler) stream order number i, located in merged catchment C with ID
x:
Cx_N: the number of streams for catchment x, or The number of
streams with a certain Strahler stream order number
Cx_L: the average stream length (km), or The average
length (km) of the streams with this Strahler order number.
Cx_A: the average area of catchments (km2) or
The average area (km2) of the catchments belonging to the streams
with this Strahler order number.
Cx_N_LSq: expected values for Cx_N by means
of a least squares fit through Cx_N, or The expected number of streams
with a certain Strahler stream order number.
Cx_L_LSq: expected values for Cx_L by means
of a least squares fit through Cx_L, or The expected average length
(km) of the streams with this Strahler order number.
Cx_A_LSq: expected values for Cx_A by means
of a least squares fit through Cx_A, or The expected average area
(km2) of the catchments belonging to the streams with this Strahler
order number.
APPENDIX 6: HORTON MORPHOLOGICAL PARAMETERS AND
STATISTICS FOR SUBSEQUENT STRAHLER ORDER
|
strahler Order
|
HORTON MORPHOLOGICAL PARAMETERS
|
Calculated
|
Expected
|
C_N
|
C_L
|
C_A
|
C_N_LSq
|
C1_L_LSq
|
C_A_LSq
|
1
|
1
|
30
|
64.74
|
3322.57
|
25.751
|
69.607
|
4205.716
|
2
|
6
|
196.02
|
21035.86
|
8.143
|
169.59
|
14619.42
|
3
|
3
|
384.32
|
49761.68
|
2.575
|
413.19
|
50818.28
|
4
|
1
|
662.01
|
158672.2
|
0.814
|
1006.7
|
176648.5
|
5
|
|
|
|
|
|
1
|
6
|
|
|
|
|
|
1
|
7
|
|
|
|
|
|
1
|
2
|
1
|
26
|
64.76
|
3258.19
|
30.213
|
76.663
|
2964.718
|
2
|
8
|
183.8
|
14855.7
|
5.925
|
131.139
|
17944.58
|
3
|
1
|
189.49
|
119366.6
|
1.162
|
224.328
|
108613.4
|
4
|
1
|
664.74
|
132257.5
|
0.228
|
383.737
|
657405.5
|
5
|
|
|
|
|
|
|
6
|
|
|
|
|
|
|
7
|
|
|
|
|
|
|
3
|
1
|
118
|
65.19
|
3137.12
|
133.107
|
80.73
|
3510.212
|
2
|
32
|
191.43
|
15777.09
|
33.277
|
178.149
|
15224.16
|
3
|
9
|
340.46
|
61493.83
|
8.32
|
393.127
|
66028.79
|
4
|
2
|
932.09
|
296725.4
|
2.08
|
867.528
|
286373.8
|
5
|
1
|
2130.4
|
646792.4
|
0.52
|
1914.403
|
1242034
|
6
|
|
|
|
|
|
|
7
|
|
|
|
|
|
|
4
|
1
|
168
|
77.44
|
2986.6
|
107.066
|
160.972
|
5432.712
|
2
|
42
|
234.59
|
16796.23
|
39.039
|
263.615
|
17644.8
|
3
|
13
|
476.94
|
63232.86
|
14.235
|
431.708
|
57308.19
|
4
|
5
|
821.84
|
177175
|
5.19
|
706.985
|
186130.1
|
5
|
2
|
1013.09
|
450879.8
|
1.893
|
1157.791
|
604528.3
|
6
|
1
|
1152.81
|
905331.4
|
0.69
|
1896.051
|
1963436
|
7
|
|
|
|
|
|
|
5
|
1
|
261
|
57.51
|
2591.04
|
236.501
|
75.237
|
3126.151
|
2
|
54
|
185.49
|
16541.88
|
55.742
|
173.055
|
14315.61
|
3
|
14
|
346.48
|
70292.39
|
13.138
|
398.046
|
65555.63
|
4
|
3
|
981.2
|
358082.7
|
3.097
|
915.553
|
300199.5
|
5
|
1
|
2265.76
|
1121600
|
0.73
|
2105.879
|
1374707
|
6
|
|
|
|
0.172
|
4843.768
|
6295207
|
7
|
|
|
|
|
|
1
|
6
|
1
|
151
|
63.27
|
3080.17
|
25.159
|
195.664
|
27871.48
|
2
|
26
|
297.12
|
27360.17
|
12.592
|
312.666
|
57809.6
|
3
|
6
|
589.78
|
129887.4
|
6.302
|
499.632
|
119905.7
|
4
|
1
|
605.16
|
779475.3
|
3.154
|
798.399
|
248702.4
|
5
|
1
|
1655.82
|
823385.2
|
1.578
|
1275.822
|
515845.9
|
6
|
2
|
1847.5
|
417141.4
|
0.79
|
2038.731
|
1069941
|
7
|
1
|
2445.2
|
859575.2
|
0.395
|
3257.842
|
2219218
|
APPENDIX 7 PEARSON PRODUCT MOMENT CORRELATION
BETWEEN HORTON AND GIUH
Friday, June 27, 2008, 3:52:27 AM Data source:
Data 1 in Notebook 1
Pearson Product Moment Correlation Friday, June 27, 2008,
3:52:27 AM
Data source: Data 1 in Notebook 1
Cell Contents:
Correlation Coefficient
P Value Number of
Samples
|
Rb
|
Rl
|
Ra
|
LHSOr
|
Tpg
|
Qpg
|
Tpg
|
|
|
|
|
|
|
|
|
Rb
|
|
0.903
|
0.952
|
-0.284
|
-0.464
|
0.504
|
-0.464
|
|
|
0.0137
|
0.00345
|
0.585
|
0.354
|
0.308
|
0.354
|
|
|
6
|
6
|
6
|
6
|
6
|
6
|
|
|
|
|
|
|
|
|
Rl
|
|
|
0.958
|
-0.287
|
-0.494
|
0.532
|
-0.494
|
|
|
|
0.00267
|
0.582
|
0.32
|
0.277
|
0.32
|
|
|
|
6
|
6
|
6
|
6
|
6
|
|
|
|
|
|
|
|
|
Ra
|
|
|
|
-0.386
|
-0.586
|
0.598
|
-0.586
|
|
|
|
|
0.45
|
0.222
|
0.209
|
0.222
|
|
|
|
|
6
|
6
|
6
|
6
|
|
|
|
|
|
|
|
|
LHSOr
|
|
|
|
|
0.965
|
-0.96
|
0.965
|
|
|
|
|
|
0.00182
|
0.00243
|
0.00182
|
|
|
|
|
|
6
|
6
|
6
|
|
|
|
|
|
|
|
|
Tpg
|
|
|
|
|
|
-0.975
|
1
|
|
|
|
|
|
|
0.000899
|
0
|
|
|
|
|
|
|
6
|
6
|
|
|
|
|
|
|
|
|
Qpg
|
|
|
|
|
|
|
-0.975
|
|
|
|
|
|
|
|
0.000899
|
|
|
|
|
|
|
|
6
|
APPENDIX 8.a. LOCAL WATER BALANCE FOR SELECTED GRID CELLS
IN THE CONGO RIVER BASIN (TABLE)
PPT = Precipitation, PEH= Effective Precipitation, PET= Potential
Evapotranspiration, AET = Actual Evapotranspiration, SOM= Soil Moisture, ROF=
Runoff, C= Vertical Integrated Moisture Convergence
Long Lat
|
Item
|
Dec Jan Feb Mar Apr May Jun Jul Aug Sep Oct Nov DJF MAM
JJA SON
|
Ann.
|
18.31
|
4.24
|
AET
|
32.2
|
18.8
|
34.6
|
89.3
|
106.5
|
121.2
|
102.3
|
102.5
|
102.5
|
105.2
|
105.6
|
91.3
|
85.5
|
317.1
|
307.2
|
302.1
|
1011.9
|
18.31
|
4.24
|
PET
|
114.7
|
117.9
|
117.8
|
136.4
|
128.8
|
121.0
|
102.2
|
102.4
|
102.4
|
105.2
|
105.8
|
105.2
|
350.5
|
386.2
|
307.0
|
316.2
|
1359.8
|
18.31
|
4.24
|
PPT
|
31.3
|
20.4
|
37.5
|
108.9
|
137.1
|
170.8
|
140.0
|
178.4
|
227.3
|
192.8
|
195.6
|
92.6
|
89.3
|
416.8
|
545.7
|
480.9
|
1532.7
|
18.31
|
4.24
|
ROF
|
1.2
|
0.4
|
0.1
|
0.0
|
0.0
|
0.0
|
0.0
|
11.8
|
33.2
|
29.3
|
13.7
|
4.1
|
1.7
|
0.0
|
45.0
|
47.1
|
93.9
|
18.31
|
4.24
|
SOM
|
0.0
|
0.0
|
0.0
|
0.0
|
0.0
|
2.9
|
9.3
|
17.6
|
17.6
|
17.6
|
17.6
|
3.9
|
0.0
|
2.9
|
44.5
|
39.1
|
86.6
|
18.31
|
4.24
|
C
|
-0.1
|
0.0
|
0.0
|
0.0
|
0.0
|
0.1
|
0.2
|
0.7
|
1.1
|
1.0
|
0.4
|
-0.3
|
-0.1
|
0.1
|
1.9
|
1.1
|
3.1
|
21.04
|
-0.04
|
AET
|
105.8
|
108.7
|
108.3
|
122.3
|
112.5
|
110.2
|
100.1
|
96.2
|
105.1
|
107.8
|
110.1
|
103.5
|
322.8
|
345.0
|
301.3
|
321.4
|
1290.5
|
21.04
|
-0.04
|
PET
|
105.8
|
113.8
|
113.6
|
122.3
|
112.5
|
110.2
|
100.1
|
96.2
|
105.1
|
107.8
|
110.1
|
103.5
|
333.2
|
345.0
|
301.3
|
321.4
|
1300.9
|
21.04
|
-0.04
|
PPT
|
165.0
|
123.3
|
135.7
|
181.5
|
159.0
|
189.1
|
143.1
|
146.5
|
184.7
|
192.2
|
233.7
|
210.9
|
424.0
|
529.6
|
474.3
|
636.8
|
2064.7
|
21.04
|
-0.04
|
ROF
|
7.4
|
2.2
|
0.7
|
0.2
|
0.1
|
0.0
|
0.0
|
0.0
|
0.0
|
8.0
|
23.4
|
24.5
|
10.2
|
0.3
|
0.0
|
55.9
|
66.4
|
21.04
|
-0.04
|
SOM
|
17.6
|
7.4
|
4.7
|
10.9
|
16.6
|
17.6
|
17.6
|
17.6
|
17.6
|
17.6
|
17.6
|
17.6
|
29.7
|
45.1
|
52.8
|
52.8
|
180.4
|
21.04
|
-0.04
|
C
|
0.1
|
-0.3
|
-0.1
|
0.2
|
0.2
|
0.5
|
0.4
|
0.5
|
0.7
|
0.8
|
1.0
|
1.2
|
-0.2
|
0.9
|
1.6
|
3.0
|
5.2
|
28.52
|
-2.31
|
PET
|
115.2
|
122.4
|
118.4
|
136.3
|
123.4
|
119.8
|
103.5
|
100.9
|
103.5
|
109.8
|
111.6
|
107.2
|
356.0
|
379.5
|
307.9
|
328.5
|
1371.9
|
28.52
|
-2.31
|
PPT
|
165.4
|
136.1
|
133.8
|
181.2
|
170.2
|
107.1
|
32.4
|
16.6
|
57.3
|
105.9
|
134.9
|
175.5
|
435.3
|
458.4
|
106.3
|
416.3
|
1416.4
|
28.52
|
-2.31
|
ROF
|
1.2
|
0.4
|
0.1
|
0.0
|
0.0
|
0.0
|
1.8
|
14.9
|
29.2
|
21.7
|
13.0
|
3.9
|
1.6
|
0.0
|
45.9
|
38.6
|
86.2
|
28.52
|
-2.31
|
SOM
|
1.2
|
0.0
|
0.0
|
0.0
|
0.0
|
11.3
|
28.2
|
28.2
|
28.2
|
28.2
|
28.2
|
17.4
|
1.2
|
11.3
|
84.5
|
73.7
|
170.6
|
28.52
|
-2.3 1
|
C
|
0.9
|
0.5
|
0.6
|
0.9
|
0.9
|
0.0
|
-0.4
|
0.0
|
0.0
|
0.0
|
0.3
|
1.0
|
2.0
|
1.8
|
-0.4
|
1.2
|
4.6
|
28.52
|
-2.30
|
AET
|
95.2
|
100.5
|
93.6
|
102.9
|
92.9
|
91.0
|
41.3
|
16.7
|
51.4
|
86.6
|
99.7
|
97.5
|
289.2
|
286.9
|
109.4
|
283.8
|
969.4
|
22.25
|
-5.56
|
AET
|
112.0
|
114.6
|
109.7
|
122.9
|
110.8
|
87.7
|
12.3
|
7.6
|
19.8
|
65.2
|
122.1
|
106.5
|
336.3
|
321.4
|
39.8
|
293.9
|
991.4
|
22.25
|
-5.56
|
PET
|
108.7
|
111.7
|
103.6
|
114.9
|
108.2
|
118.1
|
114.5
|
112.2
|
112.1
|
114.4
|
124.4
|
111.3
|
324.1
|
341.2
|
338.8
|
350.1
|
1354.2
|
22.25
|
-5.56
|
PPT
|
232.2
|
137.5
|
125.6
|
204.4
|
177.5
|
76.5
|
17.6
|
15.6
|
49.6
|
126.5
|
171.6
|
235.3
|
495.3
|
458.3
|
82.7
|
533.4
|
1569.7
|
22.25
|
-5.56
|
ROF
|
0.0
|
0.0
|
0.0
|
0.0
|
1.4
|
0.4
|
0.1
|
0.0
|
0.0
|
0.0
|
0.0
|
0.0
|
0.0
|
1.8
|
0.2
|
0.0
|
2.0
|
22.25
|
-5.56
|
SOM
|
28.2
|
23.6
|
20.9
|
28.2
|
28.2
|
4.7
|
0.1
|
0.0
|
0.0
|
0.0
|
0.4
|
28.2
|
72.7
|
61.0
|
0.2
|
28.6
|
162.4
|
22.25
|
-5.56
|
C
|
0.9
|
0.1
|
0.0
|
0.6
|
0.1
|
-0.7
|
-0.1
|
0.0
|
0.0
|
0.0
|
0.0
|
1.1
|
1.0
|
0.0
|
-0.1
|
1.1
|
2.0
|
15.22
|
-4.22
|
AET
|
105.5
|
109.8
|
107.2
|
122.2
|
114.1
|
105.4
|
25.8
|
2.8
|
3.6
|
35.9
|
98.4
|
107.8
|
322.6
|
341.6
|
32.1
|
242.1
|
938.4
|
15.22
|
-4.22
|
PET
|
105.5
|
109.8
|
109.4
|
122.2
|
114.1
|
106.0
|
90.5
|
93.6
|
106.7
|
113.8
|
116.0
|
107.8
|
324.8
|
342.2
|
290.9
|
337.7
|
1295.6
|
15.22
|
-4.22
|
PPT
|
162.8
|
142.4
|
121.1
|
180.9
|
213.7
|
125.1
|
4.5
|
1.8
|
3.5
|
38.6
|
123.6
|
246.2
|
426.2
|
519.8
|
9.7
|
408.4
|
1364.2
|
15.22
|
-4.22
|
ROF
|
13.1
|
4.1
|
1.2
|
0.4
|
8.4
|
2.5
|
0.8
|
0.2
|
0.1
|
0.0
|
0.0
|
8.5
|
18.4
|
11.3
|
1.0
|
8.5
|
39.2
|
15.22
|
-4.22
|
SOM
|
27.7
|
27.7
|
18.1
|
24.2
|
27.7
|
22.2
|
1.0
|
0.0
|
0.0
|
0.0
|
0.0
|
27.7
|
73.6
|
74.1
|
1.0
|
27.7
|
176.5
|
15.22
|
-4.22
|
C
|
0.4
|
0.1
|
-0.3
|
0.2
|
0.4
|
-0.1
|
-0.7
|
0.0
|
0.0
|
0.0
|
0.0
|
1.2
|
0.3
|
0.5
|
-0.7
|
1.2
|
1.3
|
25.11
|
0.31
|
AET
|
101.2
|
82.1
|
88.8
|
119.3
|
112.3
|
108.6
|
94.5
|
79.5
|
98.5
|
102.1
|
108.9
|
101.9
|
272.1
|
340.2
|
272.5
|
312.9
|
1197.7
|
25.11
|
0.31
|
PET
|
108.4
|
114.6
|
114.7
|
123.8
|
116.8
|
111.6
|
96.2
|
96.0
|
99.1
|
108.0
|
114.5
|
107.9
|
337.8
|
352.1
|
291.3
|
330.3
|
1311.5
|
25.11
|
0.31
|
PPT
|
101.7
|
71.5
|
96.2
|
164.0
|
179.1
|
161.7
|
109.2
|
119.5
|
156.0
|
184.9
|
214.1
|
196.3
|
269.4
|
504.8
|
384.7
|
595.4
|
1754.2
|
25.11
|
0.31
|
ROF
|
7.8
|
2.3
|
0.7
|
0.2
|
0.6
|
1.5
|
0.4
|
3.3
|
11.6
|
16.8
|
24.6
|
25.9
|
10.8
|
2.3
|
15.3
|
67.3
|
95.7
|
25.11
|
0.31
|
SOM
|
4.7
|
0.3
|
0.0
|
0.0
|
11.2
|
17.0
|
11.8
|
12.3
|
17.6
|
17.6
|
17.6
|
17.6
|
5.0
|
28.3
|
41.7
|
52.8
|
127.8
|
25.11
|
0.31
|
C
|
-0.2
|
-0.1
|
0.0
|
0.0
|
0.4
|
0.2
|
-0.2
|
0.0
|
0.5
|
0.6
|
0.8
|
0.9
|
-0.2
|
0.6
|
0.3
|
2.2
|
2.9
|
27.29
|
-11.40
|
AET
|
109.6
|
112.4
|
104.4
|
106.2
|
99.1
|
17.0
|
0.2
|
0.0
|
1.3
|
20.1
|
78.2
|
112.1
|
326.3
|
222.4
|
1.5
|
210.4
|
760.5
|
27.29
|
-11.40
|
PET
|
113.3
|
111.6
|
98.0
|
117.1
|
118.7
|
119.7
|
108.5
|
118.4
|
142.4
|
163.4
|
175.5
|
135.3
|
322.9
|
355.4
|
369.3
|
474.2
|
1521.9
|
27.29
|
-11.40
|
PPT
|
259.2
|
247.5
|
267.6
|
206.0
|
55.9
|
2.6
|
1.0
|
1.6
|
0.0
|
2.1
|
33.6
|
164.2
|
774.3
|
264.6
|
2.6
|
200.0
|
1241.5
|
27.29
|
-11.40
|
ROF
|
12.7
|
23.8
|
24.3
|
12.0
|
3.6
|
1.1
|
0.3
|
0.1
|
0.0
|
0.0
|
0.0
|
0.0
|
60.7
|
16.7
|
0.5
|
0.0
|
77.9
|
27.29
|
-11.40
|
SOM
|
28.2
|
28.2
|
28.2
|
28.2
|
2.5
|
0.0
|
0.0
|
0.0
|
0.0
|
0.0
|
0.0
|
0.0
|
84.5
|
30.7
|
0.0
|
0.0
|
115.2
|
27.29
|
-11.40
|
C
|
1.1
|
0.9
|
1.6
|
0.2
|
-0.8
|
-0.1
|
0.0
|
0.0
|
0.0
|
0.0
|
0.0
|
0.0
|
3.6
|
-0.7
|
0.0
|
0.0
|
2.9
|
16.03
|
1.37
|
AET
|
81.0
|
55.8
|
81.2
|
111.7
|
105.0
|
102.3
|
87.1
|
86.6
|
93.0
|
99.0
|
105.4
|
99.0
|
218.0
|
319.0
|
266.7
|
303.4
|
1107.1
|
16.03
|
1.37
|
PET
|
105.4
|
99.3
|
98.1
|
111.7
|
105.0
|
102.3
|
87.1
|
89.9
|
93.0
|
99.0
|
105.4
|
99.0
|
302.7
|
319.0
|
270.0
|
303.4
|
1195.2
|
16.03
|
1.37
|
PPT
|
74.2
|
60.3
|
96.1
|
151.0
|
134.3
|
179.0
|
143.7
|
91.7
|
144.0
|
223.0
|
237.1
|
172.1
|
230.6
|
464.3
|
379.3
|
632.1
|
1706.3
|
16.03
|
1.37
|
ROF
|
9.0
|
2.7
|
0.8
|
0.2
|
0.1
|
8.1
|
10.5
|
3.1
|
7.4
|
33.0
|
39.0
|
29.9
|
12.5
|
8.4
|
20.9
|
102.0
|
143.8
|
16.03
|
1.37
|
SOM
|
1.8
|
0.1
|
0.1
|
3.4
|
3.5
|
17.6
|
17.6
|
8.8
|
17.6
|
17.6
|
17.6
|
17.6
|
2.0
|
24.5
|
44.1
|
52.9
|
123.5
|
16.03
|
1.37
|
C
|
-0.2
|
0.0
|
0.0
|
0.1
|
0.0
|
0.7
|
0.3
|
-0.2
|
0.5
|
1.1
|
1.3
|
1.0
|
-0.2
|
0.8
|
0.7
|
3.4
|
4.7
|
20.04
|
-11.04
|
AET
|
114.2
|
115.8
|
107.6
|
109.9
|
101.8
|
18.3
|
0.0
|
-0.1
|
2.6
|
23.5
|
78.7
|
117.2
|
337.6
|
229.9
|
2.5
|
219.4
|
789.4
|
20.04
|
-11.04
|
PET
|
114.2
|
115.8
|
107.6
|
109.9
|
110.9
|
122.0
|
113.3
|
125.5
|
144.0
|
148.1
|
140.2
|
117.2
|
337.6
|
342.8
|
382.8
|
405.5
|
1468.6
|
20.04
|
-11.04
|
PPT
|
214.3
|
212.3
|
182.1
|
205.0
|
101.1
|
7.2
|
-0.2
|
-0.2
|
2.6
|
24.9
|
92.1
|
172.3
|
608.6
|
313.3
|
2.2
|
289.3
|
1213.4
|
20.04
|
-11.04
|
ROF
|
4.1
|
18.5
|
20.3
|
9.4
|
2.8
|
0.8
|
0.3
|
0.1
|
0.0
|
0.0
|
0.0
|
0.0
|
42.9
|
13.1
|
0.4
|
0.0
|
56.3
|
20.04
|
-11.04
|
SOM
|
28.2
|
28.2
|
28.2
|
11.1
|
0.2
|
0.0
|
0.0
|
0.0
|
0.0
|
0.0
|
7.3
|
21.2
|
84.5
|
11.3
|
0.0
|
28.5
|
124.3
|
20.04
|
-11.04
|
C
|
0.8
|
0.6
|
0.7
|
0.3
|
-0.5
|
-0.3
|
0.0
|
0.0
|
0.0
|
0.0
|
0.0
|
0.2
|
2.1
|
-0.5
|
0.0
|
0.2
|
1.9
|
23.05
|
8.00
|
AET
|
4.5
|
0.8
|
7.0
|
51.5
|
88.7
|
123.5
|
113.4
|
103.4
|
108.0
|
109.7
|
116.9
|
43.6
|
12.3
|
263.7
|
324.8
|
270.2
|
871.0
|
23.05
|
8.00
|
PET
|
114.2
|
114.6
|
99.7
|
112.7
|
110.1
|
123.6
|
128.4
|
133.2
|
136.1
|
131.7
|
136.6
|
121.3
|
328.5
|
346.4
|
397.7
|
389.6
|
1462.1
|
23.05
|
8.00
|
PPT
|
258.2
|
195.3
|
188.0
|
232.0
|
181.6
|
48.5
|
3.5
|
0.5
|
18.8
|
81.6
|
161.4
|
242.9
|
641.5
|
462.0
|
22.7
|
486.0
|
1612.2
|
23.05
|
8.00
|
ROF
|
8.8
|
8.2
|
12.0
|
8.5
|
2.5
|
0.8
|
0.2
|
0.1
|
0.0
|
0.0
|
0.0
|
0.0
|
29.1
|
11.7
|
0.3
|
0.0
|
41.2
|
23.05
|
8.00
|
SOM
|
28.2
|
28.2
|
28.2
|
28.2
|
28.2
|
1.6
|
0.0
|
0.0
|
0.0
|
0.0
|
0.0
|
26.6
|
84.5
|
58.0
|
0.0
|
26.6
|
169.0
|
23.05
|
8.00
|
C
|
0.8
|
0.7
|
1.0
|
1.0
|
0.0
|
-0.8
|
-0.1
|
0.0
|
0.0
|
0.0
|
0.0
|
0.9
|
2.5
|
0.2
|
-0.1
|
0.9
|
3.6
|
32.03
|
-5.05
|
AET
|
113.1
|
114.6
|
107.9
|
125.2
|
119.0
|
79.7
|
17.7
|
8.3
|
59.4
|
95.5
|
124.4
|
115.3
|
335.7
|
324.0
|
85.3
|
335.3
|
1080.3
|
32.03
|
-5.05
|
PET
|
116.9
|
116.0
|
110.8
|
123.0
|
118.8
|
126.5
|
129.4
|
143.0
|
158.8
|
158.8
|
159.8
|
122.9
|
343.7
|
368.4
|
431.2
|
441.5
|
1584.8
|
32.03
|
-5.05
|
PPT
|
164.1
|
127.6
|
121.8
|
152.5
|
168.9
|
55.1
|
4.2
|
0.0
|
-0.1
|
12.5
|
46.0
|
130.7
|
413.6
|
376.4
|
4.2
|
189.2
|
983.3
|
32.03
|
-5.05
|
ROF
|
25.3
|
10.0
|
5.0
|
15.6
|
3.5
|
1.0
|
0.3
|
0.1
|
0.0
|
0.0
|
0.0
|
6.5
|
40.3
|
20.2
|
0.4
|
6.5
|
67.4
|
32.03
|
-5.05
|
SOM
|
-5.1
|
5.2
|
2.1
|
0.9
|
0.6
|
12.2
|
0.1
|
0.0
|
0.0
|
0.0
|
0.0
|
0.0
|
2.3
|
13.6
|
0.1
|
0.0
|
16.0
|
32.03
|
-5.05
|
C
|
0.1
|
0.0
|
0.0
|
0.0
|
0.1
|
0.0
|
0.0
|
0.0
|
0.0
|
0.0
|
0.0
|
0.0
|
0.1
|
0.1
|
0.0
|
0.0
|
0.2
|
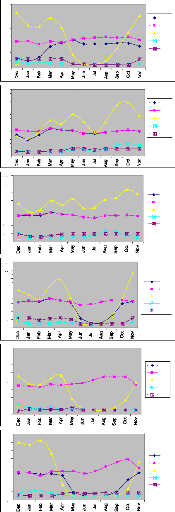
300
260
220
300
260
220
300
260
220
300
260
220
300
260
220
300
260
220
180
140
100
180
140
100
180
140
100
180
140
100
180
140
100
180
140
100
-20
-20
-20
-20
-20
-20
60
20
60
20
60
20
60
20
60
20
60
20
LUBUMBASHI(-14, 27)
UP-NORTH
BOYENDE
URAMBO
KINSHASA
OUESSO
AET PET PPT ROF SOM
AET PET PPT ROF SOM
AET PET PPT ROF SOM
AET PET PPT ROF SOM
AET PET PPT ROF SOM
AET PET PPT ROF SOM
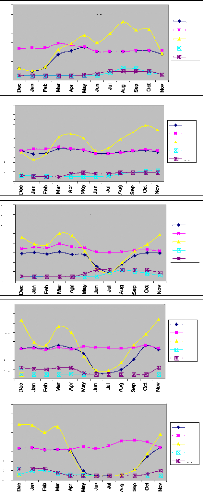
300
260
220
300
260
220
300
260
220
300
260
220
180
140
100
180
140
100
300
260
220
180
140
100
180
140
100
180
140
100
-20
-20
-20
-20
20
60
20
60
20
60
20
60
20
60
20
BUKAVU (28.52, -2.30)
SOUTH-WEST
KANANGA
BANGUI
KISANGANI
AET PET PPT ROF SOM
AET PET PPT ROF SOM
AET PET PPT ROF SOM
AET PET PPT ROF SOM
AET PET PPT ROF SOM
APPENDIX 8.b. LOCAL WATER BALANCE FOR SELECTED GRID CELLS
IN THE CONGO RIVER BASIN (GRAPHS)
APPENDIX 9: SEASONAL DISTRIBUTION OF THE CONVERGENCE
MOISTURE SAMPLES FOR SELECTED GRID CELLES OVER THE CONGO BASIN (C)
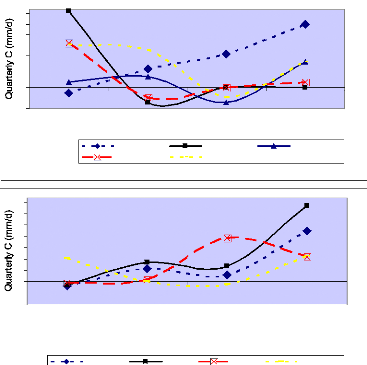
4
3
3
2
2
1
1
0
-1
-1
DJF MAM JJA SON
BOYENDE LUBUMBASHI KINSHASA
SOUTH-WEST BUKAVU
4
3
3
2
2
1
1
0
-1
-1
DJF MAM JJA SON
KISANGANI OUESSO BANGUI UP-NORTH
The first graph shows stations in the southern hemisphere. The
second corresponds to the northern hemisphere.
|