In
order to arrive at good conclusions and propose important policies, data to be
used must be stationary or made stationary .Non-stationary variables can lead
to misleading inferences. So, the following is the analysis of stationarity by
using the PP and ADF tests.
Table 5: Summary of Unity
root Test using PP and ADF tests
Variables
|
PP test
|
ADF test
|
Conclusion
|
|
Level
|
Intercept & Trend
|
Intercept
|
None
|
Intercept & Trend
|
Intercept
|
None
|
I(1)
|
LCPI
|
Level
|
-1.509203
|
-0.037775
|
1.304367
|
-1.593095
|
-0.166940
|
1.201365
|
?level
|
-5.671982*
|
-5.278210*
|
-5.107284*
|
-5.541824*
|
-5.277734*
|
-5.107284*
|
LM2
|
Level
|
-1.808800
|
-0.840839
|
-0.919552
|
-1.808800
|
-0.840839
|
-0.912034
|
I(1)
|
?level
|
-5.215006*
|
-5.172020*
|
-5.156853*
|
-5.206502*
|
-5.171833*
|
-5.156853*
|
LEXCH
|
Level
|
-1.465468
|
-0.700263
|
2.044835
|
-1.295550
|
-0.700263
|
2.189489
|
I(1)
|
? level
|
-4.560735*
|
-4.493495*
|
-3.732926*
|
-3.798612**
|
-4.506769*
|
-3.755935*
|
LLIR
|
Level
|
-2.598714
|
-2.192332
|
0.665482
|
-2.238489
|
-2.087310
|
0.279109
|
I(1)
|
? level
|
-6.873350*
|
-6.320553*
|
-6.143093*
|
-4.021954**
|
-2.074770
|
-1.859478***
|
Source: Eviews7
* Indicates statistical significant at the 1 percent level,
** Indicates statistical significant at the
5 percent level
*** Indicates statistical significant at the
10 percent level.
From the table above, it can be deduced that variables are not
stationary at level. We did not found statistical evidence of rejecting the
Null hypothesis of unit root because the asymptotic critical values are less
than the calculated value for ADF and the p values are more than 5%. However,
when all the variables are transformed to their first difference, the
null hypothesis is rejected and variables became stationary. Finally, we
concluded that all variables are integrated of order one.
4.2.2.2 Co-integration test
If two or more time series are not stationary, it is important
to test whether there is a linear combination of them which is stationary. This
phenomenon is referred to as the test for co-integration. The evidence of
co-integration implies that there is a long run relationship among the
variables. Hence, the short-run dynamics can be represented by an error
correction mechanism (Engle and Granger, 1987).
There are two most popular approaches for testing for
cointegration, the Engle- Granger two steps procedure and the Johansen
procedure. In this research, we applied the Johansen Maximum Likelihood
Methodology for the cointegration test. The obtained results are in the
following table:
Table 6: Results of Johansen
Cointegration Test
Unrestricted Cointegration Rank Test (Trace)
|
|
|
|
|
|
|
|
|
|
|
|
Hypothesized
|
|
Trace
|
0.05
|
|
No. of CE(s)
|
Eigenvalue
|
Statistic
|
Critical Value
|
Prob.**
|
|
|
|
|
|
|
|
|
|
|
None
|
0.650795
|
45.29174
|
54.07904
|
0.2391
|
At most 1
|
0.340354
|
20.04146
|
35.19275
|
0.7242
|
At most 2
|
0.221537
|
10.05623
|
20.26184
|
0.6343
|
At most 3
|
0.155133
|
4.045828
|
9.164546
|
0.4053
|
|
|
|
|
|
|
|
|
|
|
Trace test indicates no cointegration at the 0.05 level
|
* denotes rejection of the hypothesis at the 0.05 level
|
**MacKinnon-Haug-Michelis (1999) p-values
|
|
Unrestricted Cointegration Rank Test (Maximum Eigenvalue)
|
|
|
|
|
|
|
|
|
|
|
Hypothesized
|
|
Max-Eigen
|
0.05
|
|
No. of CE(s)
|
Eigenvalue
|
Statistic
|
Critical Value
|
Prob.**
|
|
|
|
|
|
|
|
|
|
|
None
|
0.650795
|
25.25028
|
28.58808
|
0.1260
|
At most 1
|
0.340354
|
9.985229
|
22.29962
|
0.8363
|
At most 2
|
0.221537
|
6.010404
|
15.89210
|
0.7868
|
At most 3
|
0.155133
|
4.045828
|
9.164546
|
0.4053
|
|
|
|
|
|
|
|
|
|
|
Source: Eviews7
Max-eigenvalue test indicates no cointegration at the 0.05
level
|
* denotes rejection of the hypothesis at the 0.05 level
|
**MacKinnon-Haug-Michelis (1999) p-values
|
|
The Trace test as well as the Maximum Eigenvalue test reveals
that variables are not co-integrated. Then, these variables are not co
integrated to run a regression line by using OLS. Such a regression can lead to
misleading inferences. So, we have to use other methods such as unrestricted
VAR.
4.2.2.2.1
Estimation of an impact of monetary policy on CPI of Rwanda
The coefficients of our model are numerous and not readily
subject to interpretation. Hence, the interpretation follows from the path of
the impulse response functions generated from the recursively-orthogonalized
VAR estimated residuals. The impulse responses show the path of CPI when there
is an increase in the monetary policy variables.
Figure 8: Response of CPI
to Monetary Policy Variables
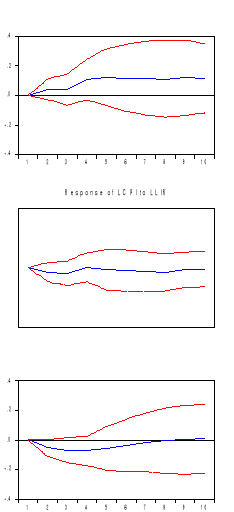
The figure 8 shows three panels of impulse response graphs
indicating how increase in respective monetary policy variables affects the CPI
of Rwanda in a period of five years. Each panel illustrates the response of CPI
to a one standard deviation innovation (corresponding to an increase) in the
monetary policy variable.
A value of zero means that the increase in monetary policy
variable has no effect on CPI of Rwanda and the CPI continues to behave as if
there was no increase in monetary policy variable. A positive or negative value
indicates that the increase in the monetary policy variable would cause the CPI
of Rwanda to be above or below its natural path as the case may be. The blue
lines depict the estimated effects, while the dashed red lines show the
boundaries of a 95% confidence interval.
In Panel 1, we observe that an increase in nominal exchange
rate has an effect of increasing quickly inflation in the first year, and in
the second year it becomes stationary while it increases again in the third
year and becomes stationary in the following years. In general, the increase in
nominal exchange rate has an effect of increasing the inflation in Rwanda as
the blue line is above the natural path. This increase of inflation resulting
from the depreciation of Rwandan currency is as expected by theories and given
the structure of Rwandan economy. Normally, when there is a depreciation of a
country's currency, the theory predicts that the country's exports become cheap
while its imports become expensive.
Panel 2 shows how the CPI of Rwanda responds to an increase of
nominal interest rate. Increase of nominal interest rate in Rwanda has an
effect of decreasing inflation in the first two years. However, in the third
year, inflation increases again and reaches its level of beginning in the
fourth and fifth year. In the following years, inflation is found to decrease.
This is logical because increase in nominal interest rate discourages people to
ask for loans and consequently reduces money into circulation.
Panel 3 shows the response of CPI to a positive shock in money
supply measured by M2. When there is an increase in money supply in Rwanda,
inflation decreases considerably in the first year. However, in the second
year, inflation start increasing again and it reaches its original level in the
seventh but it increases again in the tenth year. It means that, when monetary
authorities realize a need of stimulating production, they increase money into
circulation. That increased money is invested into productive activities which
increase production in Rwanda.
That increase in production results in reduction of prices in
the first year. However, it has been seen that the continual increase of money
supply push again prices up in the following years and prices reaches its
original level in the seventh year.
|