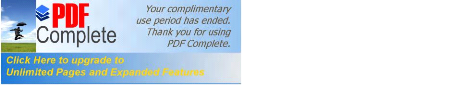
My dear parents.
uman beings who are the most to me dear:
Nothing in the world could compensate the sacrifices you have
made for my education and for my welfare so I can focus me on my studies.
Able to God, the Almighty provides you with health, prosperity and
longevity.
Thank you for your help, your support and your patience. I
dedicate this modest work as a sign of recognition and of
admiration.
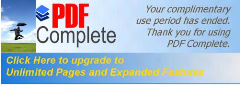
I would like to express my gratitude and respectful appreciation
to everyone who helped me close or far in the achievement of this work, and
more particularly to:
Honorable Senator Professor Dr. RWIGAMBA BALINDA, Founder and
President of ULK, for his initiative and innovation for the development of
education in our country.
We also wish to thank the Rector of ULK Dr. Alphonse NGAGI and
Faculty of ULK more specially those of the Faculty of Economics and Management,
especially those of the Economics Department for the scientific support we
receive from them.
My gratitude will also to CCA Mme Brigitte GAJU, my direct
supervisor for the follow-up given to the conduct of this work: I am very
grateful have framed me, lead this work and to ensure its development in not
gentle your time or your advice. Be ensured, expensive Supervisor, yours and
have my deep respect.
To my classmates of the 4th year in economics at
ULK, promotion 2008 / 2009. We spent of pleasant brotherhood, of camaraderie,
effort and perseverance, I hope you all will have one long career in economics
and a better life both in terms Professional staff. To all those showing me
their sympathy and that I consider as friends full.
Besides my project, I really enjoyed my stay at the NBR,
appreciated all the people I worked with and spent good moments with them.
That's why I also thank Mr. Boniface MUTABAZI manager of middle office and
Pascal MUNYANKINDI for all kind of help. Can God bless you and enables you to
perform all your projects and your aspirations!
Ferdinand GAKUBA
ADF: Augmented Dickey Fuller AER: Average Exchange rate
ARCH: Autoregressive Conditional Heteroskedasticity
BNR: National bank of Rwanda CCI: Continuous commodity index CIF:
Cost, insurance and freight COLA: Cost of Living Allowance COLI: Cost of living
index
CPI: Consumer price index
CRB: Commodity research bureau ECI: Employment cost index
ECM: Error correction model
EURO: European Money
GBP: Great Britain Pound
GDP: Gross domestic production IMF: International Monetary
Fund LR: Likelihood ratio
MINECOFIN: Ministère de la finance NSSF: National
social security fund OLS: Ordinary Least square
PCEPI: Personal consumption expenditure price index
PPI: Producer price index
RBD: Real bill doctrine
RWF: Rwanda franc
ULK: Université Libre de Kigali
ULC : Unit labour cost.
USA: United State of America
VAR: Vector autoregressive
VECM: Vector error correction model XDR: Exchange data
Representation
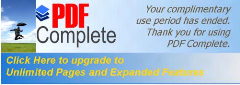
Table 2: ADF Statistics for Testing for a Unit
Root in all Time Series 34
Table 3: Cointegration analysis in the mark-up
model 35
Table 4: Results using OLS
36
Table 5: Properties of VAR residuals
37
Table 6: Standardized adjustment coefficients
38
Table 7: Properties of cointegration vector
38
Table 8: Results for long run inflation model
40
Table 9: Heteroskedasticity test
41
Table 10: Ramsey RESET test
42
Table 11: White test 48
Table 12: ARCH test 48
Table 13: Breusch -Godfrey Test
48
Table 14: Ramsey test 2 49
Table 15: Diagnostic test 52
Table 16: Prevision results
59
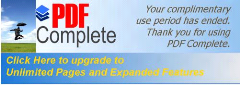
Figure 1: Evolution of Inflation in Rwanda
25
Figure 2: Evolution of money supply and CPI
26
Figure 3: Evolution of import prices and
domestic prices 27
Figure 4: Evolution of Output and inflation
29
Figure 5: CUSUM Test 42
Figure 6: CUSUM squared test
43
Figure 7: CUSUM stability test (Brown, Durbin,
Ewans) 49
Figure 8: CUSUM squared stability test
49
Figure 9: Residuals test 52
Figure 10: Cusum test for Engle- Granger ECM
53
Figure 11: Cusum of squared test for Engle-
Granger ECM 53
Figure 12: Inflation forecasting criteria
54
Figure 13: Response function of variables on
LIPC 57
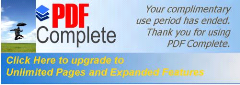
Appendix 1: Data description
Appendix 2: Data
Appendix 3: Residuals properties
Appendix 4: VECM results
Appendix 5: Variance decomposition
Appendix 6: Recursive coefficient test
Appendix 7: ECM with Hendry results
Appendix 8: ECM with Engle- Granger results
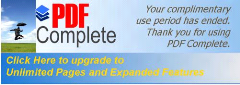
Acknowledgment iiList of acronyms and
abbreviations iiiLIST OF TABLE iv
LIST OF FIGURE v
LIST OF APPENDIX v
TABLE OF CONTENTS vii
Abstract 1
GENERAL INTRODUCTION 2
1. Interest and choice of subject. 2
1.1. Choice 2
1.2. Interests 3
2. Scope of the study
3. Problem statement 3
4. ASSUMPTIONS 4
5. RESEARCH PURPOSE 5
6. RESEARCH TECHNIQUES AND METHODS 5
6.1. TECHNICAL 5
6.1.1. TECHNICAL DOCUMENTARY 5
6.1.2. TECHNICAL INTERVIEW 6
6 .2. METHODS 6
6.2.1. ANALYTICAL METHOD 6
6.2.2. COMPARATIVE METHOD 6
6 .2 .3. STATISTICAL METHOD.
6
7. SUBDIVISION OF WORK 7
CHAPTER I LITERATURE REVIEW 8
1.1. Introduction 8
1.1.1 Inflation 8
1.1.2 Modeling inflation 9
1.1.3 Forecasting inflation 9
1.1.4 Measures of inflation 9
1.2. .Theory of inflation 10
1.2.1 Keynesian view 11
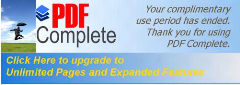
13
ons theory 14
1.2.4 Austrian theory 14
1.2.5 The theory of real bills doctrine 15
1.2.6. Anti-classical or backing theory 16
1.3 The tools for controlling the inflation
16
1.3.1. Monetary policy 16
1.3.2 Fixed exchange rates 17
1.3.3 Wage and price controls 17
1.3.4 Cost-of-living allowance 18
1.4 The history of modeling and forecasting inflation
process 19
1.4.1 Some critical issues for modelling 22
1.4.2 Overview of forecasting 22
1.4.2.1 Monetary transmission 23
1.4.2.2. Flexible price equilibrium 24
CHAPTER II. INFLATION MODELLING AND CONCEPTUAL FRAMEWORK
25
2.1. Rwanda's inflation experience 25
2.1.1. Foreign factors 27
2.1.2 Domestic factors 28
2.2. Conceptual framework 29
2.2.1. LONG- RUN RELATIONSHIPS 30
2.2.1.1. Mark up 30
2.2.1.2 Money supply determinants 31
2.2.2. Data descriptions 32
2.3. Estimation of inflation models 33
2.3.1. Mark up 33
2.3.1.1 Integration 33
2.3.1.2. Cointegration 34
2.3.2 Excess money supply 37
2.4 THE LONG RUN MODEL OF INFLATION 38
2.4.1 Interpretation of coefficients 41
2.4.2 Classical Tests 41
CHAPTER III FORECAST INFLATION OF RWANDA USING ECM
45
3.1 Introduction 45
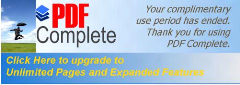
inflation 45
45
3.2.1.1 Long run and short run elasticity 46
3.2.1.2 Significativity of error correction model
47
3.2.2 The ECM with Engle - Granger 50
3.2.2.2 The model properties 52
3.3. Forecasting inflation using VECM 55
3.3.1 Why a VECM? 55
3.3.2. Forecasting performance 56
3.3.2.1 The variance decomposition 58
.3.3.2.2 Forecast results 58
General conclusion 59
Discussion 63
References 64
APPENDIX 67
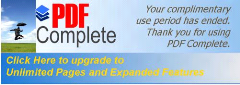
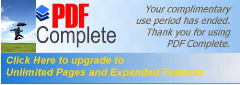
Inflation constitutes one of the major economic problems in
emerging market economies that requires monetary authorities to elaborate tools
and policies to prevent high volatility in prices and long periods of
inflation. Analysis of inflation and its relationship with the important
macroeconomic indicators is an arduous task due to data problems (availability,
measurement errors, biases, etc.) and complexity of the transition process
experienced by the economy of Rwanda. In order to model inflation dynamics we
use the general-to-specific approach. The advantage of this approach is its
ability to deliver results based on underlying economic theories of inflation,
which are also consistent with the properties of the data. A three steps
procedure is followed. In the first step, the long-run sectoral analysis of
inflation sources is conducted, yielding long-run determinants of inflation
(excess money, nominal effective exchange rate, nominal wages expressed as unit
labor cost, import prices, oil prices index and the nominal GDP). In the second
step, we estimate an equilibrium error correction model first of all by
following Hendry procedures, then building ECM by Engle Granger and compare
forecasting criteria of inflation deploying among other variables of interest
for the long-run solutions derived in the first step. Lastly, equilibrium error
correction model obtained will serve for structural model-based inflation
forecasting. Forecasting performance of the model will be compared to other
models often utilized for forecasting inflation suggests that markup and excess
money relationships are very important for explaining the short-run behaviour
of inflation, as well as the output, nominal effective exchange rate, oil
prices and import prices in Rwanda.
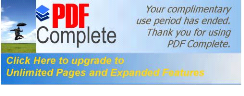
1. Interest and choice of subject
1.1. Choice
The aim of this paper is to construct a quarterly inflation
model and forecasts quarterly-year ahead inflation for Rwanda. Inflation is
considered to be a major economic problem in transition economies and thus
fighting inflation and maintaining stable prices is the main objective of
monetary policy makers. The negative consequences of inflation are well known.
Inflation can result in a decrease in the purchasing power of the national
currency leading to the aggravation of social conditions and living standards.
High prices can also lead to uncertainty making domestic and foreign investors
reluctant to invest in the economy. Moreover, inflated prices worsen the
country's terms of trade by making domestic goods expensive on regional and
world markets.
To develop an effective monetary policy, central banks should
possess information on the economic situation in the country, the behaviour and
interrelationships of major macroeconomic indicators. Such information would
enable the central bank to predict future macroeconomic developments and to
react in a proper way to shocks the economy is subject to. Thus, studying
inflationary processes is an important issue for monetary economists all around
the world. However, it is not an easy task, especially in developing countries,
where economic processes are highly unstable and volatile. Moreover, the
macroeconomic data on developing countries can be unreliable due to many
reasons: measurement error, imperfect methods of measuring, etc. Nevertheless,
there exist a number of empirical studies on inflation factors in developing
countries.
Since inflation is a phenomenon that characterizes almost all
economies as it is developed or not, but particularly developing countries
including our country is, within this framework that we considered relevant to
the topic, hence the rationale for choosing this subject.
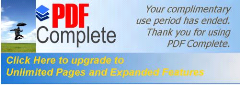
At academic level, this work will constitute an important source
of data, both theoretical and practical, researchers, students from different
faculties, professors, and all the whole community who will look for modelling
and forecasting inflation in their research. This topic has the interest to
deepen our understanding of inflation models and its prediction in the context
of developing economy and make our contribution to science. This topic has the
interest to know the relationship of inflation with others macroeconomic
variables
2. Scope of the study
Our research as any other scientific work is limited in time,
space and in the domain. In time, we focused our analysis on the period from
1995 to 2009 because the period allowed us to search recent data related to our
subject.
Regarding the delimitation of space, our study focuses on
Rwandan territory. In the field, our study focuses on macroeconomic.
3. Problem statement
Since Rwanda covered its monetary sovereignty the monetary
policy followed was with a direct character thus straightforwardly to manage
the banking function this means that the development of its activities was to
be contained within the limits deemed compatible with the overall trend of
discounting the economy to avoid any risk of deviation1.
This policy has been unchanged until the end of the Eighties
years but like almost everywhere in the world, managed finance proved in Rwanda
inefficient and its prevalence has violated not only the conditions of
financing the economy but also, and especially, the allowance of the financial
resources available and hence two major consequences could appear; the absence
of optimal allowance of the financial resources available and sometimes even by
monetary creation ex nihilo (excess
1 Ia politique
monétaire au Rwanda; une mutation constante pour une efficience accrue,
juin, 2003
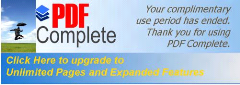
g 1990 its was the first foreground of structural
chanism started coming into force then replace the procedures
of administrative management. The direct control operated before on both sides
by the concerned official structures thus had to disappear gradually and free
plan of the supply and the demand were to allow the determination of the
different price level of the various markets.
The monetary policy of Rwanda was the subject of a deep change
with the imminent emergence of new instruments of the monetary policy which had
some modification of the structure to even giving a more and more accentuated
at indirect character.
Final objective is the price stability (ultime objective) but
the achievement of this objective is conditioned by another intermediate
objective that constitutes a walking point which is a vital crossing point of
the action of central bank initiated in this context. It acts in the case of
Rwanda by controlling the evolution of money supply expressed in a broad sense
(M2). Achieving the goal also requires an intermediate operational target which
in the context of Rwanda is the monetary base by considering the multiplier
stable.
Monetary management ensured by the central Bank of Rwanda
still faced major obstacles which affecting more the quality of the results
obtained because the lack of a management model that can serve as tool for
projecting future, this hampers monetary policy and reach on the price
volatility persistence which is his ultimate goal.
The aim of this study is to build a model for the money supply
and to build a model of inflation which can be used to forecast the future
uncertain. This allows as formulating the following assumptions;
H1: «Inflation is everywhere and always a monetary
phenomenon» we wonder if this is same case in Rwanda and what are main
causes of inflation in Rwanda?
H2: How can we use these variables to forecast future inflation
in Rwanda?
4. HYPOTHESIS
A hypothesis is an early response to questions that arose in the
problem and must be confirmed to achieve a result.
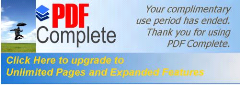
hypotheses:
d by excess money supply, However there are other factors like,
low level of production, high wages, high level of import rising market prices,
nominal exchange rates, and macroeconomic instability, etc.
· . In this way presenting a set of four-step-ahead
forecasts bounded by estimated confidence bands we can present an outlook that
is more informative about the development of the general direction of prices,
and one that explicitly recognizes the uncertainty inherent in forecasting
inflation with a long lead. Accordingly, we argue that monetary policy is
probably best served by drawing on models that summarize different paradigms of
the transmission mechanism, or that use different technical approaches to
represent the transmission mechanism. Taking such strategy, diversified
approach to inform policy judgements is likely to reduce the risk of making
serious policy errors.
5. RESEARCH OBJECTIVES
The main objective of our work is to construct the inflation
model which could be
used in short -term forecasting inflation in Rwanda.
In our work we pursue the following specific objectives:
· Show the different variables which explain inflation in
Rwanda
· Propose economic policies to fight against inflation in
Rwanda
· Give the model of inflation which should be used as
forecast tool
6. RESEARCH TECHNIQUES AND METHODS
6.1. TECHNIQUES of inflation deploying, among
other variables of interest
A technique is defined as all resources and processes that
enable researchers to gather data and information on the research
topic2.
Thus, we preferred the following techniques:
6.1.1. DOCUMENTARY TECHNIQUE
2 WELMAN J. C and KRUGER
S.J.(2001) : Research methodology course for the business and administrative
sceines , 2nd edition , Paris, Durod , page 34
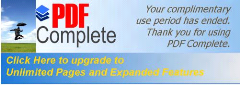
s a systematic search of all that is written related with the
research area such as books, pamphlets, monographs, unpublished documents,
reports, budgets, public records etc. the documentary technical allows us to
choice among the books available what are useful for our research and help to
use the best resources.
6.1.2. TECHNIQUE OF INTERVIEW
This technique is to maintain discussions and dialogues with
people who provide the researcher's information on his research topic. This
technique allowed us to interact with senior officials of the Rwanda Revenue
Authority, those of MINECOFIN and BNR those of us who have talked about
everything that is related to inflation in Rwanda.
6 .2. METHODS
A method is defined as an ordered set of rules and principles of
intellectual operations to do the analysis to achieve a result3.
At the completion of our work, we have chosen the following
methods:
6.2.1. ANALYTICAL METHOD
This method allows to systematically analyzing all information
and data collected. It allowed us to systematically analyze the inflation's
relationship with others macroeconomic variables, to interpret and draw the
conclusion.
6.2.2. COMPARATIVE METHOD
This method allowed us to compare model adopted with others to
identify the link between those.
6 .2 .3. STATISTICAL METHOD
3 WELMAN J. C and KRUGER
S.J.(2001) : Research methodology course for the business and administrative
sceines , 2nd edition , Paris, Durod , page 36
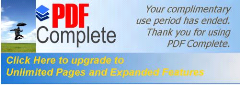
ing the data, synthesizing the results of research by first.
Second, it allowed us to present these results as graphs, tables, to facilitate
reading and understanding of our work.
7. ORGANIZATIONAL OF WORK
Besides the general introduction, this work is organised as
follows. In chapter one we will state some empirical literature. Chapter 2
illustrates the identification of the inflation models. Chapter 3 gives the
description of Error correction models, forecasting principles and the
estimation results. In that version of the VECM, some variables are exogenous
or became unstable in simulations over the long run. In the extended version of
the VECM developed here, we impose sensible long-run conditions that help to
determine the behaviour of the inflation for Rwanda, interest rates, and the
exchange rate. As result, we should be able to have more confidence in the
long-run and dynamic properties of the model.
The third chapter deals with the analysis of inflation; we
consider the forecast accuracy of the extended VECM in an unconditional,
out-of-sample exercise. We also illustrate that a more informative way to
present inflation forecasts than simply providing the point estimate of the
model is to include as well a statement about the probability distribution of
potential outcomes. By drawing on the model's estimated variance covariance
matrix, we generate confidence intervals around a set of 4-stepahead forecasts,
with each forecast projecting the one quarter inflation rate six-quarter
further into the future.
Finally, we will close our work with a general conclusion and
discussion.
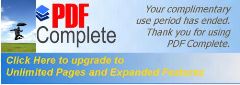
EW
1.1.Introduction
1.1.1 Inflation
In economics, inflation is a rise in the general level of
prices of goods and services in an economy over a period of time4.
When the price level rises, each unit of currency buys fewer goods and
services; consequently, inflation is also erosion in the purchasing power of
money a loss of real value in the internal medium of exchange and unit of
account in the economy5. A chief measure of price inflation is the
inflation rate, the annualized percentage change in a general price index
(normally the consumer price index over time).
The term "inflation" usually refers to a measured rise in a
broad price index that represents the overall level of prices in goods and
services in the economy. The Consumer Price Index (CPI), the Personal
Consumption Expenditures Price Index (PCEPI) and the GDP deflator are some
examples of broad price indices6. The term inflation may also be
used to describe the rising level of prices in a narrow set of assets, goods or
services within the economy, such as commodities (which include food, fuel,
metals), financial assets (such as stocks, bonds and real estate), and services
(such as entertainment and health care). The Reuters-CRB Index (CCI), the
Producer Price Index, and Employment Cost Index (ECI) are examples of narrow
price indices used to measure price inflation in particular sectors of the
economy. Asset price inflation is a rise in the price of assets, as opposed to
goods and services7. Core inflation is a measure of price
fluctuations in a sub-set of the broad price index which excludes food and
energy prices8. The Federal Reserve Board uses the core inflation
rate to measure overall inflation, eliminating food and energy
4 Abel & Bernanke
(1995); Inflation determinants in transition economy, November
,2006
5 Walgenbach P. H., Norman
E. Dittrich and Ernest I. Hanson, (1973), The Measuring Unit principle,
Page 429
6 Blundell-Wignall,
A.(ed.)(1992), Inflation, Disinflation and Monetary Policy, Reserve Bank
of Australia, Sydney, page 112
7
http://en.wikipedia.org/wiki/Asset_price_inflation
8 Robert Rich, Charles
Steindel (2005):A Review of Core Inflation and an Evaluation of Its
Measures (Staff Report, Federal Reserve Bank of New York
|
rm price fluctuations that could distort estimates of the
general economy9.
|
|
1.1.2 Modeling inflation
Modeling inflation is a simplified representation of
inflation system or phenomenon, as in the sciences or economics, with any
hypotheses required to describe the system or explain the phenomenon, often
mathematically10.
1.1.3 Forecasting inflation
The forecasting inflation is one of the tools which can be
used with the policy makers when the policy adopted by central bank has
inflation as ultime objective for monetary purpose it helps by explaining its
uses and how it relates to planning and formulate the problem to the use of the
forecast11.
1.1.4 Measures of inflation
Inflation is usually estimated by calculating the inflation
rate of a price index, usually the Consumer Price Index. The Consumer Price
Index measures prices of a selection of goods and services purchased by a
"typical consumer" The inflation rate is the percentage rate of change of a
price index over time12
Other widely used price indices for calculating price inflation
include the following:
· Cost-of-living index (COLI) is index similar to the
CPI which is often used to adjust fixed incomes and contractual incomes to
maintain the real value of those incomes.
· Producer price index (PPI) which measures average
changes in prices received by domestic producers for their output13.
This differs from the CPI in that price subsidization, profits, and taxes may
cause the amount received by
9 Kiley, Michael J. (2008).
Estimating the common trend rate of inflation for consumer prices and
consumer prices excluding food and energy prices, Federal Reserve
Board
10 Encyclopedia
11 Armstrong J. S
(2001):Principles of forecasting; A handbook for researchers and
practitioners, USA 2001
12 Taylor & Hall
1993
13 Encyclopedia
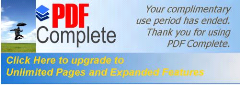
what the consumer paid. There is also typically a
e in the PPI and any eventual increase in the CPI. Producer
price index measures the pressure being put on producers by the costs of their
raw materials. This could be "passed on" to consumers, or it could be absorbed
by profits, or offset by increasing productivity.
· Commodity price indices, which measure the price of a
selection of commodities. In the present commodity price indices are weighted
by the relative importance of the components to the "all in" cost of an
employee.
· Core price indices: because food and oil prices can
change quickly due to changes in supply and demand conditions in the food and
oil markets, it can be difficult to detect the long run trend in price levels
when those prices are included.
Other common measures of inflation are:
· GDP deflator is a measure of the price of all the
goods and services included Gross Domestic Product (GDP). The Rwanda Commerce
Department publishes a deflator series for Rwanda GDP, defined as its nominal
GDP measure divided by its real GDP measure.
Asset price inflation is an undue increase in the prices of
real or financial assets, such as stock (equity) and real estate. While there
is no widely-accepted index of this type, some central bankers have suggested
that it would be better to aim at stabilizing a wider general price level
inflation measure that includes some asset prices, instead of stabilizing CPI
or core inflation only. The reason is that by raising interest rates when stock
prices or real estate prices rise, and lowering them when these asset prices
fall, central banks might be more successful in avoiding bubbles and crashes in
asset prices14.
1.2..Theory of inflation
Historically, a great deal of economic literature was
concerned with the question of what causes inflation and what effect it has.
There were different schools of thought as to the causes of inflation. Most
can be divided into two broad areas: quality
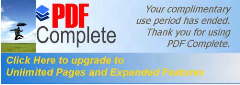
theories of inflation. The quality theory of inflation
ler accepting currency to be able to exchange that currency
at a later time for goods that are desirable as a buyer. The quantity theory of
inflation rests on the quantity equation of money, that relates the money
supply, its velocity, and the nominal value of exchanges. Adam Smith and David
Hume proposed a quantity theory of inflation for money, and a quality theory of
inflation for production.
Currently, the quantity theory of money is widely accepted as
an accurate model of inflation in the long run. Consequently, there is now
broad agreement among economists that in the long run, the inflation rate is
essentially dependent on the growth rate of money supply. However, in the short
and medium term inflation may be affected by supply and demand pressures in the
economy, and influenced by the relative elasticity of wages, prices and
interest rates15.
The question of whether the short-term effects last long
enough to be important is the central topic of debate between monetarist and
Keynesian economists. In monetarism prices and wages adjust quickly enough to
make other factors merely marginal behavior on a general trend-line. In the
Keynesian view, prices and wages adjust at different rates, and these
differences have enough effects on real output to be "long term" in the view of
people in an economy.
1.2.1 Keynesian view
Keynesian economic theory proposes that changes in money
supply do not directly affect prices, and that visible inflation is the result
of pressures in the economy expressing themselves in prices. The supply of
money is a major, but not the only, cause of inflation. There are three major
types of inflation, as part of what Robert J. Gordon calls the "triangle
model16":
14 Mankiw (2002):
Macroeconomics principles,, p. 22-32
15 Federal Reserve Board's semiannual Monetary Policy
Report to the Congress Round table; Introductory statement by Jean-Claude
Trichet on July 1, 2004
16 Robert J. Gordon
(1988), Macroeconomics, Addison Wesley, 2002 ISBN
0-201-77036-9
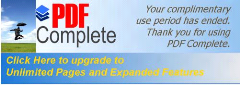
caused by increases in aggregate demand due to
overnment spending, etc17. Demand inflation is
constructive to a faster rate of economic growth since the excess demand and
favorable market conditions will stimulate investment and expansion.
· Cost-push inflation, also called
"supply shock inflation," is caused by a drop in aggregate supply (potential
output). This may be due to natural disasters, or increased prices of inputs.
For example, a sudden decrease in the supply of oil, leading to increased oil
prices, can cause cost-push inflation. Producers for whom oil is a part of
their costs could then pass this on to consumers in the form of increased
prices18.
· Built-in inflation or structural
inflation is an economic term referring to type of inflation that result from
past events and persists in the present. It thus might be called hangover
inflation, expectations, and is often linked to the "price/wage spiral". It
involves workers trying to keep their wages up with prices (above the rate of
inflation), and firms passing these higher labor costs on to their customers as
higher prices, leading to a 'vicious circle'. Built-in inflation reflects
events in the past, and so might be seen as hang over
inflation19.
Some Keynesian economists also disagree with the notion that
central banks fully control the money supply, arguing that central banks have
little control, since the money supply adapts to the demand for bank credit
issued by commercial banks20. This is known as the theory of
endogenous money, and has been advocated strongly by post-Keynesians as far
back as the 1960s. It has today become a central focus of Taylor rule
advocates. This position is not universally accepted banks create money by
making loans, but the aggregate volume of these loans diminishes as real
interest rates increase. Thus, central banks can influence the money supply by
making money cheaper or more expensive, thus increasing or decreasing its
production.
A fundamental concept in inflation analysis is the
relationship between inflation and unemployment, called the Phillips curve.
This model suggests that there is a trade-off
17
http://www.bized.co.uk/virtual/bank/economics/mpol/inflation/causes/theories1.htm,Retrieved
1/11/2009
18 Encyclopedia Britannica,
"The cost-push theory"
19
http://en.wikipedia.org/wiki/Built-in_inflation,
Retrieved 1/11/2009
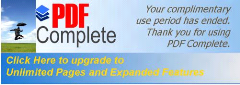
oyment. Therefore, some level of inflation could be
minimize unemployment. The Phillips curve model described the
U.S. experience well in the 1960s but failed to describe the combination of
rising inflation and economic stagnation (sometimes referred to as stagflation)
experienced in the 1970s21
1.2.2 Monetarist view
Monetarists believe the most significant factor influencing
inflation or deflation is the management of money supply through the easing or
tightening of credit. They consider fiscal policy, or government spending and
taxation, as ineffective in controlling inflation22.
Monetarists assert that the empirical study of monetary
history shows that inflation has always been a monetary phenomenon. The
quantity theory of money, simply stated, says that the total amount of spending
in an economy is primarily determined by the total amount of money in
existence. This theory begins with the identity:
Where;
M is the quantity of money.
V is the velocity of money in final expenditures;
P is the general price level;
Q is an index of the real value of final expenditures;
In this formula, the general price level is affected by the
level of economic activity (Q), the quantity of money (M) and the velocity of
money (V). The formula is an identity because the velocity of money (V) is
defined to be the ratio of final expenditure to the quantity of money (M).
Velocity of money is often assumed to be constant, and the real
value of output is determined in the long run by the productive capacity of
the economy. Under these
20 Gordon, Robert J.
(2000), "Does the 'New Economy' measure up to the great Inventions of the
Past?", Journal of Economic Perspectives 14 (4): 49-74
21 Mankiw
2002,Macroeconomics principles, p. 65-77
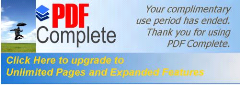
f the change in the general price level is changes in
nstant velocity, the money supply determines the value of
nominal output (which equals final expenditure) in the short run. In practice,
velocity is not constant, and can only be measured indirectly and so the
formula does not necessarily imply a stable relationship between money supply
and nominal output. However, in the long run, changes in money supply and level
of economic activity usually dwarf changes in velocity. If velocity is
relatively constant, the long run rate of increase in prices (inflation) is
equal to the difference between the long run growth rate of money supply and
the long run growth rate of real output23.
1.2.3. Rational expectations theory
Rational expectations theory holds that economic actors look
rationally into the future when trying to maximize their well-being, and do not
respond solely to immediate opportunity costs and pressures24. In
this view, while generally grounded in monetarism, future expectations and
strategies are important for inflation as well.
A core assertion of rational expectations theory is that
actors will seek to "head off" central-bank decisions by acting in ways that
fulfill predictions of higher inflation. This means that central banks must
establish their credibility in fighting inflation, or have economic
actors25 make bets that the economy will expand, believing that the
central bank will expand the money supply rather than allow a recession.
1.2.4 Austrian theory
The Austrian School asserts that inflation is an increase in
the money supply, rising prices are merely consequences and this semantic
difference is important in defining inflation26
Austrian economists believe that there is no material
difference between the concepts of monetary inflation and general price
inflation. Austrian economists
22 Lagassé, Paul
(2000).Columbia encyclpedia,ISBN-10 0787650153 page 556-558
23 Mankiw 2002,
Macroeconomics principles, pp. 81-107
24 Dowyer,J.and R. Lam (1994), Economic and Financial
Research in the Reserve Bank in 1994
25 Sargent, Thomas J. Rational Expectations and
Inflation. New York: Harper and Row, 1986.
26 Shostak, Ph. D, Frank
(2002-03-02). "Defining Inflation". Mises Institute.
http://mises.org/story/908.
Retrieved 2009-10-10
lculating the growth of new units of money that are change, that
have been created over time27.
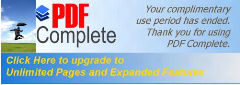
This interpretation of inflation implies that inflation is
always a distinct action taken by the central government or its central bank,
which permits or allows an increase in the money supply28. In
addition to state-induced monetary expansion, the Austrian School also
maintains that the effects of increasing the money supply are magnified by
credit expansion, as a result of the fractional-reserve banking system employed
in most economic and financial systems in the world29
Austrians argue that the state uses inflation as one of the
three means by which it can fund its activities (inflation tax), the other two
being taxation and borrowing. Various forms of military spending is often cited
as a reason for resorting to inflation and borrowing, as this can be a short
term way of acquiring marketable resources and is often favored by desperate,
indebted governments30.
1.2.5 The theory of real bills doctrine
Within the context of a fixed species basis for money, one
important controversy was between the quantity theory of money and the real
bills doctrine (RBD). Within this context, quantity theory applies to the level
of fractional reserve accounting allowed against specie, generally gold, held
by a bank. Currency and banking schools of economics argue the RBD, that banks
should also be able to issue currency against bills of trading, which is "real
bills" that they buy from merchants. This theory was important in the 19th
century in debates between "Banking" and "Currency" schools of monetary
soundness, and in the formation of the Federal Reserve. In the wake of the
collapse of the international gold standard post 1913, and the move towards
deficit financing of government, RBD has remained a minor topic, primarily of
interest in limited contexts, such as currency boards. It is generally held in
ill repute today, with Frederic Mishkin, a governor of the Federal Reserve
going so far as to say it had been "completely discredited." Even so, it has
theoretical support from a few
27 Joseph T. Salerno, (1987),
Austrian Economic Newsletter, "<a href="
http://www.mises.org/journals/aen
Retrieved 2009-10-10
28 Ludwig von Mises
Institute, "True Money Supply; page 456
29 Joseph T. Salerno,
(1987),Quarterly Journal of economics Facts, Discussion Forum
-356
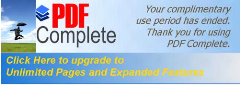
at see restrictions on a particular class of credit as nciples of
laissez-faire, even though almost all libertarian economists are opposed to the
RBD.
The debate between currency, or quantity theory, and banking
schools in Britain during the 19th century prefigures current questions about
the credibility of money in the present. In the 19th century the banking school
had greater influence in policy in the United States and Great Britain, while
the currency school had more influence "on the continent", that is in
non-British countries, particularly in the Latin Monetary Union and the earlier
Scandinavia monetary union31.
1.2.6. Anti-classical or backing theory
Another issue associated with classical political economy is
the anti-classical hypothesis of money, or "backing theory". The backing theory
argues that the value of money is determined by the assets and liabilities of
the issuing agency32. Unlike the Quantity Theory of classical
political economy, the backing theory argues that issuing authorities can issue
money without causing inflation so long as the money issuer has sufficient
assets to cover redemptions.
1.3 The tools for controlling the inflation
A variety of methods have been used in attempts to control
inflation.
1.3.1. Monetary policy
Monetarists emphasize keeping the growth rate of money steady,
and using monetary policy to control inflation (increasing interest rates,
slowing the rise in the money supply). Keynesians emphasize reducing aggregate
demand during economic expansions and increasing demand during recessions to
keep inflation stable. Control of aggregate demand can be achieved using both
monetary policy and fiscal policy (increased taxation or reduced government
spending to reduce
30 Ludwig von Mises, The
Theory of Money and Credit, page23-56
31 Selgin, G. A, "The
Analytical Framework of the Real Bills Doctrine",Journal of Institutional
and Theoretical Economics, volume 145, (1989), p. 489.
32 Ron Paul, "The Case for
Gold, page 45-56
l for controlling inflation is monetary policy. Most ping the
federal funds lending rate at a low level.
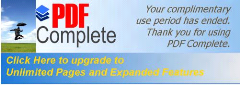
1.3.2 Fixed exchange rates
Under a fixed exchange rate currency regime, a country's
currency is tied in value to another single currency or to a basket of other
currencies (or sometimes to another measure of value, such as gold). A fixed
exchange rate is usually used to stabilize the value of a currency,
vis-à-vis the currency it is pegged to. It can also be used as a means
to control inflation. However, as the value of the reference currency rises and
falls, so does the currency pegged to it. This essentially means that the
inflation rate in the fixed exchange rate country is determined by the
inflation rate of the country the currency is pegged to. In addition, a fixed
exchange rate prevents a government from using domestic monetary policy in
order to achieve macroeconomic stability.
Under the Bretton Woods agreement, most countries around the
world had currencies that were fixed to the US dollar. This limited inflation
in those countries, but also exposed them to the danger of speculative attacks.
After the Bretton Woods agreement broke down in the early 1970s, countries
gradually turned to floating exchange rates. However, in the later part of the
20th century, some countries reverted to a fixed exchange rate as part of an
attempt to control inflation. This policy of using a fixed exchange rate to
control inflation was used in many countries in South America in the later part
of the 20th century (e.g. Argentina (1991-2002), Bolivia, Brazil, and
Chile)33.
1.3.3 Wage and price controls
Another method attempted in the past has been wage and price
controls ("incomes policies"). Wage and price controls have been successful in
wartime environments in combination with rationing. However, their use in other
contexts is far more mixed. Notable failures of their use include the 1972
imposition of wage and price controls
33 Edwards, Sebastian. (2002)
The Great Exchange Rate Debate after Argentina, The North American
Journal of Economics and Finance, Volume 13, Issue 3, pp. 237-252
essful examples include the Prices and Incomes enaar Agreement in
the Netherlands.
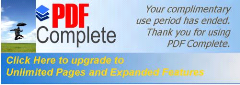
In general wage and price controls are regarded as a temporary
and exceptional measure, only effective when coupled with policies designed to
reduce the underlying causes of inflation during the wage and price control
regime, for example, winning the war being fought. They often have perverse
effects, due to the distorted signals they send to the market. Artificially low
prices often cause rationing and shortages and discourage future investment,
resulting in yet further shortages. The usual economic analysis is that any
product or service that is under-priced is over consumed.
However, in general the advice of economists is not to impose
price controls but to liberalize prices by assuming that the economy will
adjust and abandon unprofitable economic activity. The lower activity will
place fewer demands on whatever commodities were driving inflation, whether
labor or resources, and inflation will fall with total economic output. This
often produces a severe recession, as productive capacity is reallocated and is
thus often very unpopular with the people whose livelihoods are destroyed.
1.3.4 Cost-of-living allowance
The real purchasing-power of fixed payments is eroded by
inflation unless they are inflation-adjusted to keep their real values
constant. In many countries, employment contracts, pension benefits, and
government entitlements (such as social security) are tied to a cost-of-living
index, typically to the consumer price index35. A cost-ofliving
allowance (COLA) adjusts salaries based on changes in a cost-of-living index.
Salaries are typically adjusted annually. They may also be tied to a
cost-of-living index that varies by geographic location if the employee
moves.
Annual escalation clauses in employment contracts can specify
retroactive or future percentage increases in worker pay which are not tied
to any index. These
34Richard Milhous Nixon
(January 9, 1913 - April 22, 1994) was the 37th President of the United States
(1969- 1974) and is the only president to resign the office. He was also the
36th Vice President of the United States (1953-1961). See bibliography USA
president
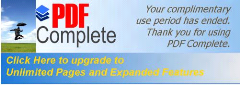
colloquially referred to as cost-of-living adjustments
se of their similarity to increases tied to
externally-determined indexes. Many economists and compensation analysts
consider the idea of predetermined future "cost of living increases" to be
misleading for two reasons:
(1) For most recent periods in the industrialized world,
average wages have increased faster than most calculated cost-of-living
indexes, reflecting the influence of rising productivity and worker bargaining
power rather than simply living costs, and
(2) Most cost-of-living indexes are not forward-looking, but
instead compare current or historical data.
1.4 The history of modeling and forecasting inflation
process
Since inflation was an economic issue numerous attempt to
model inflation in developed countries as well as developing countries was
made. For example, Juselius (1992) in her seminal paper on inflation modeling
in a small open economy studied the inflationary processes in Denmark. De
Brower and Ericsson (1998) wrote an appealing paper on inflation modeling in
Australia.
Both studies serve as important theoretical and methodological
references for our later empirical research in the field of macroeconomic
modeling. Welfe (2000) modeled inflation in Poland, accounting for a number of
important features that characterize a transition economy. Besides these, worth
noting are Ramakrishnan and Vamvakidis (2002)who worked on a model to forecast
inflation behavior in Indonesia, and Callen and Chang (1999) who conducted an
empirical study on inflation in India.
Most of the literature in the field constitutes empirical
studies for modeling inflationary processes in different countries and
inflation factors in general. These studies follow approaches based on
different economic theories, choosing the most appropriate for the economy
investigated.
35 DeLong, Brad.
«Why Not the Gold Standard?»
http://www.j-bradford-
delong.net/Politics/whynotthegoldstandard.html.
Retrieved 2009-09-25.
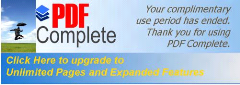
nflation, the study by Loungani and Swagel (2001)
s a starting point for understanding inflation in developing
countries. The authors present stylized facts about inflation behavior in
developing countries, focusing primarily on the relationship between the
exchange rate regime and the sources of inflation. Another important study of
inflationary processes was accomplished by Fischer, Sahay, and Végh
(2002) on the experiences of hyper and high inflations in various countries.
The authors found that there is a very strong relationship between money growth
and inflation both in the long and short run.
Golinelli and Orsi (2002) study the inflation processes in
three new EU member countries: the Czech Republic, Hungary and Poland. All
three countries possess a similar historico- conomic background and similar
economic context: they were administrative economies before and have undergone
major systemic changes during the transition to a market economy. Investigating
inflationary processes in these countries is of great importance because all
countries experienced high inflation episodes during the years of transition,
and price stabilization policies played an important role in their successful
transition to a new economic system. The authors follow a methodology very
close to that of Juselius (1992) in modeling inflation behavior in the
countries under consideration. They use the multivariate VAR approach, grouping
together those determinants that belong to main inflation theories: cost pushed
inflation, foreign prices and exchange rates, and excess money. Further, a
vector equilibrium correction model specification is used since it enables
capturing short-term dynamics by including stationary variables and past
imbalances, i.e.the «gaps» detected by previous cointegrated
relationships.
In his study on the determinants of inflation in Ukraine, the
author, Lissovolik (2003), studies the factors of inflation in Ukraine during
the period from 1993 to 2002, the so-called «transition period». The
most relevant stylized facts important for modeling inflation behavior in
Ukraine appear to be domestic financial instability, external disequilibria,
seasonality of the economy, and allowance for an increase in administered
prices. The resulting equation of an inflation model is a version of a
long-term markup of prices over wages, the exchange rate, administrative
prices, short-term factors and dummy variables.
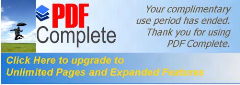
are concerned with the problem of significant dollarization in
transition economies, and its impact on the money demand and inflation. In
particular, the authors study the case of Russia, and illustrate that all the
measures of money aggregates that exclude foreign currency are negatively
correlated with the nominal depreciation rate. This could suggest that foreign
currency has been an important substitute for domestic money. The authors
estimate an equilibrium correction model (ECM) for inflation in order to
identify how the short-term dynamics of inflation are affected by deviations
from the long-term money demand equation. They found that inflation does not
react significantly to the excess supply of monetary aggregates that exclude
foreign currency. Payne (2002) explores inflationary dynamics in Croatia using
vector auto regression over the period January 1992-December 1999. The VAR
incorporated four variables: broad money supply, retail price index1, nominal
net wage per employee and the nominal effective exchange rate36. The
model results suggest that wage increase and currency depreciation are
positively correlated with inflation rates.
Building on Paynes model, BotriL and Cota (2006) model
Croatian inflation dynamics using structural vector
autoregression37. Thay found that terms of trade and balance of
payment shocks have the strongest impact on prices. The authors find
justification for such result in Croatia being a small open economy with high
import dependency and uncompetitive economic structure. In order to contrast
these findings, the authors also re-estimated Paynes model. While Paynes
conclusion on influence of wages and currency depreciation on prices still
holds, in newly estimated four-variable VAR positive correlation between broad
money and prices and some inflation inertia also emerged.
36 Payne, James E., 2002, «Inflationary Dynamics
of a Transition Economy: the Croatian
Experience», Journal of Policy Modeling, 24(3), pp.
219-30.
37 Botri[I, Valerija and Boris Cota, 2006,
«Sources of Inflation in Transition Economy: The Case of
Croatia», Ekonomski pregled, 57(12), pp. 835-855.
The quantitative macroeconomic modelling fell out of favour
during the 1970s for two related reasons: First, some of the existing models,
like the Wharton econometric model and the Brookings model, failed
spectacularly to forecast the stagflation of the 1970s. Second, leading
macroeconomists levelled harsh criticisms of these frameworks. Lucas (1976) and
Sargent (1981), for example, argued that the absence of an optimization-based
approach to the development of the structural equations meant that the
estimated model coefficients were likely not invariant to shifts in policy
regimes or other types of structural changes. Similarly, Sims (1980) argued
that the absence of convincing identifying assumptions to sort out the vast
simultaneity among macroeconomic variables meant that one could have little
confidence that the parameter estimates would be stable across different
regimes. These powerful critiques clarified why econometric models fit largely
on statistical relationships from a previous era did not survive the structural
changes of the 1970s.
1.4.2 Overview of forecasting
Forecasting inflation is clearly of critical importance to the
conduct of monetary policy, regardless of whether or not the central bank has a
numerical inflation target.There are many literatures about inflation forecast
for example using the generalized Phillips curves (i.e. using forecasting
models where inflation depends on past inflation, the unemployment rate and
other predictors) developed by Dimitris K.(2009)38 This literature
is too voluminous to survey here, but a few representative and influential
papers include Ang, Bekaert and Wei (2007), Atkeson and Ohanian (2001), Groen,
Paap and Ravazzolo (2008) and Stock and Watson (1999). The details of these
papers differ, but the general framework involves a dependent variable such as
inflation (or the change in inflation) and explanatory variables including lags
of inflation, the unemployment rate and other predictors.
Recursive,regression-based methods, have had some success. However, three
issues arise when using such methods.
38Gary K. and Dimitris
K.(2009); Forecasting Inflation Using Dynamic Model Averaging,
University of Strathclyde, June 2009
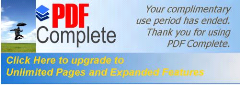
edictors can change over time. For instance, it is commonly
thought that the slope of the Phillips curve has changed over time. If so, the
coefficients on the predictors that determine this slope will be changing
(Stock and Watson, 1996).
Second, the number of potential predictors can be large. For
instance, Groen, Paap and Ravazzolo (2008) consider ten predictors. Researchers
working with factor models such as Stock and Watson (1999) typically have many
more than this. The existence of so many predictors can result in a huge number
of models.
Third, the model relevant for forecasting can potentially
change over time. For instance, the set of predictors for inflation may have
been different in the 1970s than now or some variables may predict well in
recessions but not in expansions. This kind of issue further complicates an
already difficult econometric exercise39.
Among other things, we describe the key differences with
respect to the earlier generation of macro models. In doing so, we highlight
the insights for policy that these new frameworks have to offer. In particular,
we will emphasize two key implications of these new frameworks.
1.4.2.1 Monetary transmission
Monetary transmission depends critically on private sector
expectations of the future path of the central bank's policy instrument, the
short-term interest rate. Ever since the rational expectations revolution, it
has been well understood that the effects of monetary policy depend on private
sector expectations. This early literature, however, typically studied how
expectations formation influenced the effect of a contemporaneous shift in the
money supply on real versus nominal variables (for example, Fischer, 1977;
Taylor, 1980).
In this regard, the new literature differs in two important
ways. First, as we discuss below, it recognizes that central banks typically
employ a short-term interest rate as the policy instrument. Second, within
the model, expectations of the future
39 Stock, J. and Watson M., 1999. Forecasting
inflation: Journal of Monetary Economics 44, 293-335.
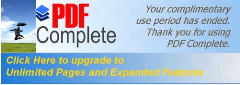
er the structural equations, since these aggregate
king decisions by individual households and firms. As a
consequence, the current values of aggregate output and inflation depend not
only on the central bank's current choice of the short-term interest rate, but
also on the anticipated future path of this instrument. The practical
implication is that how well the central bank is able to manage private sector
expectations about its future policy settings has important consequences for
its overall effectiveness. Put differently, in these paradigms the policy
process is as much, if not more, about communicating the future intentions of
policy in a transparent way, as it is about choosing the current policy
instrument. In this respect, these models provide a clear rationale for the
movement toward greater transparency in intentions that central banks around
the globe appear to be pursuing.
1.4.2.2. Flexible price equilibrium
The natural (flexible price equilibrium) values of both output
and the real interest rate provide important reference points for monetary
policy and may fluctuate considerably. While nominal rigidities are introduced
in these new models in a more rigorous manner than was done previously, it
remains true that one can define natural values for output and the real
interest rate that would arise in equilibrium if these frictions were absent.
These natural values provide important benchmarks, in part because they reflect
the (constrained) efficient level of economic activity and also in part because
monetary policy cannot create persistent departures from the natural values
without inducing either inflationary or deflationary pressures. Within
traditional frameworks, the natural levels of output and the real interest rate
are typically modeled as smoothed trends. Within the new frameworks they are
modelled explicitly.
This book has two broad goals. The first goal is to present
econometric evidence on which type of monetary policy rule is likely to be both
efficient and robust when used as a guideline for the conduct of monetary
policy in Rwanda. The second goal is to answer several current monetary policy
questions such as the effects of uncertainty about potential GDP growth or the
role of the inflation rate in the setting of interest rates that are most
naturally addressed within a framework of monetary policy rules.

ELLING AND CONCEPTUAL FRAMEWORK
A main objective of this chapter is to find the relevant long-
run relationships of inflation and economics variables which driver the Rwanda
inflation and to examine whether we can come up with a reasonable inflation
function by imposing these relationships as equilibrium corrections terms. This
chapter will help to answer the first of assumption which state as; inflation
is in Rwanda a monetary phenomenon? And what are the drivers it in Rwanda?
2.1. Rwanda's inflation experience
Inflation in Rwanda over the past fifteen years has been
mainly influenced by the excess money supply and the nature of import
arrangement and the country's openness. Over these fifteen years ago the
evolution of inflation has been notably similar to that in developed countries
and some in developing countries in the region.
Figure 1: Evolution of Inflation in Rwanda
IPC
2.0 1.8 1.6 1.4 1.2 1.0 0.8 0.6
|
|
|
1996 1998 2000 2002 2004 2006 2008
|
Source: Author plotting (see appendix 2)
On the right side (axis) there evolution in year starting by
1995 until 2009 and on the left hand (absisse) are rate of increase by
quarterly basis as we see on the figure 1 since the 1995 the inflation rise
with a constant rate of 0.3% but during 2006 the rate change 0.6% which is high
movement of change until 2009 this was due to first of all the increase in
salaries in 2006 and 2008 the oil shocks.
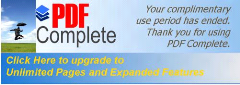
in Rwanda started to rise in the middle of 2007 8 as result of
the oils price shocks. However the
rise observed in mid 1998 was due to genocide event which hit all
economics sector and kill around one million of Rwandan people and
demonetization process which took place during that period.
Figure 2: Evolution of money supply and CPI
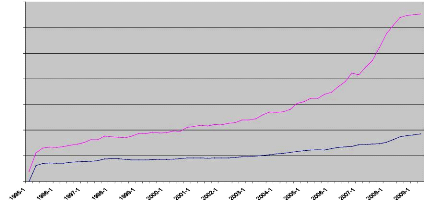
700.00
600.00
500.00
400.00
300.00
200.00
100.00
0.00
ipc M2
Source: Author plotting (data see appendix 2)
On the right hand the evolution in year and on the left hand
the increase of money supply. From 1995 the money supply was tie to economic
activities and as the money supply increased, the inflation follow the speed of
money supply until 2001 but after this period the CPI slope (red on up) become
more positive compare to slope of money supply this mean that there other
factor which come into force to explain the increase of price level among those
for example is the decrease of national output and lack of food in many area
such as Bugesera, and others.
The close correspondence between domestic and foreign
inflation points to the importance of foreign factors (the role of import
arrangement) in underpinning Rwanda major inflationary episodes.
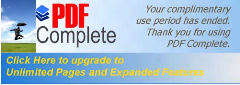
y and a variety of theoretical models give the result that for
a small country; foreign inflation will be fully imported in the long run under
a regime of fixed exchange rate. In effect, a small country with a fixed
exchange rate has very little choice but to accommodate foreign shocks to
price. Although since the Central bank hasn't this regime in Rwanda its use a
range of tools which may ameliorate the effects of foreign price shocks the
picture in Rwanda as in most other developing countries with similar
institutions structures is of the shocks originating in the balance of payments
and impacting through the exchange mechanism. With well over half of Rwanda
goods and services imported there remains a close correspondence between
foreign and domestic prices (see Figure 3).
Figure 3: Evolution of import prices and
domestic prices
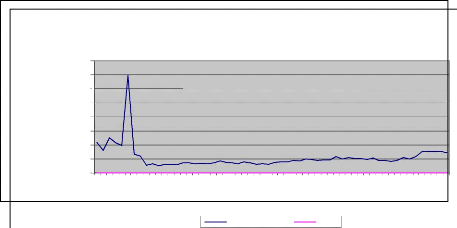
T
o
some in thousand
4000000
2500000
2000000
3500000
3000000
1500000
1000000
500000
1995-1 1996-1 1997-1 1998-1 1999-1 2000-1 2001-1 2002-1 2003-1
2004-1 2005-1 2006-1 2007-1 2008-1 2009-1
0
I lower the
import price IPC
period in years
port price
price. Since 1995 until 1996 the quantity imported was very high
and import price also was very big but at the same time the domestic price was
not very high because at this period the most commodities imported was not for
consumption. There were dominated by services and low materials to build and
medical stuffs. After the 1998 the situation change first of all by lack of
food because there was insecurity in whole country this impel people to
cultivate and making there daily activities the high level of price was due of
food products. Since the 2002 the speed in import price was is
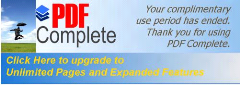
e level on domestic market.
2.1.2 Domestic factors
Its have been seen foreign factors have played a dominant
role, domestic factors also appear to have underpinned inflation over much of
the period and have been particularly important during the keys period. The
nominal wage grew at an average annual rate of over six percent over the past
fifteen years while productivity grew on average of two percent. The result was
sustained growth in nominal unit labor cost which effectively put a floor under
domestic inflation( see Figure4) sharp increase in real wages particularly
during the 2006 appear to have intensified price pressure at the time.
Figure4: Evolution of nominal wage and CPI
UUC
96 98 00 02 04 06 08
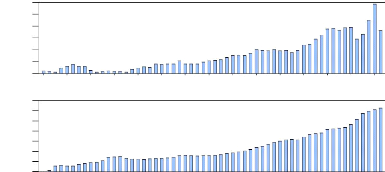
.018
.016
.014
.012
.010
.008
.006
2.0
0.8
0.6
1.8
1.6
1.4
1.2
1.0
96 98 00 02 04 06 08
IPC
Source: Author plotting (data appendix1)
One the right hand is the evolution in year and left
hand the rate of change in nominal wage and the consumer price index for
domestic goods. While broad movements in prices appear to have been largely
caused by import prices and domestic labour costs, there also appears to have
been a correlation with the cyclical pattern of output. Domestic business cycle
fluctuations often reflect a misalignment of demand and the productive capacity
of the economy. Excess demand is likely to generate price pressures in factor
and product markets (see Figure 5)
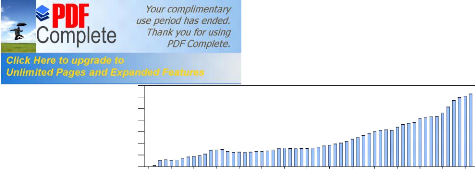
nflation
IIC
2.0
1.8
1.6
1.4
1.2
1.0
0.8
0.6
96 98 00 02 04 06 08
IIBC
6E-.-12 5E-.-12 4E-.-12 3E-.-12 2E-.-12 1E-.-12 0E-.-00
|
|
|
96 98 00 02 04 06 08
|
Source: Author plotting (data appendix1) 1
The figure above show the correlation between the increase in
out put on the right hand the two year evolution and the left hand the rate of
price level increased the expectation here is that the increase of price was
not due to increase of output but the output seems to be positive by the way
the level of domestic demand was not compensated by domestic production this
explain the need of import which was observed above.
Macroeconomic theory suggests different way to explain the
problem of inflation. The basic concept to be considered is the models
suggested by Brouwer and Neil R.Ericsson which reconciles the effects of
«demand-pull» and «cost -push» inflation theory.
2.2. Conceptual framework
Inflation is thought to be an outcome of various economic
factors. In Rwanda context we choice the factors from supply side that come
from cost-push or mark up relationships; the demand side factors that may cause
the demand pull inflation; monetary factors ; and foreign factors like exchange
rate effects. In order to capture the various determinants of inflation we will
combine the methodology developed in Brouwer and Neil R. Ericsson (1998) and
Juselius(1992). The mark up model has a
resence in economics generally; see Duesenberry 93).
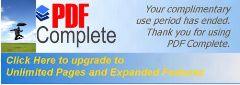
2.2.1. LONG- RUN RELATIONSHIPS 2.2.1.1. Mark
up
We Investigate a mark up relationships following Brouwer and
Ericsson(1998) for Australia inflation. In the long-run the domestic general
price level in Rwanda is a mark up(cost- push) characterized by unit labor cost
, import prices and oil prices index which we will find by segmenting variables
a priori based on some sense of economic theory. Assuming linear homogeneity
the long-run relation of the domestic consumer price level to its determinants
is;
CPI = log (13) +p*UCL +6* IP +ip*PP (1)
Where CPI is consumer price index, UCL is the unit labor cost
of out put, IP is import price in domestic currency, PP is petroleum price
index in national currency, 3-1 correspond to a mark up .the
equation assumes that linear homogeneity hold in long-run. The value of
3-1 is the retail mark up over cost and both the mark up and
cost may vary over the cycle.
In formula, (1) is express in log- linear form:
cpi = log (13)
+p*ulc +6* ip
+(p*pp (2)
Where logarithms of variables are denoted by italic letters
the log- linear form is used in the error correction model below. Linear
homogeneity implies the following testable hypothesis.
p+ 6+ (p=1 (3)
This is unit homogeneity in all prices. Under that assumption,
(2) can be rewritten as
à = log (13) +p (ulc-cpi) +6 (ip-cpi) +cp(pp-cpi) (4)
The equation (4) links real prices in the labour, foreign
goods(import prices) and oil prices index using this representation its will
allow as to interpret the empirical error
|
multiple markets influencing prices; Juselius (1992)
|
2.2.1.2 Money supply determinants
As we already define the mark up, we turn to monetary
determinant of inflation In order to measure the excess money that eventually
leads to inflationary pressures, we need to examine the long run relationship
between broad money supply(M2), CPI inflation, Nominal GDP, exchange rate
expressed in USD, landing interest rate in banking system. The estimated VAR
corresponds to Juselius (1992) and Sekine (2001).? The functional
structure of their model is given as;
Money supply (M2) = F(nwr, ngdp, cpi, tdc, echusd ) 5)
Changes in the price level (CPI) are modeled
as being positively related to the changes in broard money stock (m2),
exchange rate expressed in USA dollar (echusd),
lending rate (tdc), domestic wage rate expressed as percentage changes for
salaries (nwr), and negatively related to changes in
productivity expressed as nominal GDP (ngdp)40.
Some authors have argued that in an open economy, and
especially in a developing country, the money demand equation needs to be
augmented with the exchange rate41. That is why; our excess money
supply function above has the following functional form;
M2= a + ngdp +nwr +cpi+ tdc+ echusd +vt (6)
Where nwr is the nominal wage
constucted and echusd is the nominal exchange rate
measured in terms of RWF in USA dollar. The interest rate and the exchange rate
are in levels. If long-run money supply is stable, then the error term in the
above equation will be stationary. The long-run equilibrium level of board
money can be estimated by a long-run cointegrating relationship to see if its
has cointegrating vector.
40 BROWER, G.and ERICSSON
,N.R.(1995), Modeling inflation in Australia, Reserve Bank of Australia,
Research discussion Paper No 9510, Page 21
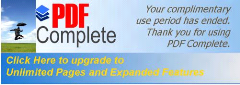
This section describes the data available and considers some
of their basic properties. Our sample period runs through the first quarterly
of 1995 to second quarterly of 2009. There is single indicator of price
movements in Rwanda Two different price indices are published in Rwanda: the
Consumer Price Index (CPI), and Producer Price Index (PPI). The movements in
primary articles are dominated by supply shocks, and the prices of fuel and
energy are administered. The central banks of Rwanda focus on core inflation
that excludes food and energy42. To take care of the issue of supply
shocks and administered price controls, we focus on the import price and oil
price index calculated by Laspeyres formula see appendix 1. We use the data
from BNR to evolution of money supply the quarterly data are available. Since
real GDP data are available only at an annual frequency, we use Eviews to get
the estimated quarterly data for nominal GDP. The interest rates in Rwanda were
administered prior to financial liberalization this imposes a problem in the
selection of an appropriate interest rate as an opportunity cost of holding
money. Moosa (1992) uses the call money rate as a measure of the opportunity
cost of holding money. The problem with using call money rate as an opportunity
cost of holding real money balance is that it is highly volatile and is
affected more by the weekly funding demands of commercial banks43.
Depending on availability of data we use the bank rate as opportunity cost of
holding real money balance (in banking system). The bank rate is the rate at
which the NBR lends liquidity to banks, broad money growth, and exchange rate
has been obtained from the NBR website unit labor cost was calculated by having
the gross salaries for all employees in central administration, local
administration, the voluntaries insured, public institutions, government
projects, mixed sector and private sector this sum was corrected by adding 5%
of contribution for employers as social contribution to pension and divided it
by nominal GDP (see appendix1).
41 Morling, S . (1997),
Modeling inflation in Fiji, Working paper , Reserve Bank of Fiji, No 23,
page 13
42 See economic Bulletin of
BNR of second quarterly 2008
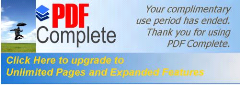
els
2.3.1. Mark up
A situation is said to be an inflationary situation when,
either the prices of goods and services or money supply rise. Friedman
mentioned inflation as `always a monetary phenomenon'. But most of the
economists today, do not agree that money supply alone is the cause of
inflation. So other factors which drive inflation in developing countries could
be expressed as mark up.
2.3.1.1 Integration
Before modeling the CPI, it is useful to determine the orders
of integration for the variables considered. Table 1 lists fourth-order
augmented Dickey- Fuller (1981) (ADF) statistics for the CPI, unit labour
costs, import prices, and petrol prices index. Under standard optimizing
behaviour, the mark-up itself should be stationary. The deviation from unity of
the estimated largest root appears in parentheses below each Dickey-Fuller
statistic: this deviation should be approximately zero if the series has a unit
root. Unit root tests are given for the original variables (all in logs), for
their changes, and for the changes of the changes. This permits testing whether
a given series is I(0), I(1),or I(2), albeit in a pair wise fashion for
adjacent orders of integration44. Where k is the number of lags on
the dependent variable, augmented Dickey-Fuller statistic ADF, and (in
parentheses) the estimated coefficient on the lagged variable. That coefficient
should be zero under the null hypothesis that is I(1). For a null order of I(2)
(I(3)), the same pairs of values are reported, but from regressions where
replace in the equation above. Thus, these ADF statistics are testing a null
hypothesis of a unit root in {1% and 5%) against an alternative of a stationary
root in {1% and 5%). The sample is 1995(1)-2009(2) for all.
43 Mishkin, F. S. (1992), Is
the Fisher effect for real: A reexamination of the relationship between
Inflation and Interest rates , Journal of Monetary Economics 30: Page 185-
215
44 For k~0
if the notation I (k) indicates that a variable must
be differenced k times to make it stationary. That is, if CPI
is I(k), then {dcpi) is I(1).
Null order
|
cpi
|
ulc
|
ip
|
opi
|
I(0)
|
1.30**
|
-3.31*
|
-5.27*
|
-2.67**
|
|
( o.o4)
|
(0.32)
|
(-0.67)
|
(-0.23)
|
I(1)
|
-4.23
|
-4.12
|
-10.11
|
-5.19
|
|
(0.12)
|
(0.58)
|
(0.18)
|
(0.17)
|
I(2)
|
-4.90
|
-5.11
|
-17.66
|
-6.87
|
|
|
(0.11)
|
(0.11)
|
(0.16)
|
(0.25)
|

g for a Unit Root in all Time
Series45
Empirically, all variables appear to be integrated of order
one. Unit labour costs,CPI and petrol prices appear to be I(1), whereas the
import prices appear to be also I(1) if inferences are made on the
Dickey-Fuller statistics alone. Thus, all four price series are treated below
as if they are I(1), while recognizing that some caveats may apply.
Specifically, it may be valuable to investigate the cointegration properties of
the series.
2.3.1.2. Cointegration
Cointegration analysis helps clarify the long-run
relationships between integrated variables. Johansen's (1988, 1991) procedure
is maximum likelihood for finite-order vector auto regressions (VARs) and is
easily calculated for such systems, so it is used here. Empirically, the lag
order of the VARs is not known a priori, so some testing of lag order may be
fruitful in order to ensure reasonable power of the Johansen procedure.
Beginning with a fourth-order VAR in CPI,ULC,IP and OPI that
includes a constant term (see appendix 7) shows that it is statistically
acceptable to simplify to a first-order VAR. Table 2 reports the standard
statistics and estimates for Johansen's procedure applied to this first-order
VAR. The maximal eigenvalue and trace eigenvalue statistics (Amax and Atrace)
strongly reject the null of no cointegration in
45Here and elsewhere in this
paper, asterisks * and ** denote rejection at the 5% and 1% critical values.
The critical values for this table are calculated from MacKinnon (1996). The
values in parentheses are the estimated coefficient on the lagged variables.
That coefficient should be zero under the null hypothesis that k
is I(1). For a null order of I(2) (I(3)), the same pairs of values are
reported,
|
ng relationship, and little evidence exists for more
|
Table 2: Cointegration analysis in the mark-up
model
Ho=rank=p
|
A
|
A max
|
95% CV
|
A trace
|
95% CV
|
p=0
|
0.0174
|
27.43
|
24.15
|
47.30
|
40.17
|
p~1
|
0.0082
|
0.047
|
3.76
|
0.047
|
3.76
|
In Johansen cointegration procedure, the Amax statistic tests
the null hypothesis that the cointegration rank is equal to p, against the
alternative of p+1 cointegration vectors. The Atrace statistic tests the null
of cointegration of rank p, against a general alternative. In both tests if a
computed test statistic exceeds the critical value, the null is rejected. In
the present case, the results indicate presence of cointegration between oil
prices and CPI at 1 and 5 percent critical levels. The values of the Amax and
Atrace statistics are such that the null hypothesis of no cointegration can be
soundly rejected. The Johansen Atrace statistics supports existence of one
cointegrating vector, while various tests on residuals properties imply
congruent VAR.
The estimated coefficient on oil price shows that if the world
oil price increases by 1 percent, the CPI will rise by 0.277 percent. This is
small number but it is statistically significant. Speed of adjustment
coefficients that measure the degree to which the variable in question responds
to the deviation from the long-run equilibrium relationship, indicate weak
exogeneity of oil prices. Weak exogeneity stands for the fact that a given
variable does not respond to the discrepancy from the long-run equilibrium
relationship.
The mark up is views to be caused by three factors in Rwanda as
using OLS the resultants are as follows; and the model proved to be
satisfactory.
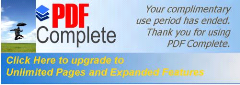
Method: Least Squares
Date: 11/04/09 Time: 10:21
Sample (adjusted): 1995Q2 2009Q2
Included observations: 57 after adjustments
Variable
|
Coefficie
nt Std. Error
|
t-Statistic
|
Prob.
|
LULC
|
0.573163
|
0.064287
|
8.915645
|
0.0000
|
LIP
|
0.178627
|
0.027577
|
6.477289
|
0.0000
|
LOPC
|
0.277785
|
0.096332
|
2.883627
|
0.0056
|
R-squared
|
0.658918
|
Mean dependent var
|
|
0.027192
|
Adjusted R-squared
|
0.646285
|
S.D. dependent var
|
|
0.273971
|
S.E. of regression
|
0.162941
|
Akaike info criterion
|
|
-0.739659
|
Sum squared resid
|
1.433691
|
Schwarz criterion
|
|
-0.632130
|
Log likelihood
|
24.08028
|
Hannan-Quinn criter.
|
|
-0.697869
|
Durbin-Watson stat
|
0.340532
|
|
|
|
Assuming one cointegrating vector and linear homogeneity, the
derived long run markup relationship becomes:
From the above empirical model of Inflation mark -up the
estimated equation using OLS methods is as follows; the low Durbin Watson is
due to the big number of variables estimated as founded by Mankiw in His book
Economic Principles (1998).
LIPC = 0.573*LULC +
0.178626*LIP + 0.27778*LOPC (7)
For the obove estimated equation the mark up is;
Lmarkup =LCPI -0.57* LULC - 0.178*LIP-0.27 LOPC
The share of unit labor cost in total unit cost (0.57) seems
reasonable considering that Rwanda economy is highly dominated by service
sector. Share of unit labour costs in total unit cost is higher than in
Australia (- 0.43), but much lower than Japan
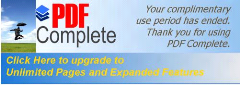
on, 1998; Sekine, 2002). Relatively small share of
ue to high import dependency of Rwanda economy and with a
large value of oil price mean how much Rwanda spend on pretroleum product due
to oil shocks and fluctuation.
2.3.2 Excess money supply
The next long -run relationship is monetary conditions.
Beginning with Friedman and Schwartz (1963), many researchers have examined
whether inflation is a monetary phenomenon. For instance advocates of the p
star approach (Hellman, Porter and Small, 1991) examine inflationary effects of
excess money in terms of difference between actual money velocity and its
long-run value (together with the output gap). Also Juselius (1992) finds
excess money in terms of cointegartion vector which represents the long-run
money demand as one source of inflation. In this case following Juselius we
have a vector of five variables: price (CPI), money (M2), income (nominal GDP),
exchange rate and interest rates ( in banking system). We estimated the VAR
corresponds to Juselius (1992) and Sekine (2001).Table 3, Table 4 and Table 5
summarize residual properties and a system cointegrating analysis of the VAR.
The Johansen test supports existence of one cointegrating vector. There are
indications of autocorrelation in the residuals (indicated by AR test), but
otherwise the VAR seems satisfactory. The occurrence of autocorrelation can be
attributed to estimated GDP series. All diagnostic tests are satisfactory.
Table 4: Properties of VAR residuals
tests
|
LM2
|
lpibc
|
ltcusd
|
ltdc
|
lipc
|
normality
|
1.88
|
I68
|
2.77
|
13.30
|
7.46
|
ARCH test
|
0.24
|
0.38
|
0.25
|
0.162
|
0.182
|
AR
|
2.43
|
6.78
|
3.10
|
1.92
|
2.36
|
Jarque - Bera
|
1.88
|
12.00
|
174.00
|
2.72
|
14. 93
|
Chi- Sq
|
0.78
|
7.73
|
6.76
|
0.01
|
1.82
|
Lm2
|
0.004
|
0.023
|
0.132
|
0.25
|
0.12
|
Lipc
|
0.003
|
0.001
|
-0.0015
|
-0.0037
|
0.0029
|
lpibc
|
0.016
|
0.0129
|
-0.008
|
0.0123
|
0.0081
|
ltcusd
|
0.005
|
0.062
|
-0.0077
|
-0.0011
|
-0.0018
|
ltdc
|
-0.024
|
0.0031
|
-0.036
|
0.0053
|
-0.0103
|
|
t coefficients
|
Table 6: Properties of cointegration vector
Eigenvalues
|
0.361
|
0.305
|
0.215
|
0.119
|
0.081
|
Hypotheses
|
r=0
|
r~1
|
r~2
|
r~3
|
r~4
|
Amax
|
24.21
|
19.65
|
13.11
|
6.89
|
4.58
|
Atrace
|
68.46
|
44.25
|
24.59
|
11.48
|
4.58
|
Table 5 reports, in bold, the eigenvalues statistically
different from zero on the basis of the trace and the maximum eigenvalue tests.
The critical values are taken from Mackinnon- Haug Michelis(1999). The trace
test points out the existence of two long-run relationships. The maximum
eigenvalue test suggests a cointegration rank equal to three (at the 10%
significance level), while its version corrected for the number of degrees of
freedom indicates a rank equal to two (at the 5% significance level). According
to Johansen (1992) the maximum eigenvalue test may produce an incoherent
testing strategy, therefore the trace test results are preferred and the
cointegration rank r is set equal to two.
LM2 = 0.093*LPIBC +
0.308*LTCUSD _ 0.174*LTDC +
1.75*LIPC
From above resultants;
Lexcess_money = LM2-0.093*LPIBC
- 0.308*LTCUSD + 0.174*LTDC -
1.75*LIPC
This model will be useful to calculating the long run
relationship of inflation and the variables coefficients are satisfactory and
prevailing the insight on inflation behavior.
2.4 THE LONG RUN MODEL OF INFLATION
Various economists have attempted to empirically analyze the
issues outlined in the previous section. Earlier studies, such as Bourne and
Persaud (1977) and Holder and Worrell (1985), emphasized the role of
structural influences and cost push
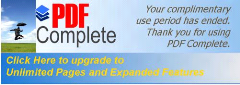
e found that monetary disequilibrium and exchange
xplaining the behaviour of prices in the Jamaican
economy46. The link between the money stock and inflation occurs via
a monetary transmission process whereby the amount of money economic agents
desire to hold is less than the available money stock. Assuming a stable demand
for money, this serves to reduce the value of money (in terms of goods) thus
increasing the price level.
We estimated a model similar to the Harberger model using
ordinary least squares. The results using quarterly are;
DLIPC =
0.104*LEXCESS_MONEY(-1) + 0.062*LMARKUP +
0.099*DLM2 - 0.0327*DLOPC +
0.20*DLPIBC + 0.21*DLTCUSD(-1) -
0.0034*DLTDC(-1) + 0.184*DLULC -
0.0067*DLIP
With R-squared= 0.42, Schwarz criterio=-4,38, F-statist= 1,23
,DW= 1.25, Chow = 1.11, Normality test= 1,32 and ARCH= 0.38 sigma= 0.96
We estimated a general model in which we regressed Dlcpi
(difference in logarithm of consumer price index) on the above mentioned long
run (and structural) relationships markup,Lexcess_moneyt-1, and Dlpibc, and
short run dynamics Dltcusd(-1), Dlulc, Dlip, DLm2 and DLtdc Our sample goes
from 1995Q1 until 2009Q2 and for that sample period our unrestricted general
model yields sigma = 0.96 percent for 9 regressors and 56 observations (Schwarz
criterion = -4.38). Next step was eliminating insignificant terms from the
model by allowing the log variables stationary
The procedure followed is general to simple approach
LIPC = 0.016*LIP(-1) +
0.81*LIPC(-1) + 0.121*LM2 -
0.047*LOPC - 0.025*LPIBC(- 1) +
0.020*DLTDC(-1) _ 0.077*DLULC(-1) +
0.406*DLTCUSD
46 Wayne Robinson (1998) :
Forecasting inflation using VAR analysis, Bank of Jamaica
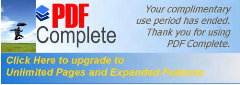
26 F-statistic= 1060,04 , Jarque- bera= 9.14,ARCH
est with also high probability over 5%. The model proved its
adequacy in terms of various diagnostic tests and also it encompasses the
unrestricted general model. For more information see appendix 6
The contemporaneous money stock, import price, oil price, and
exchange rate had expected signs and were very significant. The results suggest
that the money supply, import prices, mainly the oil prices fluctuations and
exchange rate changes had the largest impact on price changes. Using the
quarterly data the model derived is;
LIPC = 0.029 + 0.016* LIP t-1 +
0.81*LIPC t-1 + 0.121*LM2 -0.047*
LOPC- 0.026* LPIBC t-1 +0.020*
DLTDC t-1 - 0.077*DLULC t-1
+0.40*DLTCUSD
Table 7: Results for long run inflation
model
Dependent Variable: LIPC
Method: Least Squares
Date: 11/06/09 Time: 09:41
Sample (adjusted): 1995Q3 2009Q2
Included observations: 56 after adjustments
Variable
|
Coefficien
t
|
Std. Error
|
t-Statistic
|
Prob.
|
LIP(-1)
|
0.016072
|
0.008212
|
1.957190
|
0.0563
|
LIPC(-1)
|
0.815484
|
0.079861
|
10.21133
|
0.0000
|
LM2
|
0.121700
|
0.042441
|
2.867535
|
0.0062
|
LOPC
|
-0.047478
|
0.018775
|
-2.528729
|
0.0149
|
LPIBC(-1)
|
-0.026858
|
0.037109
|
-0.723760
|
0.4728
|
DLTDC(-1)
|
0.020468
|
0.025706
|
0.796245
|
0.4299
|
DLULC(-1)
|
-0.077711
|
0.061559
|
-1.262391
|
0.2130
|
DLTCUSD
|
0.406268
|
0.137711
|
2.950146
|
0.0049
|
C
|
0.029796
|
1.020664
|
0.029193
|
0.9768
|
R-squared
|
0.994488
|
Mean dependent var
|
|
0.036243
|
Adjusted R-squared
|
0.993550
|
S.D. dependent var
|
|
0.267714
|
S.E. of regression
|
0.021500
|
Akaike info criterion
|
|
-4.695275
|
Sum squared resid 0.021726
|
Schwarz criterion
|
|
-4.369772
|
Log likelihood
|
140.4677
|
Hannan-Quinn criter.
|
|
-4.569078
|
F-statistic
|
1060.045
|
Durbin-Watson stat
|
|
1.268751
|
Prob(F-statistic)
|
0.000000
|
|
|
|
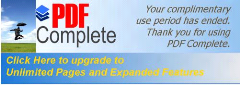
ighly influenced by lagged inflation, money supply
ese results also highlight the significant role of oil prices
and import prices, starting from the hypothesis of the Quantity Theory,
estimated the relationship between money supply and prices in Rwanda between
1995 and 2009. The changes in prices were examined as a function of changes in
the money supply (M2), previous price changes and changes in the exchange rate.
Using quarterly data, the estimated model most preferred was;
LIPC = 0.029 + 0.016* LIP t-1 +
0.81*LIPC t-1 + 0.121*LM2 -0.047*
LOPC- 0.026* LPIBC t-1 +0.020*
DLTDC t-1 - 0.077*DLULC t-1 +0.40*DLTCUSD
2.4.1 Interpretation of coefficients
R- squared= 99.44% this mean that the 99.44 % fluctuations of
prices are explained by last inflation, money supply, exchange rate, import
price and oil shocks.
Most coefficients are statistically significant only the
coefficients of LPIBC, DLTDC, and DLULC have a high probabilities the reason
for that is because the PIBC was estimated for quarterly the ULC was calculated
using NSSF data. This could lead to estimators bias.
2.4.2 Classical Tests
-The T- Student of LIP t-1, LIPC t-1, LM2, LOPC and DLTCUSD have
the significant influence on inflation.
-Homocedasticity test of correlation of errors
Ho= the model is homocedastic H1= the model is heteroscedastic
Table 8: Heteroskedasticity test
White heteroskedasticity test: No cross terms
F- statistic
|
1.093951
|
Probability
|
0.3842
|
Obs*R- squared
|
8.79061
|
Probability
|
0.3603
|
White heteroskedasticity test: cross terms
|
F- statistic
|
2.034542
|
Probability
|
0.0508
|
Obs*R- squared
|
44.46531
|
Probability
|
0.1572
|
With the two option test above we accept the first assumption
that there is homoskedasticity because the probabilities are high than 5%
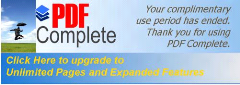
H1= errors correlated
We dispose here the sample n= 56 observations. The number of
real explanatory variables is K=8 on Durbin Watson table at 5% level of
freedom; Dinf=1,39 and Dsup= 1,51 and our DW calculated is =1, 26 this mean
that there is presumably a positive autocorrelation of errors.
We correct the autocorrelation with the Cochrane Orcutt method by
adding the inverted AR root
The DW find after new estimation is= 1.68 and K have been 9 so
Dinf =1,32 and Dsup= 1.58 mean that we presumably resolve the autocorrelation
problems by Cochrone Orcutt method
Ho is accepted no correlation among errors.
Test of Ramsey RESET
The assumptions for this test are as follows; Ho= the
specification of model is good
H1= the model is badly specified
Table 9: Ramsey RESET test
Ramsey RESET TEST
F- statistic
|
1.3055
|
Probability
|
0.2702
|
Log likelihood ratio
|
13.3559
|
Probability
|
0.1002
|
These two probability show that the model specification is good
because the probalitity are higher than 5% mean that we accept Ho.
Figure 5: CUSUM Test
If the cusum curve is out of corridor means that the coefficients
of the models are unstable and curve does not leave the corridor mean that the
coefficients are stable.
CUS UM 5% S ignific anc e
|
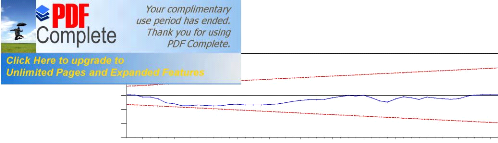
0
- 10
- 20
- 30
98 99 00 01 02 03 04 05 06 07 08
Figure 6: CUSUM squared test
This test allow to detect the punctual instability
1.4 1.2 1.0 0.8 0.6 0.4 0.2 0.0
- 0.2
- 0.4
|
|
|
98 99 00 01 02 03 04 05 06 07 08
|
|
CUSUM of Squares 5% Significance
|
|
With the cusum squared line which lie between the corridor line
mean that the model is stable in all coefficients
Partial conclusion
From this we conclude that rise of price is influenced by
changes in the money supply, but not directly as the Quantity Theory suggests.
Monetary changes affect inflation indirectly because of the prevalence of
mark-up pricing. This also provides the channel for the impact of exchange rate
adjustments (i.e. changes in the exchange rate affect variable cost) and lagged
prices.
Here taking consideration of Rwanda context the inflation
appears to be driven by both foreign and domestic factors in a manner
consistent with conventional theoretical models and the Rwanda inflation is
not only the monetary phenomenon.
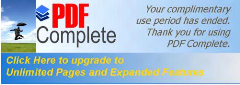
hat monetary policy has a lag effect of `at least' two
e perpetuated by the nature of the stabilization process, the
structure of the economy, the production function, and other institutional
factors. Other factors such as the oil pricing mechanism, import level and
exchange rate, which are captured in the own innovations of the CPI and
exchange rate are also very significant and create very strong inertial
tendencies. Stabilization policies must therefore be cognizant of these
influences that frustrate the stabilization process.
|
TION OF RWANDA USING ECM MODEL
|
|
In the second chapter we have seen the inflation long run
model but Monetary policy-makers face a difficult task when evaluating the
current state of the economy and deciding what actions are needed to achieve
their objectives, such as keeping inflation within a given range. Because long
and variable lags exist between a monetary policy action and its effects on
economic variables, policy-makers need a way to assess whether their actions
are having, or indeed will have, the desired effect.
The model used in this paper is similar to Hendry's original
model in that it estimates a unique and stable long-run cointegrating vector
between quarterly data for cpi, nomimal output, the M2, exchange rate, import
price , oil price index, unite labor cost and a short-term interest rate.
3.2. Error correction model of inflation
The error correction model represents one of remarkable
property which had been demonstrated by Granger (1983). The whole variables
cointegrated could set in form of error correction model where all variable are
stationary and the coefficients could be estimated using the classical
econometric approach without correlation risks.
Here we propose two types of error collection models;
3.2.1 The Hendry model
The model based on an error correction mechanism first
introduced by Sergan (1964) and popularized by Hendry in numerous papers has
enjoyed a revival in popularity in empirical macroeconomics research is
expressed as47;
DLIPC =130+ 131*DLIP(-1)
+132*DLIPC(-1) + 133*DLM2 +134*DLOPC
+ 135*DLPIBC(-1) +136*DLTDC(-1) +
137*DLULC(-1) +138*DLTCUSD
+139*LIPC(-2)+ 1310*LM2(-1)+
1311*LOPC(-1)+1312*LPIBC(-2)+ 1313*
DLTDC(-2)+ 1314*DLULC(-2)+
15*DLTCUSD(- 1)+ Vt
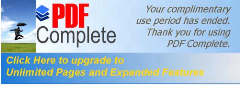
and Vt is error terms ,the coefficients 130 138
ic and the â10 â15 characterized the long run
equilibrium the 139 is the error correction coefficient which
could be under one unity and has a negative sign. The error correction
coefficient indicate the speed of adjustment of explained variable IPC by
returned on equilibrium in long run follow the shock. The 130 represent the
model constant.
Using the OLS the resultants coefficients are as follow;
DLIPC = -0.63 +
0.0089*DLIP(-1) + 0.207*DLIPC(-1) +
0.0348*DLM2 - 0.015*DLOPC +
0.0277*DLPIBC(-1) - 0.0116*DLTDC(-1) -
0.018*DLULC(-1) + 0.183*DLTCUSD
+0.010*LIP(-2) -0.224*LIPC(-2)
+0.111*LM2(-1) _0.027*LOPC(-1)
--0.10*DLPIBC(-2) - 0.0279*DLTDC(-2)
--0.082*DLULC(-2) - 0.21*DLTCUSD(-1)
The interpretation of 139 coefficients (restoring force to
balance) has a negative sign as predicted. We find that the coefficients
associated with the restoring force is - 0.224 and clearly significant
different from zero at level of confidence equal to 5% (his T- student is
greater than 1.96 in absolute value) mean that there is therefore a mechanism
for error correction; in long run the disequilibrium between IPC and all others
explanatory variables is compensated so that all series have similar evolution.
B9 represent the speed at which any imbalance between desired levels and the
effective level of inflation is eliminated in the year following shock. We get
adjusted 22.4% of the imbalance between desired and actual level of
inflation.
3.2.1.1 Long run and short run elasticity
The short run elasticity is represented by 131 138
coefficients; if the level of
import increase by 10% then the inflation rise by 0.089%, if
the money supply increase by 10% the inflation rise by 0.348%, if the oil price
increase by 10% the rise in prices will be 0.15%,if the GDP rise by 10% the
prices level reduce the 0.277%, if interest rate rise the one point the prices
level rise the 0.116% and if the Rwanda currency appreciated the 10% compare to
foreign currency the prices level will rises the 1.83%.
47 James P. Le sage@ (1990)
; A Comparison of the Forecasting Ability of ECM and VAR Models, page
23
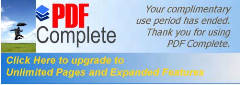
ted by 1310 1315 coefficients;
0.111
Money supply elasticity = = = 0.495
B9 0.224
this means that in long run if the money supply rise by 10 % the
level of price will rise by 4.95%.
1315 0.210
Exchange rate elasticity = = = 0.937
B9 0.224
If the Rwanda currency appreciate by 10% the level of price will
rises by 9.37% in whole economy.
B13 0.0279
Interest rate elasticity in long run= = = 0.124
B9 0.224
This mean that as soon as the interest rate increases by one
point the level of price
rise by 1.24% in long run.
B14 0.082
Wage elasticity = = = 0.366
B9 0.224
The wage is one of the significant variables which cause the
price fluctuations in
economy as soon as the nominal rise the 10% the price level pass
at 3.66% in long run.
B12 0.027
Oil price elasticity = = = 0.12
139 0.224
Oil shock have also impact on price fluctuations in long run,
the rise of 10% on oil
prices rise 1.2% of prices level in general.
3.2.1.2 Significativity of error correction
model
T- student all variables are not significant
F- statistic show that is small mean that the model is not fit
as well
R- squared is also small which mean than model is not good.
Jarque - Bera test
With the probability equal to 0.000545 which is lower than 5%
allow as to reject Ho state than the error distribution doesn't follow the
normal law of distribution.
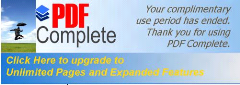
|
|
F- statistic
|
0.576303
|
probability
|
0.8819
|
obs*R-squared
|
10.73988
|
probability
|
0.8252
|
|
The errors of ECM are homoskedatic
ARCH test
Ho= errors are homoskedastic
H1= errors are heteroskedastic
The errors are homoskastic if the probability is higher than 5%
and the errors are heteroskedastic if the probability is lesser than 5%.
Table 11: ARCH test
ARCH heteroskedasticity Test
|
F- statistic
|
0.001992
|
probability
|
0.9646
|
obs*R-squared
|
0.002069
|
probability
|
0.9637
|
|
The errors are homoskedastic
Breusch- Godfrey test
Ho= errors are not correlated
H1= errors are correlated
We accept the no correlation assumption when the probability is
higher than 5%.
Table 12: Breusch -Godfrey Test
Breusch- Godfrey Serial Correlation test
|
F- statistic
|
6.770304
|
probability
|
0.0030
|
obs*R-squared
|
14.43214
|
probability
|
0.0007
|
|
The errors of ECM are correlated the estimated coefficients get
are bias.
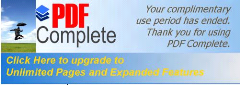
Ramsey RESET test
|
F- statistic
|
3.960093
|
probability
|
0.0090
|
likelihood
|
19.95602
|
probability
|
0.0005
|
|
With the two probability above are less than 5% mean that the
model is badly specified
Figure 7: CUSUM stability test (Brown, Durbin,
Ewans)
20 15 10 5 0 -5
- 10
- 15
- 20
|
|
|
|
CUSUM5% Significance
The Cusum curve lie between corridor lines mean that the ECM is
structurally stable
Figure 8: CUSUM squared stability test
1.4 1.2 1.0 0.8 0.6 0.4 0.2 0.0
- 0.2
- 0.4
|
|
|
99 00 01 02 03 04 05 06 07 08
|
CUSUM of Squares 5% Significance
|
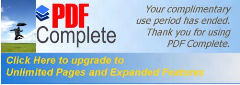
e ECM is instable the 2005-2006 is instable period.
e unadequancy and is instable at certain period so due to lack
of certain dummies variables which could be included to correct it, so we
didn,t have during this estimation so we propose another ECM recommended by
Engle- Granger with two-step.
3.2.2 The ECM with Engle - Granger
The model of Granger enables the long-run equilibrium
relationship and short-run dynamics to be estimated simultaneously particularly
for finites samples, where ignoring dynamics when estimating the long run
parameters can lead to substantial bias48. One of the advantages of
this specification is that it isolates the speed of adjustment parameter C(9),
which indicates how quickly the system returns to equilibrium after a random
shock. The significance of the error correction coefficient is also a test for
cointegration. Kremers, Ericsson and Dolado (1992) have shown this test to be
more powerful than the Dickey-Fuller test applied to the residuals of a static
long-run relationship. Another reparameterisation, the Bewley (1979)
transformation, isolates the long-run or equilibrium parameters and provides
t-statistics on those parameters. Inder (1991) shows these approximately
normally distributed t-statistics are less biased than the Phillips-Hansen
adjusted t-statistics The model is expressed as follow;
DLIPC = C(1) +
C(2)*LEXCESS_MONEY + C(3)*DLMARKUP +
C(4)*RESID01(-1) + C(5)*LTDC(-1) +
C(6)*DLM2(-2) + C(7)*LOPC(-1) +
C(8)*DLTCUSD(-1) + C(9)*DLPIBC(-1) +
C(10)*LULC(-1)
The results for estimated model are as follow;
DLIPC = -0.0199-
0.173*LEXCESS_MONEY + 0.038*DLMARKUP -
0.319*RESID01 (-1) - 0.030*LTDC (-1) +
0.067*DLM2 (-2) + 0.033*LOPC (-1) +
0.062*DLTCUSD (-1) + 0.165*DLPIBC (-1) +
0.019*LULC (-1)
48 Banerjee et al. (1993) and Inder (1994) show that
substantial biases in static OLS estimates of the cointegration parameters can
exist, particularly in finite samples, and the unrestricted error correction
models can produce superior estimates of the cointegrating vector.
e level of priced in Rwanda is negatively related to elated
to mark up and this is a short run correlation
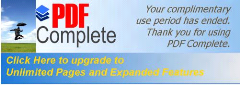
between variables
3.2.2.1 Long-run elasticity and short run
elasticity
The short run elasticity is represented by 134 139 coefficients;
if the level of
money supply grow by 10% then the inflation rise by 0.067%, if
the exchange rate fluctuate by 10% the inflation rise by 0.030%, If the oil
price increase by 10% the inflation rise by 0.033%, if the salaries rise by 10%
the inflation rise by 0.019, if the interest rate grow by one point(mean by
100%) the inflation also rise by 0.062% and if the GDP grow by 10% the
inflation decrease by 0.165% ,this allow as by making sure that the inflation
is also originated from foreign fluctuations.
The long run elasticity is represented by 131 132
coefficients;
131 0.173
Money supply elasticity = = = 0.542
134 0.319
this means that in long run if the money supply rise by 10 % the
level of price will rise by 5.42%.
131 0.038
Exchange rate elasticity = = = 0.119
134 0.319
If the Rwanda if foreign price rise as results of import changes
by 10% the level of price will rises by 1.19 in whole economy.
Before turning to the results, it is necessary to consider the
statistical properties of the model. The model was tested for normality, serial
correlation, autoregressive conditional heteroskedasticity, heteroskedasticity,
specification error and stability. The results, reported in Table 5, suggest
the model is well specified. The diagnostics indicate that the residuals are
normally distributed, homoskedastic and serially uncorrelated and the
parameters appear to be stable.
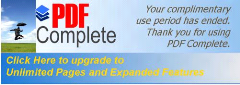
test
|
|
values
|
Probability
|
Jarque-Bera
|
q 2-statistic
|
2.7080
|
0.0980
|
Breusch-Godfrey
|
F-statistic
|
0.213537
|
0.9911
|
Correlation LM test
|
q 2-statistic
|
2.245793
|
0.9870
|
ARCH LM test
|
F- statistic
|
0.008989
|
0.9248
|
|
q 2-statistic
|
0.009327
|
0.9231
|
white
|
F- statistic
|
0.140097
|
0.9982
|
|
q 2-statistic
|
1.494021
|
0.9972
|
|
Chow breakpoint Test(
|
F- statistic
|
1.801709
|
0.0958
|
mid sample)
|
L-R-statistic
|
18.01709
|
0.0547
|
Chow Forecast test
|
F- statistic
|
0.638972
|
0.8506
|
(1995-2009)
|
L-R-statistic
|
57.86670
|
0.0065
|
Ramsey RESET test
|
F- statistic
|
4.049290
|
0.0502
|
|
L-R-statistic
|
4.825140
|
0.0280
|
Notes :**(*) denotes significance at the one (five) per cent
levels. No terms were
|
significant at these levels. LR is a likelihood ratio
statistic.
|
Figure 9: Residuals test
.04 .03 .02 .01 .00
-.01
-.02
-.03
-.04
|
|
98 99 00 01 02 03 04 05 06 07 08
Rec urs ive Residuals #177; 2 S .E .
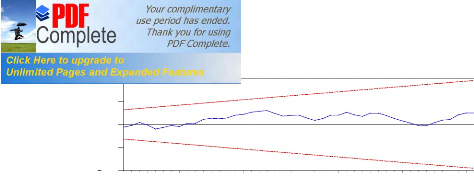
Granger ECM
10
0
-10
20
98 99 00
01 02 03 04 05 06 07 08
Figure 11: Cusum of squared test for Engle- Granger
ECM
1. 4 1. 2 1. 0 0. 8 0. 6 0. 4 0. 2 0. 0 -0. 2 -0. 4
|
|
98 99 00 01 02 03 04 05 06 07 08
CUSUM of S quares 5% Signific ance
|
From the above test results the model proves its adequacy in
term of coefficients and p-values. The results provide strong support for the
conventional ECM model as a description of inflationary processes in Rwanda.
They are consistent with the conventional theory, and with the findings of many
overseas studies. They are also consistent with our understanding of the
institutional structure of the domestic economy. The results suggest that about
three quarters of the long-run movement in prices in Rwanda has been
underpinned by import prices; about one quarter has been driven by domestic
labour costs. The small coefficient on the error correction term points to
protracted periods of disequilibrium for long run and drawn out adjustment
processes, particularly in respect of changes to unit labour costs. In the
interim, domestic demand conditions play an important role. Many indicators are
using to evaluate the quality of the model proved to be used as prediction
model the more used are MAPE (mean absolute percentage error), and the
Inequality coefficient of THEIL, the model with small MAPE is judged
sion and Theil comprised between 0 and 1 indicate r instance (see
figure 13)
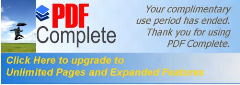
Figure 12: Inflation forecasting criteria
Forecast: DLIPCF
Actual: DLIPC
Forecast sample: 1995Q1 2009Q2 Adjusted sample: 1995Q4 2009Q2
Included observations: 55
Root Mean Squared Error
|
0.012813
|
Mean Absolute Error
|
0.009682
|
Mean Abs. Percent Error
|
93.46577
|
Theil Inequality Coefficient
|
0.250929
|
Bias Proportion
|
0.000000
|
Variance Proportion
|
0.119487
|
Covariance Proportion
|
0.880513
|
.10 .08 .06 .04 .02 .00 -.02 -.04 -.06
|
|
|
1996 1998 2000 2002 2004 2006 2008
|
|
|
DLIPCF #177; 2 S.E.
|
|
The two models seen above have the same characteristics and
don't allow us to make the best forecast because the mean absolute percentage
error is high in two equations above see Figure 6. Instead of using one of them
we extend our understanding by apply the VECM as theoretical suggest. The main
reason for estimating a VECM system of equations is because arguments call for
the lag between cause and effect to be shorter than the forecast horizon.
Forecast the causal variables are needed and estimating VECM will automatically
provide them. We initially estimate equation in level not in first differences
because it's often possible to find a group of variables that is stationary
even thought the individual variable are not. Such a group is cointegrating
vector, if the values of two variables tend to move together over time so that
their values are always in the some ratio to each other, then the variables are
cointegrated. This is the desirable long run relationship between a causal and
dependent variables. The value of the causal predicts the value of the
dependent variable but in particular time the prediction is not
er be large. An article that should become classical
1994)49.
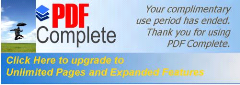
3.3. Forecasting inflation using VECM
An error correction model contains one or more long run
cointegration relationship as seen above but using the VECM will while not
necessary to go back to cointegration concepts is helpful in making the
connection between a VAR with variables in levels the error correction form and
a VECM with variable in differences. From a theory standpoint the parameters of
the system will be estimated consistently and even if the true model is in
differences, hypothesis tests based on an equation in levels will have the same
distribution as if the correct model had been used.
3.3.1 Why a VECM?
Because any exercise in empirical macroeconomic must recognize
the conclusion drawn from times series analyses of macroeconomic data, and
utilize specifications that are consistent with these results. Such analyses
starting with the classic study of Nelson and Plosser (1982), consistently have
demonstrated that macroeconomic time series data likely include a component
generated by permanent or nearly permanent shocks. Such data series are said to
be integrated, difference stationary, or to contain unit roots. On the other
hand, economic theories suggest that some economic variables will not drift
independently of each other forever, but ultimately the difference or ratio of
such variables will revert to a mean or a time trend50.
Granger defined variables that are individually driven by
permanent shocks (integrated), but for which there are weighted sums (linear
combinations) that are mean reverting (driven only by transitory shocks), as
cointegrated variables. He then demonstrated in the Granger Representation
Theorem (Engle and Granger, 1987; Johansen, 1991) that variables, individually
driven by permanent shocks, are
49 Murray, M. P(1994),
«A drunk and her dog: An illustration of cointegration and error
correction,» American Statistician, 48, 37-39.
50 Klein, L.B. and B. F. Kosobud (1961), «Some
Econometrics of Growth: Great Batios of Economics», Quarterly Journal
of Economics, 75:173-98.

xists a Vector Error Correction representation of the
3.3.2. Forecasting performance
While the cointegrating vectors determine the steady-state
behavior of the variables in the Vector Error Correction Model, the dynamic
responses of each of the variables to the underlying permanent and transitory
shocks are completely determined by the sample data without any restriction.
Forecasting performance may be gauged in a number of different
ways. Papers by Clements and Hendry (1993) and Hoffman and Rasche (1996b)
employ measures of system performance, while Clements and Hendry (1993) and
Christofferson and Diebold (1996) argue that conventional RMSE criterion may
not capture some of the advantages of long-run information into the system. The
basic conclusion of this body of literature is that incorporating cointegration
may improve forecast performance, but improvement need not show up only at
longer horizons as predicted originally by Engle and Yoo (1987). The advantage
presumably accrues from the addition of error correction terms in VECM
representations. Christofferson and Diebold (1996) contend that conventional
RMSE criterion will not capture this forecast advantage at long forecast
horizons simply because the importance of the error correction term diminishes
with increases in the forecast horizon. For the exercise we have in mind, the
relevant issue is forecast performance for a subset of the variables in our
system, at various horizons, and the most relevant measure of that performance
is the standard mean squared error criterion. We employ RMSFE as a criterion
while recognizing that it may not capture all the advantages that the long-term
information has to offer.
The results for VECM are in the following table 16
The results show that the fluctuations of price level are
positively related to previews price level and the mark up but negatively
related to nominal GDP, exchange rate, the interest rate and the excess money
supply.
51 Engle, R.F. and C.W.J. Granger (1987),
«Cointegration and Error Correction: Representation, Estimation, and
Testing» Econometrica, 55:251-276.
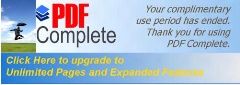
esponse of shocks on errors for all variables. For
al to 2 standard deviation of errors. The temporal Horizon for
response is set at 10 quarterly, this horizon represent the time needed in
which the variable recover the long run level.
Figure 13: Response function of variables on
LIPC
Response of LIPC to Cholesky Response of LMARKUP to Cholesky
Response of LEXCESS_MONEY to Cholesky
One S.D. Innovations One S.D. Innovations One S.D. Innovations
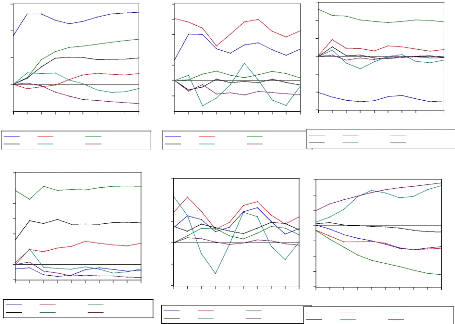
Response of LM2 to Cholesky
LPIBC LTCUSD
One S.D. Innovations
Response of LPIBC to Cholesky One S.D. Innovations
LIPC LMARKUP LEXCESS_MONEY
LM2
Response of LTCUSD to Cholesky One S.D. Innovations
1 2 3 4 5 6 7 8 9 10
1 2 3 4 5 6 7 8 9 10
1 2 3 4 5 6 7 8 9 10
.08
.06
.04
.02
.00
-.02
-.04
.06
.04
.02
.00
-.02
-.04
-.06
.10
.03
.02
.01
.00
-.01
LIPC LMARKUP LEXCESS_MONEY
LM2 LPIBC LTCUSD
LIPC LMARKUP LEXCESS_MONE
LM2 LPIBC LTCUSD
1 2 3 4 5 6 7 8 9 10
1 2 3 4 5 6 7 8 9 10
.06
.05
.04
.03
.02
.01
.00
-.01
.06
.04
.02
.00
-.02
-.04
-.01
-.02
-.03
-.04
.03
.02
.01
.00
1 2 3 4 5 6 7 8 9 10
LIPC LMARKUP LEXCESS_MONE
LM2 LPIBC LTCUSD
LIPC LMARKUP LEXCESS_MONEY
LM2 LPIBC LTCUSD
LIPC LMARKUP LEXCESS_MONEY
LM2 LPIBC LTCUSD
The shock is positive on LIPC generated by the negative effect
of money supply, exchange rate, interest rate, and nominal GDP as seen above
(seen figure 14) then the shock is negative to LIPC generated to positive
effect of previews price level and mark up the whole fructuation on any
variable have a significant impart on price level the figure above shows how
change is made by mark up, excess money supply, money demand and GDP every
period.
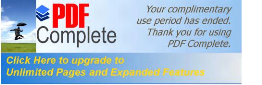
ition
The aim of decomposition of the variance of errors of
prevision is to calculate for each innovation have as contribution on the
errors variance expressed in percentage, when the innovation explain the big
part in variance of error we conclude that the considered economy is sensible
on shock which affect the series seen the appendix 5. The decomposition of the
variance show that the variance of error prevision of the LIPC is due to 80% on
its own innovation, 1% of the mark up innovation,10% of excess money supply
innovation, 7% of M2 innovation ,1% of GDP innovation and 2% of exchange rate
innovation.
The variance of error of the others variable is represented on
the appendix 5 all of them show the accuracy.
.3.3.2.2 Forecast results
The forecast was produced using the data available through the
first quarter of 1995. Table 16 provides the numeric forecasts both
quarter-by-quarter and on an annual basis. The annual values for the levels of
real GDP, nominal M2, and the two interest rate series are four-quarter
averages. The annual values for the inflation rates and growth rates are
measured on a fourth-quarter to fourth-quarter basis. Real growth for the third
quarter of 2009 is forecast to be very slow, indeed to decline from the rate of
2010.1. In retrospect we know that this was a really large forecast error,
since the third quarter of 2009 came in exceptionally strong. The forecast is
for continued slow real growth (< 1 percent per quarter) though the end of
2010. This certainly will be an underestimate of real growth for 2009, and
appears at this time (January, 2010) to be an underestimate of real growth for
2010.
In addition, the forecast did not catch the large increase in
long rates and the accompanying increase in the slope of the yield curve that
began in the last part of the 2010:1 and continued through third quarterly. In
comparison, the money supply (M2) is projected to remain essentially constant
through 2009 (around 6.10 percent), not far from the actual experience. Thus
the long-term rate forecast errors in 2010 are attributable to errors in the
implicit short-run term structure relationship. Taken literally, the model is
forecasting a reduction in the exchange rate target to around
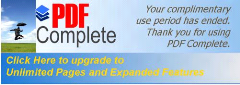
10. Finally, the model projects no change in the
o inflation rates (at roughly 2.5 percent per annum in terms
of the CPI inflation rate) through the end of 2010. Hence the model is not
predicting any increase in the long-run rate of inflation over this period.
Table 15: Prevision results
period
|
ipc
|
Lmarkup
|
Lexcess money
|
Lm2
|
lpibc
|
ltcusd
|
2009 q3
|
0.648560
|
0.184016
|
0.200919
|
6.078984
|
29.08976
|
6.370031
|
2009q4
|
0.538254
|
0.016532
|
0.262129
|
6.431286
|
29.28959
|
6.331708
|
2010q1
|
0.564918
|
0.297554
|
0.464941
|
6.664537
|
28.93297
|
6.303760
|
2010q2
|
0.621301
|
0.263436
|
0.607381
|
6.931436
|
29.97537
|
6.221759
|
2010q3
|
0.886635
|
0.114588
|
0.73921
|
6.506427
|
29.03635
|
6.295411
|
2010q4
|
0.701047
|
0.234921
|
0.499361
|
6.863611
|
29.95079
|
6.204930
|
The table15 above show the prevision of future variables and
demonstrate how change will have the price level due to change of other
variables here the variable are in percentage change. For example to comparing
two period in 2nd quarterly of 2009 inflation was 185.30 expressed
as CPI and 3rd quarterly the price level is 185.75 mean the
evolution of 0.65% per/quarterly, the price level in 4th quarterly
will be 186.04 mean evolution of 0.54%, the price level of 1st
quarterly 2010 will be 186.38 mean evolution of 0.62% per quarterly, in the
2nd quarterly of 2010 the price level will be 186.86 mean evolution
of 0.89% per period and the 3rd quarterly price level will be 187.23
mean the evolution of 0.7% per period. These fluctuations are results of demand
shocks on one hand and the supply shocks on other.
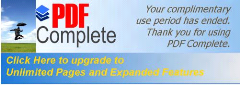
model for forecasting because there are weighted sums (linear
combinations) that are mean reverting (driven only by transitory shocks), as
cointegrated variables and each variables is individually driven by permanent
shocks, are cointegrated if and only if there exists a Vector Error Correction
representation of the data series Nelson and Plosser (1982). The VECM has
predicted inflation reasonably well over history and still appears to be a good
forecasting model, especially in light of modifications like using adjusted,
identifying policy shocks, and deriving probabilities for inflation
outcomes.
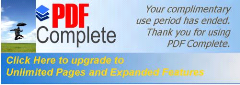
The Rwanda inflation is driven in great part by the increase
in money supply as it have been seen above but the excess money supply doesn't
have the direct impact on price level its act through the exchange rate, and
interest rate channel. These results provide useful insights into the behavior
of inflation in Rwanda and the role of the National Bank of Rwanda in its
determination. Inflation appears to be driven by both foreign and domestic
factors in a manner consistent with conventional theoretical models and our
understanding of the institutional structure of Rwanda economy. The results
suggest that maintaining low inflation in coming years will depend largely on
low level of interest rate, import, oil products in partner's countries and
moderate growth of money supply and domestic unit labour costs. We have
analyzed the inflation in two ways; first by the demand side as demand push
inflation and on other hands by the supply side as cost push inflation.
1. On demand side for its part, the Rwanda national bank will
need to maintain monetary conditions consistent with low inflation and low
inflation expectations. In part, this is done through the pegged exchange rate,
which acts as a nominal anchor for the economy. In this paper we wanted to
analyze the driving forces of inflation process in Rwanda as a transition
economy. First we derived, according to the theory, long-run sectoral
relationship affecting inflation (markup, excess money, nominal exchange rate
and GDP). Then we estimated short-run structural inflation function by imposing
above mentioned long-run relationships together with various short-run
variables which might contribute to explaining the inflation process in Rwanda,
we conclude that inflation in Rwanda rise when money supply increase this by
the increase in aggregate demande due also by the increased private and
government spending.
2. On the supply side the mark up is seen to be significant
because the increase of oil price, and import price increase at the sime time
and immediately the price of domestic goods. As economic policy which is
liberalized the producers to whom the oil is part of their cost could then pass
(this change in price level) on to consumers in the form of increased price. In
the derive quarterly ahead model of inflation, we found that all long run
relationships except GDP significantly influence quarter-on-quarter
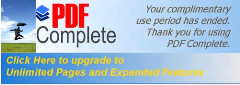
monetary variables are found to be important in
ar as narrow monetary aggregate is concerned, one can notice
that the magnitude of its influence on consumer price inflation is quite
marginal.
For forecast purpose we designed ECM model first by Hendry
equations is not a perfect one, secondly by Engle Granger which proven instable
by forecast criteria this is because of a high number of parameters estimated,
while the definability of some equations is relatively high (a low relative
coefficient of determination). However, they may be applied to the forecasting
of inflation because the mean square errors of the forecast conform to the
selected minimum criteria (1 or 5% should be mentioned). One of the main
drawbacks of the VECM model, where the VAR methodology is used, is the fact
that each time additional observations appear and the model is estimated as new
equation of each group may be complemented (or reduced) by different variables.
However, given stable parameters, the accuracy of results is not damaged.
Nevertheless, the designing of a structural VAR or multi-equation econometric
model for the forecasting of the CPI should probably be considered in
future.
The VECM has predicted inflation reasonably well over history
and still appears to be a good forecasting model, especially in light of
modifications like using adjusted, identifying policy shocks, and deriving
probabilities for inflation outcomes. Forecasts from the VECM can augment the
information coming from other models used at the Bank. They can provide
alternative views of what could happen in the economy and give some information
about the «balance of risks.» Multiple models could be especially
helpful to policy-makers during times of extreme uncertainty and/or structural
shifts, but even in relatively stable times, advice from different models helps
to balance risks about the outlook for the future.
According to the accuracy of the forecast calculated by the VECM
model, this model could be suggested as a tool applied by the
economists-analysts in the decision making procedure.
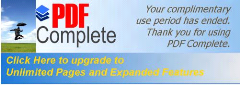
Some caveats are in order. First, the approach in this paper
has been to evaluate forecasting performance using a simulated out of sample
methodology. This methodology provides a degree of protection against
overfitting and detects model instability. However, because a large number of
forecasts were used, some over fitting bias nonetheless remains. This suggests
that some of the best-performing forecasts produced using individual economic
indicators might deteriorate as one move beyond the end of our sample. That is
why the model used here could be improved in the future because;
- Coefficients of predictors can change over time
- The number of predictors can be large
- The relevant model for forecasting can potentially change over
time
- The variables can also change over time
Second, we have considered only linear models. To the extent
that the relation between inflation and some of the candidate variables is
nonlinear, these results understate the forecasting improvements that might be
obtained. Moreover, with few exceptions, incorporating other variables could be
necessary to improve upon the short run forecasts and must be considered by
National Bank agent in their future research.
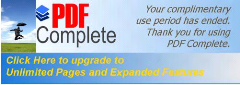
1. Adam Smith. (1776), «Wealth of
nations»
2. Ando, A. and F. Modigliani (1963), «The
`Life-Cycle' Hypothesis of Saving: aggregate Implications and Tests»,
American Economic Review.
3. Banerjee, A., J. Dolado, J.W. Galbraith and D.H.
Hendry (1993). Co-integration, Error-Correction, and the Econometric
Analysis of Non-Stationary Data, Oxford University Press, Oxford.
4. Bewley, R.A. (1979). The Direct Estimation
of the Equilibrium Response in a Linear Dynamic Model, Economic Letters.
5. Bomhoff, E.J. (1991). Inflation in Western
Europe, paper prepared for the Fifth International Conference sponsored by the
Institute for Monetary and Economic Studies, Bank of Japan, October.
6. Campbell, J.Y. and R.J. Shiller
(1987),»Cointegration and tests of Present value Models»
Journal of political Economy, 95
7. Duesenberry, J. (1950). The Mechanics of
Inflation, Review of Economics and Statistics, 32 (2).
8. Edwards, Sebastian. (2002), «The great
exchange rate debate after Argentina», The North American Journal of
Economics and finance, Volume 13, Issue 3
9. Engle, R.F. and C.W.J. Granger (1987),
«Cointegration and Error Correction: Representation, Estimation,
and Testing» Econometrica, 55
10. Gary K. and Dimitris K, (2009), Forecasting
Inflation Using Dynamic Model Averaging University of Strathclyde, June 2009
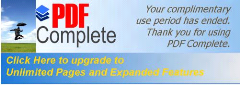
Principle of forecasting; A handbook for
A
12. Kiley, Michel J. (2008).
«Estimating the common trend rate of inflation for consumer
prices and consumer prices excluding food and energy prices». Federal
reserve Board.
13. Klein, L.R. and R. F. Kosobud (1961),
«Some Econometrics of Growth: Great Ratios of Economics»,
Quarterly Journal of Economics, 75
14. Kremers, J.J.M., N.R. Ericsson and J.J. Dolado
(1992). The Power of Cointegration Tests, Oxford Bulletin of Economics
and Statistics, 54 (3)
15. Mankiw, N.G.(1998) «Economic
principles». New York: university Publishers,de Croisillon de Harcourt.
16. Mishkin, F. S. (1992), Is the Fisher effect
for real: A reexamination of the relationship between Inflation and Interest
rates, Journal of Monetary Economics 30
17. Murray, M. P(1994), «A drunk and her
dog: An illustration of cointegration and error correction,» American
Statistician, 48
18. Paul H. Walgenbach, Norman E. Dittrich and Ernest I.
Hanson,(1973).»The Measuring Unit principle»
19. Payne, James E., (2002), «Inflationary
Dynamics of a Transition Economy: the Croatian Experience», Journal of
Policy Modeling, 24(3),
20. Sargent, Thomas J. (1986), «Rational
Expectations and Inflation», New York; Harper and Row.
21. Selgin, G.A,(1989) «The analytical
Framework of real bill Doctrine» Journal of institutional
and theoretical economics, Volume 145,
22. Stock J. and Watson M. (1999), Forecasting
inflation. Journal of Monetary Economics 44,
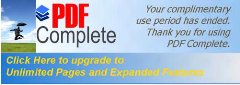
2001) : Research methodology course for the es ,
2nd edition , Paris, Durod
Working Papers
1. Bandawe, H.P. (1997). Causes of Inflation in
Fiji: Experience during 1979-1994, working Paper, Reserve Bank of Fiji.
2. Brower, G. and N.R. Ericsson (1995).
Modeling Inflation in Australia, Reserve Bank of Australia, Research
discussion Paper No. 9510
3. Debelle, G. and G. Stevens (1995). Monetary
Policy Goals for Inflation in Australia, Reserve Bank of Australia, Research
Discussion Paper No. 9530.
4. Jean Claude Trichet, (2004), Federal reserve
board's semiannual Monetary Policy Report to the congress round table, July 1,
2004
5. Morling, S. (1997). Modeling Inflation in
Fiji, Working Paper, Reserve Bank of Fiji.
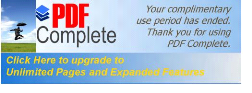
APPENDIX 1: DATA DEFINITIONS
This appendix describes the data. The list the definitions of the
data and give their sources. All data are quarterly, and the sample period is
1995(1)-2009(2).
2. Consumer Price Index (IPC)
Definition: The Consumer Price Index is a
Laspeyres index that covers household consumption as it is used by national
accounts. The reference population for the CPI consists of all households
living in urban areas in Rwanda. The household basket includes 438 products
observed in many places spread all over the administrative centers of all
provinces in Rwanda. All kinds of places of observation are selected: shops,
markets, services, etc. More than 25,000 prices are collected every month by
enumerators of the National Institute of Statistics of Rwanda and of the
National Bank of Rwanda. The base year for the CPI is the year 2003. The
weights used for this new index are the result of the Household Living
Conditions Survey (EICV) conducted in 2000-2001 with a sample of 6,450
households. The splicing with the old index is feasible using the splicing
coefficient of 3.889. If you divide the old index by this coefficient, you will
be able to make comparisons with the new index based in 2003.
The consumer price index is calculated by the RNIS and published
every quarterly the base is 2003=100 (NISR) is adjusted.
Source: National Institute of Statistics of
Rwanda (NISR), P.O. BOX 6139 Kigali,
Tel.: (250) 750545 Fax: (250) 575719, Web site:
www.statistics.gov.rw /CPI
Indexes
3. Unit Labour Costs (ULC)
Definition: Nominal cost of all labour per unit
of output.
Nominal unit labour costs are defined as:
salaries + payroll taxes - employment subsidies divided by gross
domestic product Where salaries refers to the wages, salaries and supplements
of all employee in public and private sector including volontaries wage and
salary earners. The class «wage and salary earners» is only a subset
of all employed people in the economy,

f-employed, employers. Unit labour costs of wage
scaled up to that by adding the 5% as legal percentage of ratio
payed in national social security fund( NSSF).
Source: Author calculation using then NSSF data.
4. Import Prices (IP)
Definition: The NBR publish every month the
quantity of goods imported and they values, using these data we calculate the
import price index of merchandise imports, excluding without the oil import
items. Import prices are measured as the implicit price on seasonally adjusted
merchandise imports, excluding exogenous imports. Exogenous imports are goods
which are lumpy in nature, subject to government arrangements or significantly
affected by factors other than the general level of economic activity in
Rwanda. Specifically, this covers fuel, defense equipment, and ships, aircraft
and other large items of equipment acquired by selected public and private
enterprises.
The monthly data are adjusted on quarterly basis using the
moving average, Author calculation
Source: NBR, Foreign Exchange Inspection and Balance of Payments
Department
5. Petrol Prices (PP)
Definition: Automotive fuel price index or oil
price index.
On the monthly basis the NBR publish the quantity and the
values of energy and lubricant which include piles and electrics accumulators,
fuels, gaz oils, lubricating oils and others fuels products using these
available data we calculate the oil price index on Layperes basis and finally
adjusted on quarterly.
Source: NBR, Foreign Exchange Inspection and Balance of Payments
Department 5. M2
Definition: M2 is the monetary base which
include the currency in circulation plus commercial bank reserves at the
central bank this mean M1( currency in circulation
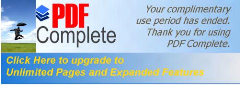
nd saving deposit which is published by the national s deflated
using the current CPI.
Source: NBR, Research department.
6. The exchange rate (TCUSD)
Definition: The nominal exchange rate used
in the study is defined as unit of Rwanda franc per United States dollar. The
basic calculation is made by dairly basis and later adjusted on the quarterly
base.
Source: NBR, Foreign Exchange Inspection and Balance of Payments
Department
7. Interest rate (TDC)
Definition: The interest rate represents the
spread between the 91-day treasury bills and the average annual interest
offered on time and saving deposit and this spread represent the return on
domestic financial assets. The interest rate of central has been used as the
measure of the spread.
Source: NBR, Foreign Exchange Inspection and Balance of Payments
Department
8. The nominal GDP
The GDP represent the national output is published on the year
basis we estimated it using the eviews to get the quarterly data. This involves
adjusting a linear interpolation of annual GDP series with weigthed percentage
errors between actual quarterly data. Then the resulting was seasonally
adjusted to minimize the impact of the cyclical components.
PIB
|
ULC
|
IP
|
TCUSD
|
OIL PRICE INDEX
|
72.90
|
0.0064
|
1082168
|
172.99
|
341.84
|
81.60
|
0.0063
|
810447
|
273.42
|
347.02
|
89.00
|
0.0062
|
1265720
|
312.94
|
339.49
|
95.50
|
0.0069
|
1092288
|
304.43
|
439.08
|
96.10
|
0.0072
|
986104
|
305.16
|
315.97
|
102.20
|
0.0075
|
3478570
|
307.14
|
339.08
|
109.00
|
0.0072
|
663810
|
306.94
|
315.97
|
116.70
|
0.0072
|
606585
|
307.42
|
315.89
|
129.70
|
0.0065
|
282688
|
305.35
|
318.2
|
137.00
|
0.0062
|
329202
|
301.67
|
310.61
|
143.20
|
0.0063
|
265033
|
299.65
|
319.95
|
148.30
|
0.0064
|
309131
|
304.13
|
319.95
|
150.90
|
0.0063
|
317448
|
307.25
|
326.39
|
154.30
|
0.0063
|
292589
|
309.30
|
328.44
|
156.90
|
0.0062
|
361544
|
315.44
|
350.65
|
159.10
|
0.0067
|
359701
|
325.17
|
373.87
|
157.50
|
0.0069
|
330049
|
332.73
|
403.09
|
162.20
|
0.0071
|
338890
|
335.24
|
410.89
|
165.50
|
0.0070
|
319282
|
334.18
|
437.56
|
171.10
|
0.0076
|
368840
|
342.67
|
458.61
|
174.80
|
0.0075
|
434809
|
362.87
|
454.38
|
178.20
|
0.0076
|
377019
|
379.39
|
457.77
|
181.50
|
0.0076
|
356082
|
405.45
|
459.32
|
183.10
|
0.0081
|
335797
|
425.22
|
461.17
|
186.50
|
0.0076
|
394560
|
433.65
|
466.04
|
190.20
|
0.0076
|
361482
|
439.91
|
466.43
|
194.30
|
0.0076
|
304842
|
444.86
|
495.12
|
199.20
|
0.0079
|
329135
|
456.52
|
496.18
|
203.80
|
0.0081
|
310087
|
460.78
|
517.58
|
208.40
|
0.0082
|
372846
|
466.90
|
532.32
|
213.40
|
0.0083
|
395299
|
482.01
|
533.5
|
216.00
|
0.0087
|
395426
|
502.01
|
563.25
|
222.20
|
0.0090
|
454943
|
513.80
|
517.2
|
229.30
|
0.0091
|
430441
|
531.69
|
493.6
|
237.60
|
0.0090
|
495160
|
550.98
|
500.8
|
249.70
|
0.0094
|
485623
|
570.24
|
497.0
|
259.10
|
0.0100
|
455757
|
582.43
|
459.5
|
268.10
|
0.0099
|
462887
|
579.73
|
388.3
|
277.20
|
0.0098
|
466906
|
576.23
|
386.9
|
285.40
|
0.0100
|
593191
|
568.84
|
361.7
|
294.60
|
0.0098
|
507757
|
562.92
|
420.4
|
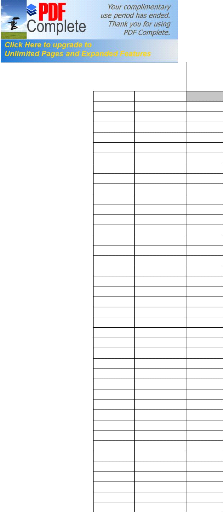
TDC
IPC
PERIOD M2
39.80
|
10.04
|
-
|
49.74
|
12.06
|
61.87
|
59.27
|
12.04
|
70.80
|
62.64
|
12.12
|
71.73
|
62.13
|
9.00
|
70.90
|
64.58
|
9.36
|
71.10
|
66.60
|
9.43
|
74.40
|
68.87
|
11.26
|
76.33
|
74.14
|
8.39
|
77.63
|
84.29
|
9.07
|
78.40
|
81.11
|
8.52
|
81.43
|
88.60
|
8.24
|
88.10
|
85.45
|
9.94
|
88.77
|
82.66
|
9.21
|
89.27
|
84.18
|
9.16
|
86.20
|
91.99
|
7.76
|
84.47
|
102.79
|
7.91
|
84.90
|
103.04
|
9.19
|
83.93
|
107.38
|
8.53
|
85.00
|
102.51
|
8.75
|
86.00
|
103.80
|
9.20
|
86.23
|
109.72
|
9.44
|
86.93
|
107.36
|
9.41
|
88.57
|
119.39
|
10.11
|
91.47
|
122.17
|
9.69
|
92.03
|
127.75
|
10.16
|
91.13
|
125.32
|
9.91
|
90.90
|
130.69
|
10.18
|
91.07
|
129.70
|
10.15
|
91.20
|
135.60
|
10.00
|
91.80
|
135.30
|
9.34
|
93.53
|
144.31
|
9.10
|
95.80
|
143.25
|
8.94
|
96.87
|
145.89
|
9.11
|
98.87
|
158.31
|
9.27
|
100.83
|
167.53
|
9.33
|
103.43
|
162.41
|
8.91
|
107.24
|
161.28
|
9.26
|
109.82
|
167.25
|
9.33
|
113.56
|
187.23
|
9.43
|
117.18
|
190.84
|
13.50
|
120.06
|
1995-1
1996-1
1997-1
1998-1
1999-1
2000-1
2001-1
2002-1
2003-1
2004-1
2005-1
|
|
122.70
|
|
123.39
|
|
122.49
|
220.07
|
8.08
|
128.01
|
237.64
|
8.18
|
132.81
|
254.15
|
8.32
|
134.84
|
285.98
|
8.24
|
136.24
|
273.63
|
7.90
|
143.08
|
301.76
|
7.69
|
144.33
|
326.77
|
7.57
|
145.81
|
375.27
|
7.32
|
146.91
|
422.16
|
7.56
|
152.70
|
445.39
|
8.21
|
163.03
|
465.03
|
10.10
|
174.40
|
468.16
|
9.23
|
179.40
|
468.16
|
7.90
|
181.90
|
468.00
|
8.10
|
185.30
|
303.70
|
0.0099
|
549816
|
556.99
|
320.1
|
313.20
|
0.0095
|
511935
|
554.44
|
230.2
|
322.20
|
0.0099
|
519140
|
553.73
|
371.5
|
332.10
|
0.0108
|
486034
|
553.86
|
506.3
|
342.10
|
0.0109
|
541193
|
552.04
|
364.5
|
352.80
|
0.0118
|
439671
|
551.29
|
345.5
|
358.60
|
0.0125
|
452765
|
550.01
|
328.4
|
354.20
|
0.0135
|
411030
|
547.87
|
399.4
|
371.85
|
0.0136
|
439717
|
546.37
|
357.8
|
387.76
|
0.0133
|
556641
|
547.89
|
444.5
|
403.46
|
0.0137
|
504527
|
545.12
|
605.5
|
419.54
|
0.0138
|
594475
|
543.89
|
574.8
|
517.68
|
0.0118
|
755817
|
543.35
|
645.4
|
500.73
|
0.0126
|
771322
|
547.13
|
574.8
|
451.19
|
0.0150
|
778,849
|
553.01
|
621.7
|
369.12
|
0.0177
|
773299
|
566.00
|
605.5
|
515.22
|
0.0132
|
721312
|
572.30
|
532.0
|
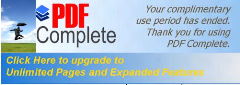
2006-1
2007-1
2008-1
2009-1
2009-2
APPENDIX 3: RESIDUALS PROPERTIES

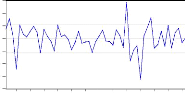
1996 1998 2000 2002 2004 2006 2008
.06
.04
.02
.00
-.02
-.04
-.06
.3
.2
.1
.0
-.1
-.2
-.3
-.4
.10 .05 .00
-.05 -.10 -.15
.2
.1
.0
-.1
-.2
DLPIBC Residuals
DLIPC Residuals
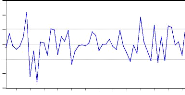
1996 1998 2000 2002 2004 2006 2008
DLTDC Residuals
DLM2 Residuals
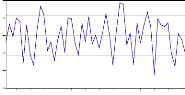
1996 1998 2000 2002 2004 2006 2008
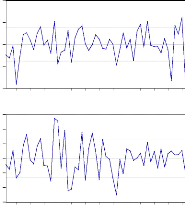
1996 1998 2000 2002 2004 2006 2008
DLTCUSD Residuals
DLULC Residuals
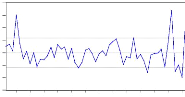
1996 1998 2000 2002 2004 2006 2008
1996 1998 2000 2002 2004 2006 2008
.20 .15 .10
.05 .00
-.05 -.10 -.15
.03
.02
.01
.00
-.01
-.02
-.03
Page 71
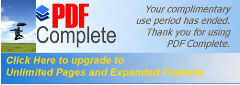
Vector Autoregression Estimates
Date: 11/22/09 Time: 11:49
Sample (adjusted): 1995Q4 2009Q2
Included observations: 55 after adjustments Standard errors in (
) & t-statistics in [ ]
LIPC
LIPC(-1) 1.101540
(0.26175)
[ 4.20837]
LIPC(-2) -0.422070
(0.27787)
[-1.51896]
LMARKUP(-1) -0.056816
(0.03854)
[-1.47435]
LMARKUP(-2) 0.013979
(0.03739)
[ 0.37387]
LEXCESS_MONEY(
-1) -0.083627 (0.13791) [-0.60639]
LEXCESS_MONEY(
-2) -0.027293 (0.14280) [-0.19113]
LM2(-1) 0.111751
(0.14672)
[ 0.76165]
LM2(-2) 0.031747
(0.16028)
[ 0.19807]
LPIBC(-1) 0.089677
(0.06716)
[ 1.33521]
LEXCESS_
LMARKUP MONEY
|
LM2
|
LPIBC
|
LTCUSD
|
2.141111
|
-2.130141
|
-1.578700
|
-1.192560
|
-0.352104
|
(1.26259)
|
(0.84661)
|
(0.65981)
|
(0.84264)
|
(0.17829)
|
[ 1.69581]
|
[-2.51609]
|
[-2.39264]
|
[-1.41526]
|
[-1.97489]
|
-3.151490
|
1.704996
|
1.269049
|
-0.239753
|
0.231334
|
(1.34034)
|
(0.89874)
|
(0.70045)
|
(0.89453)
|
(0.18927)
|
[-2.35127]
|
[ 1.89709]
|
[ 1.81177]
|
[-0.26802]
|
[ 1.22224]
|
0.450103
|
0.170646
|
0.012606
|
0.227207
|
-0.033242
|
(0.18589)
|
(0.12464)
|
(0.09714)
|
(0.12406)
|
(0.02625)
|
[ 2.42140]
|
[ 1.36908]
|
[ 0.12977]
|
[ 1.83144]
|
[-1.26640]
|
0.231217
|
-0.193665
|
-0.092581
|
0.010903
|
-0.035885
|
(0.18035)
|
(0.12093)
|
(0.09425)
|
(0.12037)
|
(0.02547)
|
[ 1.28204]
|
[-1.60144]
|
[-0.98230]
|
[ 0.09058]
|
[-1.40905]
|
0.564419 (0.66523) [ 0.84845]
|
0.032940 (0.44606) [ 0.07385]
|
-0.761326 (0.34764) [-2.18996]
|
-0.568270 (0.44397) [-1.27997]
|
-0.026493 (0.09394) [-0.28202]
|
-1.341830
|
0.298516
|
0.279204
|
-0.394038
|
0.055375
|
(0.68882)
|
(0.46187)
|
(0.35997)
|
(0.45971)
|
(0.09727)
|
[-1.94802]
|
[ 0.64631]
|
[ 0.77563]
|
[-0.85714]
|
[ 0.56931]
|
-0.810507
|
0.660783
|
1.492038
|
0.765855
|
-0.076622
|
(0.70774)
|
(0.47456)
|
(0.36986)
|
(0.47234)
|
(0.09994)
|
[-1.14521]
|
[ 1.39241]
|
[ 4.03410]
|
[ 1.62141]
|
[-0.76668]
|
1.375501
|
-0.332027
|
-0.249299
|
0.523881
|
0.027241
|
(0.77313)
|
(0.51841)
|
(0.40403)
|
(0.51598)
|
(0.10917)
|
[ 1.77914]
|
[-0.64047]
|
[-0.61704]
|
[ 1.01531]
|
[ 0.24952]
|
-0.013350
|
0.295970
|
0.442492
|
0.448134
|
0.010639
|
(0.32397)
|
(0.21723)
|
(0.16930)
|
(0.21622)
|
(0.04575)
|
[-0.04121]
|
[ 1.36245]
|
[ 2.61359]
|
[ 2.07261]
|
[ 0.23257]
|
LTCUSD(-1) -0.281546
(0.14066)
[-2.00166]
LTCUSD(-2) 0.222899
(0.13707)
[ 1.62619]
C -1.676818
(1.69667)
[-0.98830]
R-squared 0.996371
Adj. R-squared 0.995334
Sum sq. resids 0.013769
S.E. equation 0.018106
F-statistic 960.8409
Log likelihood 150.0073
Akaike AIC -4.982082
Schwarz SC -4.507621
Mean dependent 0.043181
S.D. dependent 0.265052
-0.016498
|
-0.358248
|
-0.444169
|
-0.358673
|
0.176711
|
(0.43672)
|
(0.29283)
|
(0.22822)
|
(0.29146)
|
(0.06167)
|
[-0.03778]
|
[-1.22338]
|
[-1.94619]
|
[-1.23059]
|
[ 2.86547]
|
-0.974949
|
0.733304
|
0.431368
|
0.233600
|
1.218535
|
(0.67847)
|
(0.45494)
|
(0.35456)
|
(0.45281)
|
(0.09581)
|
[-1.43697]
|
[ 1.61187]
|
[ 1.21662]
|
[ 0.51589]
|
[ 12.7186]
|
0.800388
|
-0.862067
|
-0.552112
|
-0.127980
|
-0.311163
|
(0.66117)
|
(0.44334)
|
(0.34552)
|
(0.44126)
|
(0.09336)
|
[ 1.21056]
|
[-1.94450]
|
[-1.59792]
|
[-0.29003]
|
[-3.33279]
|
-0.864729
|
0.918994
|
-0.389253
|
18.86430
|
-4.492430
|
(8.18413)
|
(5.48774)
|
(4.27694)
|
(5.46204)
|
(1.15568)
|
[-0.10566]
|
[ 0.16746]
|
[-0.09101]
|
[ 3.45371]
|
[-3.88725]
|
0.737300
|
0.627502
|
0.995287
|
0.986779
|
0.998160
|
0.662242
|
0.521074
|
0.993941
|
0.983002
|
0.997634
|
0.320365
|
0.144041
|
0.087492
|
0.142695
|
0.006388
|
0.087337
|
0.058562
|
0.045641
|
0.058288
|
0.012333
|
9.823162
|
5.896025
|
739.2045
|
261.2321
|
1898.408
|
63.46310
|
85.44554
|
99.15586
|
85.70373
|
171.1258
|
-1.835022
|
-2.634383
|
-3.132941
|
-2.643772
|
-5.750031
|
-1.360561
|
-2.159923
|
-2.658480
|
-2.169312
|
-5.275570
|
0.014606
|
-0.001208
|
5.025937
|
28.44066
|
6.090165
|
0.150278
|
0.084622
|
0.586355
|
0.447072
|
0.253544
|
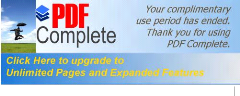
[-0.47015]
Determinant resid covariance
|
|
(dof adj.)
|
4.13E-19
|
Determinant resid covariance
|
8.19E-20
|
Log likelihood
|
740.3481
|
Akaike information criterion
|
-24.08538
|
Schwarz criterion
|
-21.23862
|
Appendix 5: Variance decomposition of VECM
Variance Decomposition of LIPC: Perio
d S.E. LIPC LMARKUP
|
LEXCESS _ MONEY
|
LM2
|
LPIBC
|
LTCUSD
|
1
|
0.018297
|
100.0000
|
0.000000
|
0.000000
|
0.000000
|
0.000000
|
0.000000
|
2
|
0.032540
|
96.90690
|
0.261702
|
0.595329
|
0.499544
|
1.721004
|
0.015519
|
3
|
0.043520
|
90.62316
|
0.191614
|
4.832142
|
2.512064
|
1.817140
|
0.023875
|
4
|
0.052351
|
83.63004
|
0.136518
|
8.777639
|
5.243455
|
1.894989
|
0.317361
|
5
|
0.059778
|
78.48380
|
0.195292
|
12.07487
|
6.924228
|
1.525553
|
0.796257
|
6
|
0.066923
|
75.02531
|
0.419849
|
14.20681
|
7.772816
|
1.218449
|
1.356770
|
7
|
0.074009
|
72.88328
|
0.648207
|
15.70749
|
7.907380
|
1.077777
|
1.775871
|
8
|
0.081031
|
71.31248
|
0.750457
|
16.86669
|
7.916372
|
1.034312
|
2.119692
|
9
|
0.087759
|
70.04459
|
0.799915
|
17.85948
|
7.905447
|
0.980837
|
2.409730
|
74863 18.69154 7.959918 0.875919 2.673639
RKUP:
LEXCESS
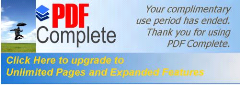
MONEY LM2 LPIBC LTCUSD
Perio
d S.E. LIPC LMARKUP
_
1
|
0.085733
|
10.12798
|
89.87202
|
0.000000
|
0.000000
|
0.000000
|
0.000000
|
2
|
0.131525
|
25.71178
|
72.02151
|
0.006302
|
0.899849
|
0.264277
|
1.096287
|
3
|
0.163858
|
29.98207
|
63.86561
|
0.275853
|
0.849396
|
4.217105
|
0.809964
|
4
|
0.177746
|
30.92442
|
60.75896
|
0.698480
|
0.730917
|
5.229595
|
1.657623
|
5
|
0.192141
|
29.93022
|
62.02403
|
0.724407
|
0.649359
|
4.550724
|
2.121260
|
6
|
0.214214
|
28.82513
|
62.77342
|
0.613363
|
0.525383
|
4.773778
|
2.488929
|
7
|
0.234401
|
28.50856
|
63.99687
|
0.619720
|
0.452586
|
3.987878
|
2.434389
|
8
|
0.248487
|
27.96734
|
63.66144
|
0.782854
|
0.407589
|
4.605363
|
2.575415
|
9
|
0.259852
|
27.17995
|
63.00427
|
0.842011
|
0.383605
|
5.795693
|
2.794468
|
10
|
0.271840
|
27.12109
|
63.31215
|
0.786374
|
0.399906
|
5.369609
|
3.010871
|
Variance Decomposition of LEXCESS_MONEY:
Perio
d
|
S.E.
|
LIPC
|
LMARKUP
|
LEXCESS _ MONEY
|
LM2
|
LPIBC
|
LTCUSD
|
1
|
0.066526
|
37.38368
|
0.041603
|
62.57472
|
0.000000
|
0.000000
|
0.000000
|
2
|
0.095655
|
40.93250
|
3.918057
|
53.35775
|
1.232881
|
0.537617
|
0.021192
|
3
|
0.117338
|
44.87656
|
3.191635
|
50.20760
|
0.825644
|
0.747469
|
0.151092
|
4
|
0.135264
|
47.85993
|
2.807033
|
46.95173
|
0.631856
|
1.615467
|
0.133980
|
5
|
0.149603
|
50.12986
|
2.460321
|
45.22608
|
0.531474
|
1.481649
|
0.170621
|
6
|
0.161253
|
50.98821
|
2.647058
|
44.45449
|
0.484415
|
1.275346
|
0.150483
|
7
|
0.171989
|
51.31621
|
2.706138
|
44.27728
|
0.428598
|
1.134399
|
0.137376
|
8
|
0.183281
|
51.89167
|
2.584419
|
43.92870
|
0.377480
|
1.096480
|
0.121256
|
9
|
0.194634
|
52.81078
|
2.376649
|
43.25861
|
0.336331
|
1.102984
|
0.114654
|
10
|
0.204847
|
53.63574
|
2.288540
|
42.62055
|
0.306779
|
1.039664
|
0.108725
|
Variance Decomposition of LM2: Perio
d S.E. LIPC LMARKUP
|
LEXCESS _ MONEY
|
LM2
|
LPIBC
|
LTCUSD
|
1
|
0.051043
|
0.350690
|
0.055566
|
89.42647
|
10.16727
|
0.000000
|
0.000000
|
2
|
0.073794
|
0.264612
|
1.755991
|
76.19698
|
19.86784
|
1.871757
|
0.042818
|
3
|
0.094336
|
0.678710
|
1.849955
|
75.90141
|
20.13481
|
1.184020
|
0.251098
|
4
|
0.111029
|
0.990596
|
2.250991
|
73.81612
|
21.58103
|
0.911013
|
0.450254
|
5
|
0.125225
|
1.091928
|
2.660878
|
73.37158
|
21.37689
|
0.776895
|
0.721820
|
6
|
0.137984
|
0.961006
|
3.373420
|
72.87852
|
21.27225
|
0.652282
|
0.862522
|
7
|
0.150007
|
0.835199
|
3.709558
|
72.80418
|
21.07802
|
0.593375
|
0.979670
|
8
|
0.161627
|
0.761029
|
3.813367
|
72.71875
|
21.01232
|
0.633132
|
1.061403
|
9
|
0.172552
|
0.731259
|
3.834466
|
72.64545
|
20.98426
|
0.636342
|
1.168230
|
10
|
0.182762
|
0.693408
|
3.985485
|
72.54635
|
20.92388
|
0.593409
|
1.257465
|
Variance Decomposition of LPIBC:
Perio
d S.E. LIPC LMARKUP
LEXCESS
MONEY LM2 LPIBC LTCUSD
_
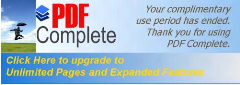
22762 29179 04580
4 0.098952 14.50754 37.38808
5 0.102642 15.49777 38.18783
6 0.116172 18.32080 38.82996
7 0.130118 20.95032 39.69009
8 0.136354 21.16591 39.71859
9 0.140460 20.28022 39.00492
10 0.143889 20.17289 40.02714
0.000290
|
7.565386
|
59.66085
|
0.000000
|
0.689948
|
5.504930
|
39.01509
|
0.361021
|
2.706326
|
7.924112
|
31.11456
|
0.446975
|
4.071785
|
8.757723
|
34.88760
|
0.387276
|
4.196817
|
9.282737
|
32.44972
|
0.385130
|
3.372594
|
7.782297
|
31.39360
|
0.300751
|
3.178140
|
7.329111
|
28.57389
|
0.278452
|
4.142156
|
8.617926
|
26.08331
|
0.272105
|
4.870003
|
9.756058
|
25.82769
|
0.261103
|
4.902321
|
10.00941
|
24.61460
|
0.273639
|
Variance Decomposition of
LTCUSD:
Period LEXCESS
S.E. LIPC LMARKUP MONEY LM2 LPIBC LTCUSD
_
1
|
0.011168
|
0.173076
|
7.267890
|
8.255018
|
1.279036
|
4.236467
|
78.78851
|
2
|
0.022223
|
1.053871
|
11.07978
|
18.00470
|
1.116873
|
7.527846
|
61.21693
|
3
|
0.035166
|
3.220732
|
13.36857
|
23.19984
|
0.463252
|
12.33115
|
47.41645
|
4
|
0.050203
|
4.226496
|
10.96903
|
26.08153
|
0.228007
|
20.42038
|
38.07456
|
5
|
0.065036
|
4.829875
|
9.019364
|
27.59094
|
0.143855
|
24.71258
|
33.70338
|
6
|
0.078331
|
5.752720
|
8.409146
|
29.00032
|
0.109422
|
24.39696
|
32.33143
|
7
|
0.090758
|
6.952437
|
8.811006
|
30.28924
|
0.113849
|
22.24306
|
31.59041
|
8
|
0.103117
|
7.713250
|
9.178553
|
31.46720
|
0.170577
|
20.71041
|
30.76000
|
9
|
0.115532
|
7.744092
|
9.075099
|
32.37502
|
0.258851
|
20.64557
|
29.90137
|
10
|
0.127537
|
7.499270
|
8.803140
|
32.89073
|
0.319129
|
21.15154
|
29.33619
|
Cholesky Ordering: LIPC LMARKUP LEXCESS_MONEY LM2 LPIBC
LTCUSD
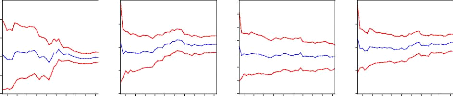
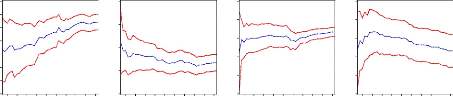
1.5 1.0 0.5 0.0 -0.5
-1.0
00 01 02 03 04 05 06 07 08

Recursive C(1) Estimates #177; 2 S.E.
.05 .00 -.05 -.10 -.15 -.20 -.25
-.30
00 01 02 03 04 05 06 07 08
.2 .0 -.2 -.4
-.6
00 01 02 03 04 05 06 07 08

Recursive C(2) Estimates #177; 2 S.E.
.6 .4 .2 .0
-.2
00 01 02 03 04 05 06 07 08
.25 .20 .15 .10 .05 .00 -.05
-.10
00 01 02 03 04 05 06 07 08

Recursive C(3) Estimates #177; 2 S.E.
.2 .1 .0
-.1
-.2
-.3
00 01 02 03 04 05 06 07 08
0.4 0.0 -0.4 -0.8
-1.2
00 01 02 03 04 05 06 07 08

Recursive C(4) Estimates #177; 2 S.E.
.6 .4 .2 .0 -.2
-.4
00 01 02 03 04 05 06 07 08

Recursive C(5) Estimates #177; 2 S.E.

Recursive C(6) Estimates #177; 2 S.E.

Recursive C(7) Estimates #177; 2 S.E.

Recursive C(8) Estimates #177; 2 S.E.
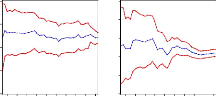
.8 .4 .0 -.4
-.8

Recursive C(9) Estimates #177; 2 S.E.
00 01 02 03 04 05 06 07 08
.3 .2 .1 .0
-.1
-.2

Recursive C(10) Estimates #177; 2 S.E.
00 01 02 03 04 05 06 07 08
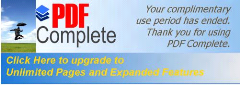
|